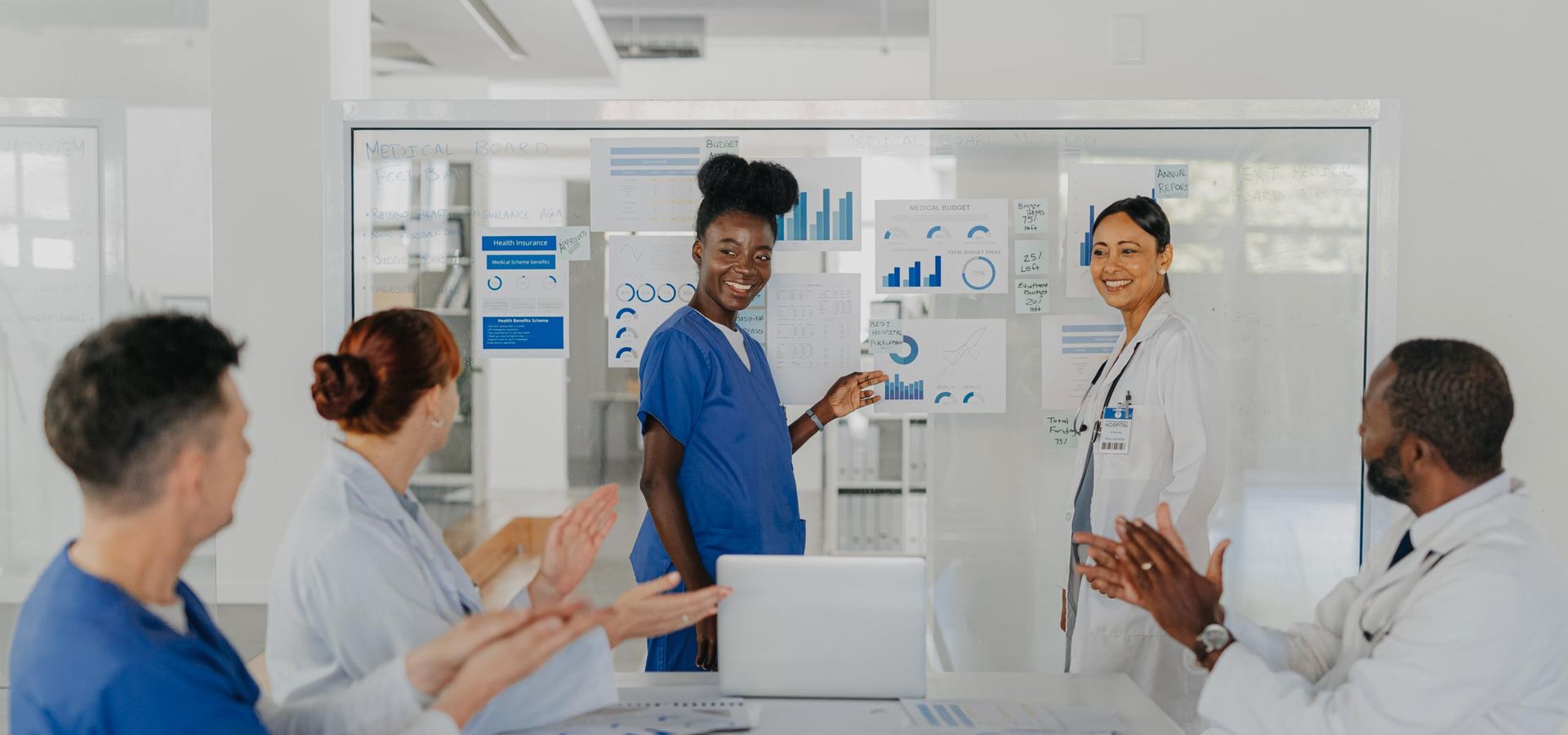
Healthcare data analytics services: key types, features, and applications
- Home
- Healthcare software development solutions & services
- Data analytics
Healthcare data analytics interprets current and historical data from legacy systems, cloud, portable devices, and external sources into actionable insights for medical teams. Itransition builds custom healthcare data analytics solutions using suitable data analysis techniques as well as AI and ML.
