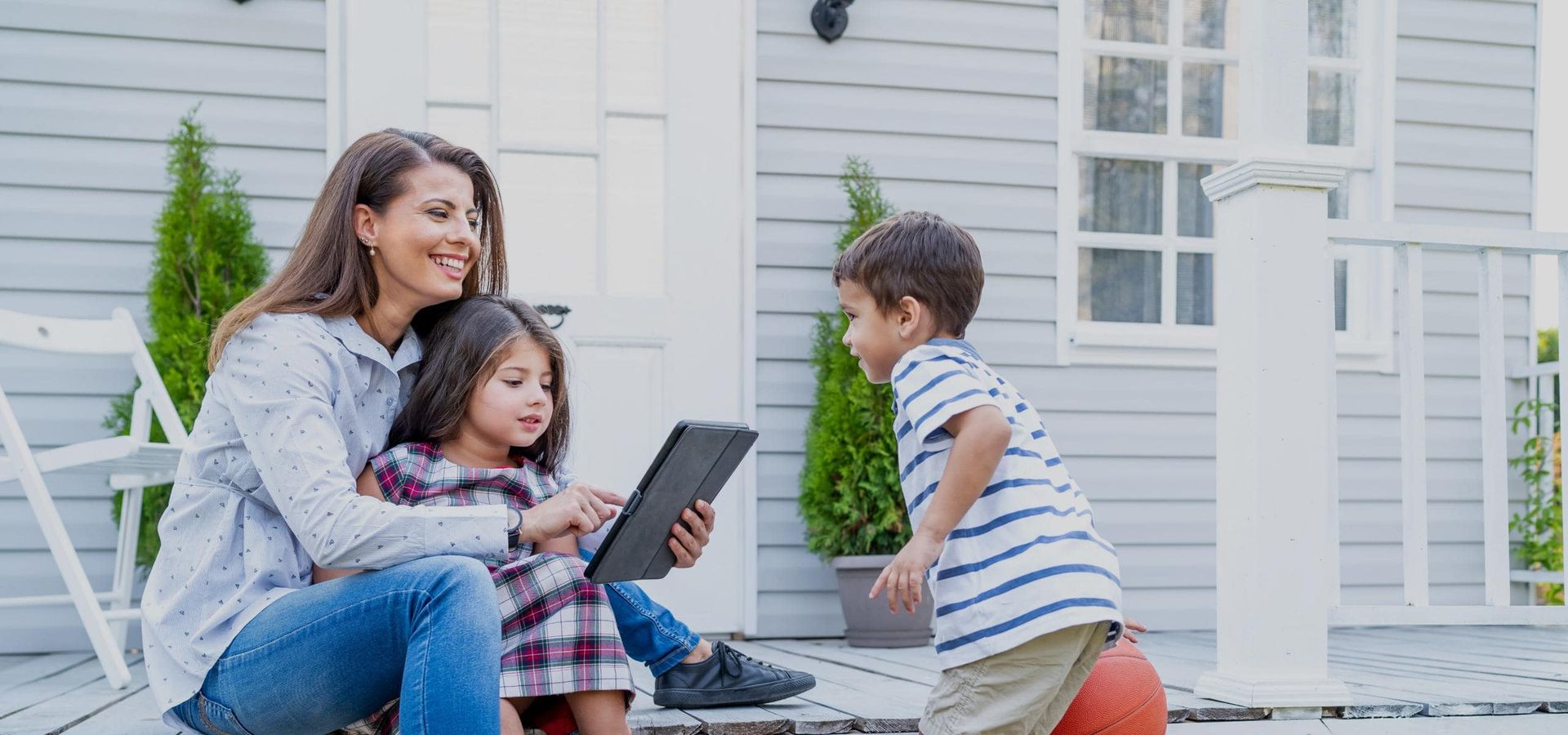
Machine learning in education: 10 use cases, examples, and benefits
June 23, 2023
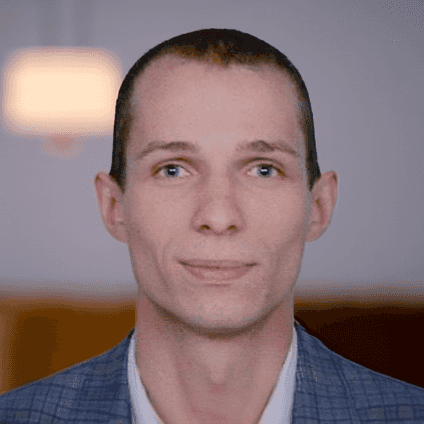
Head of AI/ML Center of Excellence
Let's explore how machine learning developers can improve multiple processes in the educational sector on the most popular use cases and real-life examples. Then, consider the potential challenges of its implementation and see how machine learning experts can solve them.
Table of contents
The role of ML in the educational sector
Machine learning technologies boost students' academic performance. According to McKinsey, 71% of students cited machine learning-powered teaching assistants as positive innovations in their educational process.
