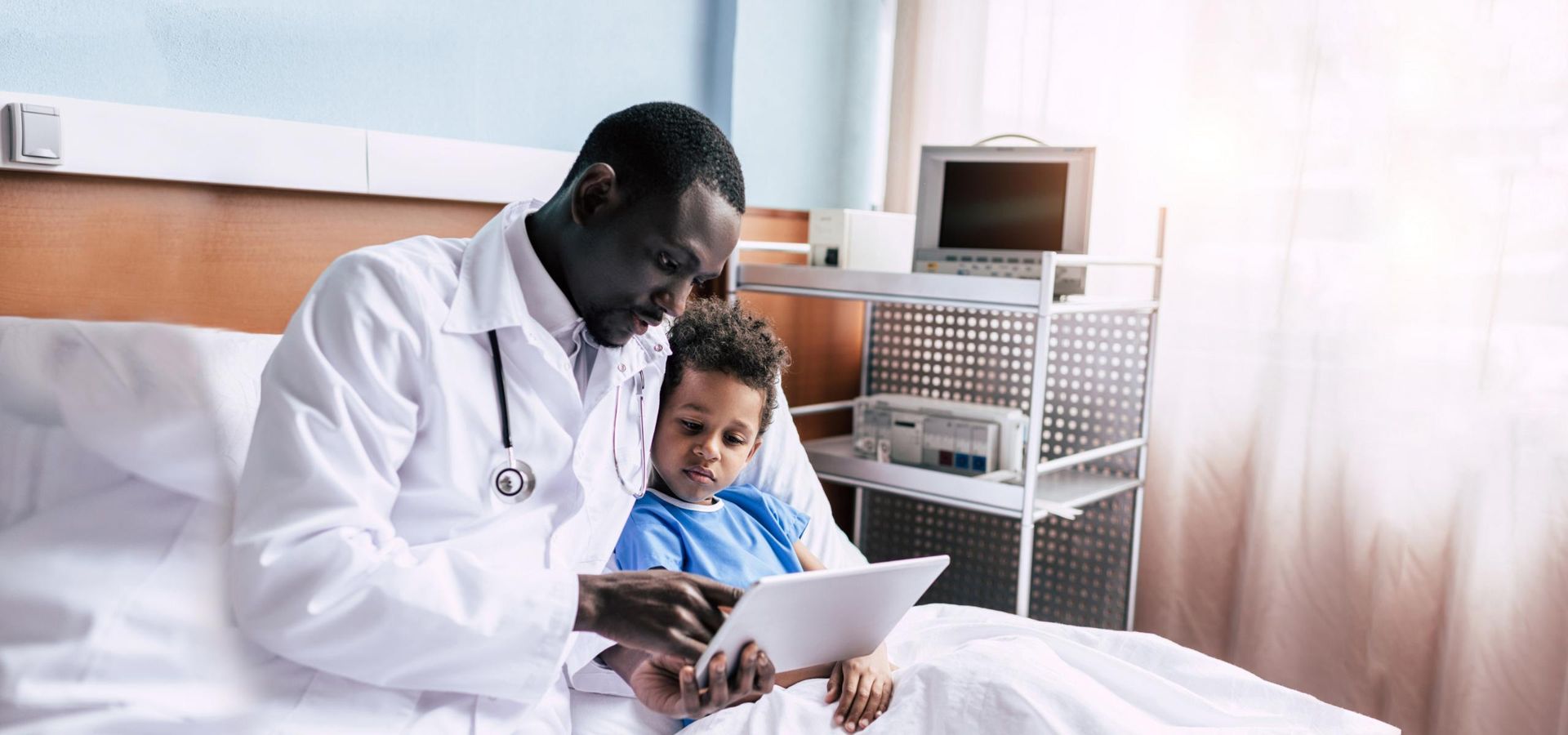
Custom healthcare software development: solutions & best practices
- Home
- Industries
- Healthcare
Custom healthcare software development enables healthcare interoperability, better patient engagement, and more accurate diagnostics by equipping medical practices with software best suited to their unique workflows and requirements.
Our healthcare software development services include consulting, design, development, maintenance, and upgrade of medical apps for hospitals, diagnostic and research centers, long-term care facilities, pharmaceutical companies, medical device manufacturers, health startups, and other healthcare organizations.
Healthcare software development market overview
the expected healthcare IT market CAGR from 2020 - 2030
Grand View Research
of healthcare organizations invest in AI, ML, and predictive analytics
PWC Health Research Institute
of users expect to manage their health information online
McKinsey
Boost your healthcare business with high-quality software
Why Itransition
25+ years of experience in healthcare IT
Forrester's 2022 Modern Application Development Services report recognition
Ranked high in 2021 Execution Zone for Telemedicine and IoT by Zinnov Zones
HIPAA- and HITECH-compliant software development
Adherence to OWASP, IEC 62443 and other medical development security criteria
Working knowledge of the major healthcare standards and coding systems, including DICOM, FHIR, ICD-10, and CPT
Expertise with FDA classes II and III, and IEC 62304 classes A, B and C of medical devices
Internal Healthcare Center of Excellence
Our healthcare software development services
We are ready to handle end-to-end healthcare software development or help you at any stage of the project.

Consulting
Our consultants help medical organizations establish optimal digital environments by advising on the technologies that fit into your company’s ecosystem, helping choose solutions most suitable for your business, and drawing up an efficient plan for their implementation.
Development
Itransition’s development team is ready to deliver any type of healthcare system and implement a platform-based solution according to your business vision. We employ application implementation best practices, keeping security and industry requirements in mind and adhering to the methodology best suited for your case.
Integration
We make healthcare IT ecosystems more connected by integrating your software with third-party applications and ensuring their interoperability and security while also simplifying the adoption of value-adding extensions down the road.
Support & maintenance
Our experts help healthcare companies keep their software fully operable, flexible, secure, and cost-effective. We provide support services on multiple levels, including pre-support audits, 24/7 performance monitoring, and timely upgrades and customizations.
Legacy software reengineering
We help medical institutions revamp their legacy software by upgrading, extending, or completely reworking it. Our developers can revamp your existing software so it becomes more secure, reliable, and compliant with the current standards.
Expand your healthcare service portfolio with comprehensive software solutions
Healthcare solutions we develop
Itransition builds internal and customer-facing healthcare software which can be easily integrated into your IT infrastructure.
We deliver electronic health record and medical record software to help healthcare providers enhance their organization’s interoperability, efficiency, and patient care by enabling them to acquire, store, manage and transfer patient records between specialists, departments, and institutions.
- Digital patient history with treatment plans and lab results
- Schedules and automated reminders about upcoming tasks
- Patient data collection from healthcare software and patient devices
- Voice recognition for accelerated data input
Itransition promotes healthcare interoperability by setting up smooth and secure data flow between electronic medical records systems and other software and devices. We have extensive EHR/EMR integration experience with the Epic, Cerner, Meditech, and Allscripts platforms, as well as custom software.
- Integration consulting
- End-to-end enterprise integration
- Integration with particular software
- Testing and optimization
- Healthcare professionals’ user training
We develop standalone healthcare applications for patients, medical professionals, and organizations or mobile app versions of existing medical software. Depending on your needs, we can equip mobile medical apps with a variety of custom features and integrate them with third-party systems and wearable devices.
- Remote patient monitoring
- Team collaboration
- Access to EHR
- Rostering
- E-prescribing
- Real-time health data tracking
- Appointments management
- Reminders and alerts
- Online consultations
- Education and training
Itransition delivers custom CRM software with healthcare providers’ needs in mind. Our fully compliant and secure CRMs help medical organizations deliver next-level patient experience by automating their practice management as well as marketing and sales operations.
- Patient management
- Customer service integration
- Sales and marketing automation
- Referral management
- Document management
- Task management and collaboration
- Reporting and analytics
Our team can deliver patient portals, remote condition monitoring software, educational tools, and other patient engagement solutions that encourage patients to be more proactive in managing their own health, boost patient engagement and loyalty, facilitate hospital-patient communication, and improve population health.
- Real-time communication tools
- Wellness and lifestyle apps
- Personal health information management features
- Educational apps
- Analytics and reporting
We deliver tools for medical practices to digitize and improve their processes, creating an IT ecosystem for their hospitals that streamlines patient, data, staff, and inventory management, financial and cross-department operations, or provides analytical insights on hospital productivity.
- Patient and contract management
- Supply chain management
- Asset, inventory and medication management
- Billing and insurance claims management
- E-prescriptions
- Ward management
- Resource planning
- Appointment management
Itransition develops and implements software that can be integrated even with the most strictly-regulated types of medical devices. We build apps to power and manage diagnostic, monitoring, and treatment devices, as well as solutions that can perform medical device functions on their own (SaMD).
- Patient wearables
- Inhalers and defibrillators
- Smart carts
- Blood collection devices
- Barcode scanners, biometric and RFID readers
- Temperature, humidity and cardiac board sensors
- Smart pens, scales, and tubing welders
We equip pharmaceutical companies with secure, reliable, and compliant inventory, prescription, documentation, and financial management tools, as well as powerful analytics solutions that help conduct business more effectively, protect customers from fraud, and improve the patient experience.
- Document management
- Inventory management
- Prescription management
- Financial management
- Analytics and reporting
Itransition helps healthcare organizations monetize their services by implementing custom solutions for order and product management, customer relations, as well as sales and marketing operations, which in turn can be integrated with other healthcare software solutions and ecommerce tools.
- Product management
- Omnichannel customer service
- Customer relations and marketing
- Order management
We deliver platform-based and custom patient portal software for patients to manage their health more proactively by accessing their health records, scheduling appointments, and communicating with the care provider.
- Secure access to patient health information
- Patient self-service
- Online doctor consultations
- Feedback aggregation
- Personal health data analytics
- Patient information and education
- Insurance and payment management
- Appointments management
Our experts create telehealth software that can provide remote clinical services, including diagnostic and treatment, or enable a more engaging online medical education and training while ensuring medical data security.
- Doctor consultations and virtual care
- Mobile telemedicine
- Patient data management
- Screen sharing
- Annotation tools
We employ scalable and secure data warehouses, OLAP analytics, data mining, and artificial intelligence algorithms to deliver actionable insights for every department, from diagnosticians to sales and marketing, and a 360-degree view of your healthcare facility.
- Descriptive, predictive and prescriptive analytics
- Precision medicine analytics
- Medical image analysis
- Performance and facility management analytics
- Population health analytics
Our business intelligence and machine learning experts deliver custom software that automates the extraction and visualization of meaningful insights from medical big data coming from EHRs, medical devices, laboratory information management systems, etc., as well as from information contained in CRMs, practice management software, customer survey tools, and other systems.
- Extract, transform, and load (ETL)
- Enterprise data warehouse (EDW)
- Data mining and discovery
- Data visualization and reporting
Itransition delivers custom software solutions for all types of health data collection devices that help medical practices to elevate the quality of medical assistance by securely collecting, storing, processing, and transferring patients’ health information.
- Population health management
- Post-discharge care management
- Hospital-at-home
- Remote diagnostics
Success stories
For over 25 years, Itransition has consistently delivered successful custom medical software implementation projects, helping healthcare organizations reach new heights.
