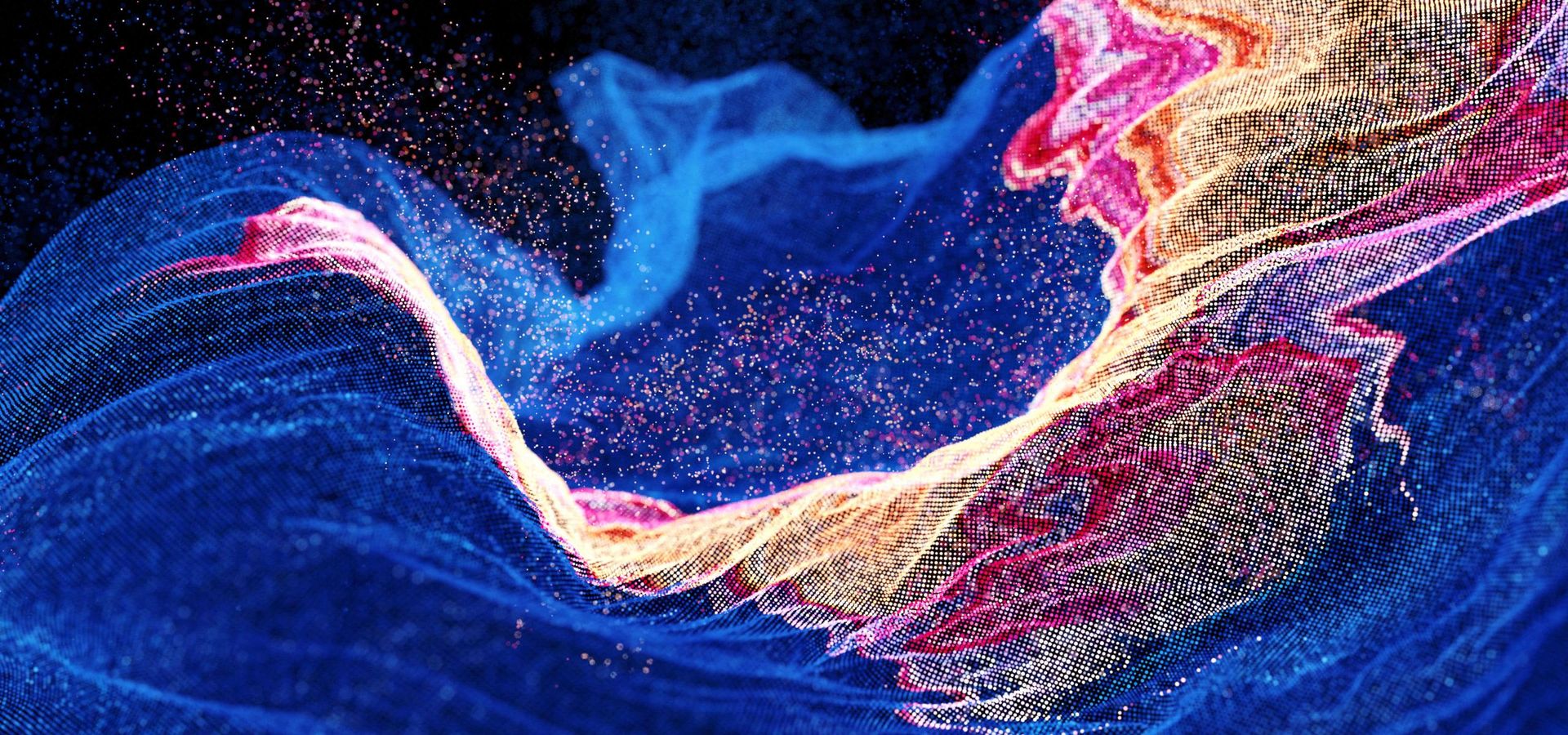
AI in CRM: top use cases, best platforms, and guidelines
June 29, 2023
- Home
- Artificial intelligence
- AI in CRM
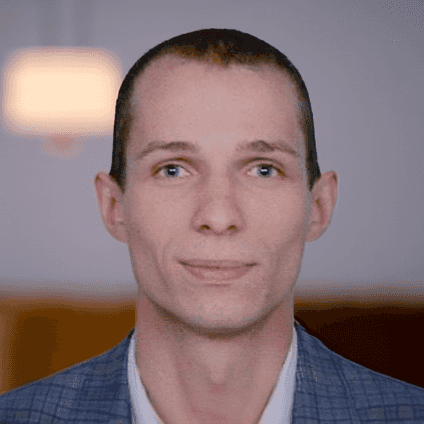
Head of AI/ML Center of Excellence
Artificial intelligence in customer relationship management (CRM) software helps businesses automate routine processes like inputting and cleaning customer data, reduce human errors, and improve decision-making via data analytics and forecasting capabilities.