Scheme title: Students’ appreciation of learning technologies
*This technology helps me to improve my learning and my grade
Data source: mckinsey.com — Machine learning meets higher learning, 2022
$30B
predicted worth of the AI in education market by 2032, including ML
Global Market Insights
20%
the ML share of the AI in education market revenue in 2022
Global Market Insights
Reinvent education with Itransition’s machine learning solutions
ML for education: key functions
A sub-branch of AI technologies used in education, machine learning encompasses a comprehensive array of learning and training tools powered by self-improving deep learning algorithms that can process big data, identify key metrics, recurring patterns, and relations among variables, and therefore understand how such factors influence the core trends and scenarios in this sector.
EdTech service providers, corporate trainers, and educational institutions typically use machine learning in two ways:
Analytical functions
Some ML-based solutions use machine learning algorithms to analyze education-related data and provide valuable insights to enhance the learning experience. These include the recommendation engines of eLearning platforms, adaptive learning applications, and data analytics software for student performance tracking.
Operational functions
Key areas of AI application like natural language processing (NLP) and computer vision rely on machine learning to identify linguistic and visual patterns and autonomously learn how to replicate innate human capabilities, such as sight and communication. These represent the core of interactive tools like virtual tutors, educational robots, and real-time translation software.
10 ML use cases in education
Machine learning has numerous use cases in education and positively affects multiple learning environments, spurring massive interest in the broader adoption of AI-driven technologies.
ML-based predictive data analytics helps cater to the unique needs of every student. This technology has been implemented into several eLearning software solutions to monitor various parameters (such as exercise completion time, time spent on the platform, number of logins, and test results) and assess student performance, engagement, and learning patterns, or even forecast learning outcomes.
With the help of ML, adaptive platforms such as MobyMax, SchooLinks, Carnegie Learning's LiveLab, and Kidaptive can adjust individual learning activities in real-time or help teachers and students plan personalized curricula. Similar tools can deliver special educational programs for students affected by specific conditions, such as autism.
Inclusive learning
Assistive learning devices and software systems
Input technologies
- Braille keyboard
- Eye, head, and foot control systems
- Sip and puff mouth-controlled joystick
- Mouse emulation
- Single-switch entry devices
- Speech input/dictation software
Output technologies
- Refreshable Braille display
- Screen magnification software
- Screen reader
Text input improvement software
- Abbreviation expansion
- Efficient text entry interface
- Spelling and grammar check software
- Word prediction
Assistive listening systems
- Audio induction loops
- FM microphone and receiver systems
- Infrared (IR) listening systems
AT for blind and partially sighted people
- Braille embosser
- Close circuit television (CCTV)
- Scanner with optical character recognition (OCR)
- Tactile diagrams
AT for deaf and hard-of-hearing people
- Sign language-text conversion software
- Subtitles (closed captions)
Dropout mitigation
Scheme title: ML-based identification of students at risk of attrition
Data source: mckinsey.com — Using machine learning to improve student success in higher education, 2022
Real-life phenomena exhibit complex nonlinear patterns
Example
Machine learning can be used in making the decision to reach out to a particular student at risk of attrition:
- Student has a 3.2 GPA
- Student has excellent community engagement and extracurriculars, and has demonstrated leaderchip
- Excellent attendance and on-time assignment submissions
- First-generation college student and has some delayed payments
AI tutor and chatbots
Today, we interact with several virtual assistants daily, and students are no exception. NLP-powered tutors and chatbots can perform various educational and administrative functions, such as recommending eLearning content, engaging students with quizzes and training activities, moderating online learning communities, and providing 24/7 user support to streamline student enrollment.
Image title: Juji's cognitive AI assistants
Data source: Juji
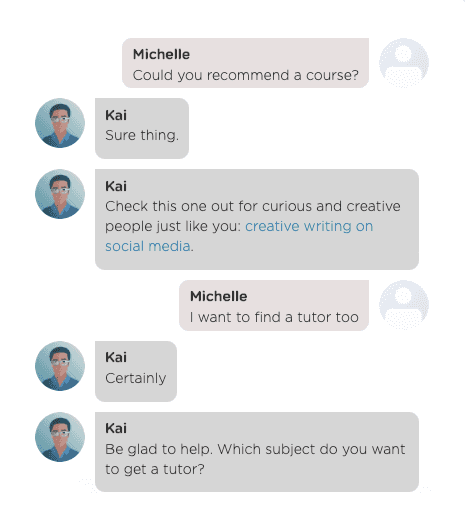
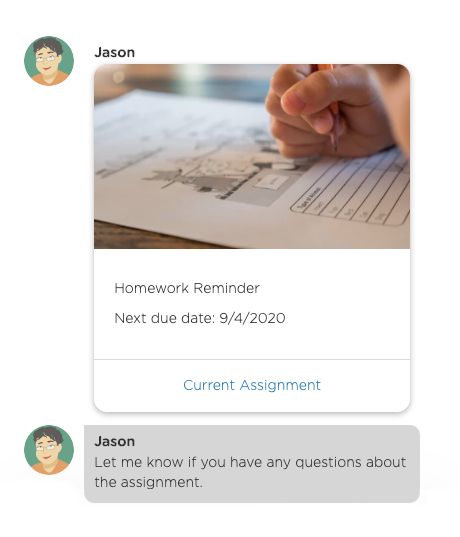
Robot teachers
Task automation
Targeted marketing
Targeted ads represent a step before recommendation systems in guiding prospective students toward a personalized learning experience that best meets their needs. With the same process adopted by ML-powered recommendation systems, data analytics software can peer into user data collected from social media and other sources to identify learners potentially interested in specific services, be it eLearning platforms or private educational institutions, and target them with personalized ads to promote subscriptions and matriculations, respectively.