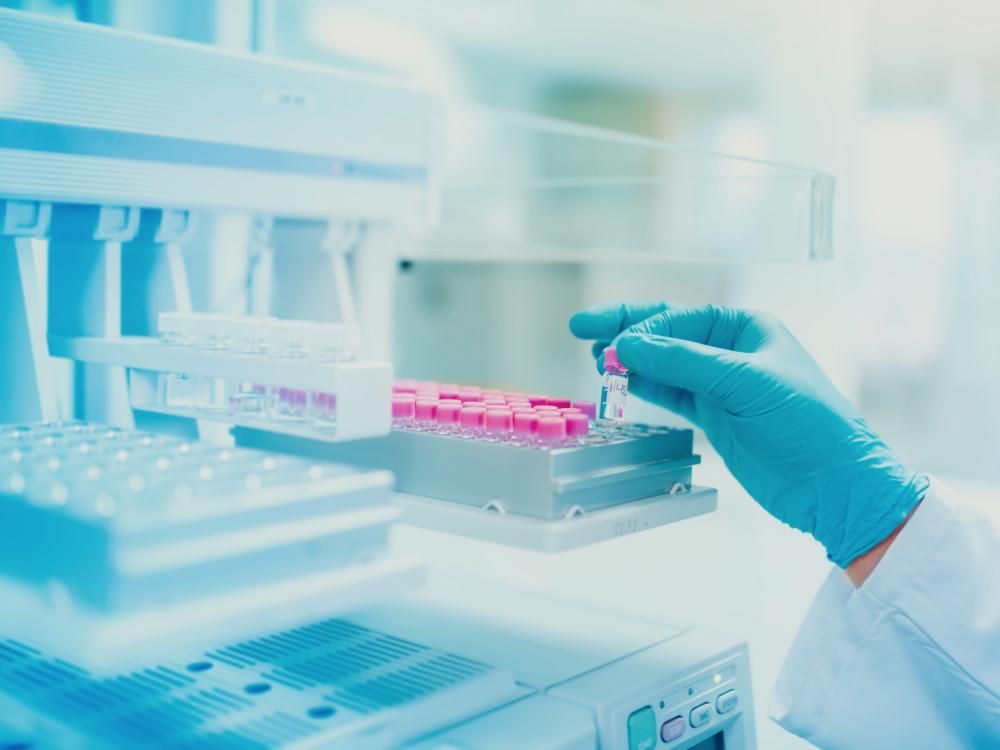
10x
faster data processing
Pharmaceutical data analytics suite
Itransition developed and then continuously supported the client’s analytics suite that handles over 500 million patient records and several dozen petabytes of proprietary data.
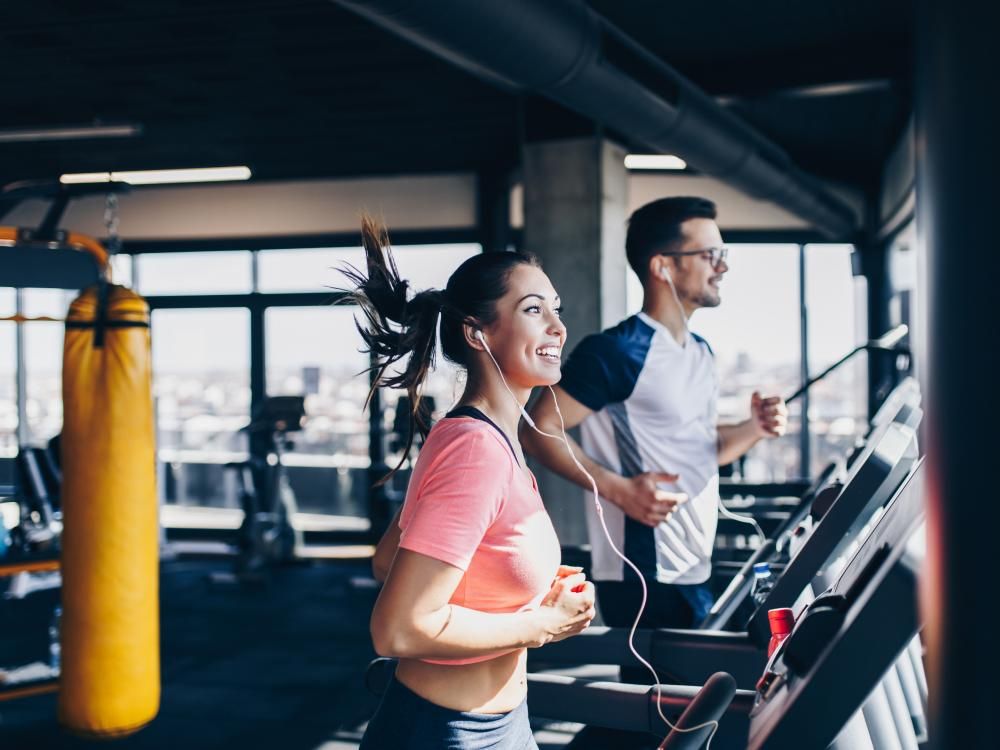
100,000+
registered users
SaaS wellness platform
Our team developed a HIPAA-compliant SaaS platform with complementary OS-agnostic mobile applications that helps lifestyle, nutrition and fitness specialists to provide wellness services.
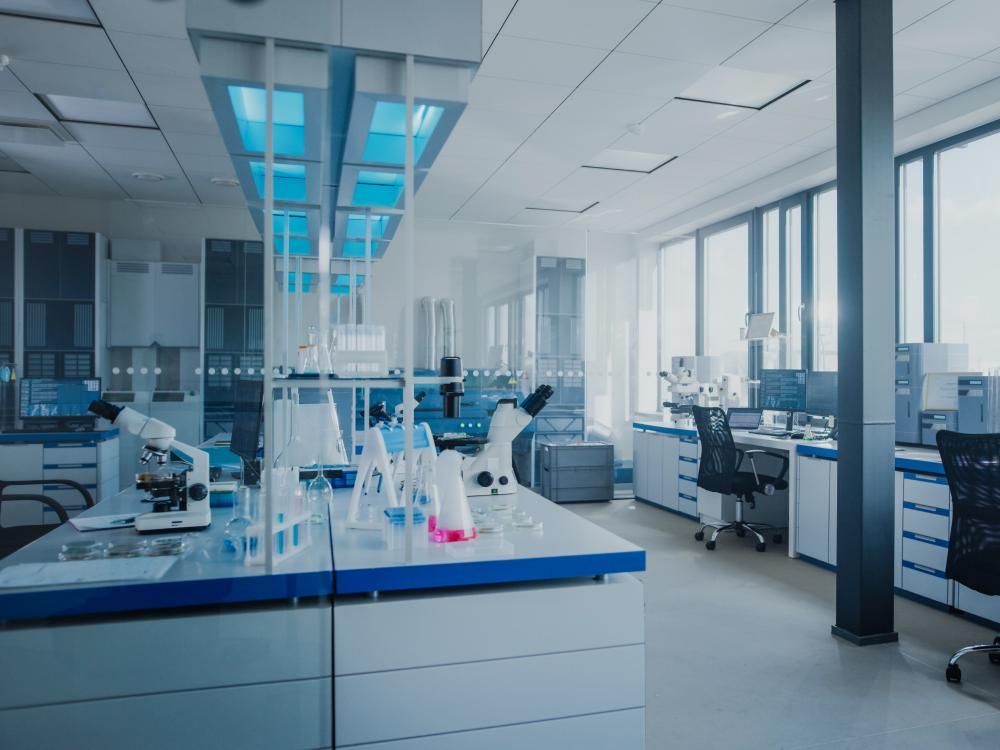
400
blood centers use our solution
Medical equipment management software
Our experts developed an FDA- and IEC 62304-compliant web solution that automatically integrates and manages blood transfusion devices and enables collection and processing of large amounts of blood data.
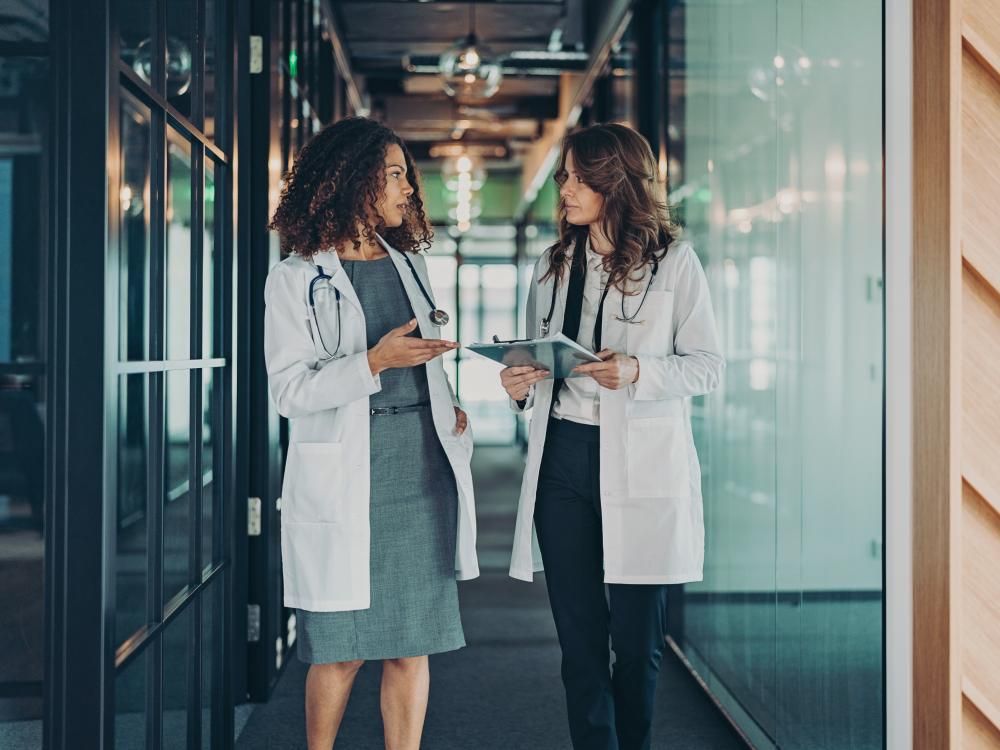
3-in-1
custom solution
CRM/ERP/HR for staffing provider
We delivered a comprehensive Odoo-based solution for a US-based healthcare staffing service provider that allows conducting all contract- and tender-related business processes in one place.
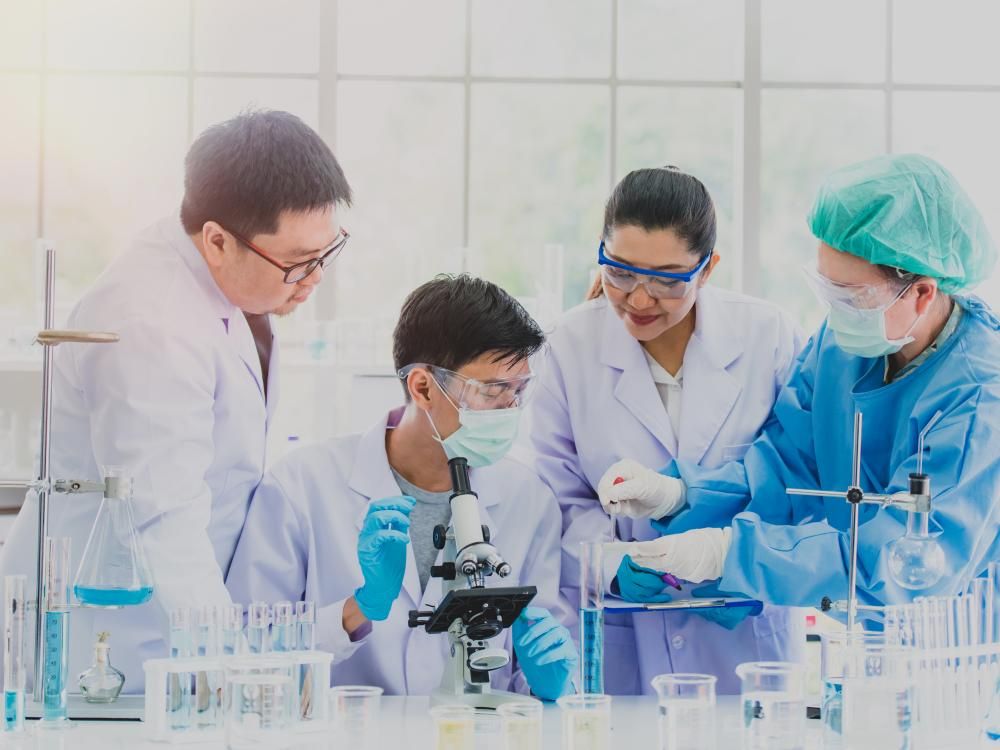
2x
faster test runs
Sample analysis solution revamp
Itransition developed a part of a complex sample submission solution, delivering intuitive sample management, accelerated delivery and increased code quality, and a centralized app store for chemists.