Itransition offers comprehensive CRM consulting and development services to help you select, design, and adopt an AI-powered solution matching your corporate goals and requirements.
Table of contents
expected CRM market value by 2029, with AI as a major demand driver
Fortune Business Insights
of companies consider improving customer experience the top AI use case
Statista
faster response to customers and partners by companies adopting AI
Oracle
12 use cases of AI in CRM by business area
AI-based technologies сan augment your CRM's functionality in various use cases and workflows.
Sales
- Assess leads and forecast customer lifetime value based on sales, activity, and demographic data to increase potential customers’ conversion rate.
- Automate routine tasks like scheduling appointments and sending follow-up emails to customers to free your sales managers’ time for tasks requiring a more personalized approach to particular customers.
- Analyze salesperson performance and provide account-specific recommendations via smart assistants to assess your sales team and adjust their KPIs.
Image title: SAP lead scoring feature interface
Data source: SAP
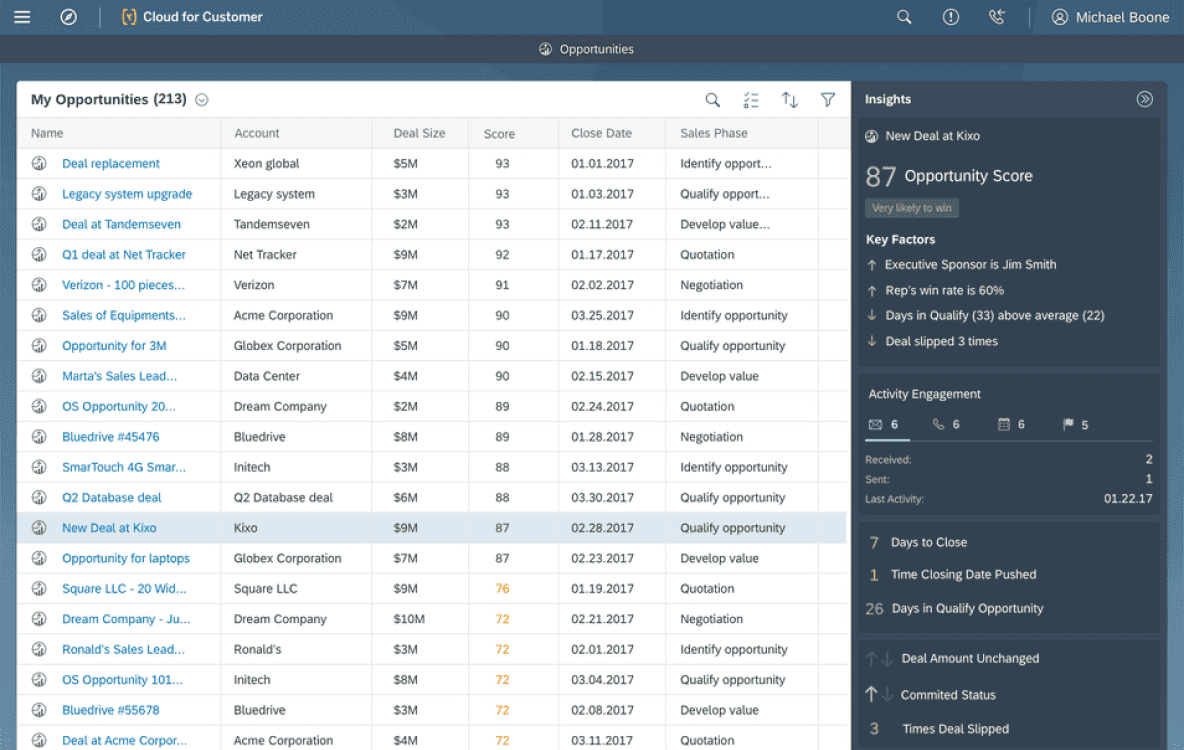
Marketing
- Analyze user sentiment based on online interactions across multiple channels to improve customer experience and mitigate customer churn.
- Segment customers into different user cohorts according to demographic, geographic, and psychographic data to predict consumer behavior, optimize budgeting and create effective ad campaigns.
