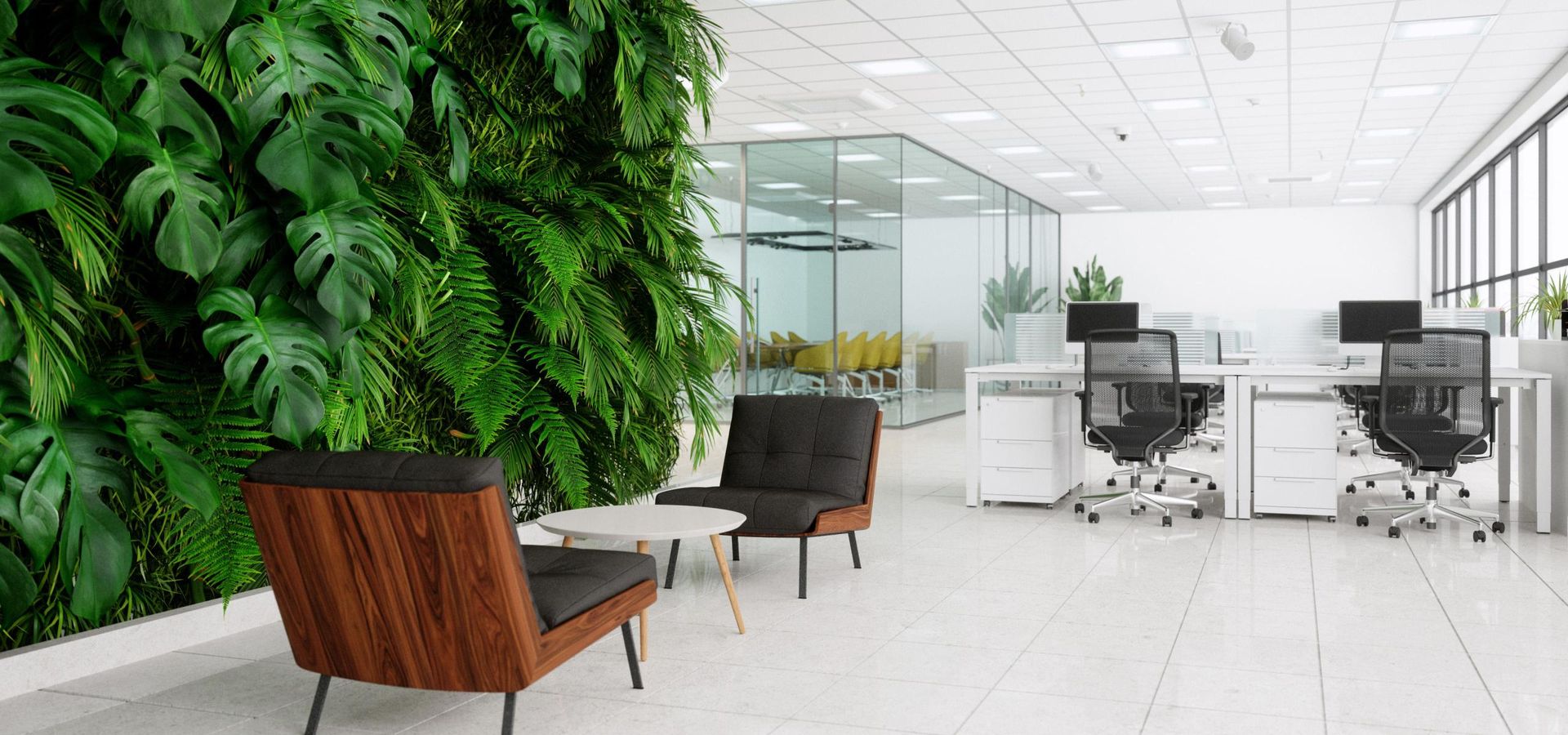
AI in the workplace: 10 key use cases, benefits, and challenges
September 12, 2023
- Home
- Artificial intelligence
- AI in the workplace
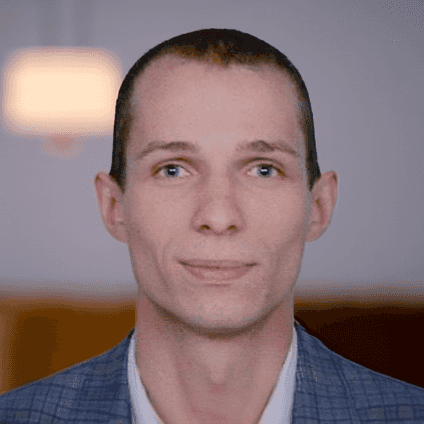
Head of AI/ML Center of Excellence
The implementation of AI in the workplace helps augment staff performance, streamline human resources operations, improve employee experience, and promote cross-team collaboration.
Find out how AI services and software solutions can support your workforce and help your organization achieve its corporate goals.
Table of contents
AI trends in the workplace
57%
of executives would invest in AI and automation in the event of an economic downturn
Mercer’s Global Talent Trends Study, 2023
77%
of workers believe their organizations will provide training on AI-driven job changes
Mercer’s Global Talent Trends Study, 2023
79%
of respondents achieved full-scale deployment for 3+ types of AI applications
Deloitte’s State of AI in the Enterprise Report, 2022
76%
of respondents plan to increase their investments in AI to gain more benefits
Deloitte’s State of AI in the Enterprise Report, 2022
50%
of employees will need reskilling initiatives by 2025 to embrace AI-enabled work trends
WEF’s Future of Jobs Report, 2020
Acquiring AI talent
Acquiring AI solutions
Scheme title: AI talent and software acquisition options
Data source: deloitte.com — Deloitte’s State of AI in the Enterprise, 2022
10 applications of AI in the workplace
From recruiting and onboarding to business process automation, companies are increasingly propelling the adoption of AI in the workplace and its influence on our careers and the labor market.
Headhunting
The effects of AI begin even before we set foot in a new office. For example, predictive analytics software powered by machine learning algorithms can segment and filter suitable candidates based on resumes and relevant metrics, matching their job experience with the required profiles. For instance, the recommendation systems of major job search social media leverage ML, including LinkedIn’s engine and its Recruiter feature for candidate ranking.