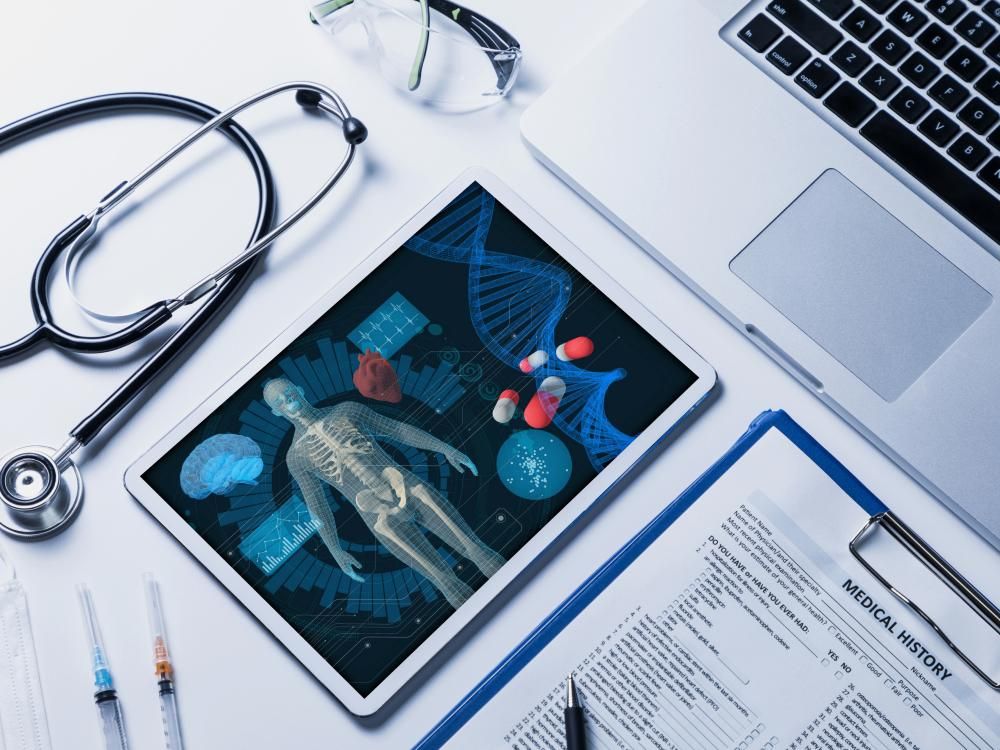
HIPAA, ONC, CEHRT, and Section 508 compliance
compliance
Telemedicine-ready EHR system
We delivered an EHR system that optimizes patient records input and facilitates doctor-patient communication through speech recognition, smartpen, clinical text analysis, NLP, and online medical conference tools.
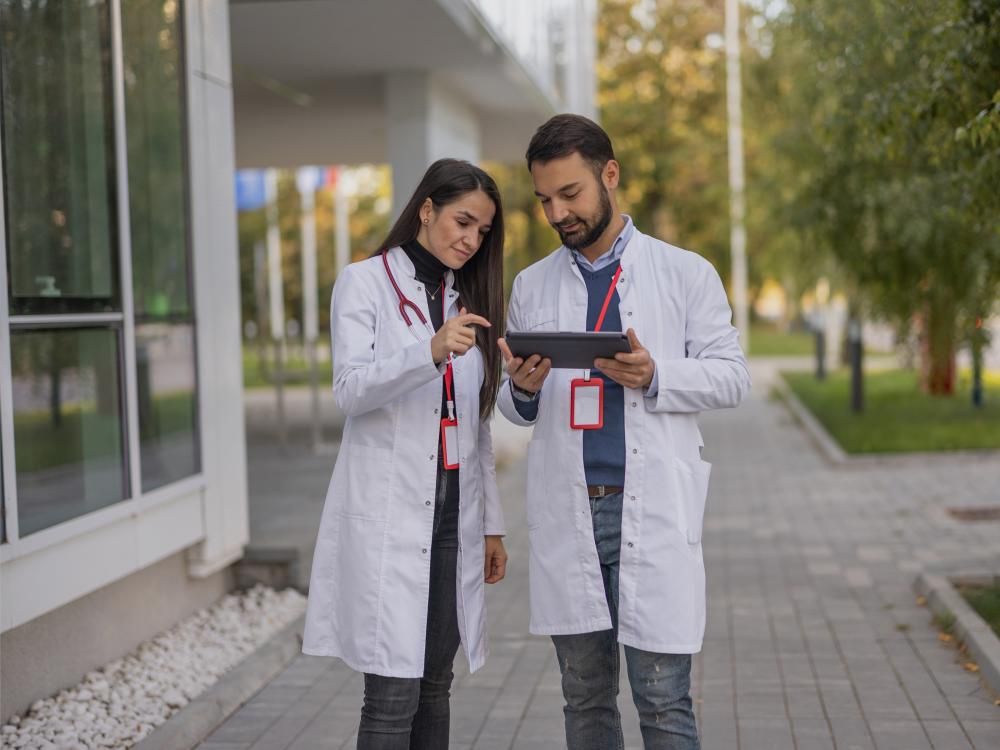
30+
new healthcare institution clients
Clinical data exchange PoC
Our software development team has become an integral part of the customer’s in-house team, successfully delivering a PoC and proceeding to develop a full-featured Flutter-based application for ambulance doctors and paramedics to securely store and exchange clinical data.
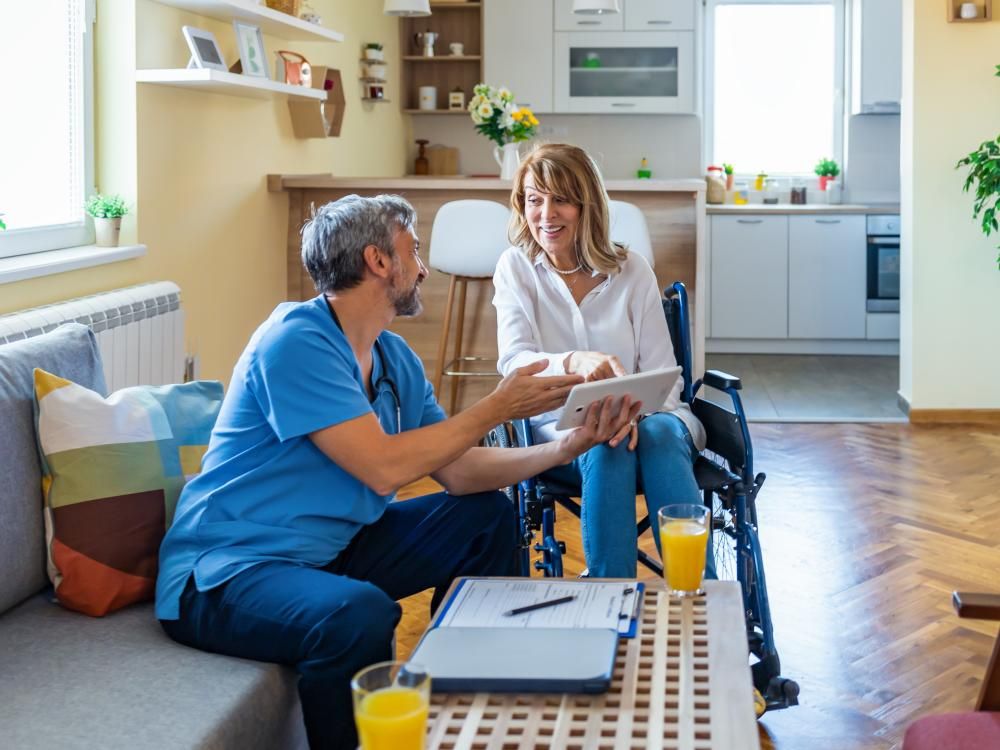
1,000
therapy orders created monthly
Oncology treatment platform
Itransition created a platform that enables doctors to make oncology treatment decisions based on medical research results, quickly create treatment orders, and review auto-generated reports for recommended therapies.
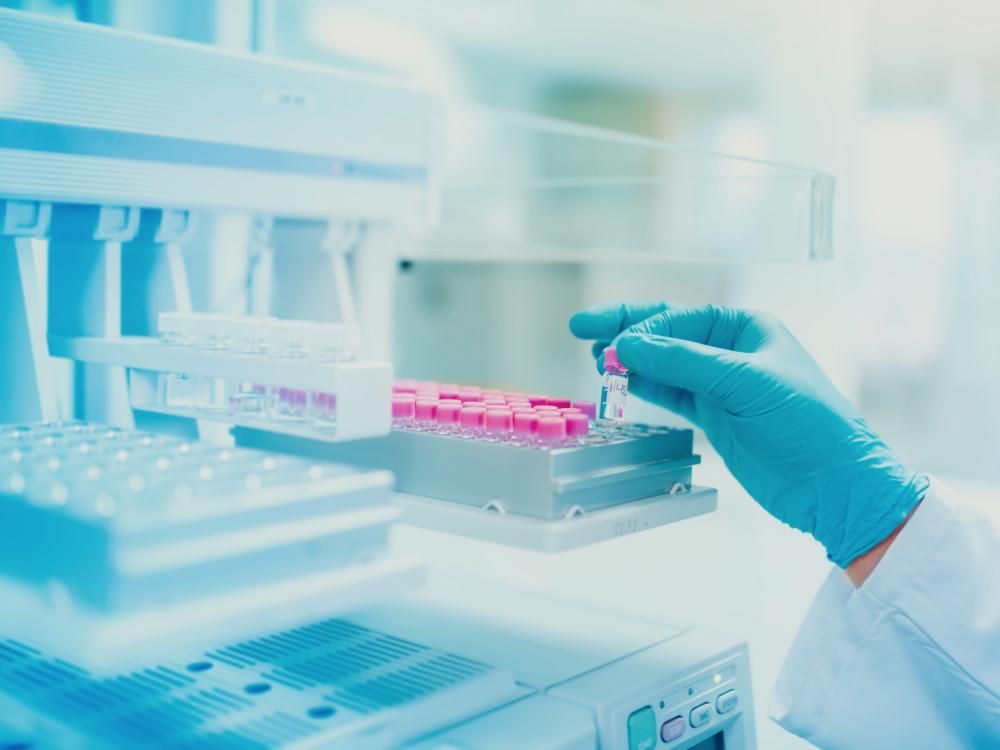
10x
faster data processing
Pharmaceutical data analytics suite
Itransition developed and then continuously supported the client’s analytics suite that handles over 500 million patient records and several dozen petabytes of proprietary data.
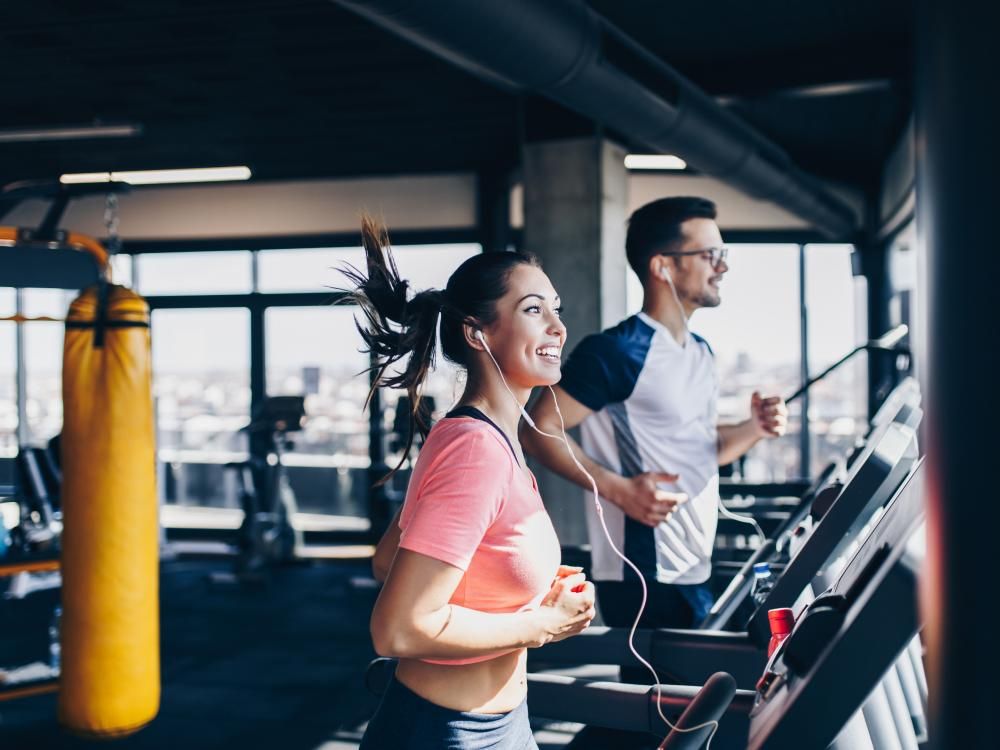
100,000+
registered users
SaaS wellness platform
Our team developed a HIPAA-compliant SaaS platform with complementary OS-agnostic mobile applications that helps lifestyle, nutrition and fitness specialists to provide wellness services.