- Personalize user experience through tailored ads, content, engagement strategies, targeted offers, and customer journeys to increase their engagement.
Image title: Salesforce customer segmentation feature interface
Data source: Salesforce
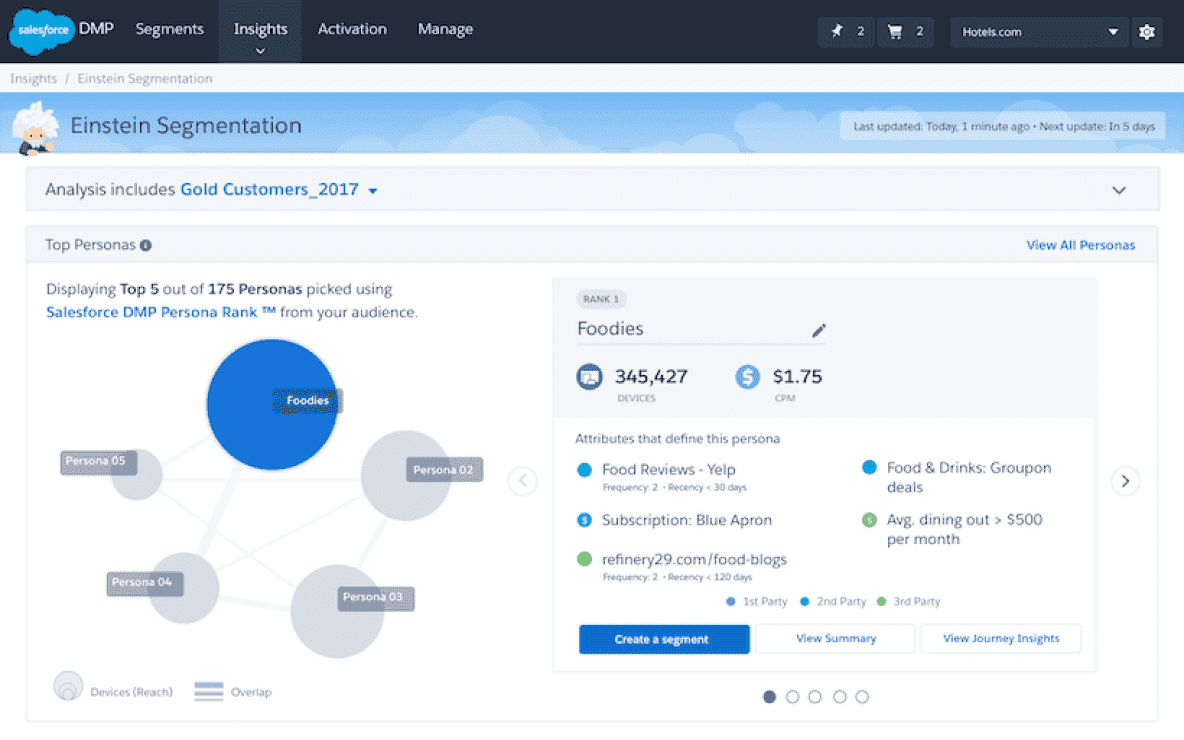
Customer service
- Offer personalized support and recommendations both in-store and on ecommerce platforms to boost customer engagement and loyalty.
- Deliver multi-channel, 24/7 remote assistance via chatbots and automate email responses to create uninterrupted customer experience and reduce bounce rate.
- Proactively contact customers in case of delayed shipping or other issues via push notifications to deliver timely support and prevent clients’ dissatisfaction.
Scheme title: Digital Assistant architecture
Data source: Oracle
IT
- Protect customer data and corporate systems via fraud detection and AI-based cybersecurity to ensure your business data safety and prevent cyber breaches.
- Leverage development frameworks and low-code or no-code platforms with AI-based code generators to reduce the development time.
- Automate customer data entry and updates via AI-enabled RPA bots while facilitating data mapping and integration to eliminate human error, streamline the data cleansing process and identify anomalies, duplicates, or inaccurate information.