Table of contents
of world data volume is generated by the healthcare industry
RBC Capital Markets
of healthcare leaders in the US have adopted predictive analytics
Statista
expected CAGR of the global healthcare analytics market between 2021 and 2030
Precedence Research
Healthcare data analytics options
Big data analytics
Image analysis
IoMT analytics
Business analytics
Data warehousing
Predictive analytics
Data visualization
Population health analytics
Healthcare data analytics services we offer
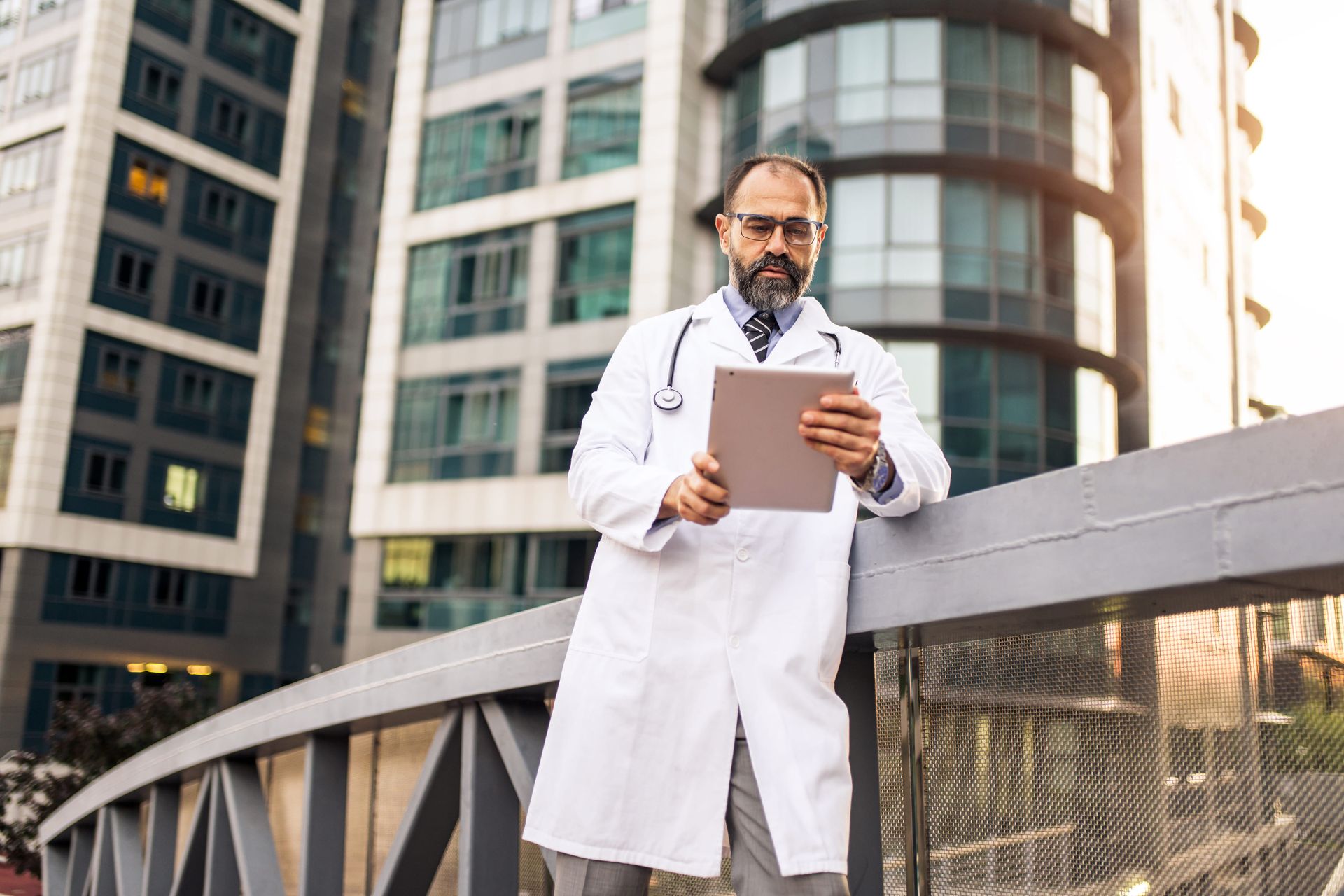
Consulting
Implementation
Migration
Modernization
Compliance
Looking to get actionable insights from healthcare data?
Medical data analytics development scenarios
Our team can follow a data analytics development approach that fits with the particular organization’s goals, available resources, and budget.