Here are the results of an ML-based analytical solution adopted by a private nonprofit university and reported by McKinsey:
Scheme title: Conversion rates of an ML-based targeted marketing solution
Data source: mckinsey.com — Using machine learning to improve student success in higher education, 2022
Real-time translation
Corporate training platforms
The modern challenges professionals and corporations face, including faster innovation cycles, higher workforce mobility, and a general shortage of specialized talent, foster the adoption of ML-powered EdTech solutions in the workplace. ML-powered corporate eLearning systems can optimize training budget planning, calculate the ROI of your training initiatives, and assess your workforce expertise to generate suitable upskilling plans.
Several online platforms specifically designed for corporate eLearning, such as SAP learning hub, LinkedIn Learning, and AstraZeneca's mentoring portal, have already implemented a mix of ML-based tools.
Fraud detection
Transform traditional education with ML
Emerging technologies to pair with ML in education
Machine learning goes hand in hand with other technologies capable of disrupting a traditional educational process and making it interactive, engaging, and accessible for everyone.
Technology/Description
Technology
Value for education
Description
Value for education
VR creates an immersive digital reality simulation.
Virtual reality transforms the traditional approach to education, offering students an immersive learning environment that is more interactive and engaging. VR in education provides hands-on experience, eliminating some risks and potential dangers associated with practical studies, for example, in flight or driving simulation.
AR enhances the physical environment with additional digital content.
Augmented reality overlays digital information, including graphics, videos, and sounds, on top of the real-world environment. With the help of AR, teachers can enable students to grasp abstract concepts better.
IoT is a network of connected devices that can gather and exchange information and turn it into actionable insights.
IoT technologies enable schools to efficiently track their resources, improve the safety of their campuses and create new learning environments using smart boards and other connected devices.
