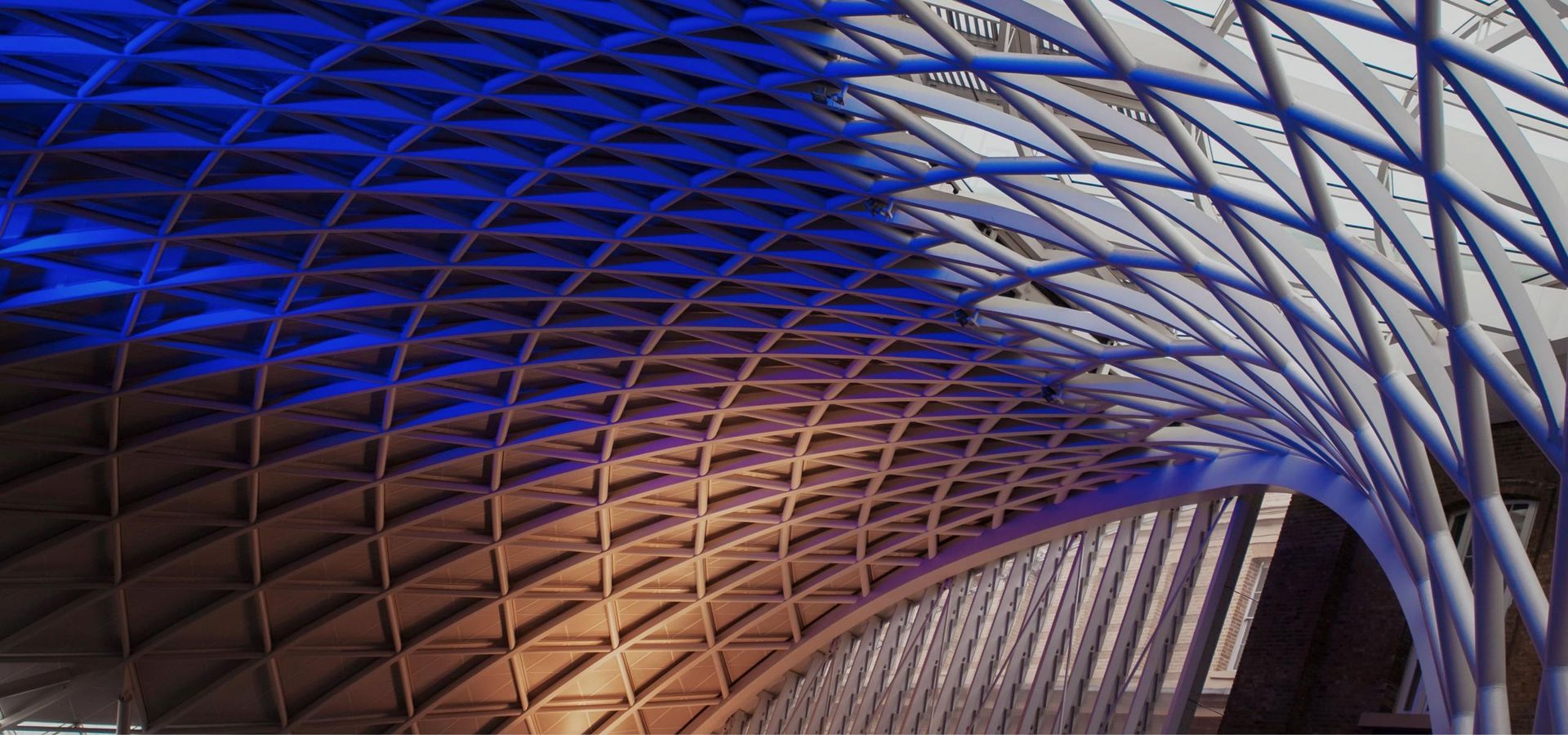
Artificial intelligence in cybersecurity: applications & the future
December 26, 2022
- Home
- Artificial intelligence
- AI in cyber security
In recent years, artificial intelligence (AI) has become essential in cyber security. As the threat environment has evolved, so has the need for AI services and cyber security solutions to help organizations defend against new and increasingly sophisticated attacks. Cybersecurity is a complex and ever-changing field, and AI can provide the speed and agility needed to handle emerging threats.