Custom solutions
Embedded BI
Platform customization
We implement a third-party analytics platform, adapting it to your business needs. We work with Microsoft Cloud for Healthcare, Microsoft Power BI, Tableau, Qlik, and other platforms.
Itransition’s medical data analytics portfolio
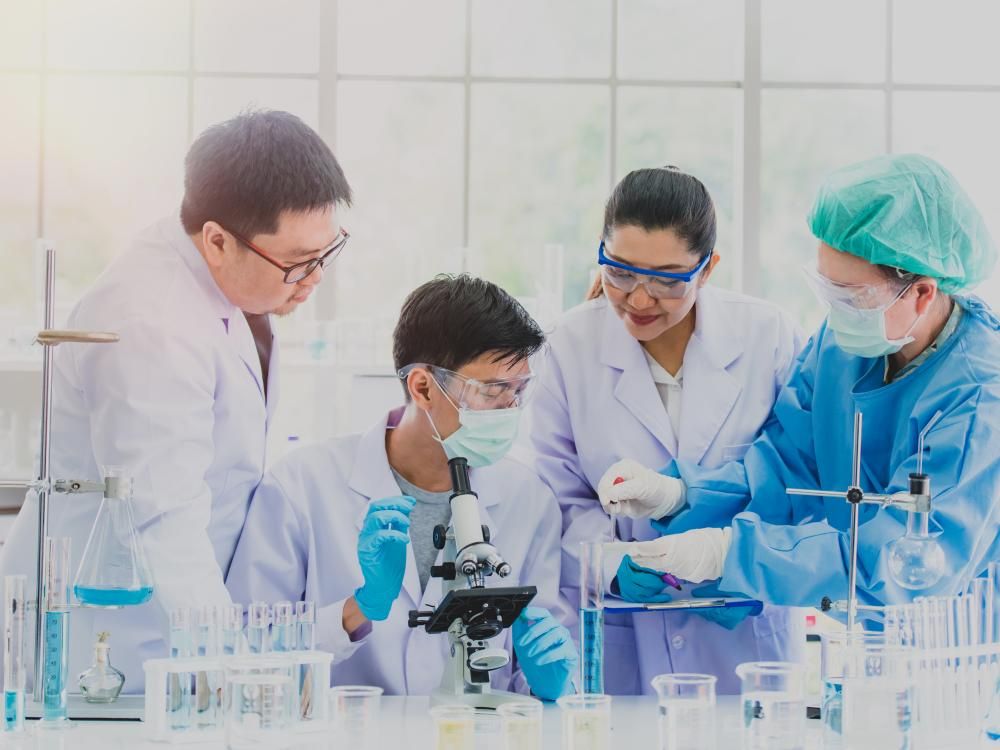
2x
faster test runs
Healthcare analytics applications revamp
We upgraded a suite of healthcare and pharmaceutical analytics solutions, performing development, QA and DevOps services to enhance user experience and boost app performance.
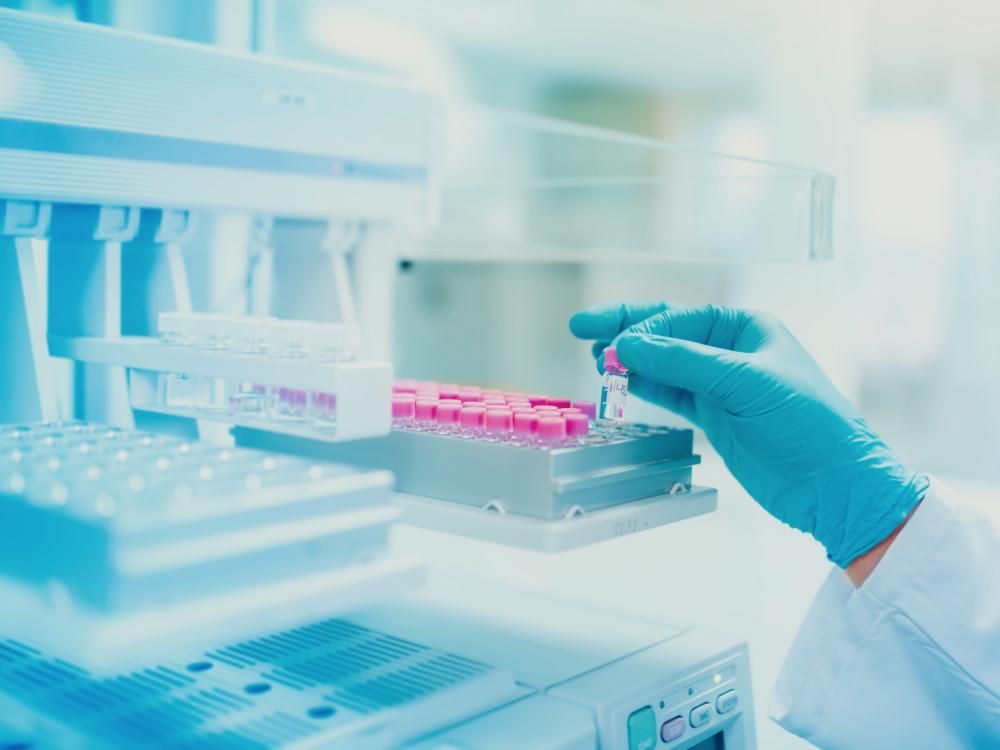
10x
faster data processing
Pharmaceutical data analytics suite
We migrated the customer’s infrastructure to the cloud and redeveloped their data analytics solutions used by pharma corporations globally.