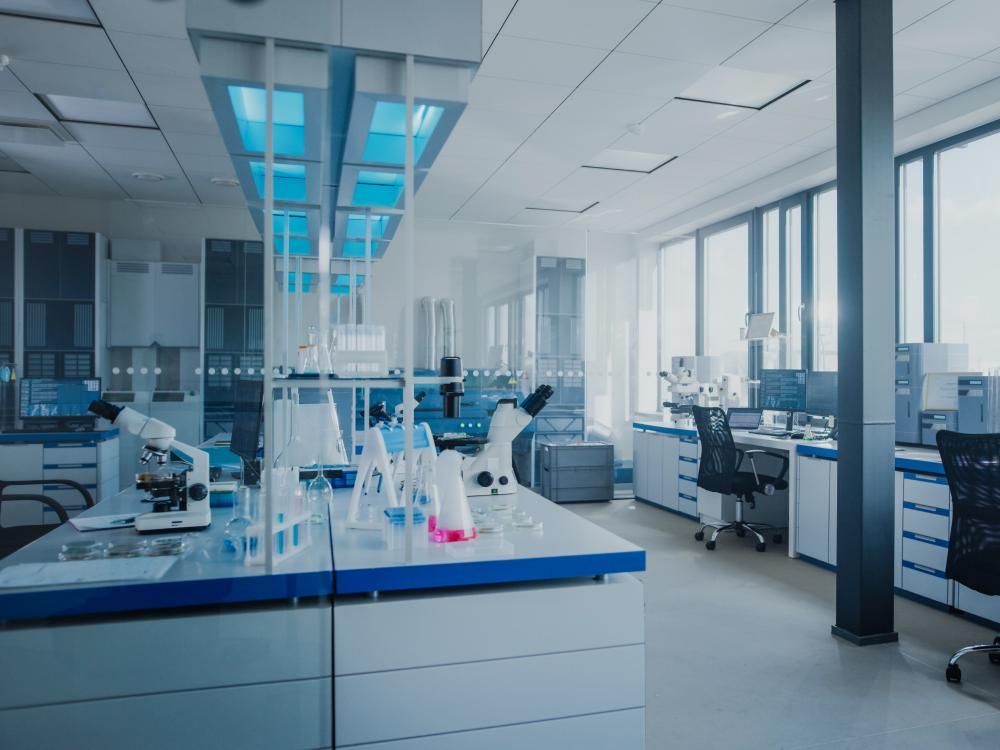
400
blood centers use our solution
Medical equipment management software
Our experts developed an FDA- and IEC 62304-compliant web solution that automatically integrates and manages blood transfusion devices and enables collection and processing of large amounts of blood data.
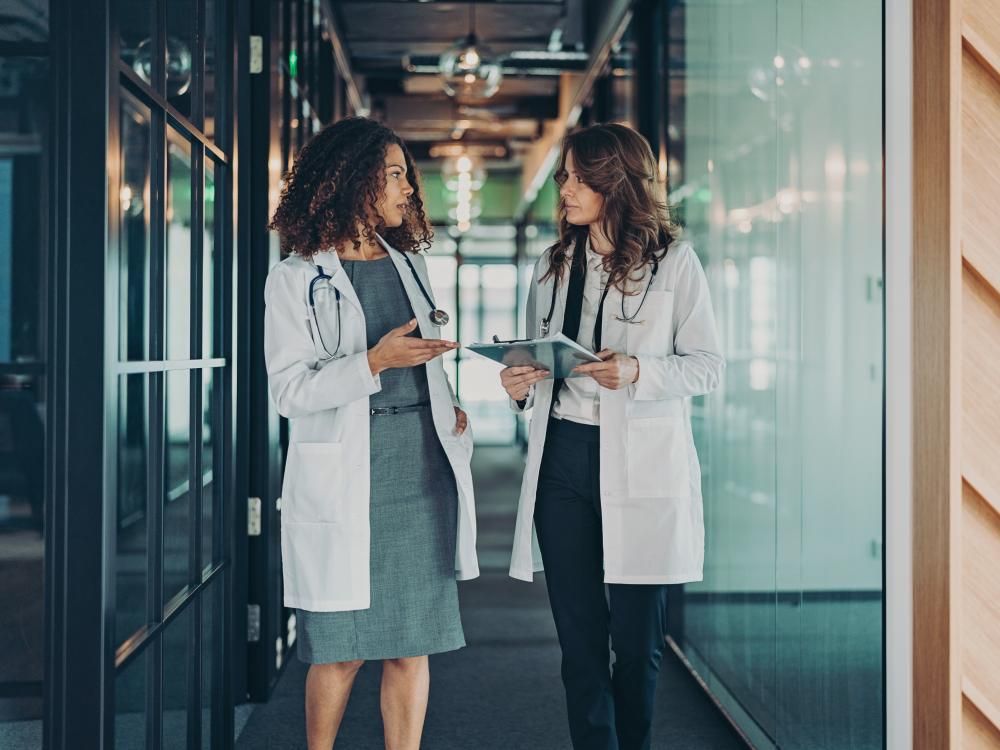
3-in-1
custom solution
CRM/ERP/HR for staffing provider
We delivered a comprehensive Odoo-based solution for a US-based healthcare staffing service provider that allows conducting all contract- and tender-related business processes in one place.
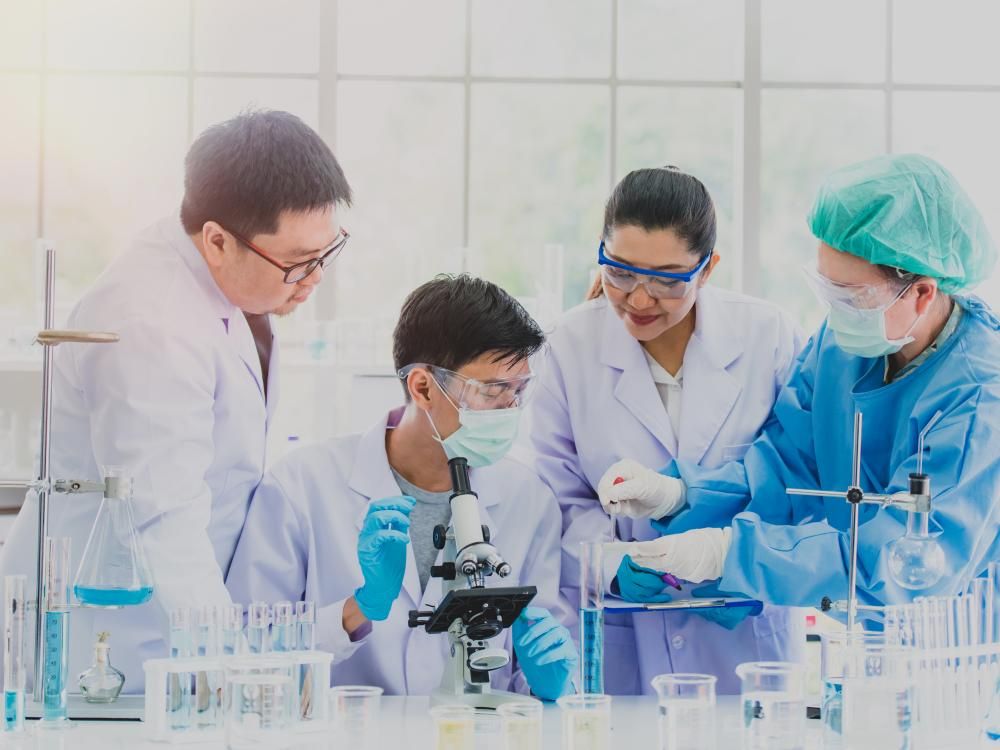
2x
faster test runs
Sample analysis solution revamp
Itransition developed a part of a complex sample submission solution, delivering intuitive sample management, accelerated delivery and increased code quality, and a centralized app store for chemists.
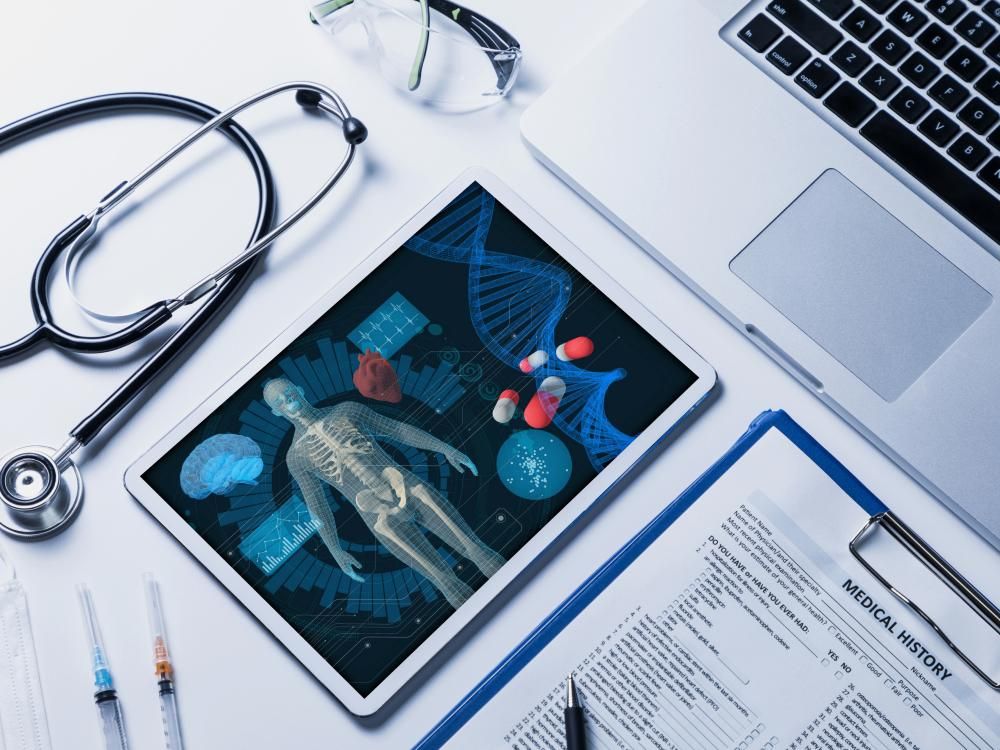
HIPAA, ONC, CEHRT, and Section 508 compliance
compliance
Telemedicine-ready EHR system
We delivered an EHR system that optimizes patient records input and facilitates doctor-patient communication through speech recognition, smartpen, clinical text analysis, NLP, and online medical conference tools.