AI in cybersecurity: a summary
While the use of AI in cybersecurity is a decade-old conversation, the recent advancements in computing power and cloud technologies have finally made AI feasible for threat intelligence. In this article, we discuss the role of AI in cybersecurity, outline its most important applications, provide real-life examples and consider the future outlook of this technology.
The future of AI in cybersecurity
CAGR of AI in the cybersecurity market from 2022 to 2033
Acumen Research and Consulting
the global average cost of a single data breach
IBM
of executives consider AI the best technology to counter nation-state cyberattacks
Pillsbury
Why apply AI in cybersecurity?
Today, industry players rely on the latest technological advancements to stay on top of the competition. At the same time, the mass proliferation of these technologies and devices has created new opportunities for cybercriminals. Hackers are constantly coming up with new ideas to infiltrate enterprises via desktop computers, webcams, routers, printers, and smartphones and expose sensitive consumer and business data. As a result, the established security protocols and practices, some dating back decades, are insufficient to properly address new unconventional exfiltration, phishing, identity theft, network incursion and password-cracking attacks. This is why forward-looking organizations are investing in AI to bolster their security systems.
The history of cybersecurity, and really any type of security, is an age-old game of cat and mouse. Just as we develop AI tools to protect ourselves, antagonists are developing AI to further complicate their attacks.
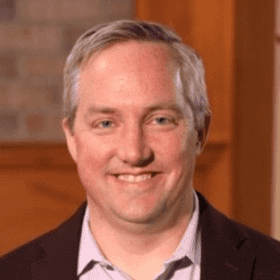
Mike Chapple
Information security leader and IT, analytics, and operations teaching professor, University of Notre Dame
AI vs data analytics
To better understand why exactly AI is the most effective technology for combating cyberthreats, let’s compare this technology to its closest relative – data analytics.
Artificial intelligence
By its nature, AI is designed to learn and evolve. This means that it can keep pace with the ever-changing landscape of cybersecurity threats. Additionally, AI can process large amounts of data much faster than humans to quickly identify patterns and anomalies indicating a potential threat.
Data analytics