Scheme title: LinkedIn’s recommendation system architecture
Data source: The AI Behind LinkedIn Recruiter search and recommendation systems
Job interviews
Several organizations rely on predictive analytics solutions for HR to partially automate and assist job interviews. For example, the British multinational company Unilever adopted an AI-powered hiring system developed by HireVue, which can analyze job applicants’ body language, use of keywords, and tone of voice. This solution enabled them to hire more suitable candidates for different roles, saving 50,000 hours of candidates' time and reducing the hiring process length from 4 months to 4 weeks.
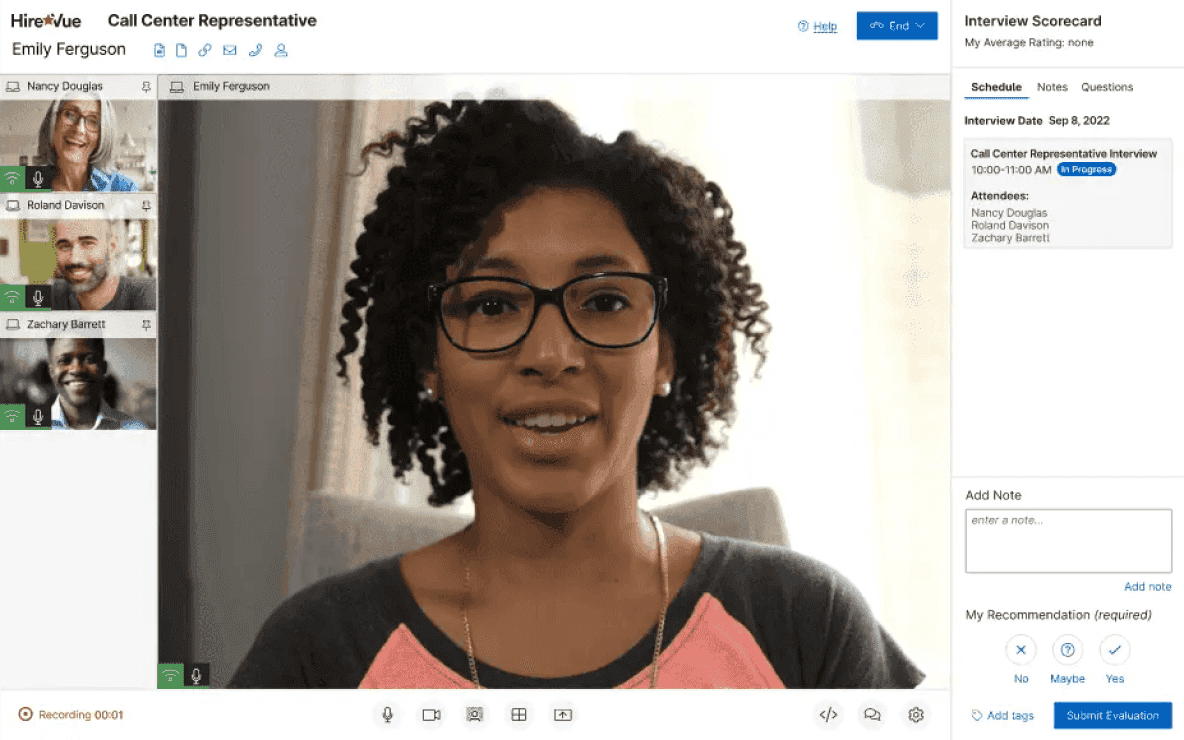
Image title: The interface of HireVue’s AI-based hiring platform
Data source: hirevue.com
Staff performance appraisal
AI-based analytics software can facilitate employee performance monitoring and assessment. The Japanese automotive manufacturer Denso, for example, implemented an action recognition solution developed by Drishti Technologies and powered by AI and computer vision. The system’s main purpose is to analyze manual tasks performed by production employees in real time and provide workers and managers with insights into bottlenecks and downtimes, helping to fine-tune processes and improve efficiency.
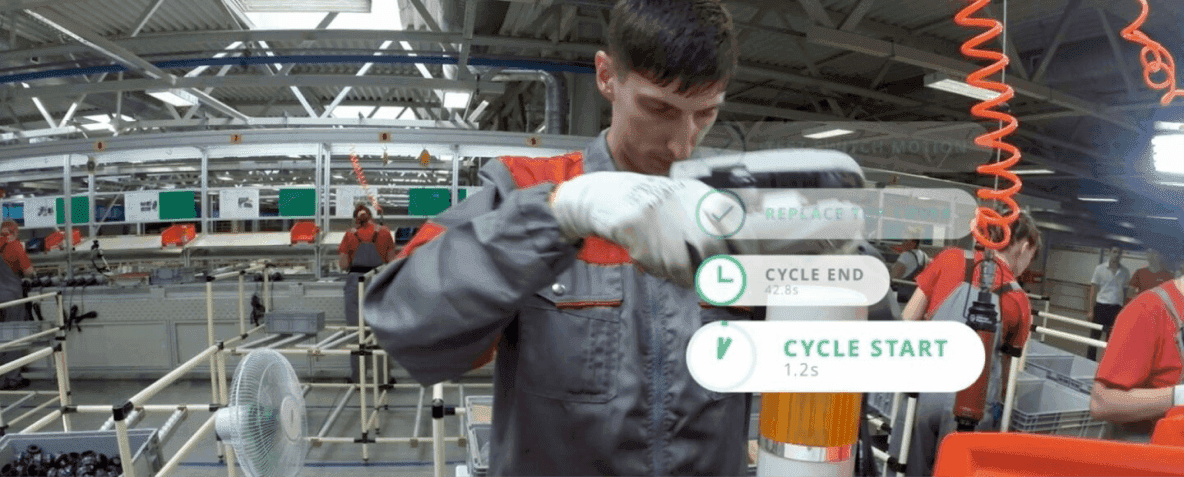
Image title: Drishti’s action recognition solution deployed in an assembly line
Data source: drishti.com — Introducing Drishti: Making factory workers more competitive in an increasingly automated world
Workforce development
The growing adoption of educational AI tools and machine learning algorithms in education can also catalyze employees’ professional development. For instance, the Faethm data analytics platform deployed by Mastercard monitors employee performance to offer personalized suggestions on learning strategies and career progression. Honeywell, on the other hand, implemented a training solution named Skills Insight combining analytics, IoT, and VR to provide task simulations.