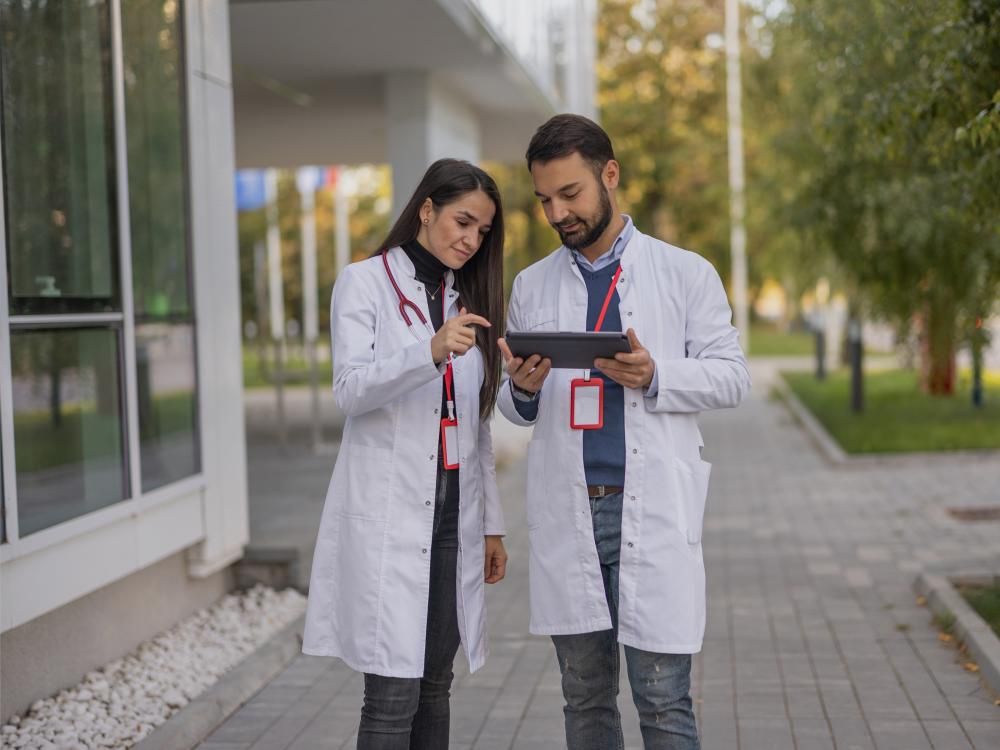
30+
new healthcare institution clients
Clinical data exchange PoC
Our software development team has become an integral part of the customer’s in-house team, successfully delivering a PoC and proceeding to develop a full-featured Flutter-based application for ambulance doctors and paramedics to securely store and exchange clinical data.
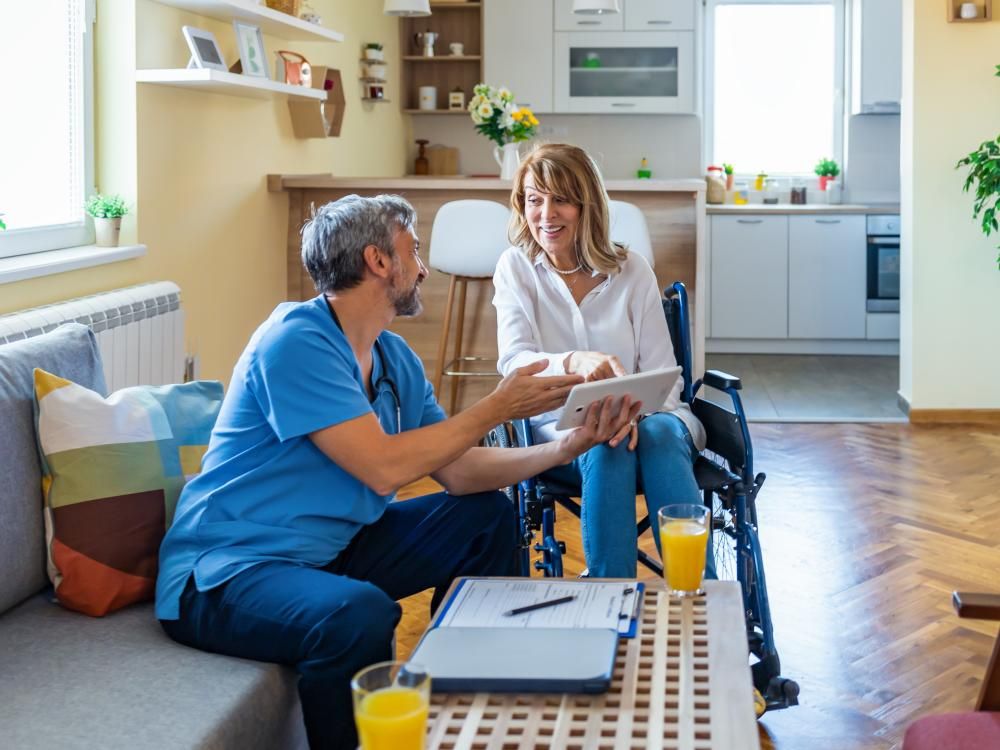
1,000
therapy orders created monthly
Oncology treatment platform
Itransition created a platform that enables doctors to make oncology treatment decisions based on medical research results, quickly create treatment orders, and review auto-generated reports for recommended therapies.
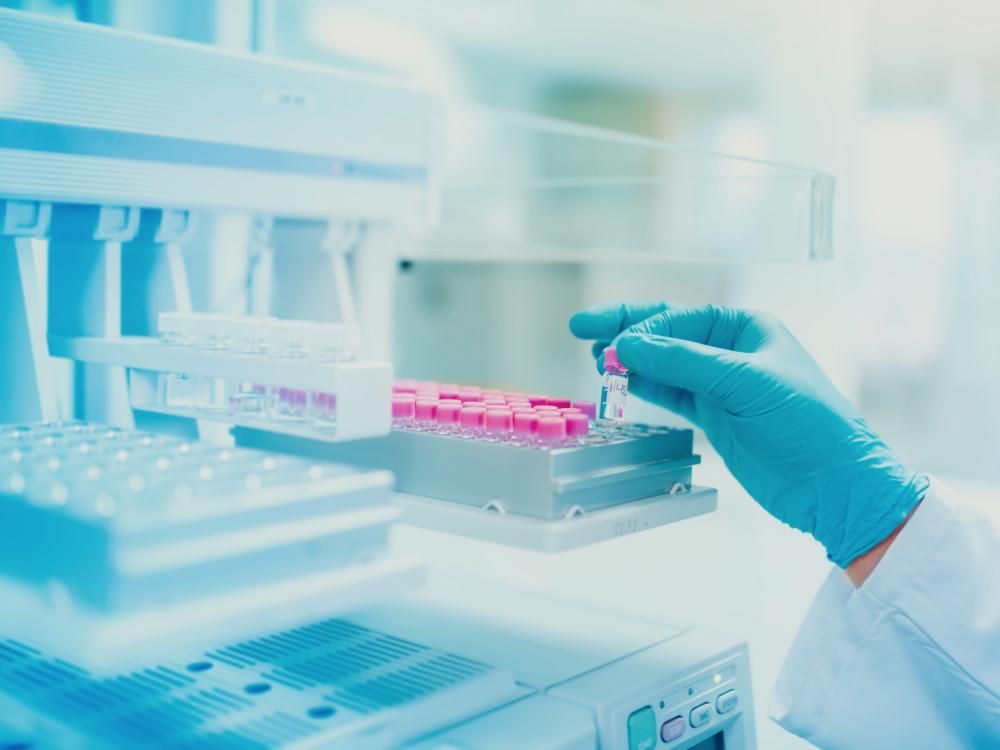
10x
faster data processing
Pharmaceutical data analytics suite
Itransition developed and then continuously supported the client’s analytics suite that handles over 500 million patient records and several dozen petabytes of proprietary data.
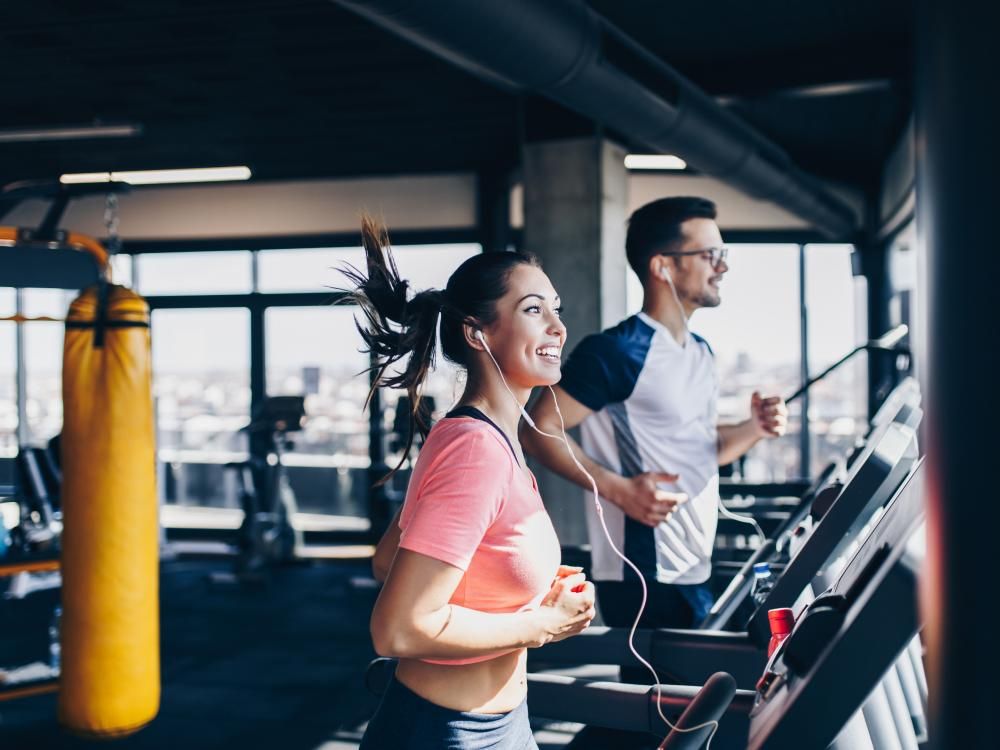
100,000+
registered users
SaaS wellness platform
Our team developed a HIPAA-compliant SaaS platform with complementary OS-agnostic mobile applications that helps lifestyle, nutrition and fitness specialists to provide wellness services.
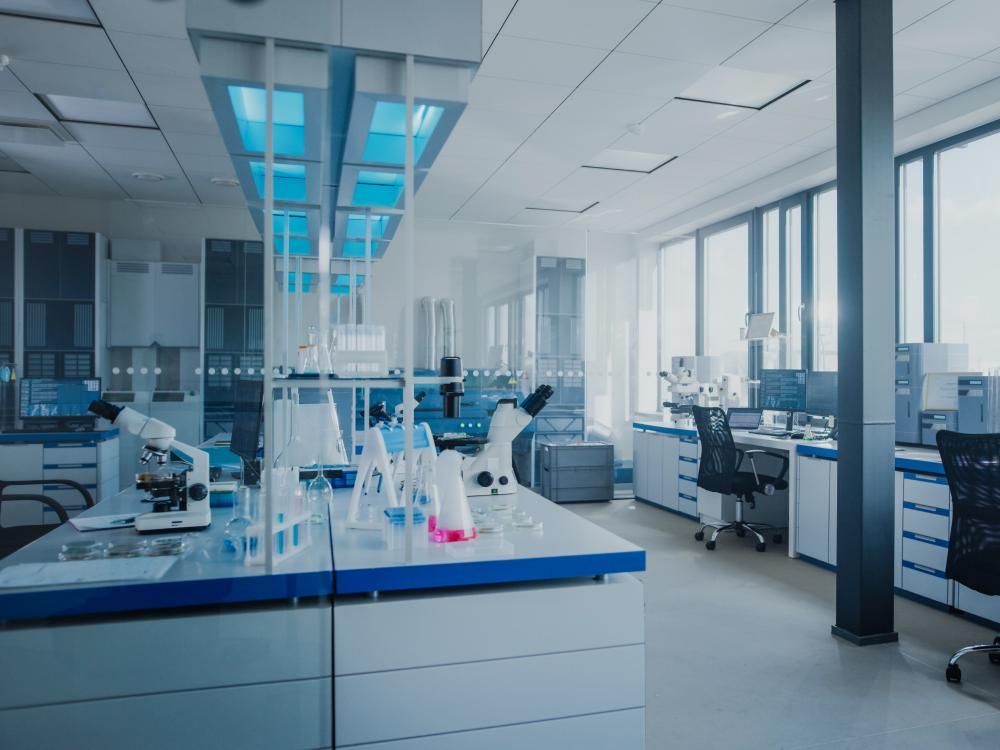
400
blood centers use our solution
Medical equipment management software
Our experts developed an FDA- and IEC 62304-compliant web solution that automatically integrates and manages blood transfusion devices and enables collection and processing of large amounts of blood data.