Image title: Dynamics 365 fraud protection feature interface
Data source: Microsoft
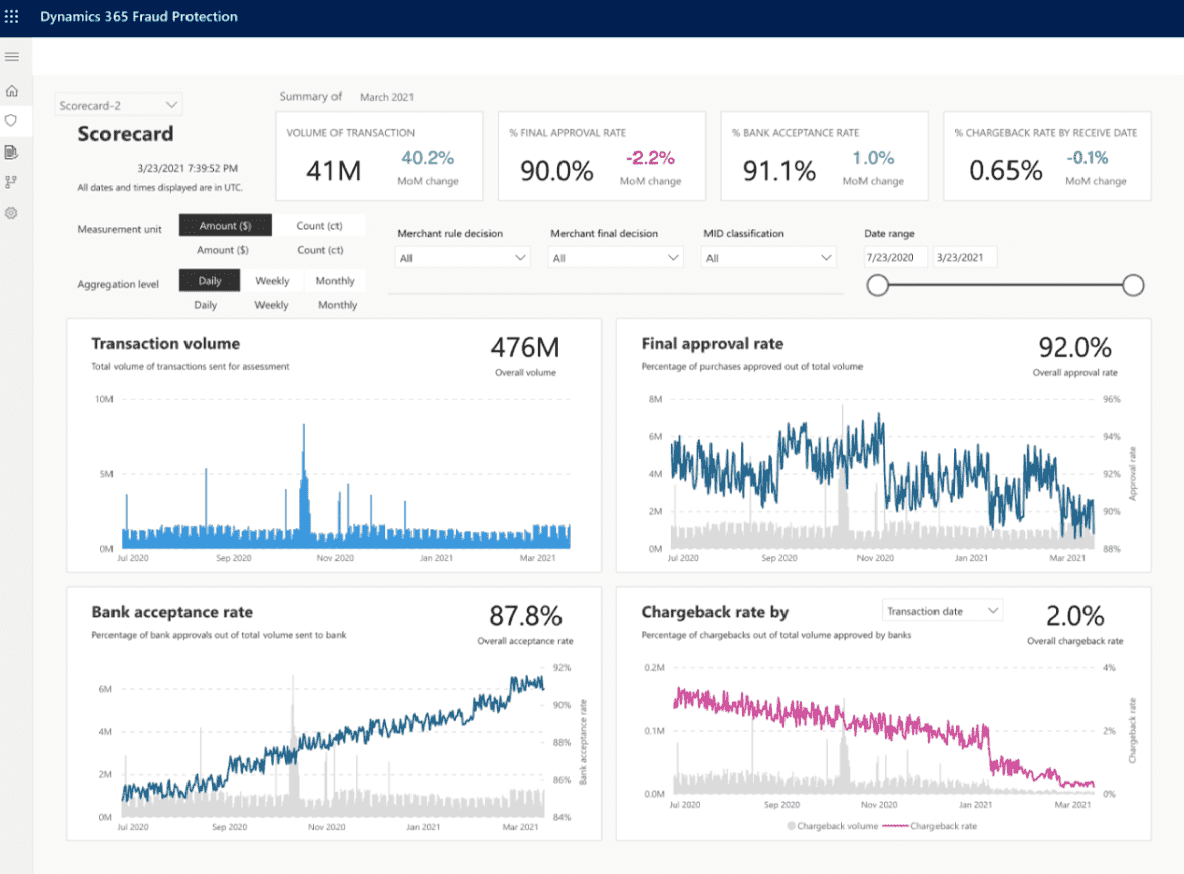
Enhance your CRM solution with Itransition’s guidance
AI-enabled CRM implementation examples
Look at real-life CRM implementation scenarios to assess the potential impact of artificial intelligence on your customer-centric operations.
A major commercial property management company in the UK partnered with Itransition to implement a Salesforce-based CRM solution. Its AI features include automated reporting and predictive analytics to forecast customer behavior.
+ 15%
conversion rate
The system built by Itransition helped us improve decision-making and get a 360-degree view of our customers.
Sam Wilson
Solutions Architecture Director, Bruntwood
This London-based analytics and brand consulting organization automated its CRM operations with a bot powered by SAP Conversational AI, achieving faster query resolution, reduced call center costs, and higher user satisfaction.
- 10%
inbound calls
A major superyacht brokerage company deployed a CRM solution built on top of Dynamics 365, featuring AI-powered capabilities such as behavioral data analysis, customer segmentation, lead scoring, and brand affinity assessment to identify and target clients with potentially higher conversion rates and personalize their offering to enhance engagement and drive sales.
- 20%
days on market
The leading telecommunications provider in Switzerland relied on Adobe Experience’s AI functionality to improve marketing campaigns’ response rate and effectiveness while providing its online users with a more personalized customer experience, including multilingual support and tailored campaign messaging.