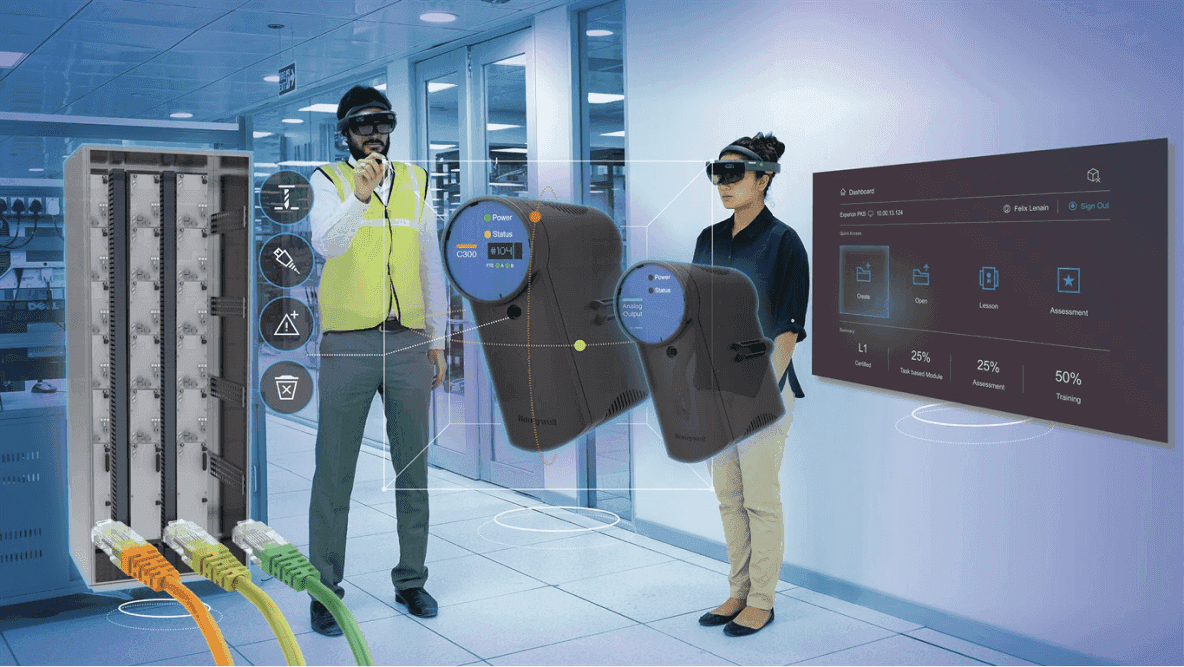
Image title: The operation of Honeywell’s Skills Insight
Data source: apps.microsoft.com — Skills Insight Immersive Competency
Process automation
The automation of business processes involves multiple industries and corporate functions. We can find examples of automation in robots applied across several manual processes. For instance, Mitsubishi Electric deployed AI-based machines in its assembly lines, increasing production by 30%. At the same time, clerical tasks can be automated through RPA. For example, Deloitte reduced report preparation time from 8 days to 1 hour via software bots.
Reduction of agents’ workload
In this approach, AI enhances and supports employees, especially in such customer-facing roles like support agents, during peak hours so that machines' accuracy and speed merge with human intelligence and empathy. The US CDC, for instance, implemented an AI-powered chatbot based on Microsoft’s Healthcare Bot, which autonomously provided information and suggestions, freeing up doctors and nurses to focus on the most critical cases of COVID-19.
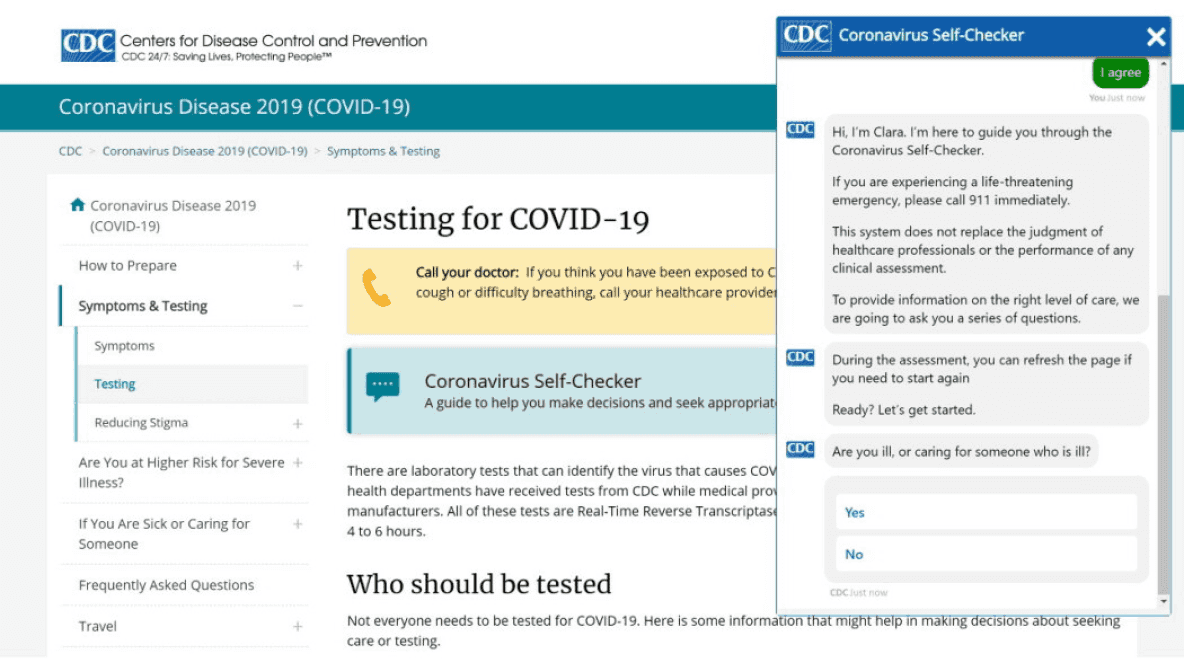
Image title: CDC’s COVID-19 assessment bot
Data source: pcmag.com — CDC Creates Coronavirus Chatbot Called Clara to Check Your Symptoms
Customer relationships
AI can help your staff connect with clients and personalize customer experience. This involves data-driven tools and practices such as lead scoring, customer segmentation and targeting, sentiment analysis, chatbots and smart assistants, AI-based CRM, and more. For example, Vodafone Italy embraced a similar approach, generating customized promotional messages via natural language processing and increasing customer subscriptions by 40% in 2020.