RPA bots emulate human actions, automating various repetitive and time-consuming tasks.
RPA can streamline various educational processes, from attendance management and processing transcripts to answering queries and automating student feedback and survey analysis.
ML-powered eLearning apps and edTech platforms
Following the rise of eLearning, the education sector has experienced a proliferation of apps and platforms that allow individual users to study at their own pace and learning groups to interact in a highly collaborative virtual environment. Here are a few examples of online tools leveraging machine learning for various functions:
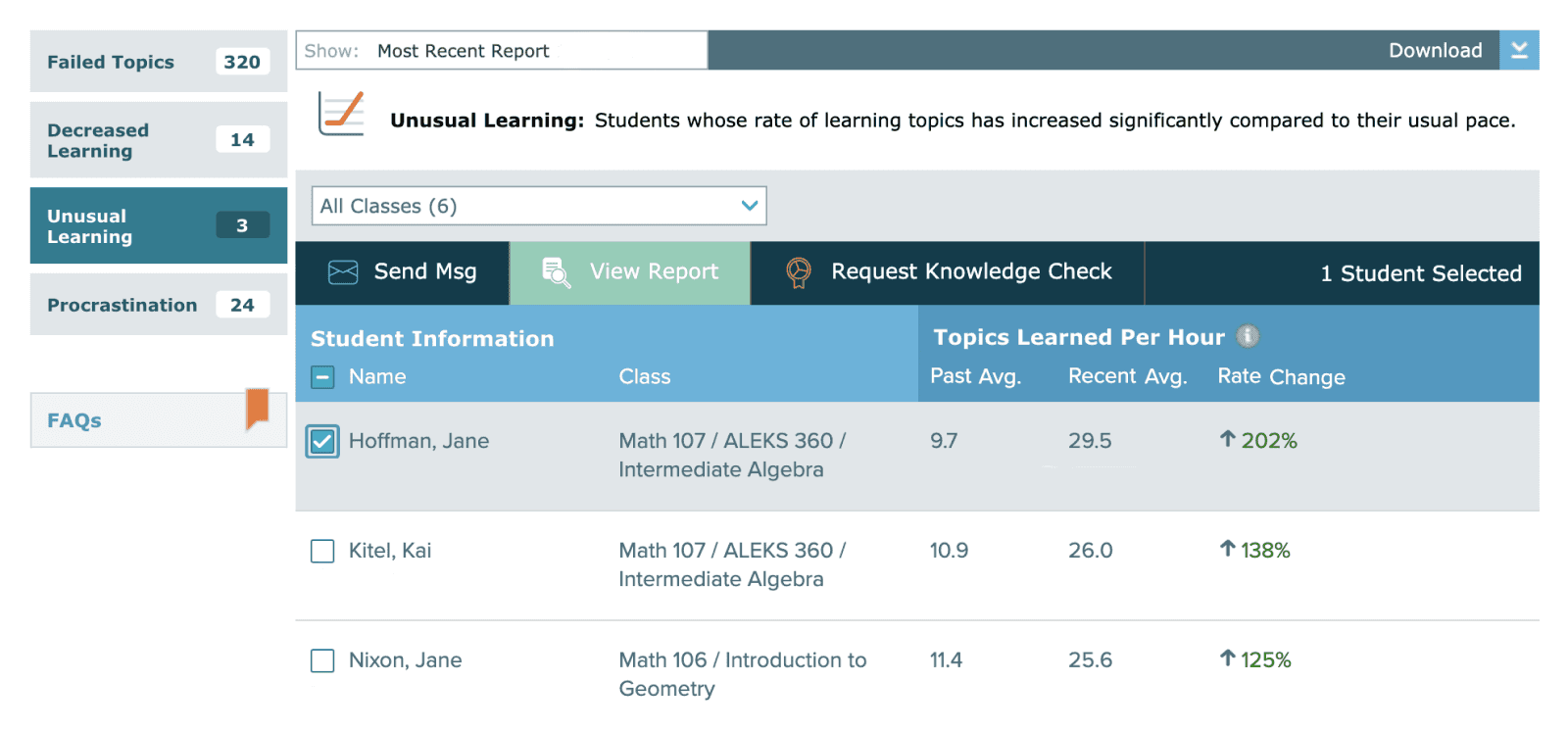
Image title: ALEKS student progress report
Data source: aleks.com
This AWS-based eLearning platform offers a fully personalized learning experience by tapping into Amazon's machine learning-focused services. It also includes features such as smart content search and ML-based fraud detection to supervise content uploading and detect spam and copyright infringements.
Prioritizing reports for all of our courses: Each language direction plotted by how much data we have and how accurate the machine learning system is for it
Image title: Duolingo’s ML-based analysis of user reports to prioritize course improvements
Data source: blog.duolingo.com — How machine learning helps Duolingo prioritize course improvements
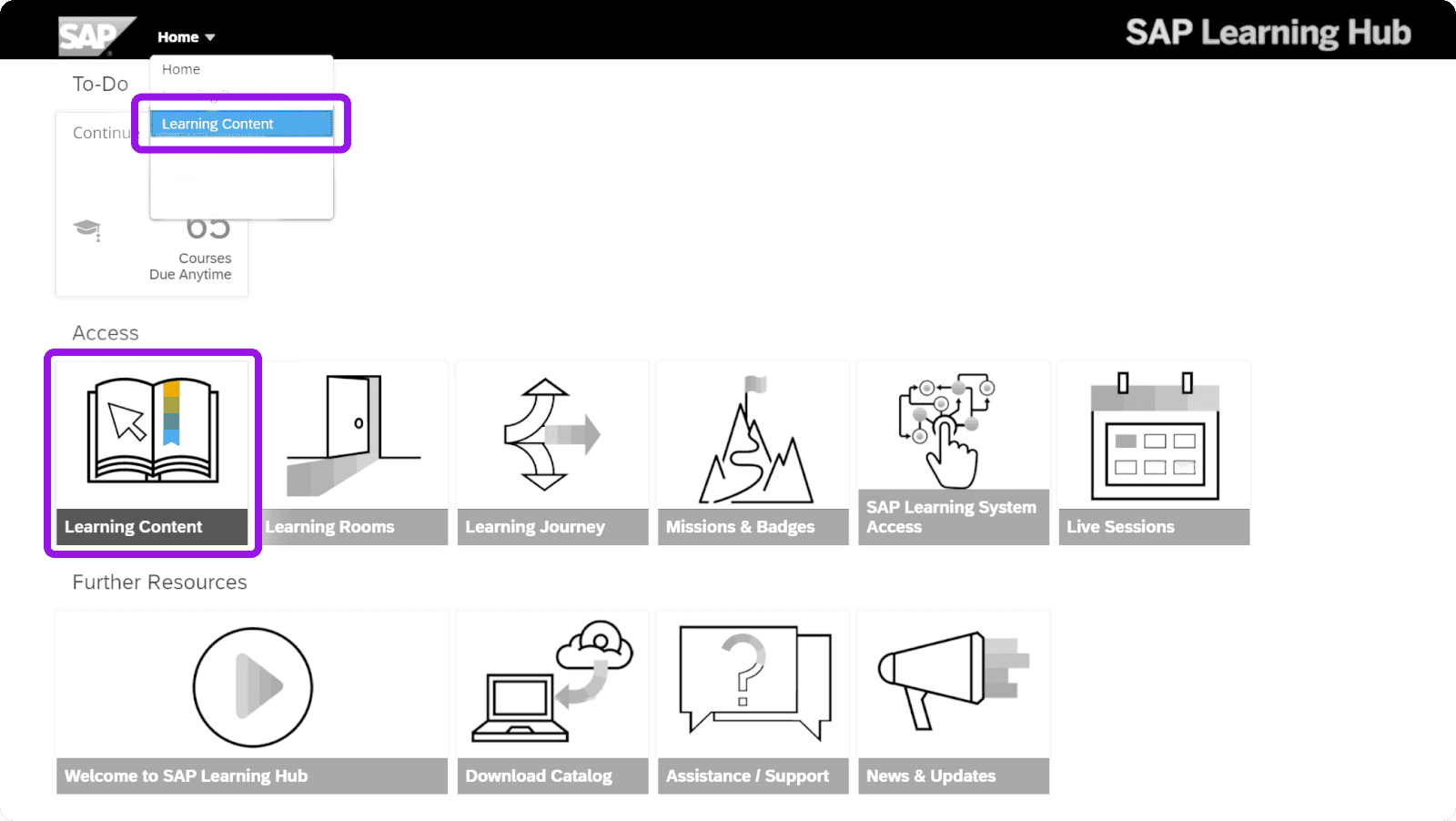
Image title: ML-powered SAP Learning courses
Data source: learninghub.sap.com
Benefits of machine learning in education
Applying machine learning helps to evolve traditional teaching methods, reduce excessive paperwork handled by teachers and administrators, and ensure greater accessibility to education for everyone.
Personalized learning experience
Improved learning outcomes
Enhanced operational efficiency
Increased profitability
Fostered inclusivity
Reduced school dropout rate
Benefits
Personalized learning experience
The challenges of ML-driven education
AI-driven technologies, including ML for the education industry, are becoming more and more accessible every day. However, several persistent implementation challenges impede their widespread adoption, especially in socio-economically disadvantaged societies. How to overcome ML adoption barriers?
Challenge
Solution
Privacy and data security
Privacy and data security