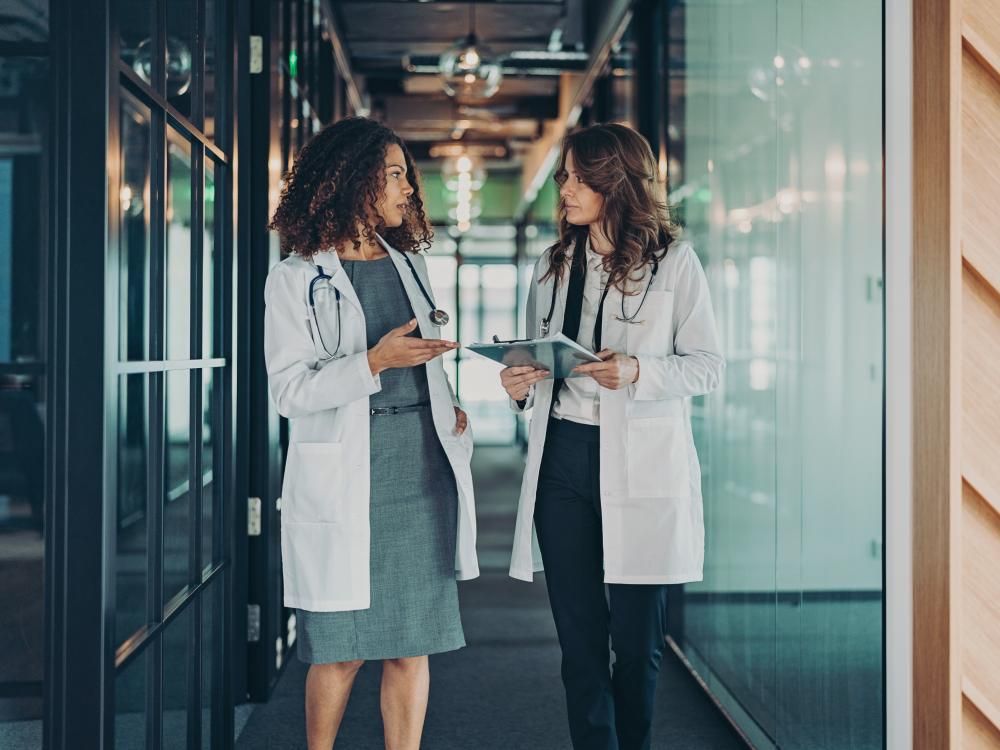
3-in-1
custom solution
CRM/ERP/HR for staffing provider
We delivered a comprehensive Odoo-based solution for a US-based healthcare staffing service provider that allows conducting all contract- and tender-related business processes in one place.
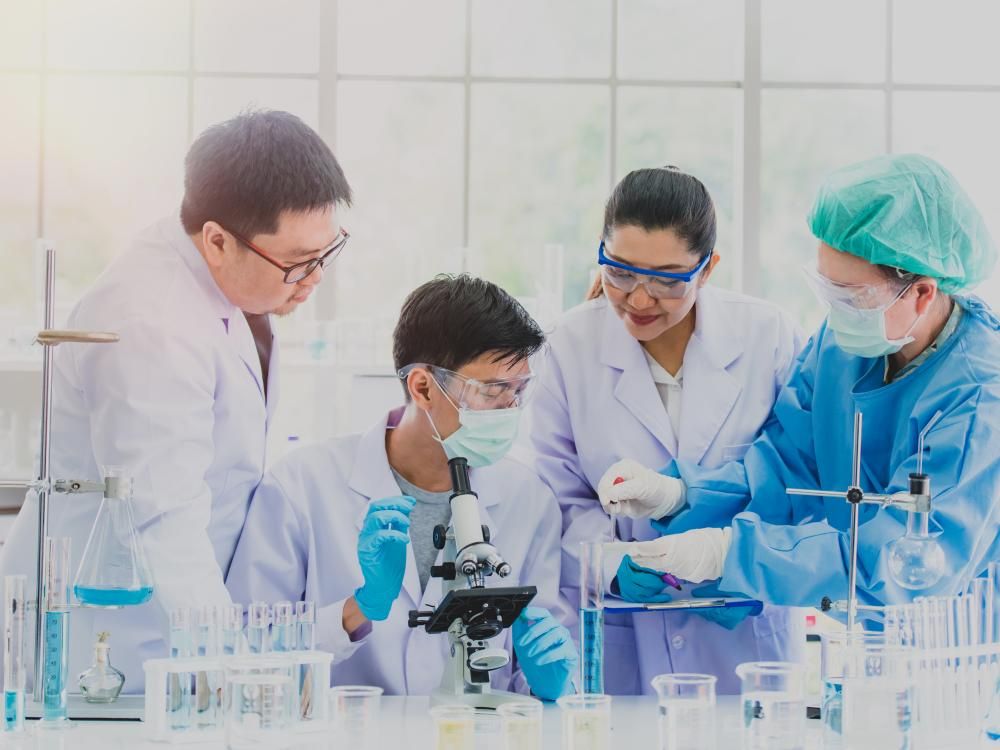
2x
faster test runs
Sample analysis solution revamp
Itransition developed a part of a complex sample submission solution, delivering intuitive sample management, accelerated delivery and increased code quality, and a centralized app store for chemists.
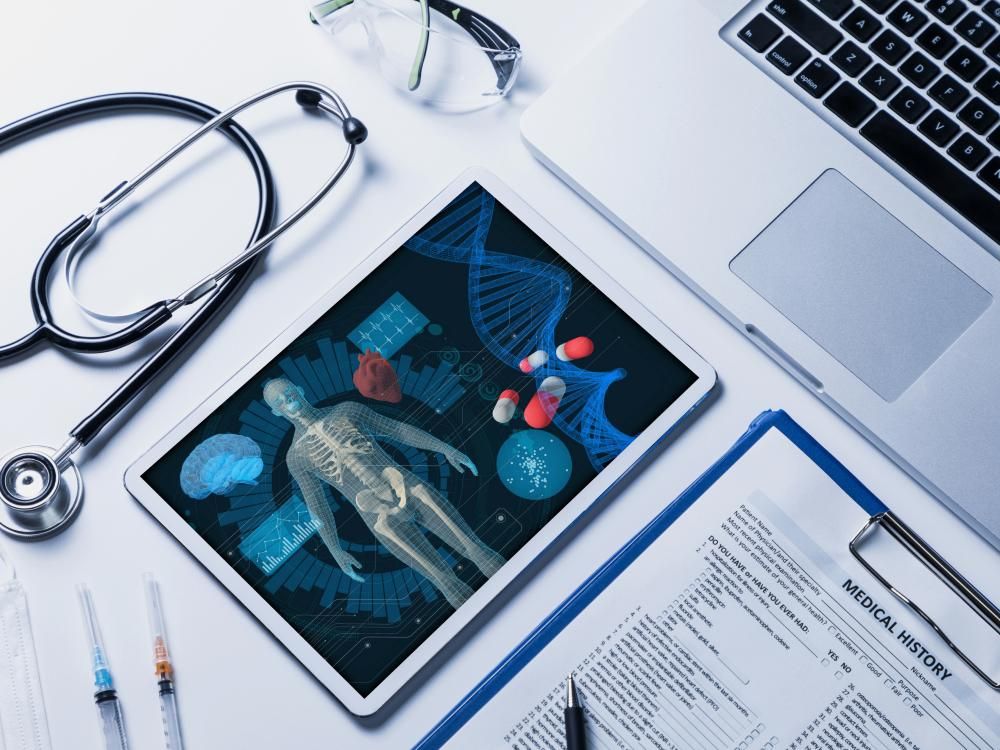
HIPAA, ONC, CEHRT, and Section 508 compliance
compliance
Telemedicine-ready EHR system
We delivered an EHR system that optimizes patient records input and facilitates doctor-patient communication through speech recognition, smartpen, clinical text analysis, NLP, and online medical conference tools.
Our clients say
Together with Itransition, we managed to develop a solution that transformed the way wellness services are provided. The platform already boasts the following results: 700+ providers offering their services through the platform, 10k+ registered users, and 10+ organizations using the solution’s white-label version.
Jillian Bridgette
CEO & Co-founder Virtual Health Partners, Inc
Thanks to Itransition’s technology expertise and experience in setting up development processes in multinational distributed teams, we have been able to accelerate delivery, scale teams up without slowing down development, and increase team velocity and productivity. All above helped us speed up proving product viability, hitting important milestones, and showing demos to key company players.
Oren Wiesler
Director of Engineering Management Waters Corporation.
Custom vs platform-based healthcare software
The choice between custom and platform-based software can be complicated but it largely determines how beneficial it will be for your company. Our consultants can help you recognize which works best for your organization, improving healthcare professionals’ and patients’ satisfaction, an organization’s efficiency, and profits. Whenever you have a clear understanding of the type of desired software, our team will be glad to develop a fully custom system or implement a platform-based one.
Custom software
Platform-based software
Pros
Custom software
Platform-based software
Only valuable functionality Tailored to your specific workflows Provides full control over the system and data
Affordable upfront cost Fast implementation Highly interoperable with software from the same vendor
Cons
Custom software
Platform-based software
Relatively high upfront cost Takes longer to implement Requires additional time and effort to integrate with other systems
Functionality and interface are mostly pre-determined by the vendor Less flexible and scalable than custom solutions
Healthcare software development roadmap
We perform the full scope of services necessary for successful healthcare software adoption in any healthcare organization, ensuring the solution’s scalability and secure personal data management in any deployment mode.
1
Discovery
We start by closely collaborating with medical staff and management and defining an organization’s requirements
2
Development
Together with our client’s team, we decide on the tech stack and map out the project’s milestones
Our experts create a custom healthcare app or tune a platform-based solution
Our integration team connects the new healthcare solution to other hospital software or third-party apps
We perform all-round quality assurance and ensure GDPR/HIPAA compliance throughout the entire process
3
Implementation
We install the new healthcare solutions into the organization’s IT ecosystem and migrate all the relevant data
4
Post-deployment
Depending on the service package, we keep monitoring the system’s performance, solving operational issues, and implementing upgrades after the deployment
Core tech stack we use
As a custom healthcare software development company, Itransition uses an extensive set of engineering tools and runs an in-house R&D lab to explore and adopt emerging technologies.
Languages
C# Python
Java C
C++ Ruby
PHP Javascript
Frameworks and libraries
.NET Core ASP.NET
ASP.NET Core Angular
React Ruby on Rails
Vue.js
Cloud
Microsoft Azure Amazon Web Services
Google Cloud Platform
Apprenda
Rackspace
Mobile technologies
iOS (swift/Objective C) Android (Kotlin/Java)
Flutter React Native
Kotlin Multiplatform Mobile (KMM)
Xamarin
Platforms
Salesforce
Pega
Odoo
Microsoft
Database-related
SQL Server Postgres
MongoDB Amazon DynamoDB
Azure Cosmos DB Redis
SSIS (Microsoft SQL Server Integration Services)
Entrust medical software development to industry experts
Our expertise with innovative technologies
Our experts constantly monitor the most recent trends in the healthcare industry and employ innovative technologies to develop smarter solutions.