Staff safety and well-being
AI helps ensure staff well-being and working environment safety in various operational scenarios. AI solutions are commonly deployed in manufacturing facilities, where computer vision systems can oversee workers and machinery to identify potential hazards. Speaking of offices, startups like Erudit AI developed workforce intelligence software to detect signs of distress based on employee behavioral and communication patterns.
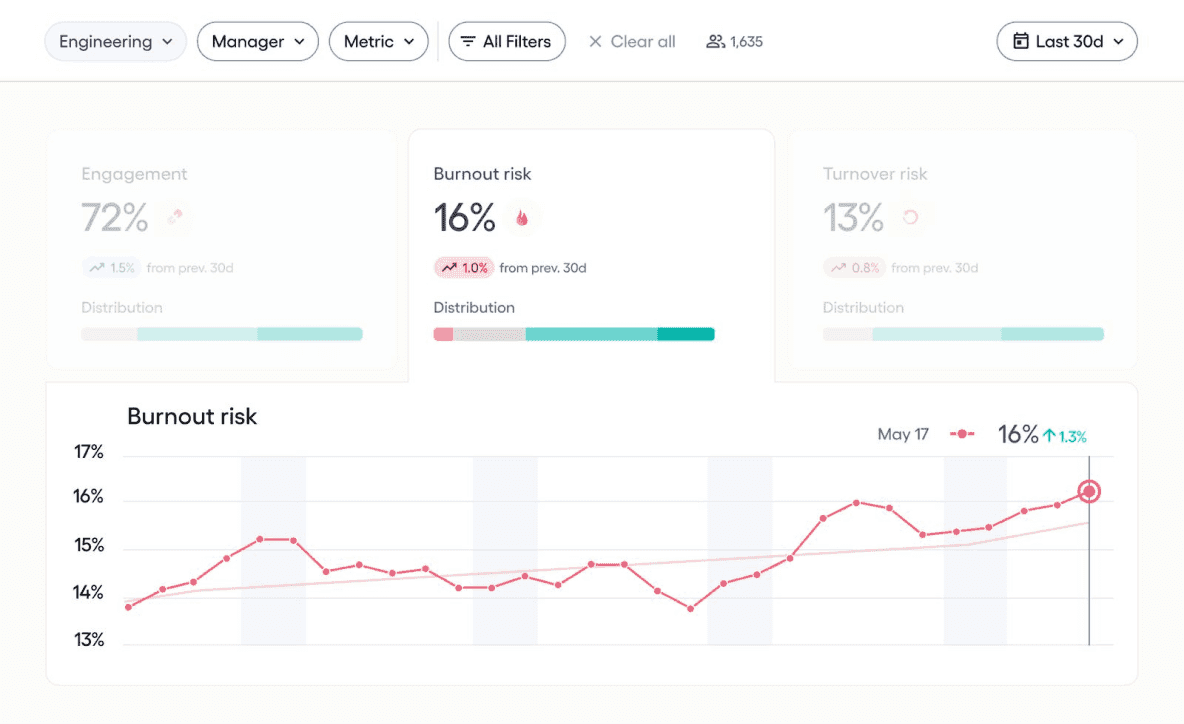
Image title: Erudit’s burnout detection tool dashboard
Data source: erudit.ai — Detect teams at high risk of burnout
Benefits management
To meet the needs of a more diverse workforce while maximizing talent retention, many companies have opted for an AI-driven and more tailored approach to benefits management. Four out of five companies, according to Mercer, already use or plan to implement AI-based benefit enrollment platforms. The one developed by Nayya Health, for instance, provides employees with personalized benefits suggestions, including health insurance and retirement plans.
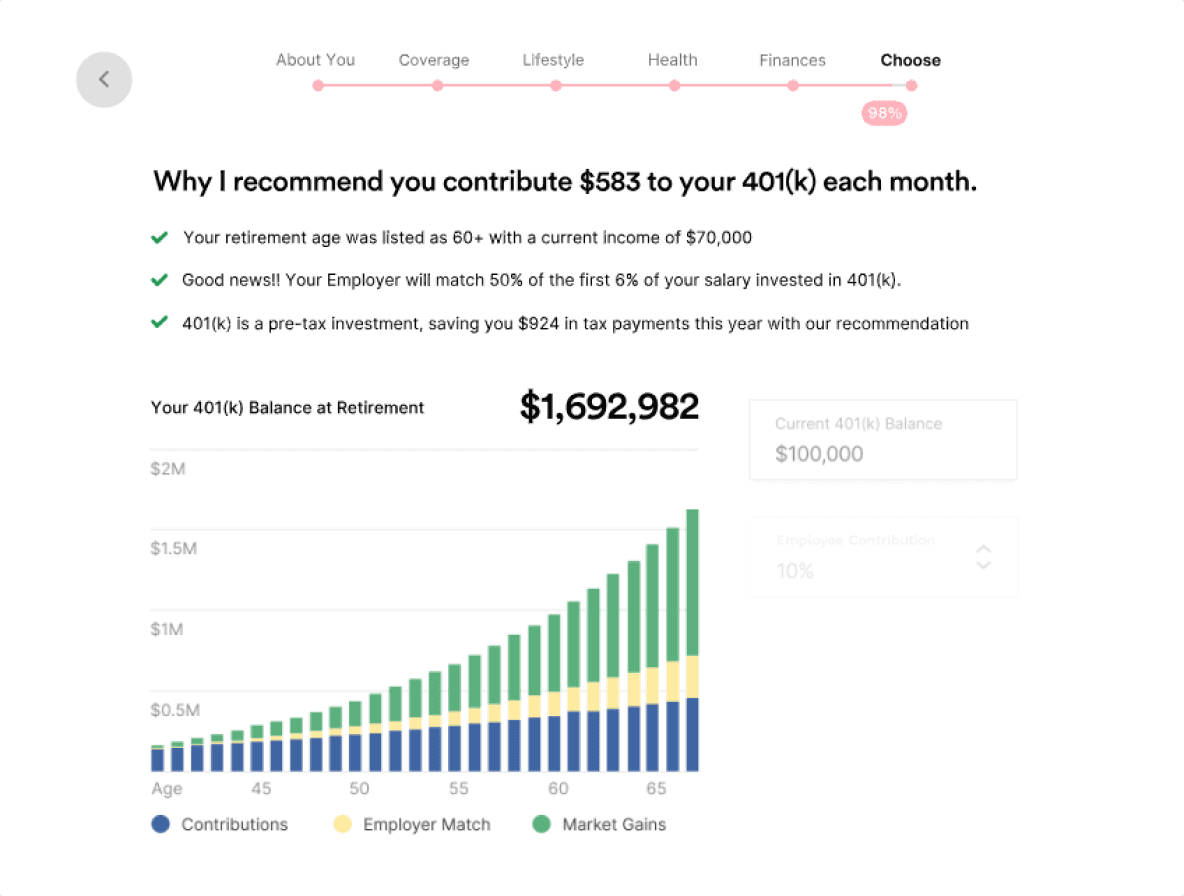
Image title: Nayya’s AI-powered benefits recommendation platform in action
Data source: nayya.com
Corporate innovation
AI implementation can foster creativity and drive innovation in corporate scenarios. Organizations like Airbus, for instance, have adopted Autodesk's generative design software, an AI-based tool to complement human inventiveness. The application provides multiple design alternatives based on a list of parameters set by the designer, suggesting shapes, materials, and manufacturing methods. Combined with 3D printing, Autodesk also simplifies the prototyping process.