Types of healthcare data analytics
There are five types of data analytics used in healthcare, each with their distinct computing power and data requirements and potential impact.
Predictive
What might happen? (What could complicate the condition?)
Diagnostic
Why did it happen? (What caused the condition?)
Descriptive
What happened? (What does the condition look like?)
Prescriptive
What should happen? (How should we treat the condition?)
Discovery
How do we know it happened? (Which of the patient’s vitals should be used to determine the cause/type/outcome of the condition?)
Top 10 healthcare data analytics use cases
Here are ten most popular applications of data analytics in the healthcare industry.
Treatment planning
Chronic condition management
Self-harm prevention
Resource allocation
Patient load management
Supply chain management
Fraud prevention
Patient engagement
Data security
Benefits of healthcare data analytics
Analytical solutions enable healthcare organizations to use available data to their and patients’ advantage.
Early diagnostics and disease prevention
Patients’ health history analysis can help identify a variety of health risks in advance and prevent upcoming diseases. This allows care providers to avoid hospitalizations, alleviate pressure on the ER and ICU, and speed up patient recovery.
Care optimization and personalization
A more holistic understanding of all patient health factors helps create treatment plans best suited to each patient’s individual case, needs, and health specifics. This improves patient satisfaction, boosts treatment success, and makes care more inclusive.
More efficient operations
Performance analysis helps detect redundant workflows, areas of unproductive resource and personnel usage, and poor management approaches. It also helps establish cost-effective operational strategies, create successful marketing campaigns, and discover opportunities for patient base expansion.
Better crisis management
Predictive analytics recognize signs of an upcoming crisis like an epidemic, personnel shortages, and facilities overcrowding and find effective management approaches, like resources and tasks redistribution, data backup, or patient flow redirection.
Faster drug discovery and rollout
In the pharmaceutical industry, big data analytics and modeling can speed up the processing of research and trial data while also immensely lowering its cost, making new drugs cheaper and safer.
Challenges of implementing healthcare data analytics
Although healthcare analytics solutions give care providers undisputable advantages, their implementation and use may pose some challenges, especially for non-technical professionals.
Common challenges
How we can help
Data formats inconsistency
Data formats inconsistency
With software interoperability yet to be achieved in many healthcare organizations, data coming from different systems is often in disparate formats and therefore difficult to process and share.
Our experts review and clean up a healthcare organization’s datasets as well as audit data quality to make sure all data is ready to be processed. Then we integrate analytical tools into digital environments and ensure their future interoperability.
Storage with slow access to data
Storage with slow access to data
Healthcare organizations generate high volumes of data and sometimes have to opt for cheaper storage options that make it hard to access data.
We build DWH architectures and ETL processes to establish seamless data connectivity, system stability and scalability, taking into account your organization’s requirements, data volumes, and budget.
Data governance
Data governance
A data governance strategy is the best way to ensure data quality and protection for reliable analytics outputs. However, many providers are unfamiliar with such practices.
Our consultants work closely with the organization’s IT department to ensure the adoption and proper execution of all activities aimed at keeping data reliable, structured, accessible, and protected. We can conduct personnel training, monitor system performance, and give recommendations for further data governance enhancement.