Supervised by humans, data analytics examines large data sets and identifies patterns, trends or behavior that can point to malicious activity. On the downside, data analytics can be time-consuming and expensive and is limited to human ability to process and interpret data. Most importantly, data analytics systems are highly dependent on humans to evolve, meaning that, unlike AI, such systems are not self-learning.
6 use cases of AI in cyber security
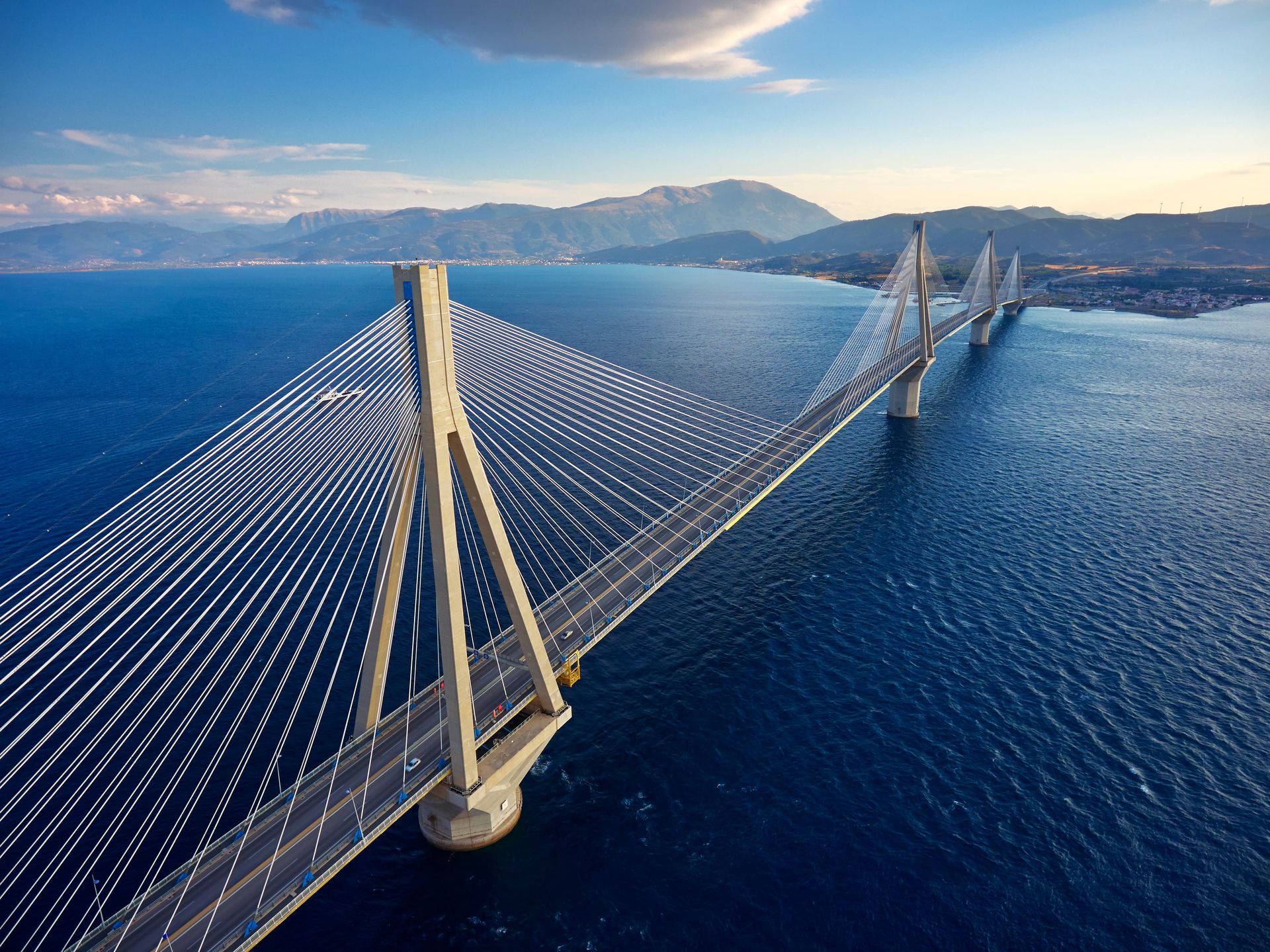
1 Threat detection
Artificial intelligence can identify and prioritize new, previously unknown cyberthreats by analyzing data sets for patterns and anomalies. This allows organizations to respond quickly and efficiently to new threats. In addition, AI can correlate different data sets to provide a complete picture of an attack, so that security teams can understand its scope and nature and better mitigate it in the future. By comparison, traditional methods of security analysis often involve security specialists manually sifting through large data sets, which can be time-consuming and lead to errors.
2 Automated malware detection
Malware is software designed to cause disruption in organizations’ networks by exploiting connected devices. Traditional malware detection methods involve monitoring the network for signature matches, which requires a considerable amount of manual work from security experts. In comparison, AI systems can automatically pinpoint even zero-day malware by analyzing huge amounts of historical data. Moreover, AI can identify patterns and trends in past attacks and alert companies, helping to improve the existing security strategy and proactively protect corporate systems from such future exploits.
3 Social engineering detection
Verizon's 2021 Data Breach Investigation report observes that 85% of cyber security attacks are now social engineering attacks that prey upon humans’ trusting nature. Previously, the problem with using AI for social engineering attacks was that they couldn’t be fully “explained” to ML systems. However, deep learning models, the most sophisticated machine learning models that mimic human thinking, can analyze even unlabeled data and learn independently. This makes deep learning-based systems more effective in combating social engineering attacks than traditional Secure Email Gateway and similar systems. Additionally, AI can also be used to create “phishing simulations” that can test user susceptibility to social engineering attacks. This is a great way of raising awareness and ensuring that employees are better equipped to deal with real-life attacks.