Our team will augment your workflows with AI
Benefits of AI in the workplace
Adopting artificial intelligence and related technologies in a business scenario can benefit both your staff and the organization as a whole, resulting in enhanced performance, cost optimization, new job opportunities, and a better work experience.
Increased productivity
Deloitte’s 2023 Global Human Capital Trends Report highlights that artificial intelligence and machine learning will contribute to a 37% increase in labor productivity by 2025. In fact, data-driven decision-making will enhance human impact at work and organizational performance.
Cost reduction
According to Bain & Company’s 2023 The Augmented Workforce Report, intelligent automation will lead to a greater focus on higher-value work (including problem-solving, creativity, and interpersonal communication) and a 21-30% cost reduction.
New job positions
The World Economic Forum estimated that by 2025, AI-powered automation could create 97 million new jobs (data analysts and scientists, business development professionals, digital transformation specialists, etc.), compared to the 85 million lost (such as accountants or data entry clerks).
Employee satisfaction
In its 2022 State of AI in the Enterprise Report, Deloitte pointed out that 82% of respondents expect AI to enhance job satisfaction. Specifically, many AI adopters see value in leveraging automation to reallocate workers from repetitive tasks to creative activities.
Bias-free decisions
Adopting AI-powered analytics helps minimize human bias and its impact on workplaces and careers. From automated candidate screening to accurate, data-driven staff performance assessments, professionals can expect a fairer approach to recruitment and talent management.
Accuracy and compliance
While AI's reliance on data (including sensitive information) can raise concerns among regulators and should be addressed carefully, its adoption can be beneficial for corporate compliance. For example, AI-based RPA can increase reporting consistency and accuracy by about 80%, according to Deloitte, while lowering the number of employees who have access to sensitive information.
Risk mitigation
AI safeguards your workforce from operational risk in industrial scenarios via anomaly detection and predictive maintenance, minimizing the likelihood of failures and consequent disasters. It can also identify signs of fatigue or psychological discomfort to trigger targeted support initiatives.
Inclusive workplaces
AI fosters workplace diversity and inclusivity by enabling data-driven skill assessments and blind hiring. For instance, AI-based talent intelligence platforms can rank candidates based on their actual expertise while masking identifiable attributes and protected traits (age, gender, disabilities, etc.).
AI implementation roadmap in the workplace
Organizations implementing AI in their workplaces usually go through these key steps, which can vary based on their business scenarios, selected use cases, and technologies involved.
1
Business needs analysis
Assess business needs and expectations via discovery workshops, interviews, and process observations
Audit the existing technical environment
Highlight the project's scope, objectives, deliverables, and timeframes
Define the future solution’s functional and non-functional requirements
2
Initial data analysis
Carry out an exploratory analysis to map and assess available corporate data sources
Identify external data sources, such as public databases
3
Solution design
Design the AI solution’s architecture, main modules, and features
Define a project plan, budget, and timeline
Identify a suitable tech stack based on the technical and business evaluation
Optionally deliver a PoC to ascertain the AI solution’s feasibility, financial viability, and potential limitations
4
Building the AI solution
Carry out data pre-processing, including data cleansing, annotation, and transformation
Outline the solution’s assessment criteria
Train one or more AI models via supervised, unsupervised and reinforcement learning to achieve the desired output
5
Integration and rollout
Integrate the AI model into the solution to power its features with the model’s output
Deploy the product to the target environment (on-premise or in the cloud)
6
Support
Retrain the AI model with new data over time to enhance the accuracy of its output
Perform ongoing maintenance, fixes based on user feedback, and upgrades with new features
Challenges and guidelines for adopting AI in the workplace
Despite the payoffs unlocked by the use of AI in the workplace, adopting and scaling it across your organization can involve a range of business and technical challenges. Here are a few tips to mitigate such issues while maximizing the value of AI for your workforce.
Barriers
Insufficiencies
Difficulties
Ethical risks
Scheme title: Barriers and risks when adopting AI in corporate scenarios
Data source: deloitte.com — Deloitte’s State of AI in the Enterprise, 2022 deloitte.com — AI for work relationships may be a great untapped opportunity, 2022
Use case identification
Issue
Implementing AI solutions can be very impactful but also time-consuming and financially demanding due to their complex architectures, processing power requirements, and long algorithm training times. Deciding whether AI represents a better option for your use case over conventional technologies is essential for making its adoption worth the effort and investment, maximizing ROI and ensuring stakeholders’ and executives’ buy-in.
Recommendations
It makes sense to implement AI when facing serious inefficiencies affecting key business processes, which cannot be solved with "traditional" software solutions. Investing in AI can also be a good choice when it comes to improving the most profitable corporate functions depending on your industry. For example, McKinsey mentions supply chain management for retailers, product development for high-tech corporations, service operations in telecommunications industries, product assembly in the automotive sector, and risk management for financial services.