Our experts will solve all emerging issues during healthcare analytics development and integration
Related services
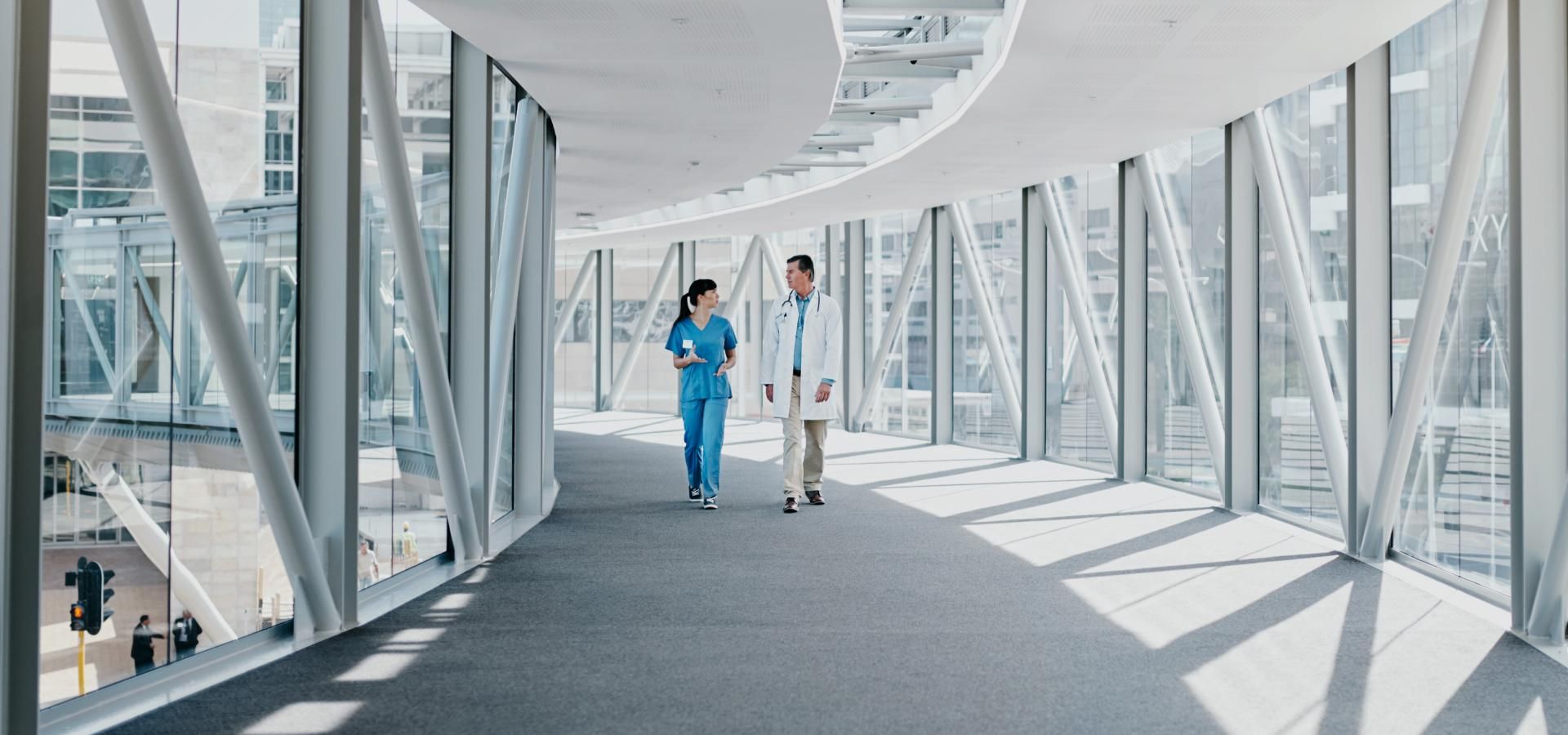
EHR/EMR
We provide software for secure patient data management, storage and sharing, enabling providers to have a full, up-to-date view of patients’ health history.
Healthcare CRM
Our CRM solutions are tailored to the healthcare industry, helping enhance patient experiences and expand service area coverage.
Hospital management software
We deliver solutions that digitize and facilitate all hospital operations, from locating ward equipment to task distribution.
Pharmacy management solutions
Our solutions help pharma companies handle complex procedures from supply chain, order and pharmaceutical data management to dangerous substance control.