Learning experience enhancements shouldn't come at the expense of personal data protection, which has always been a pain point of any ML-powered application requiring high-volume data sets. To comply with data management regulations ML models are trained with non-personal and obfuscated data.
Obsolete infrastructure
Obsolete infrastructure
While several embodiments of machine learning in education aim to promote inclusivity, the requirements for hardware availability, data sources, and internet reliability can turn machine learning and EdTech into a source of further inequalities. Before implementing ML-based systems, education institutions should assess the available infrastructure, upgrade it if needed, and ensure they have an adequate budget.
Deployment complications
Deployment complications
Educational institutions can also face ML adoption challenges triggered by a general lack of professional expertise, IT infrastructures, and implementation strategies required to readapt teaching methods. ML implementation will require the help of professional AI/ML experts for proper integration, continuous maintenance, and ML model retraining.
Unsupervised machines
Unsupervised machines
Delegating repetitive tasks, such as grading, to ML-fuelled bots without human supervision may lead to unexpected results. For example, the International Baccalaureate implemented an automated scoring system to replace exams in a classroom with data-based evaluations during the COVID-19 lockdown, resulting in numerous anomalous grades and consequent outrage among students. ML software providers should monitor outliers and fine-tune the system to eliminate this risk and ensure algorithms work correctly.
Education 4.0: ML-driven future of learning
AI and machine learning in education drive growth and innovation, just like in any other industry. As cyber-physical systems become ubiquitous in various domains, schools and universities should incorporate emerging technologies into their curriculum to continue producing successful graduates. The upgraded learning process will help students prepare for new professional endeavors. Machine learning in education can reforge the approach to this essential component of personal development and make learning more enticing, productive, and inclusive. And our professional consultants and developers can make the ML adoption journey smooth and effective.