40%
campaign uplift
Top 5 CRM platforms with AI-based features
Most major CRM platforms currently include an extensive range of AI-powered features to enhance customer-related operations. Check out the following options on the market:
Customer 360 relies on Einstein, a powerful AI that integrates easily with Salesforce’s services and provides valuable business insights
AI-based features
- Prediction Builder to forecast customer churn, lifetime value, and other business outcomes
- Customizable chatbots automating customer support tasks across multiple digital channels
- Identification of potential leads and opportunities based on past deals and sentiment analysis
- New Einstein GPT functionality to deliver AI-generated content, such as personalized emails
Pricing
- Four bundles (sales + cloud services)
- from $25 to $325 per user per month, billed annually
Customer Experience is part of SAP Business Suite, an ensemble of tools featuring advanced AI functionalities powered by AI algorithms.
AI-based features
- Dynamic scheduling of sales staff visits to customer locations based on real-time data
- Deal Intelligence feature to rank and prioritize high-propensity opportunities
- Correlation analysis between seller behavior, sales outcomes, and other parameters
Pricing
- Three plans with different add-ons
- Monthly or annual subscription
- Price upon request
Microsoft’s portfolio of CRM and ERP applications leverages the cognitive capabilities of Dynamics 365 AI, a set of functionalities focusing on analytics.
AI-based features
- Fraud Protection feature to safeguard customer accounts from fraudulent access and bot attacks
- Sales Insight for predictive lead scoring, guided selling, and improved sales cycle visibility
- Customer experience personalization via tailored marketing campaigns and offers
Pricing
- Multiple plans by business area
- A full licensing guide available on the website
This cloud-based, modular suite of services includes ERP, CRM, and ecommerce functionalities, which can be enhanced with Oracle’s native artificial intelligence.
AI-based features
- Built-in advanced BI and analytics tool to assess currently active leads and increase potential conversion
- Sales Automation feature enables sales representatives with real-time dashboards for streamlined selling, upselling, order management, and sales forecasting
- NetSuite enables integrations with ecommerce storefronts, online marketplaces, point of sale (POS) systems, and third-party logistics providers
Pricing
- Initial setup fee + annual license fee
- Price upon request
Sensei is the AI layer operating across the Adobe Experience platform, which provides comprehensive data analytics and automation capabilities.
AI-based features
- Smart budgeting and campaign monitoring to adjust ad delivery based on targeting criteria
- Content intelligence and automation enhancing document creation, editing, and search
- Customer insights and forecasts to personalize user journeys depending on attributes or conversion factors
Pricing
- Several packages offering targeted services
- Price upon request
How to implement AI in CRM
While the roadmap to build and adopt an AI-powered CRM solution can vary depending on the model selected, these are some common stages you should be ready to go through.
1
Discovery
Start by framing your current process and CRM flaws, business goals, and user requirements to choose the main AI use cases your future solution should target. Then, set up an implementation plan defining an MVP, budgeting, and change management. You should also select suitable internal and external data sources to integrate your CRM with.
2
Design
Prepare a functional specification encompassing various scenarios for different user roles and describing the CRM architecture, along with a visual mock-up and wireframes for the user interface. You should also detail the tools, technologies, and AI-based platforms you’ll rely on, along with the deployment model and the software integrations you plan to configure.
3
Development
Proceed with the coding phase, covering front-end, back-end, and integrations. As for the AI and ML features developed from scratch for a custom CRM, their operation will rely on ML models built through complex data preparation and algorithm training. Alternatively, you can opt for CRM based on platforms like NetSuite or Salesforce and customize built-in AI functionalities.
4
Rollout and support
After performing accurate functional, performance, and usability tests, you can deploy your AI-powered CRM solution on local servers or in a cloud-based environment. The adoption should be facilitated through ongoing user training and support, followed by regular audits and software maintenance to solve issues or address user feedback.
Benefits of AI in CRM systems
Supported by AI technologies like machine learning, a CRM platform will strengthen your customer relationship management strategy and improve business outcomes.
Improved decision-making
based on data-driven insights and trend forecasts
Better sales performance
via segmentation, targeted marketing, and lead scoring
Enhanced customer engagement
due to personalization and 24/7 user support
Faster and more accurate operations
through robotic process automation
Improved data reliability
via automated mapping, cleansing, and integration
Easier development
with AI-based platforms enabling low-code CRM customization
Safer data management
through ML-based anomaly detection and fraud prevention
Benefits of AI in CRM systems
AI-based CRM implementation challenges and solutions
When implementing AI functionalities in your CRM software, you can face a number of business and technical challenges. Here are some recommendations from our experts.