Telehealth solutions
We create sophisticated telehealth suites that enable remote consultations, diagnostics, and treatment to make healthcare more accessible and help providers increase revenue.
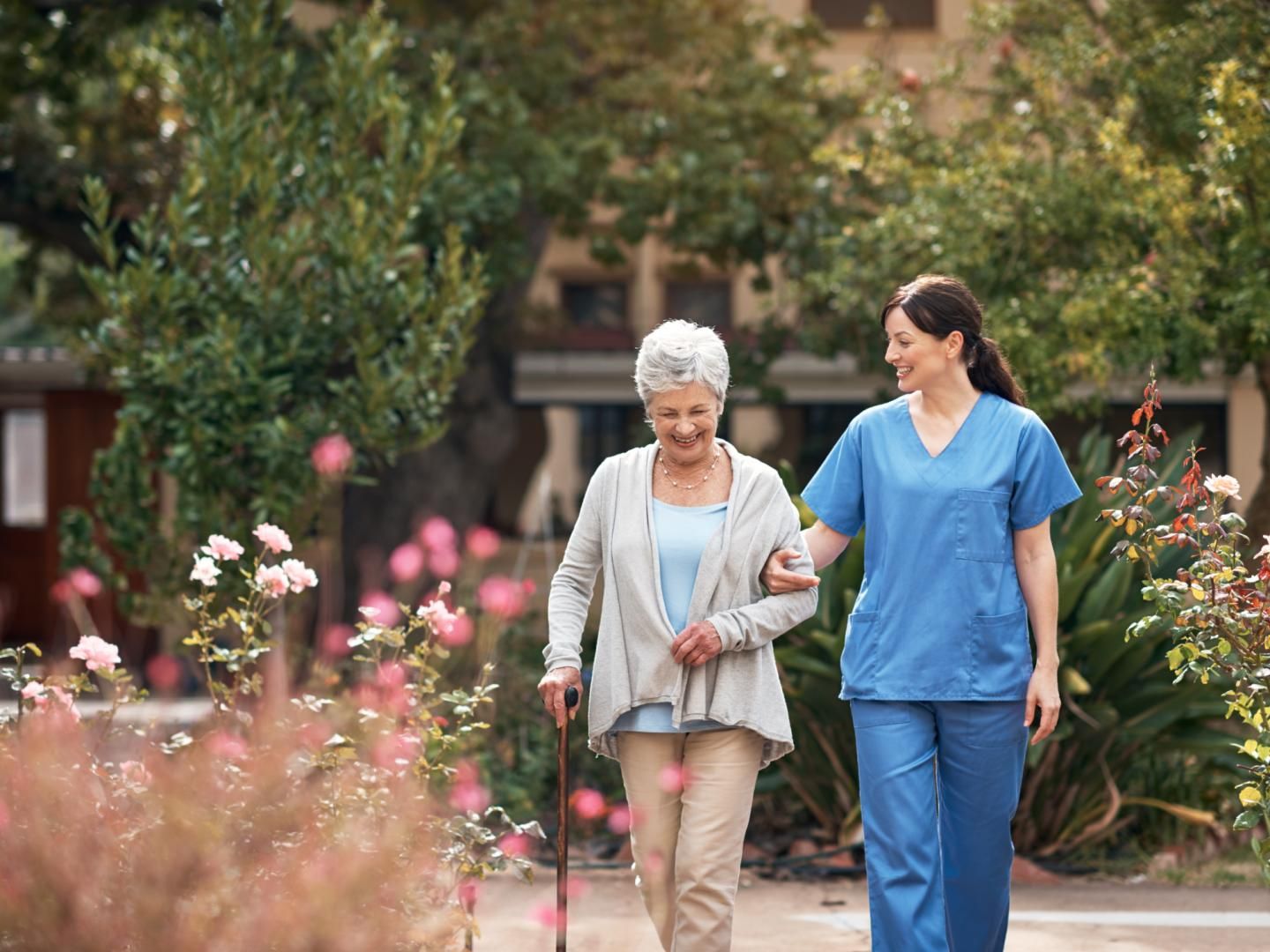
Patient engagement tools
Our patient engagement solutions, including patient portals and mHealth applications, facilitate communication between providers and patients, increase patients’ loyalty and help them proactively manage their health.