Skill gap and job disruption
Issue
Widespread automation can spark uncertainty and concern due to skill gaps and potential job losses, causing skepticism and resistance to AI adoption among your staff. Indeed, Mercer points out that according to executives, only 45% of their employees could adapt to a job market reforged by AI. Still, the prospect of simply replacing workers with new, AI-ready staff is inviting but also naïve in a job market notoriously affected by talent shortage.
Recommendations
The WEF stressed the importance of proactively investing in staff retraining and upskilling to reallocate the affected workforce to new positions opened through AI-driven innovations. Specifically, the focus should move from manual skills to technological, cognitive, and emotional competencies. Role transitions can be complemented by external partnerships with experts in relevant fields, including AI and data science. The rapid spread of remote work models fostered by collaboration tools and virtual workplaces can help in this regard.
Workflow harmonization
Issue
Some enterprises struggle to harmonize AI tools with existing operations and workflows, which leads to further inefficiencies and bottlenecks instead of synergies and performance gains. For example, an ecommerce company can enhance its marketing strategies with AI-driven user segmentation and targeted ads to boost sales while disregarding order management, inventory, and other functions to fulfill the consequent increase in orders.
Recommendations
Your operating model should evolve to accommodate AI software. Consider establishing centers of excellence and appoint the most skilled professionals to coordinate workflow adjustments. You can also opt for a progressive adoption, allowing your staff to get familiar with new technologies, collecting feedback from initial deployments, and gradually updating legacy processes. Another way to balance out different workflows is through BPM best practices and platforms, such as Pega and Odoo.
Data and AI model reliability
Issue
AI model training is a complex procedure, and relying on low-quality, inconsistent datasets offering an incomplete vision over key trends can prevent algorithms from identifying patterns across data points. This, in turn, can lead to biased analyses and inaccurate predictions once the model is deployed. Furthermore, its performance can degrade over time due to changes in input features and relationships among variables (the so-called ML model drift).
Recommendations
You should train your model on reliable, high-volume data from selected sources. This requires data mapping, cleansing, and transformation via ETL pipelines, along with its consolidation in a data lake or data warehouse to create a single source of truth. Prepared data can be divided into training, validation, and test sets to split modeling into multiple phases and mitigate overfitting issues. After deployment, the model should be monitored and fine-tuned with “fresh” datasets in multiple iterations based on MLOps best practices.
Data privacy and security
Issue
Given AI's data-centric processes and policymakers' growing interest in data protection, companies should effectively address privacy concerns and cope with the higher risk of cyber exposure. In fact, AI solutions can handle sensitive information, such as financial transaction data, IP, or trade secrets, which can become the target of frauds, hacking, and breaches. Moreover, integrations with external systems can further amplify such issues.
Recommendations
Protect your AI solution and data with solid cybersecurity features. These encompass identity and access management, multi-factor authentication, user activity monitoring, and encrypted data exchange via cryptographic protocols (such as Transport Layer Security or X.509 certificates). Also, adopt a solid data governance strategy, including best practices and internal policies to properly manage your data assets, and ensure your software complies with the US HIPAA, European Union’s GDPR, or other applicable standards.
System integration
Issue
AI-enabled analyses and operations require a stream of information from multiple corporate systems (including legacy software and IoT devices), which can handle various data types or formats and use different communication protocols, namely sets of rules defining how to transmit or exchange data across a network. When such systems are fragmented and do not interact properly, the result is information silos, data inconsistencies, and inaccurate insights.
Recommendations
You should integrate your systems via APIs. Cloud data integration services offered by major cloud providers (such as AWS Glue or Azure Data Factory) and iPaaS platforms (including Informatica, Boomi, and Workato) facilitate this process by providing pre-built APIs. You can also rely on data virtualization techniques or build middleware architectures like ESBs to convert different protocols. Whenever possible, however, adopting systems with shared protocols or complying with your industry's interoperability standards can be the best option.
AI and the future of work
In recent years, together with other technological advances, AI has reshaped our physical and digital workplaces. However, its adoption may raise concerns due to potential skill gaps, along with the tension between regulations and AI’s need for big data. To address these and other implementation challenges, rely on Itransition's expertise in AI consulting and development.
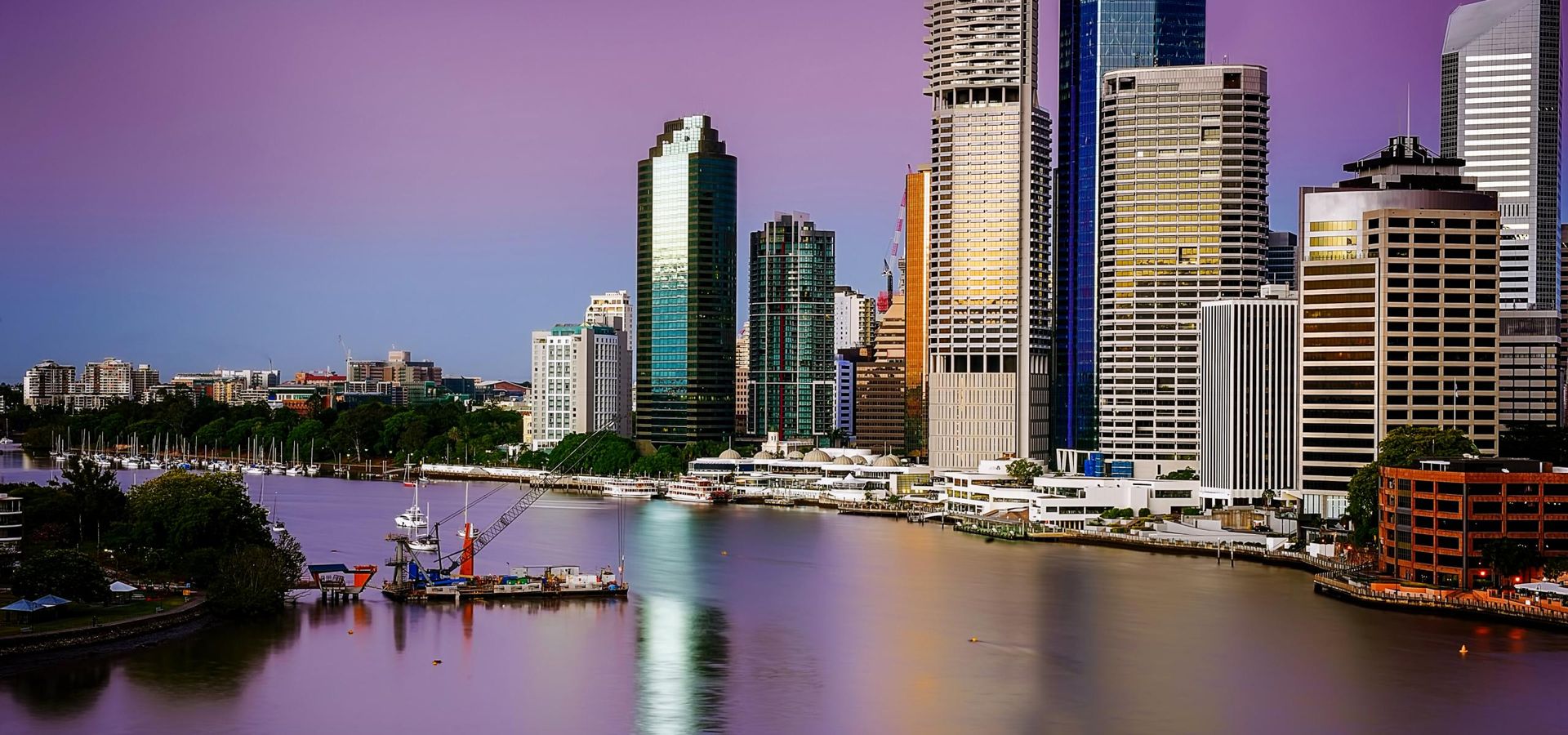
Service
Digital enterprise
Our team implements solutions increase enterprise efficiency through reduced human effort, minimized resource consumption, and expedited workflows.