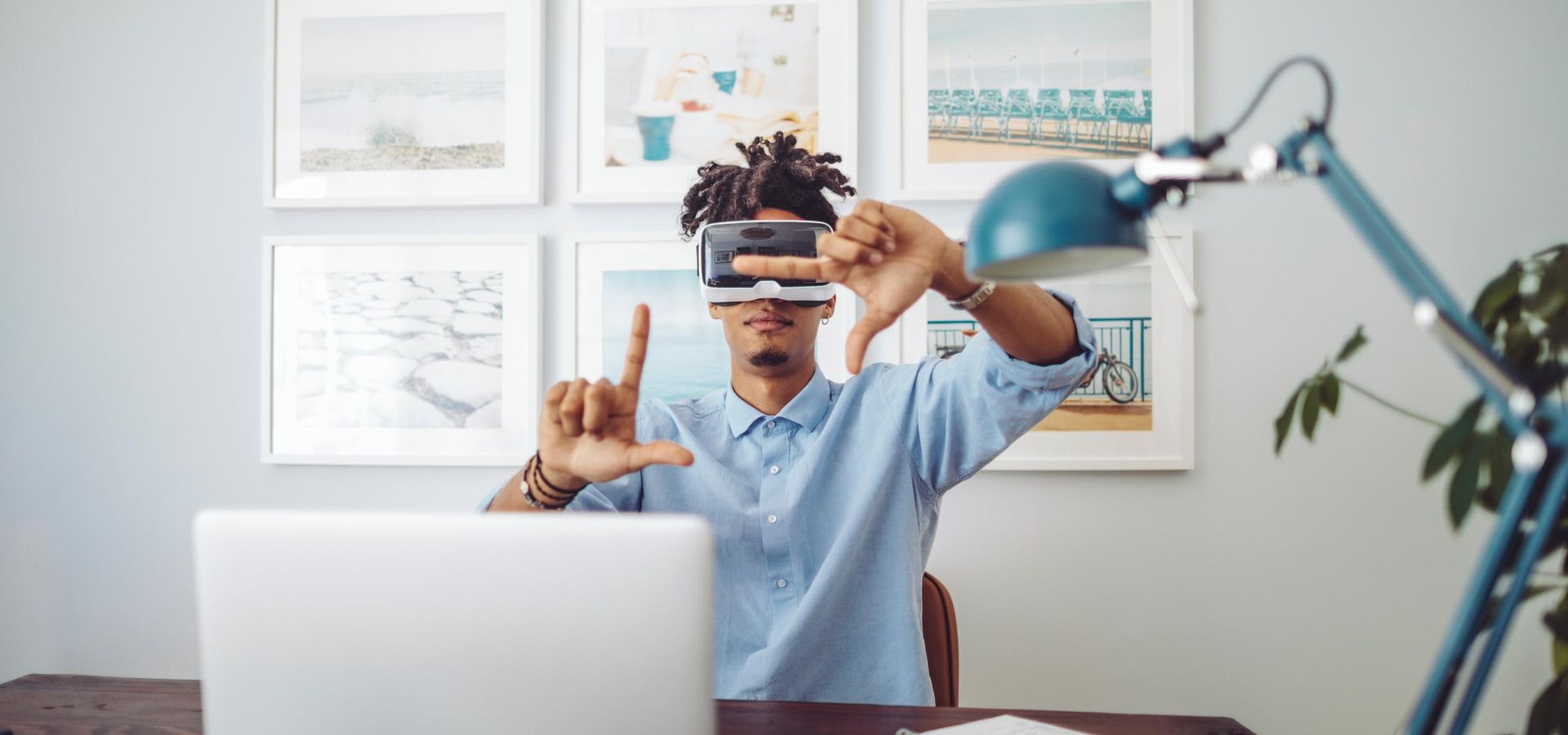
VR, AR & MR
Our team of medical app developers offers their hands-on experience in implementing VR, AR, and MR to facilitate surgery, ensure accurate diagnostics, or provide interactive education for medical practitioners and students.
RPA
We introduce time- and cost-saving bots into healthcare institutions’ workflows to help with routine tasks, such as answering common patient requests, filling out forms, compiling reports, sending appointment reminders, and facilitating document management.
Machine learning
Our experts employ sophisticated ML algorithms that enhance diagnostic and analytical precision, streamline routine operations, and enable modern examination methods.
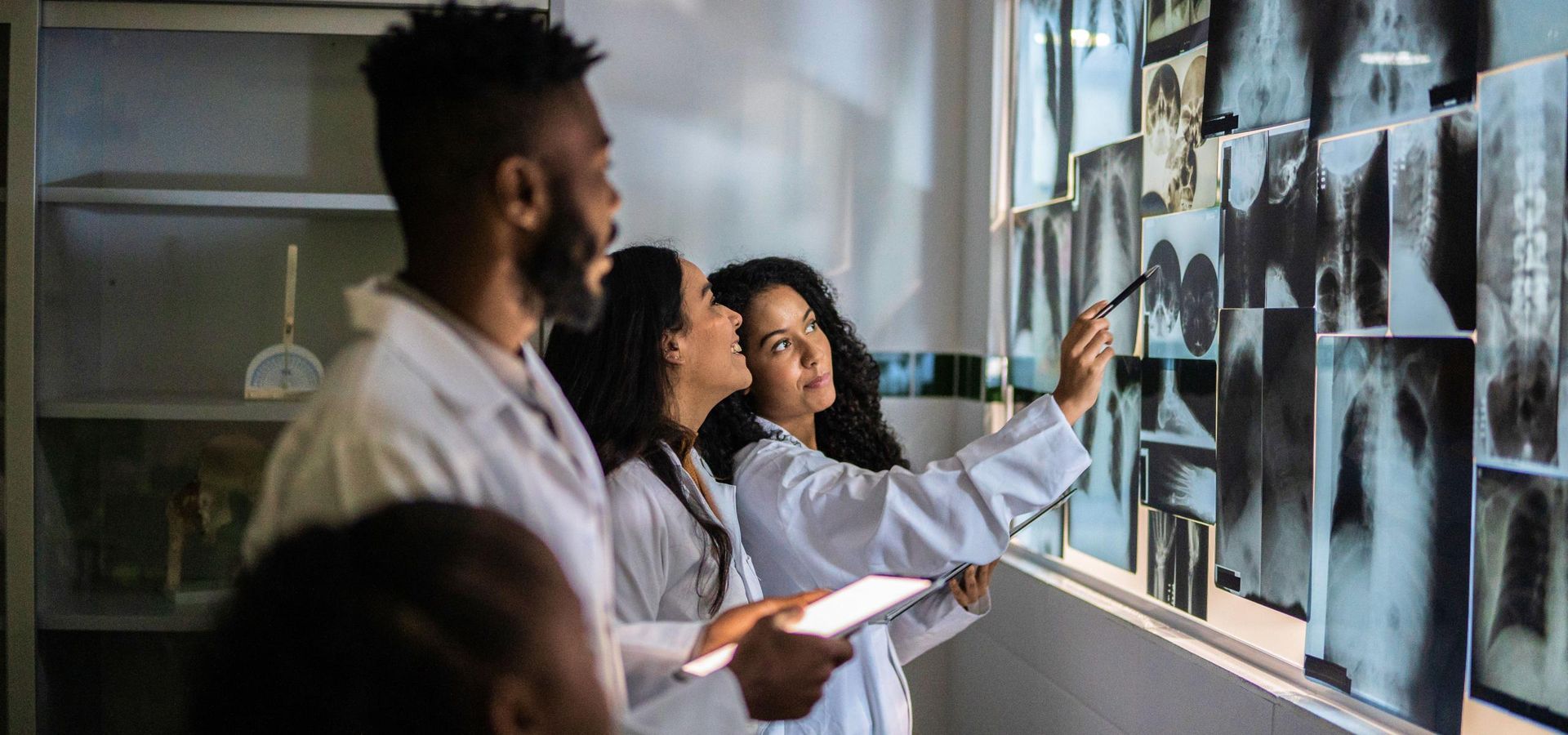
Medical image analysis
We deliver medical imaging software for research and clinical needs. Our solutions support different medical image modalities, raise the effectiveness of early diagnostics, and reduce the risk of medical errors and excessive tests.
Benefits of building custom medical software with Itransition
We employ modern implementation techniques and tools to ensure the highest quality of our services and to bring our clients maximum value.
Tailored functionality
Our custom healthcare software completely fits the required functionality, offering only valuable modules that don’t overcomplicate the system, a user-friendly interface, smooth integrations, and relevant support options.
Enhanced interoperability
Our experts support healthcare interoperability by facilitating fast, smooth, and secure data exchange between healthcare systems. This increases healthcare professionals’ satisfaction with the software and the quality of care and also enhances patient experience at various interaction touchpoints.
Better health outcomes
Implementing high-quality healthcare software, we help medical professionals to focus more on each patient, get medical data of better quality, thus making more data-driven and accurate medical decisions, and exchange information with other specialists faster and more securely.
Improved workflows
We develop healthcare solutions that reduce paperwork, help speed up routine processes, extract more value from data, redistribute resources where they are most needed, and make internal changes faster without disrupting care.
Full regulatory compliance
Our healthcare software is developed according to the industry’s major security and interoperability protocols (OWASP, FHIR, etc.) to ensure that health data is handled in accordance with international and local regulations like HIPAA and GDPR.
Powerful scalability
Our team understands the importance of implementing solutions that grow alongside our clients’ businesses. We make sure our systems are highly scalable and also provide maintenance, upgrade, and support services after deployment so the software won’t become outdated.
Reduced cost of care
We implement healthcare solutions that yield quick ROI while helping cut down the number of readmissions, ER visits, and hospitalizations, automating care processes, alleviating medical personnel from burdensome routine tasks, eliminating silos, and lowering the overall cost of care.
Fail-proof security
We employ multi-factor authentication, data encryption, role-based access to medical information, and other techniques to protect our clients from cybercrimes and guarantee patient data protection.
Frequently asked questions
What is the average cost of healthcare app development?
The cost of custom healthcare software development services depends on the number and complexity of the desired solution’s features, the size of the user base, the number of integrations it should have, and other factors. To discover the price for the custom healthcare solution you have in mind, please reach out to our sales team – it’s free.
How much time does medical app development take?
The timeline of healthcare application development varies significantly depending on the quantity and type of its features, the technologies we use for building it, and the platforms it has to run on. Contact us to calculate the approximate time it would take to develop the app you have in mind.
I haven’t found the type of healthcare software product I want to adopt on your list. Will you be able to help me anyway?
We take pride in the flexibility of our medical software development services and constantly expand our portfolio. You can contact our experts and describe a desired solution to learn for sure if we can help you implement it. Additionally, our consultants can recommend the best way to proceed with the particular software adoption.
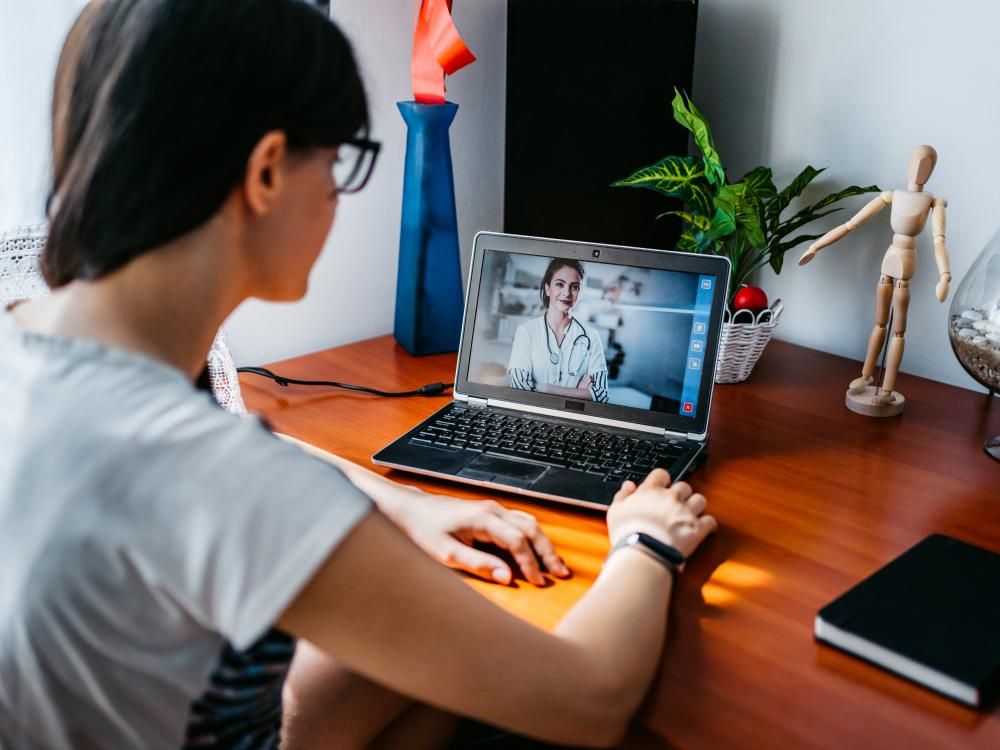
Insights
Digital transformation in healthcare: today’s trends and their positive effects
We look into digital transformation in healthcare and explore its positive impact on care delivery and waste reduction.