FAQ about healthcare data analytics
Here are some questions worth asking before healthcare analytics adoption.
Where does medical data come from?
Depending on your IT ecosystem, analytical algorithms can use surveys, EHR/EMR, CRM, insurance claims, laboratory systems and medical device data.
What is advanced analytics in healthcare?
This term is often used to describe automated data analysis that uses techniques and tools more complex than traditional business intelligence (AI, machine learning, blockchain, etc.).
What algorithms do data analytics platforms employ?
The “big five” algorithms used in medical data analytics are linear regression, logistic regression, classification and regression trees, K-nearest neighbors, and K-means clustering. However, you can ask the vendor what algorithm is used in a particular case.
What is the cost of healthcare analytics solutions?
The cost varies significantly across solutions depending on the functionality and the amount of data they are processing. You’d better discuss the cost with your developer company when you have a solid idea of your future analytical software.
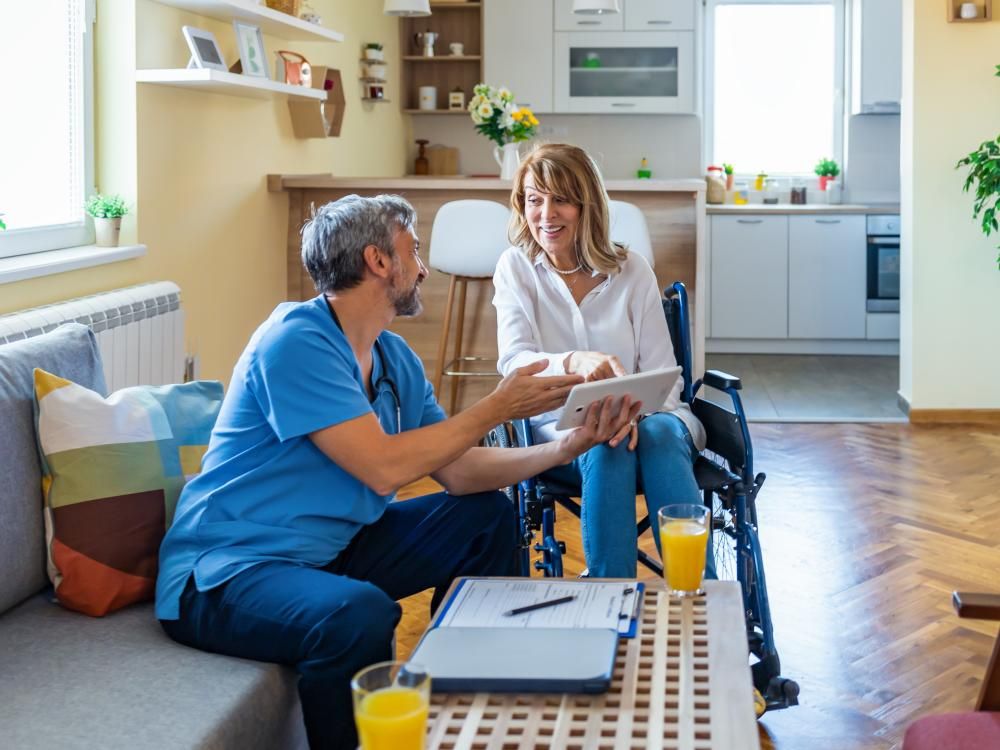
Case study
Oncology treatment platform
Learn how we developed an oncology treatment platform that streamlines therapy order creation and facilitates evidence-based patient treatment decisions.