Challenge
Solution
Data quality and availability
Data quality and availability
The performance of the algorithms powering AI-based CRM systems largely depends on the data they process. Such information can be outdated, inaccurate, or spread across multiple data silos.
Collecting high-volume, reliable data requires a solid data management strategy. This includes data mapping of suitable sources, data cleansing and transformation, and integration among multiple systems via APIs or ESBs. The top CRM providers generally offer data preparation features (such as Salesforce Data Prep) and cloud data integration tools with built-in APIs (like SAP Data Services).
Feature and use case selection
Feature and use case selection
Whether you design a custom CRM or select an AI-powered CRM platform, you should define a suitable feature set addressing essential use cases in your business scenario.
When implementing AI-based features, prioritize use cases based on feasibility and impact. McKinsey suggests campaign retargeting and brand management. Some platforms have tools to identify suitable AI use cases and CRM functionalities you can fully leverage (if your data availability matches their requirements), including Einstein’s guide to AI use cases and readiness assessor.
Data processing
Data processing
The ML algorithms used to process information and build the data models that fuel most AI-based features should be trained on vast datasets. Also, AI systems require massive computing power.
If you build your solution on top of an AI-based CRM platform, model training and data processing won't be a major issue, as you'll rely on the selected platform’s native capabilities. As for a custom CRM, you can still integrate your software with cloud-based ML tools, such as Amazon SageMaker, to benefit from their built-in algorithms, pre-trained models, and scalable computing resources, or purchase your own infrastructure with powerful GPUs, depending on your business and technical requirements.
Bridging AI and customer data
.jpg)
As customer interactions, driven by the rise of ecommerce and other digitalization trends, progressively shift towards virtual spaces, the volume of generated data constantly expands. The latest advancements in AI technologies provide the right tools to turn these data assets into valuable insights. AI-enabled CRM represents the perfect embodiment of this data-driven approach to customer management. Equipped with AI functionalities, CRM platforms enhance decision-making, streamline business processes, and improve customer satisfaction and retention. To fully seize the benefits of an AI-driven CRM, rely on an experienced partner like Itransition.
Looking for a CRM consulting partner?
FAQ
What is the best AI-powered CRM?
While each major CRM platform with AI-based features has its strengths and can fit various business scenarios, both Gartner Magic Quadrant and G2 Grid identify Salesforce as the current market leader.
What is the tech stack of an AI-based CRM?
The tools and technologies commonly used in an AI-based CRM include:
- Cloud computing platforms such as Amazon Web Services or Microsoft Azure
- Big data processing tools like Apache Hadoop, Apache Spark, or Apache Kafka
- Machine learning frameworks such as TensorFlow, Keras, or PyTorch
- Natural language processing (NLP) libraries, including NLTK, SpaCy, or Gensim
- Data visualization tools like Tableau or Power BI
- Robotic process automation platforms such as UiPath or Automation Anywhere
Cloud or on-premise CRM with AI features?
Companies prioritizing security or performing large data transfers could prefer an on-premise CRM. However, a cloud environment providing scalable resources can be a natural fit for a CRM with AI capabilities that typically require high computational power.
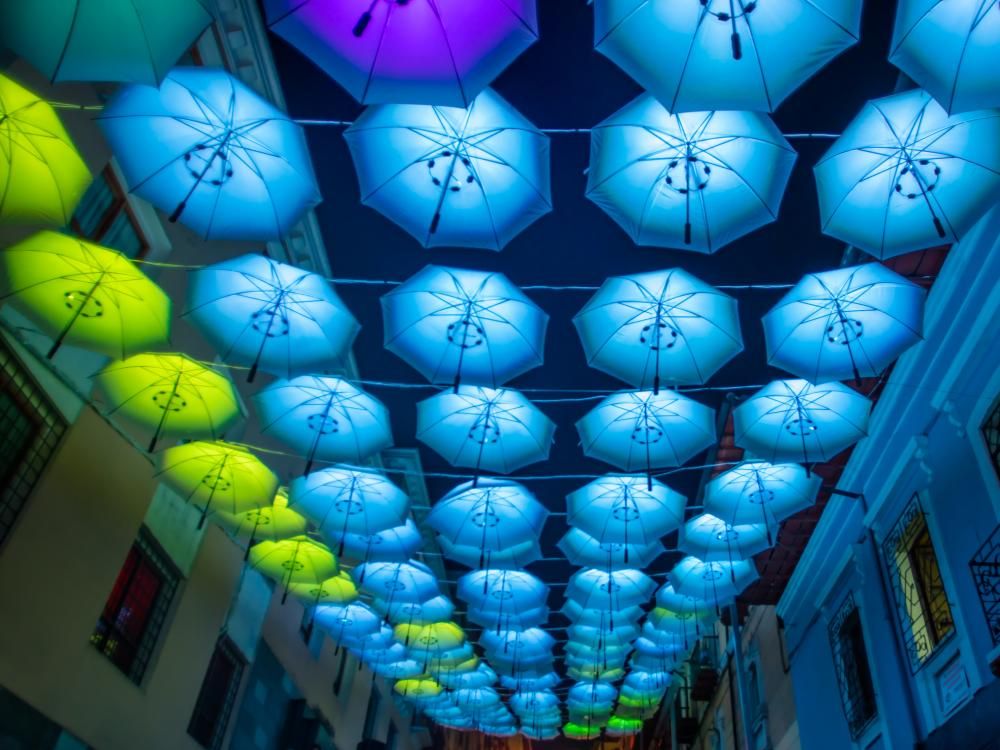
Case study
Salesforce CRM for a US insurance buyout agency
Learn how Itransition’s customized Salesforce CRM has brought a US insurance buyout agency 54% more leads.