4 Helping to work smarter
Even for seasoned cybersecurity professionals, one of the biggest pain points is incident response prioritization. According to research by Trend Micro Incorporated, 70% of employees at security operation centers are emotionally overwhelmed by security alert volumes. And while AI can’t replace cybersecurity professionals, it can streamline their work and make it easier. Today’s AI models can keep track of the constantly evolving cyber threat landscape and help human analysts decide what security alert to respond to first. As a result, AI can help enterprises allocate their efforts to the most critical threats first, reducing the overall risk to the organization.
5 Managing vulnerability
As the world switched to remote working, old cybersecurity challenges have become more prevalent while new ones arose as well. However, traditional vulnerability assessment tools have grown outdated in increasingly more hybrid environments often containing mobile and IoT devices. The most important distinction between AI-based and conventional vulnerability assessment tools is that the former can accurately define the risk score of all devices, regardless of how critical they are to running a given business. There are situations when even the most isolated devices without an internet connection can pose a significant threat to the organization’s well-being, and AI can provide additional context for assessing their vulnerability.
6 Spotting cyberattack trends
Prolific marketing specialists are already relying on various AI-driven tools to analyze social media trends and learn what topics customers engage with the most. Similarly, AI can be used to analyze forums, cybersecurity social media pages, and news websites to better understand which cyberattacks are becoming more popular in a given sector, and what attacks cybersecurity experts currently deem the most concerning.
Performing such large-scale analysis on a daily basis is almost impossible for human analysts, but by applying modern natural language processing techniques, companies can grasp valuable insights from an endless information stream on the internet.
Real-life examples of AI cyber security adoption
Looking for professional AI consultants?
The benefits of AI in cybersecurity
Faster threat detection
Automated AI-driven systems can speed up incident response times.
Reduced false positives
Artificial intelligence can reduce the number of false positives that often overwhelm security teams.
Enhanced accuracy
Machine learning can provide more accurate results than traditional security analysis methods.
Improved correlation of data
AI-enabled tools can correlate different data sets to provide a more comprehensive picture of an attack.
Self-sufficiency
AI-based systems can generate their own security rules and signatures, meaning they are less reliant on human input and more effective at stopping new threats.
Increased automation
Automated security systems can free up time for analysts to focus on other tasks.
Better detection of zero-day threats
By its nature, AI is good at spotting novel patterns, which can help detect previously unknown attacks.
Greater flexibility
AI systems quickly adapt to changing circumstances and new data sets, which makes them more resilient in the face of ever-changing threats.
AI in cybersecurity implementation advice
While the benefits of AI in cybersecurity are apparent, organizations often struggle to implement this technology, so we provide some important tips on making it a success.
1
Prepare datasets
One of the most persistent problems with AI implementation is that a company’s existing datasets are of insufficient quality for AI integration. Since the reliability of an AI system’s output depends on the quality and availability of data that it consumes, you must ensure that your data is clean, complete, and up-to-date.
2
Select the right use cases
3
Integrate SOAR
4
Employ a governance framework
It is tempting to add machine learning tools into many business processes, and it is indeed becoming ubiquitous, but AI tools suffer from bias, vulnerability to attack, and a lack of explainability. Without proper governance and oversight, we are simply exposing industry, people, and the environment to significantly more risk.