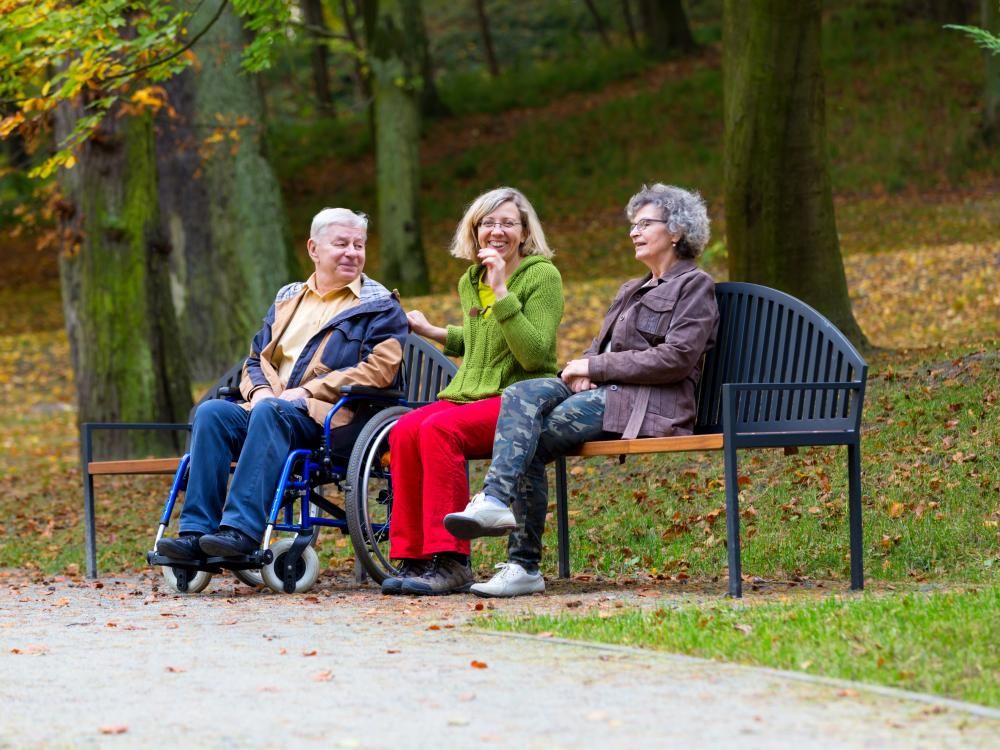
Case study
Custom database development for a healthcare charity
Learn how Itransition’s custom database development provided healthcare professionals with accurate data on defibrillators within their areas.
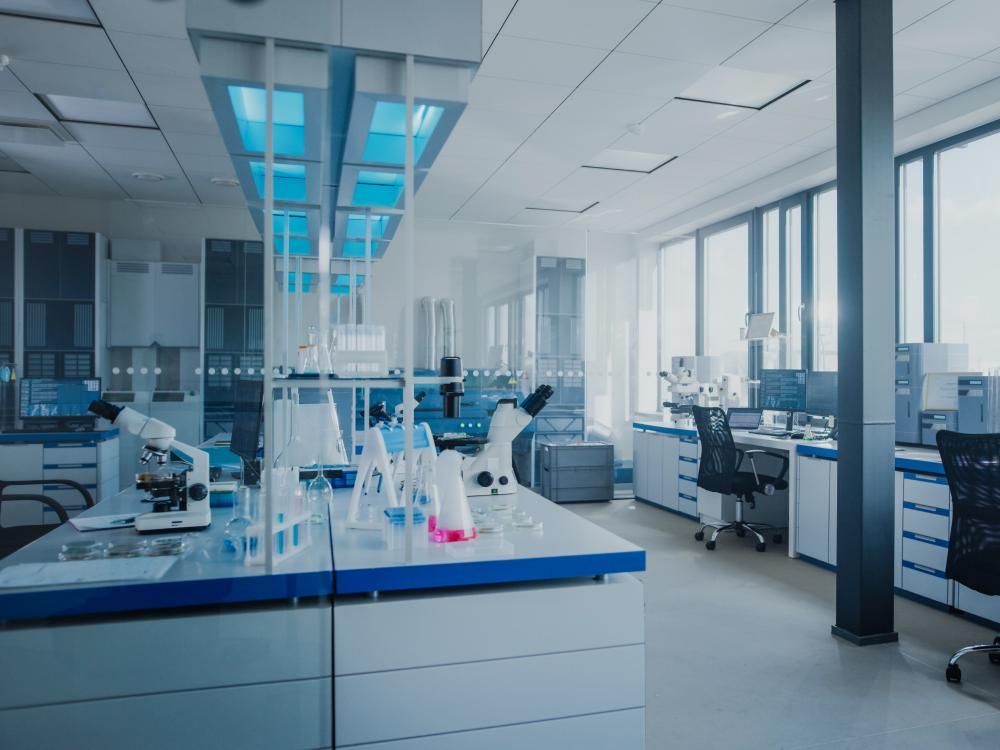
Case study
Medical equipment management software
Learn how Itransition developed seven versions of TOME blood data collection and management software for Terumo.
More about healthcare software services
Services
EHR
Telehealth