Education 4.0
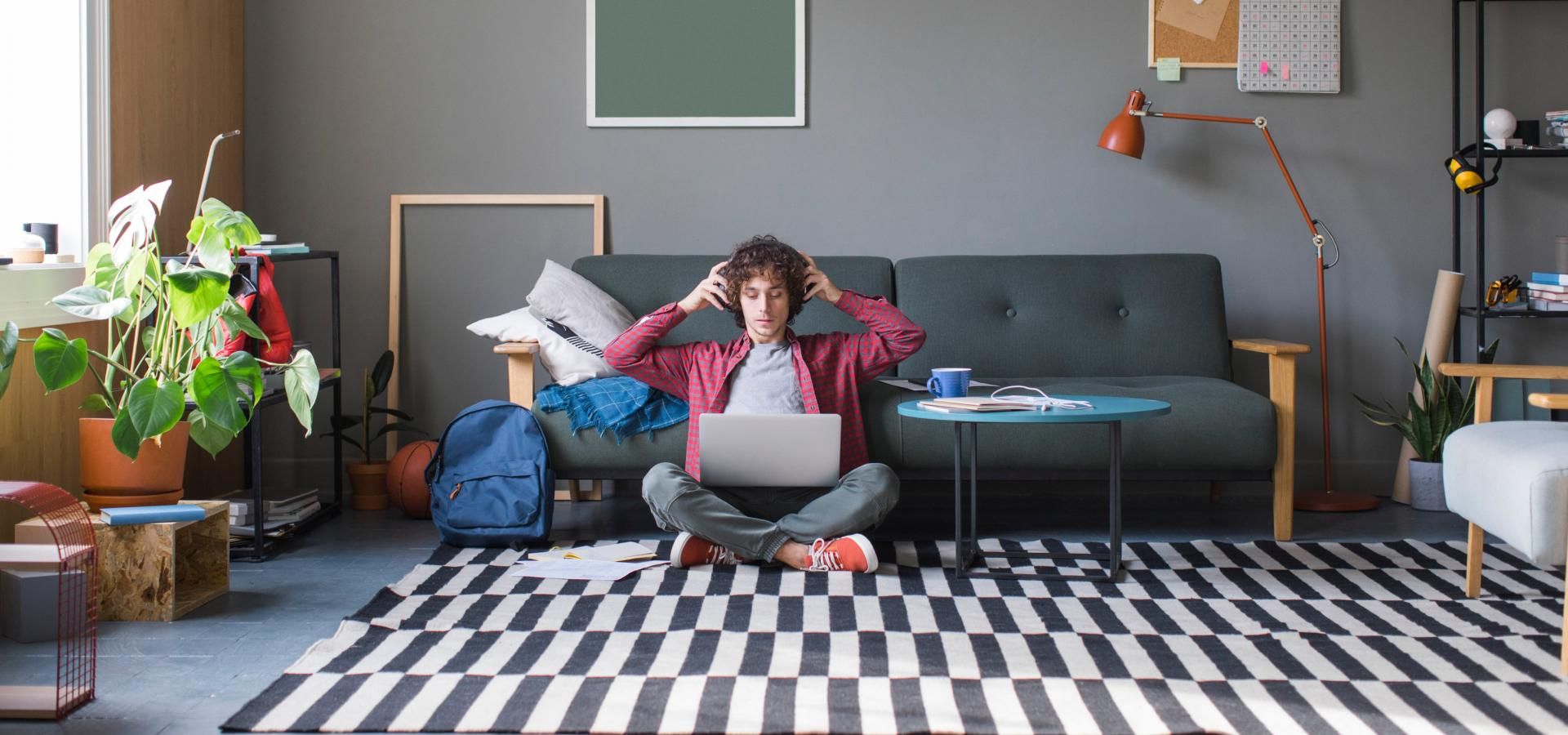
Service
eLearning software development services
Explore Itransition’s eLearning software development services, along with key application types, features, integrations, technologies, and guidelines.
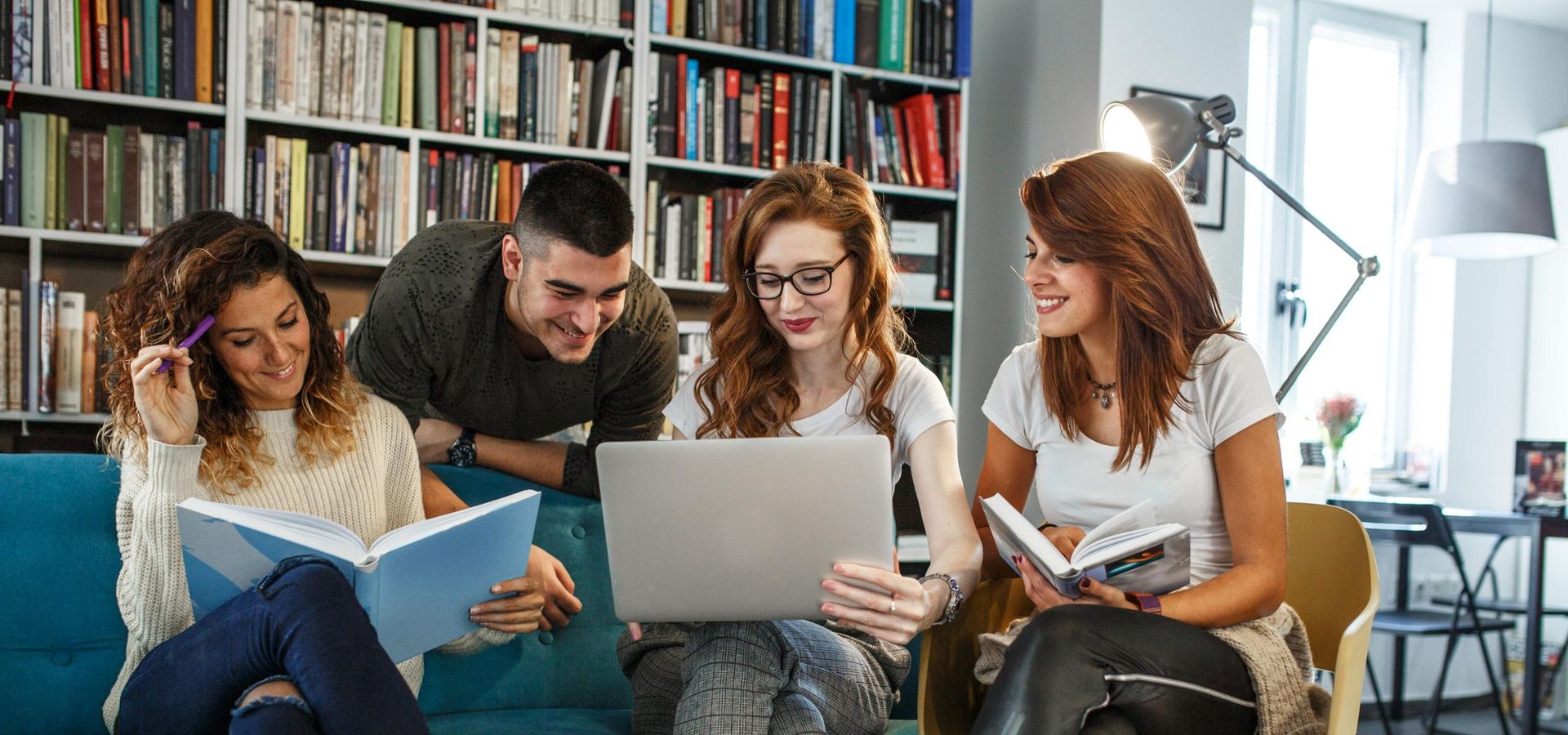
Service
eLearning portals