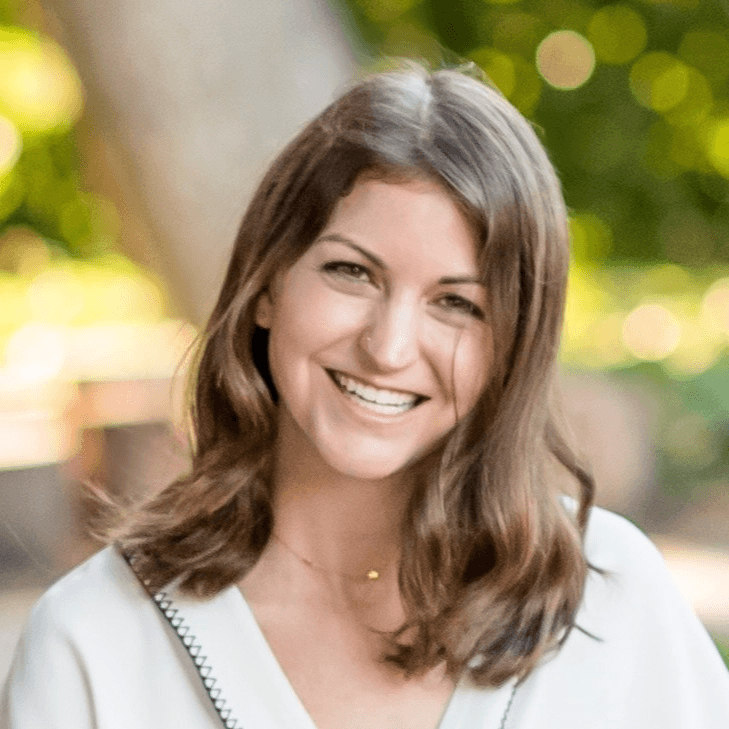
Jessica Newman
Program Lead at UC Berkeley’s AI Security Initiative
How cybercriminals use AI
AI is a controversial topic in the context of cybersecurity because it can be used both by companies to defend against cybercriminals and by cybercriminals to attack companies.
Data poisoning
Data poisoning
Data poisoning refers to a cyberattack where cybercriminals alter AI model integrity by injecting minimally-perturbed samples into the training datasets. The wrongdoers can subtly manipulate datasets for AI algorithms training using their own AI models and gradually change AI systems’ outputs the way they want without raising suspicion.
Password guessing
Password guessing
Those popup windows that ask you to use more complex passwords are more than just an annoyance. Modern deep-learning-based password guessing systems can easily link many data traces that you have left on the limitless expanse of the internet to your personality and guess a simple password in a matter of seconds. People that use their favorite color or model of car, dog’s name, date of birth, or beloved song name for passwords stand no chance against today’s AI-based systems. This is why it’s highly advisable to use long passwords that make absolutely no sense to thwart such AI-powered attacks.
Deepfake manipulation
Deepfake manipulation
Most flashy uses of AI among hackers revolve around deepfake manipulation. It's now possible to impose a new deepfake identity onto a participant in real time, thanks to DeepFaceLive, a streaming implementation of DeepFaceLab, the most popular open-source project for superimposing photorealistic identities onto individuals in video footage. The most famous deepfake crime to date was defrauding $243,000 from a company CEO, with an audio deepfake impersonation of a chief executive used to obtain a wire transfer. Now that it's possible to add video to audio deepfakes in real time, the attacks can be highly realistic.
Improve your cybersecurity with AI
Facing the sophistication and scale of modern cyberattacks, it has become clear that conventional approaches to cybersecurity no longer suffice. Cybercriminals are using AI to automate their attacks and evade detection, so businesses need to fight fire with fire by integrating AI into their security solutions. This will help them improve their threat detection, identify emerging trends and stay one step ahead of the attackers. As the reliance on technology grows, cyber threat protection will become ever more important, and AI will play a vital role. If you are looking for a robust AI-based cybersecurity solution to withstand even the most sophisticated cyber threats, feel free to contact Itransition’s experts to discuss your project.