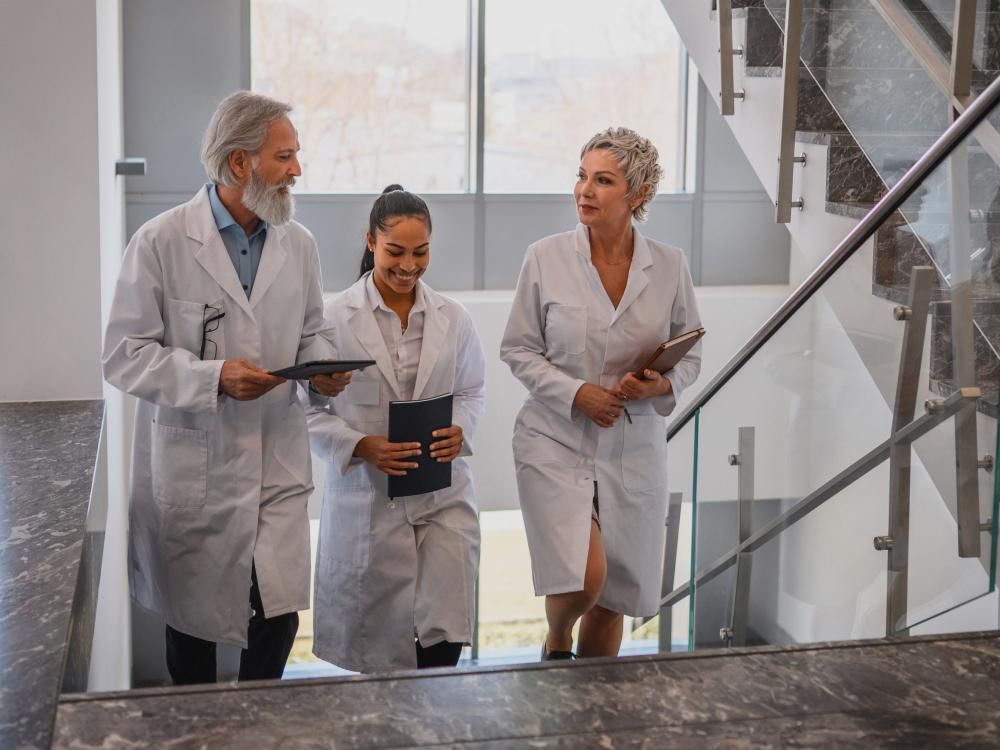
Insights
EHR implementation strategy & a comprehensive roadmap
Discover an actionable electronic health records implementation plan to help you successfully adopt EHR software, increasing your organization’s productivity.
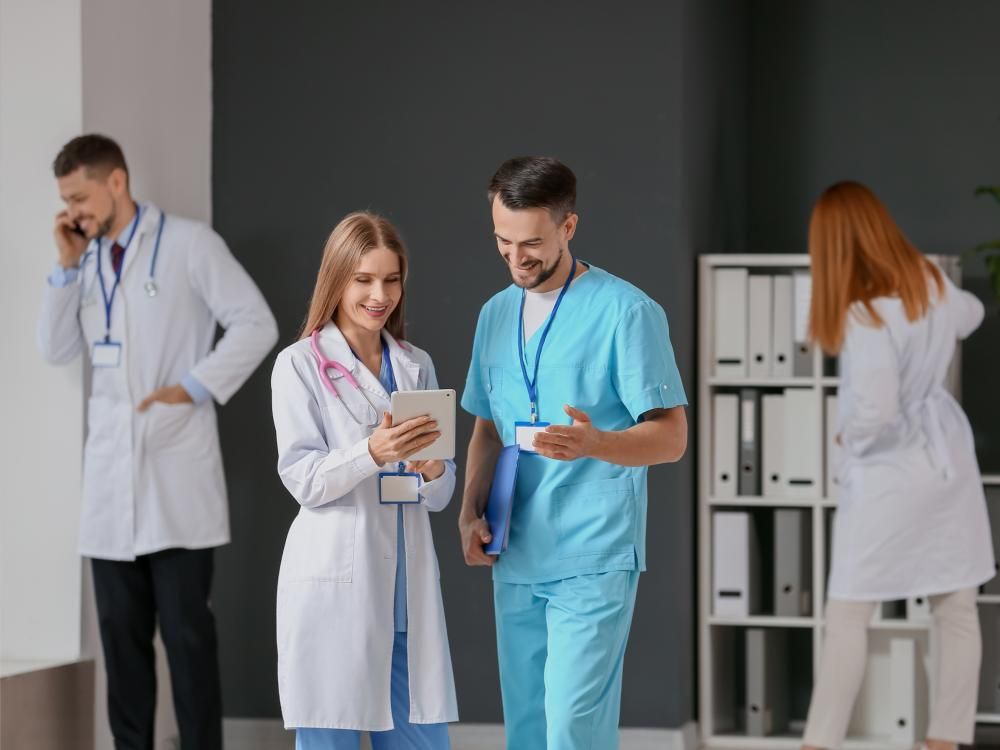
Insights
RPA in healthcare: use cases, benefits, and challenges
Learn how robotic process automation streamlines processes in the healthcare industry and improves care delivery. Explore top RPA vendors, use cases, and costs.
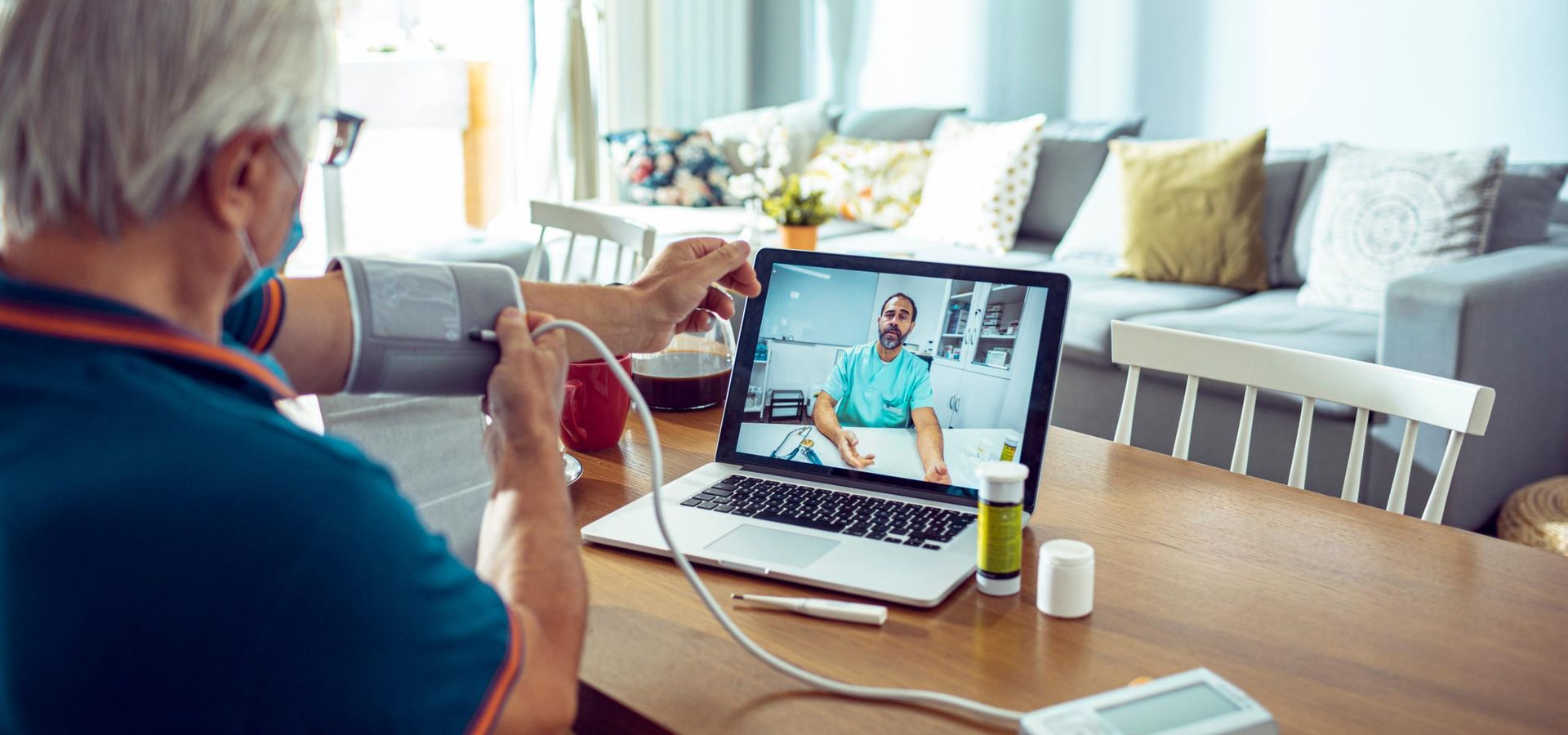
Service
Telemedicine software development: key features, integrations & services
Itransition offers end-to-end custom telemedicine software development and implementation services for healthcare organizations of any size and specialty.
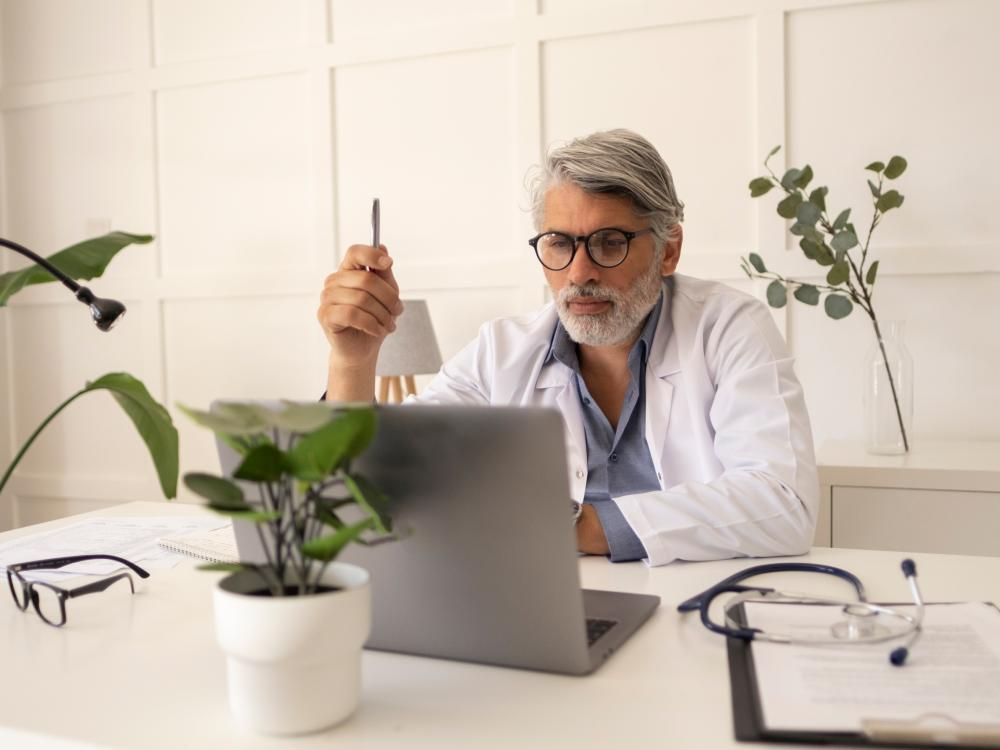
Insights
Virtual hospital: key features, examples, and benefits of remote care
Healthcare providers can expand their services with a virtual hospital. Read more about telehealth solutions to implement them into your virtual care practice.