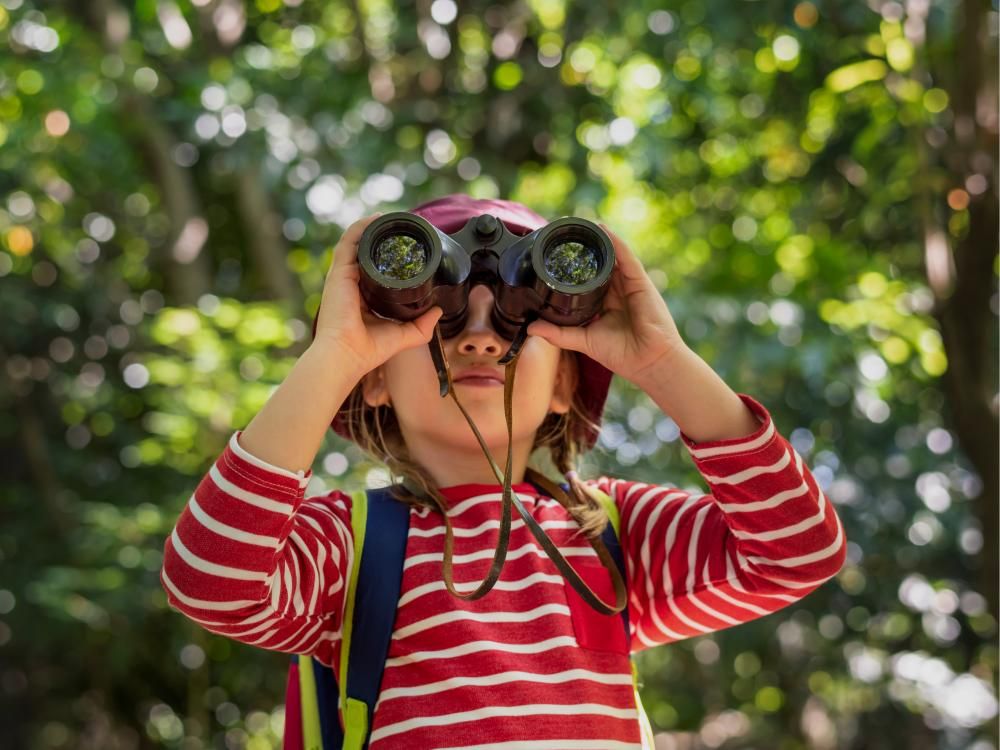
Insights
RPA in human resources: 10 use cases, examples & best tools
Explore popular applications and real-life examples of robotic process automation for human resources management and learn best implementation practices.
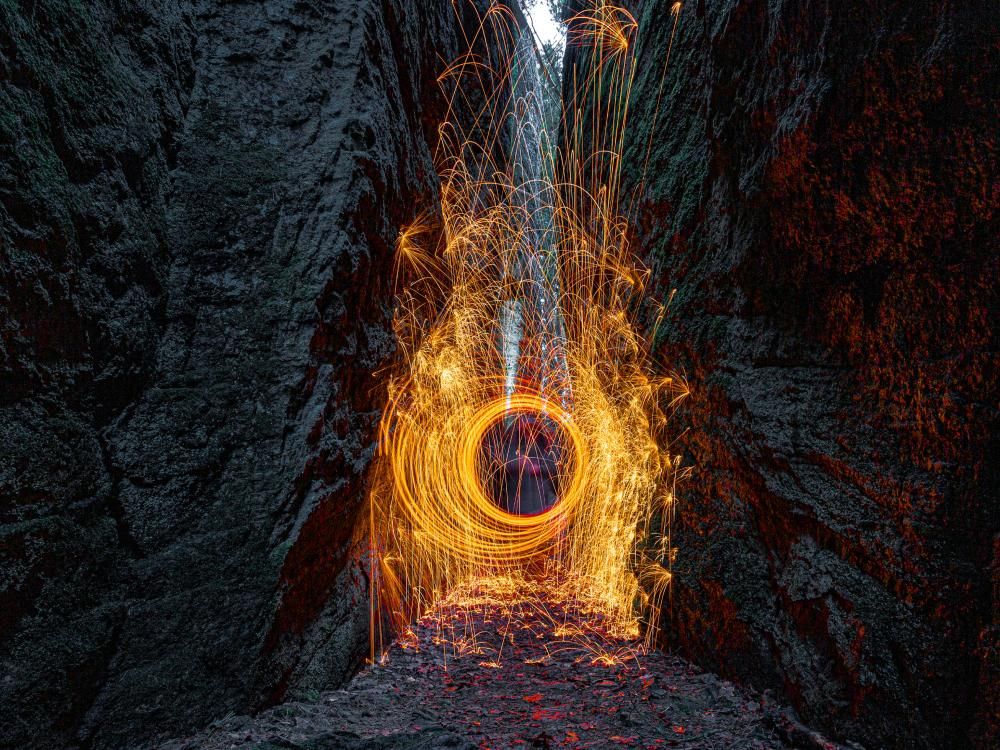
Insights
Business process automation benefits and applications
Find out about the major business process automation benefits and why the technology is worth adopting.