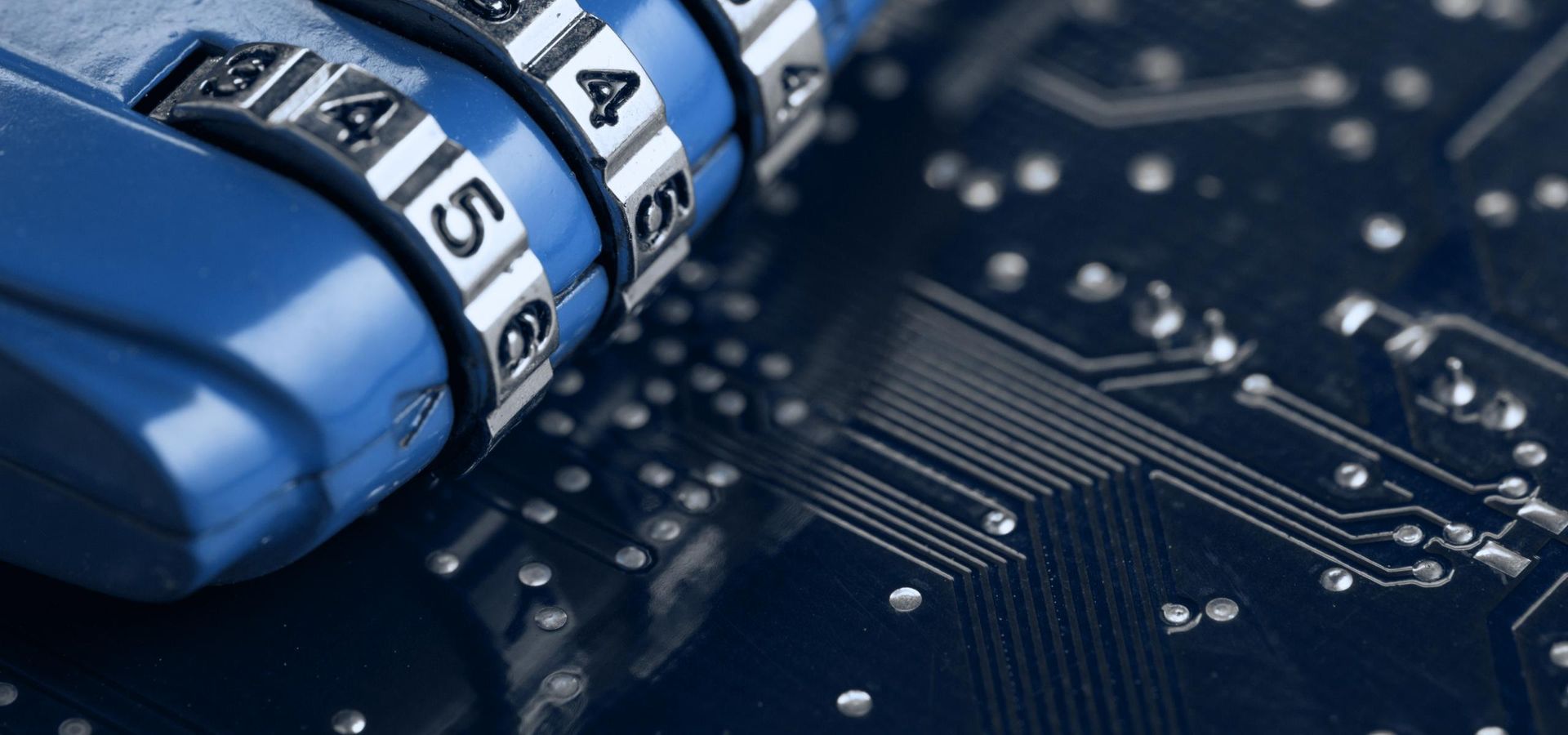
Service
Cyber security consulting
We provide businesses with a broad range of cyber security services, covering all types of organizational IT assets. Get our assistance