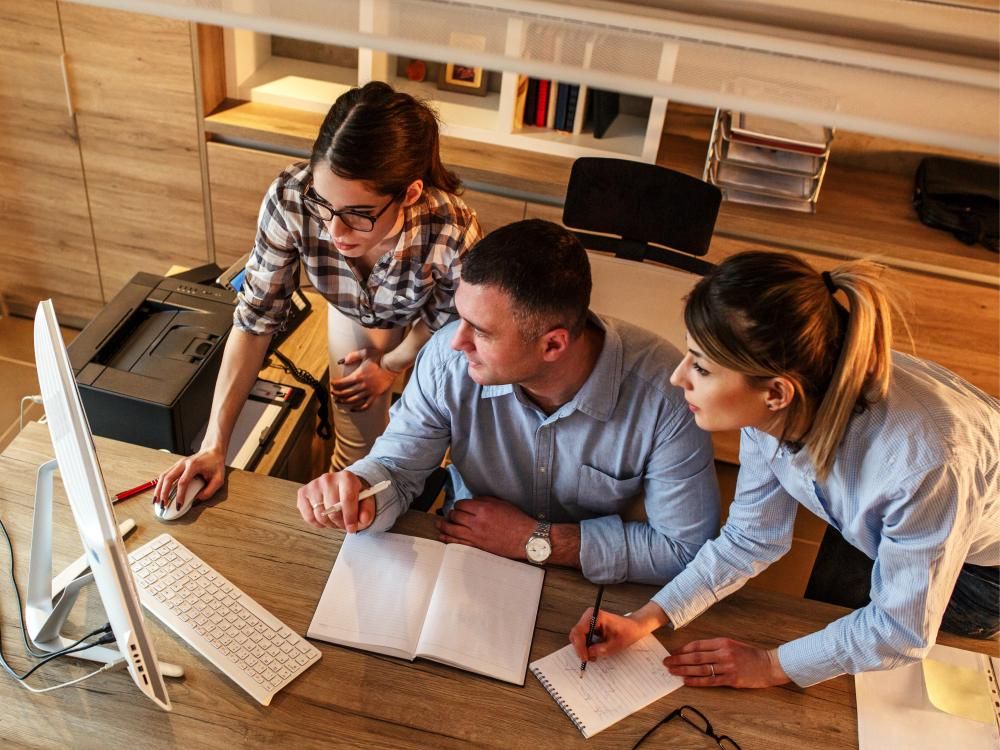
Insights
Why low-code CRM customization gains ground
Learn why low-code CRM customization is a number-one choice for SMBs and enterprises alike and what benefits and problems adopters may get when opting for it.