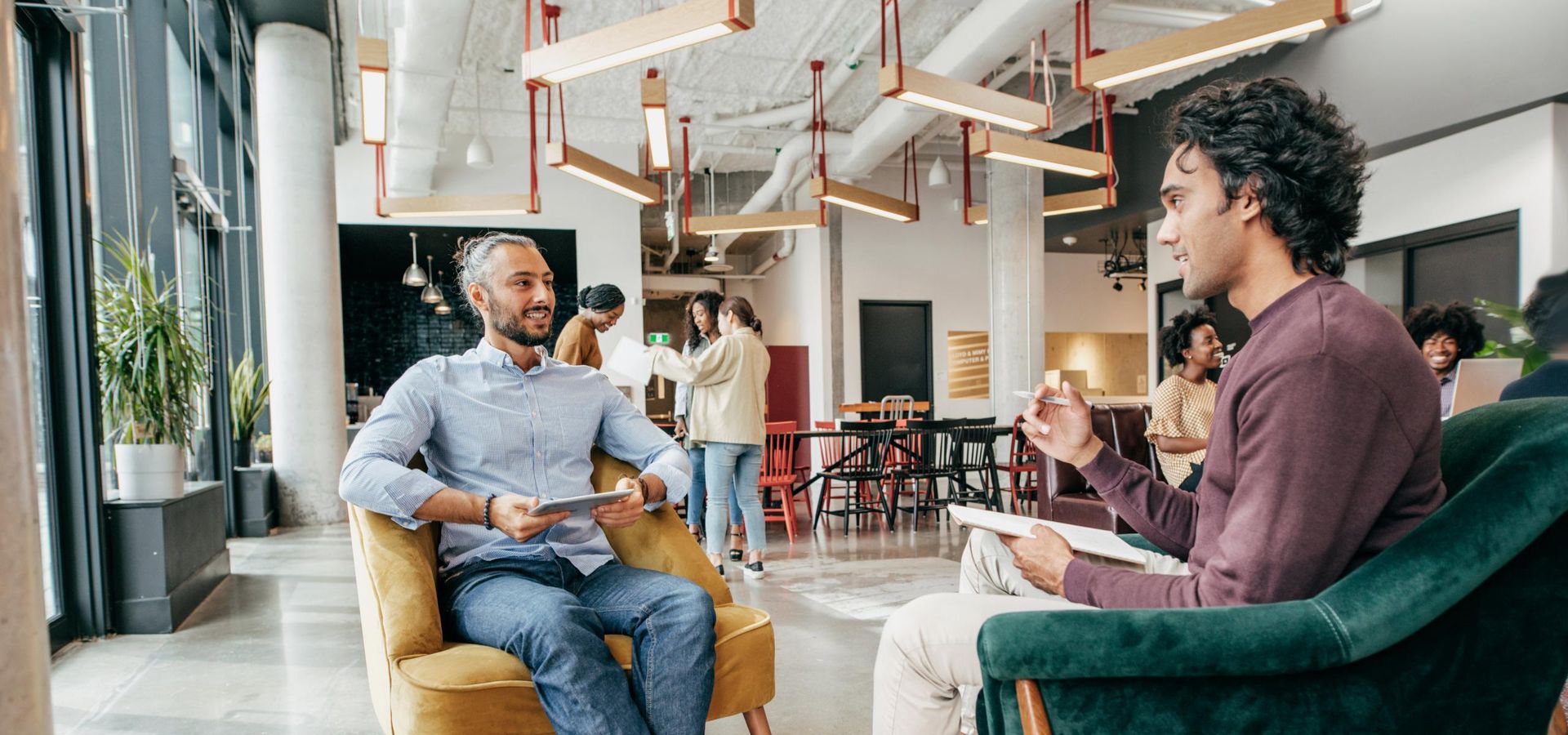
Service
HR data analytics
Discover HR data analytics' top use cases, key metrics, and enabling technologies, as well as Itransition's range of related services.
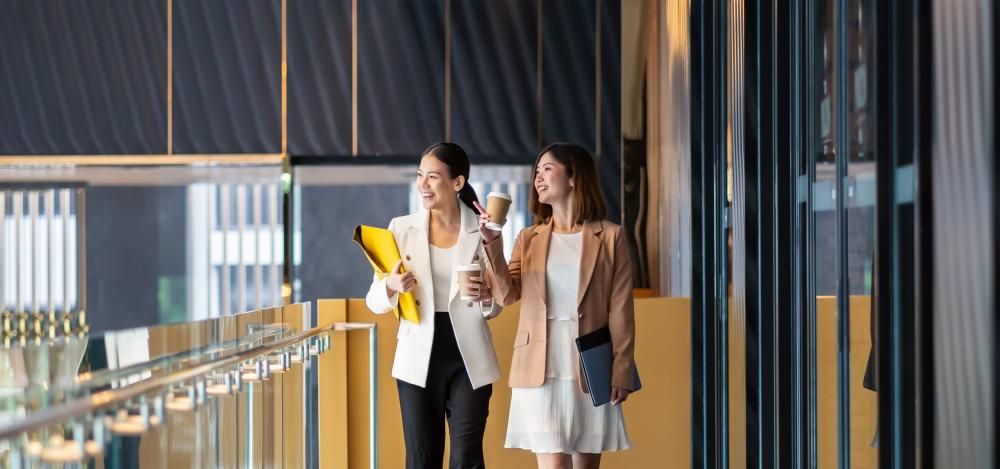
Case study
Robotic process automation HR PoC
Learn how Itransition developed an RPA bot that automates addition of new candidates to the HRM, significantly increasing process efficiency and reliability.