Here is your guide to eLearning portals' features, potential returns, and development by Itransition. Book a consultation to discuss your project.
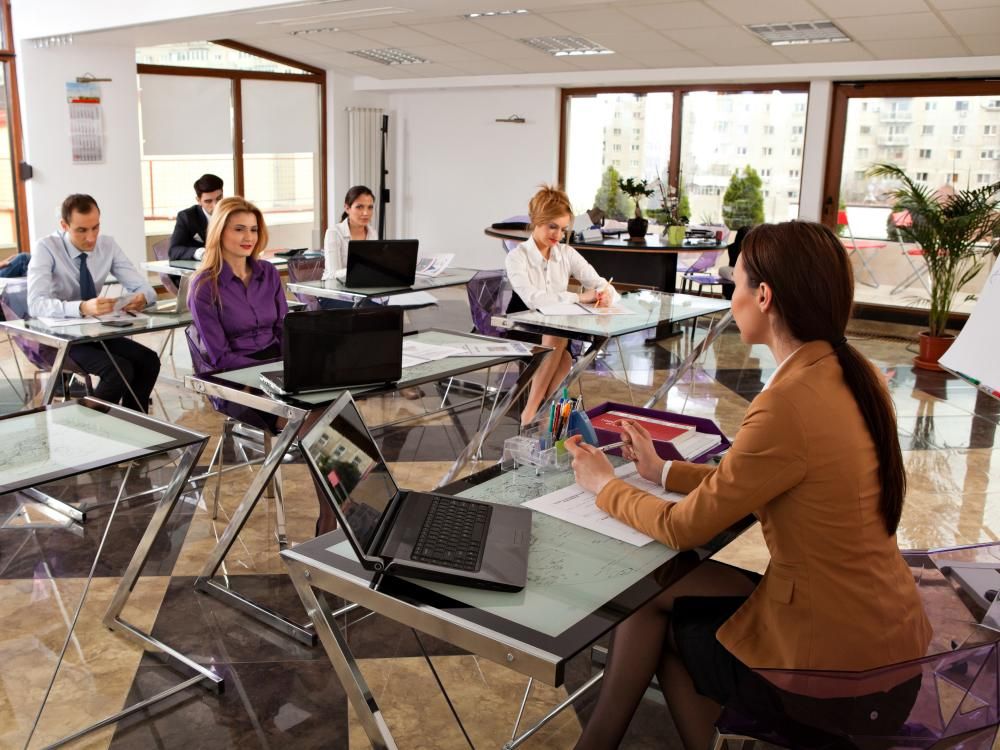
Case study
Corporate learning portal for PayPal
This is how Itransition delivered a platform-based new hire training portal for PayPal, helping to reduce the learning curve for newcomers.