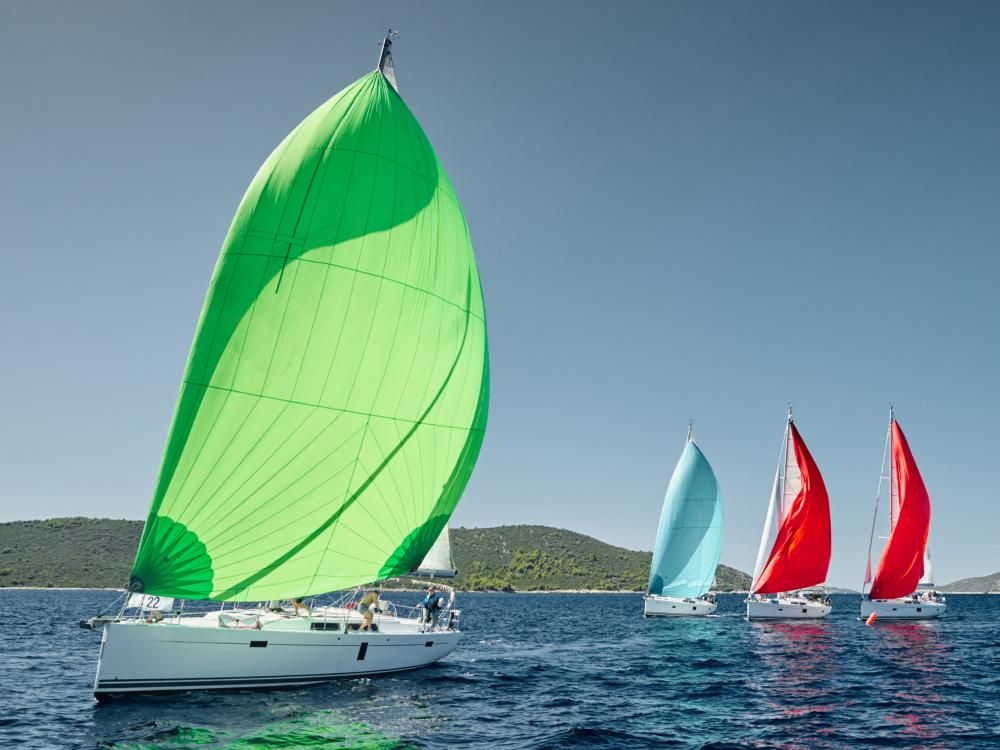
Insights
CRM vs ERP: which one you need?
Learn the difference between CRM and ERP systems and find out whether your business needs a CRM, an ERP, or both.
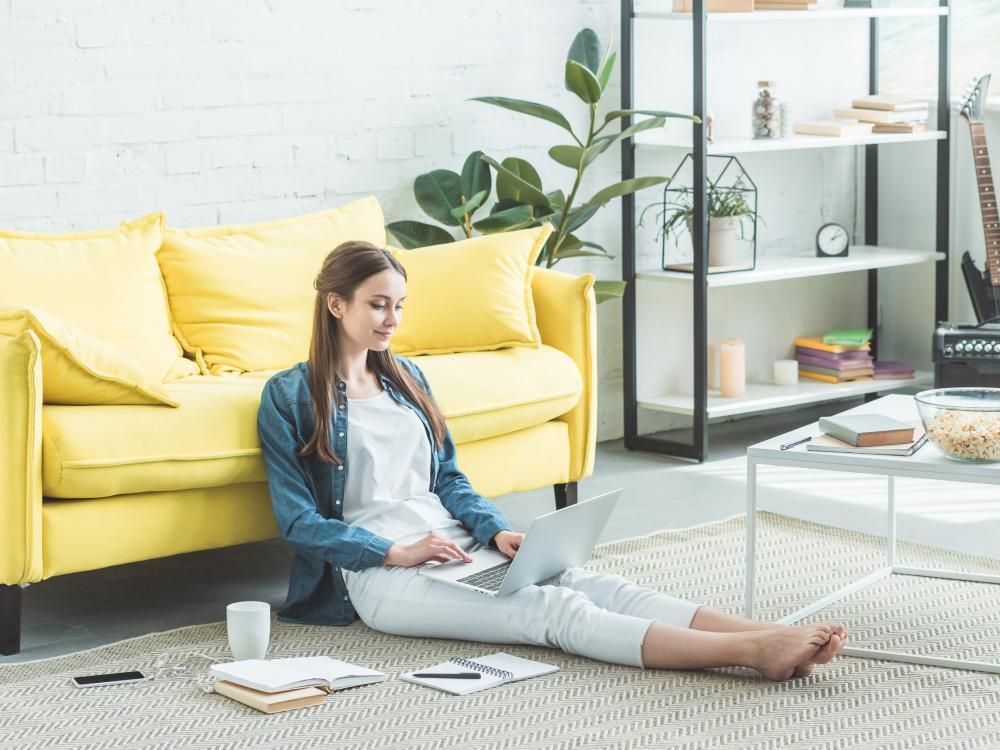
Insights
Collaborative CRM for the remote-working world
Find out why collaborative CRM became a game-changer in the work-from-home reality and learn about its major advantages and features.