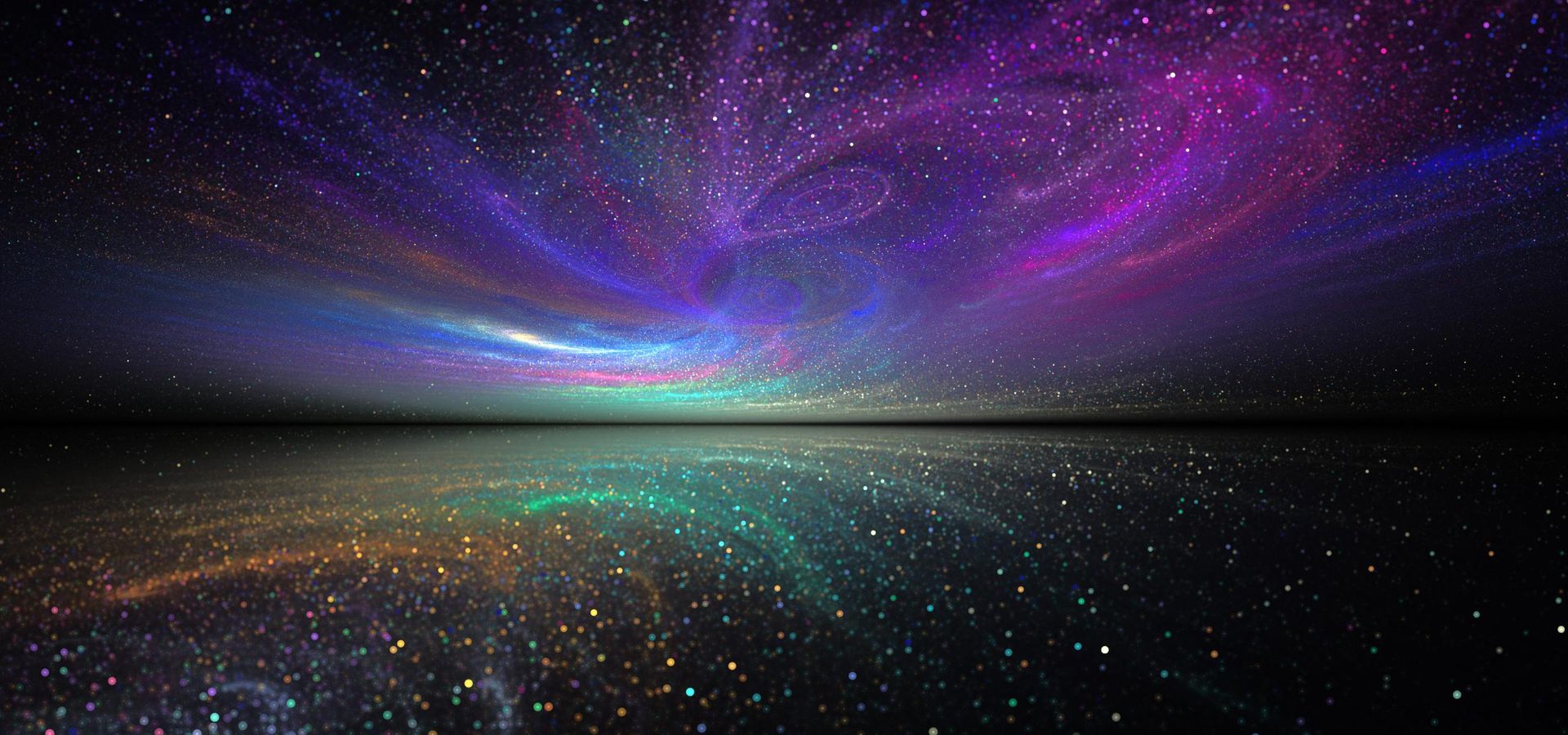
Service
AI services & solutions
Explore our range of AI services, along with key AI use cases, related technologies, and adoption guidelines.