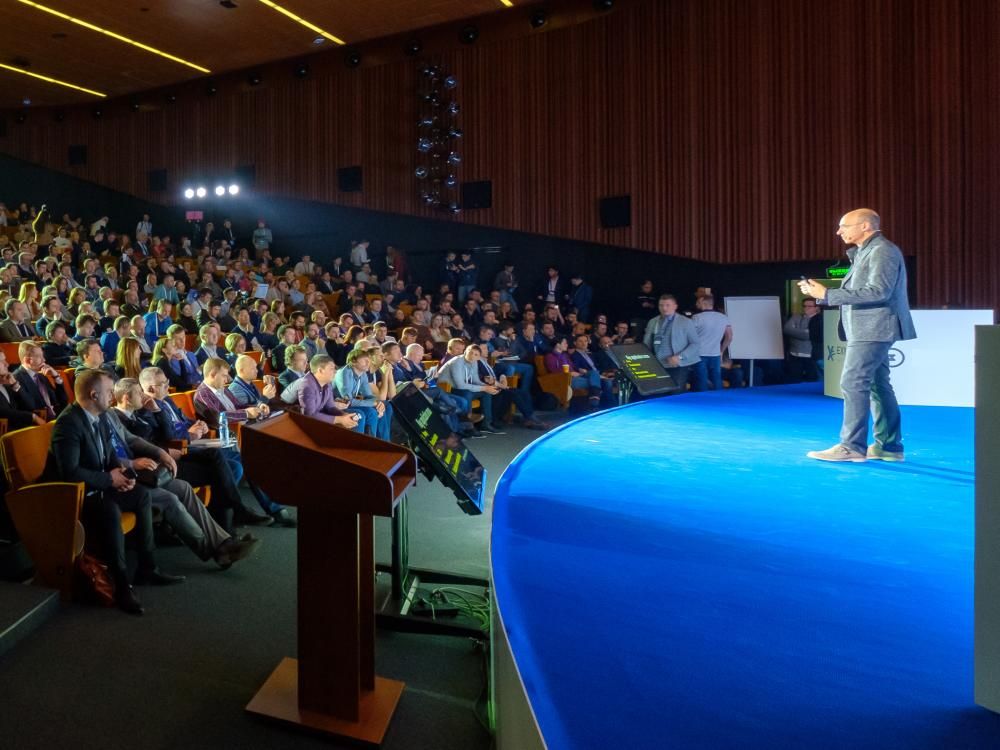
Case study
Custom conference management software for an educational society
Learn how Itransition delivered a suite of custom conference management software and enriched it with bespoke modules automating event organization.
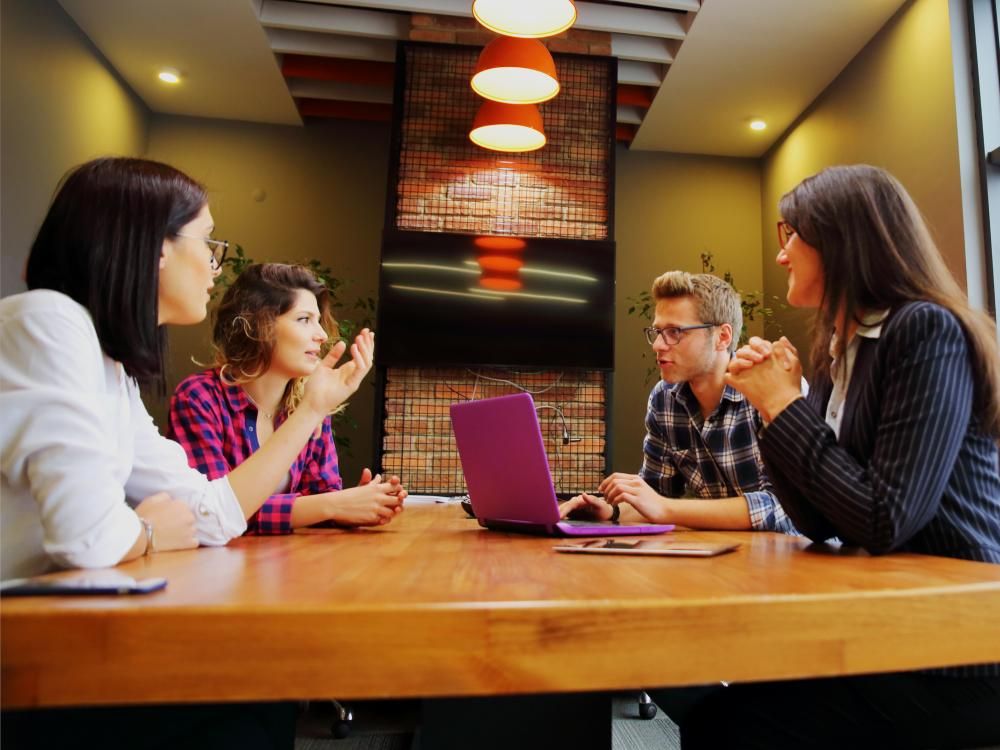
Case study
Internal talent marketplace for a global IT services company
Learn how we created Talenteer, an internal talent marketplace with AI features to optimize talent allocation in the company and reduce external hiring.