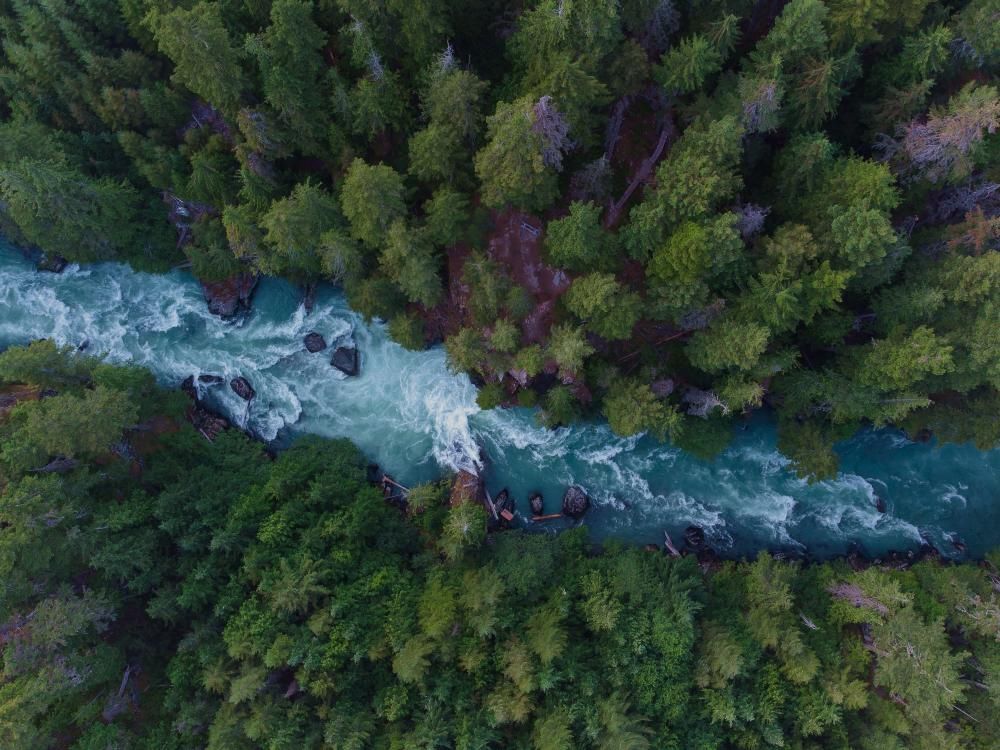
Insights
Machine learning in manufacturing: key applications, examples & adoption guidelines
Learn how machine learning can help manufacturers to improve operational efficiency, discover real-life examples, and learn when and how to implement it.
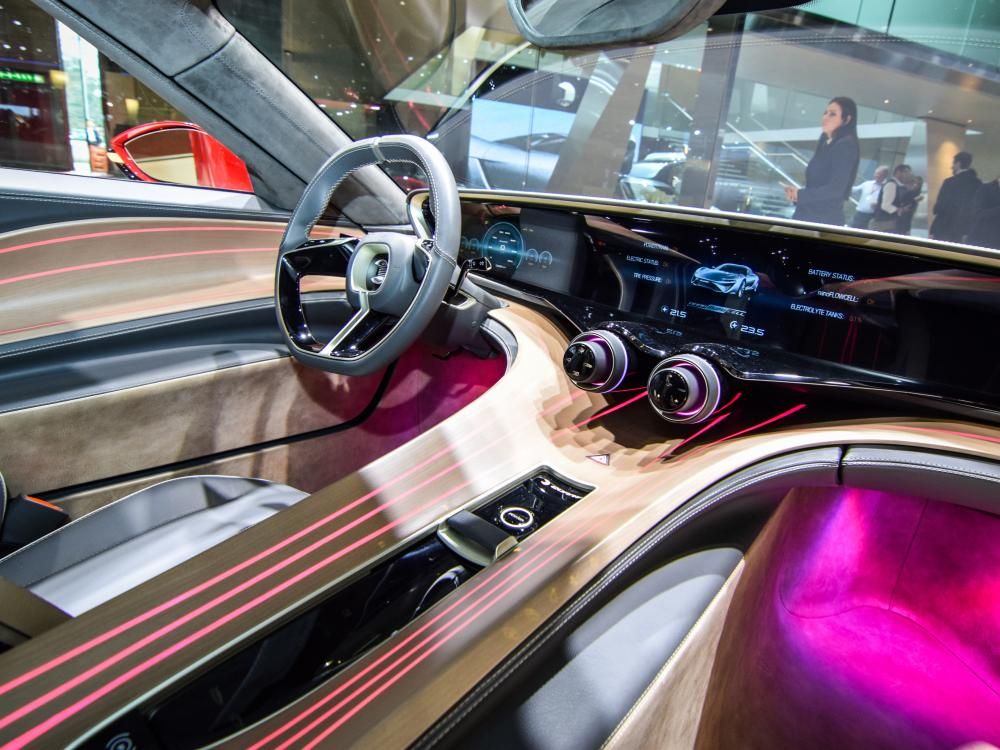
Insights
AI in the automotive industry: use cases, success stories & adoption guidelines
Explore key use cases, payoffs, and real-life examples of AI in the automotive industry, along with common adoption challenges and tips to address them.