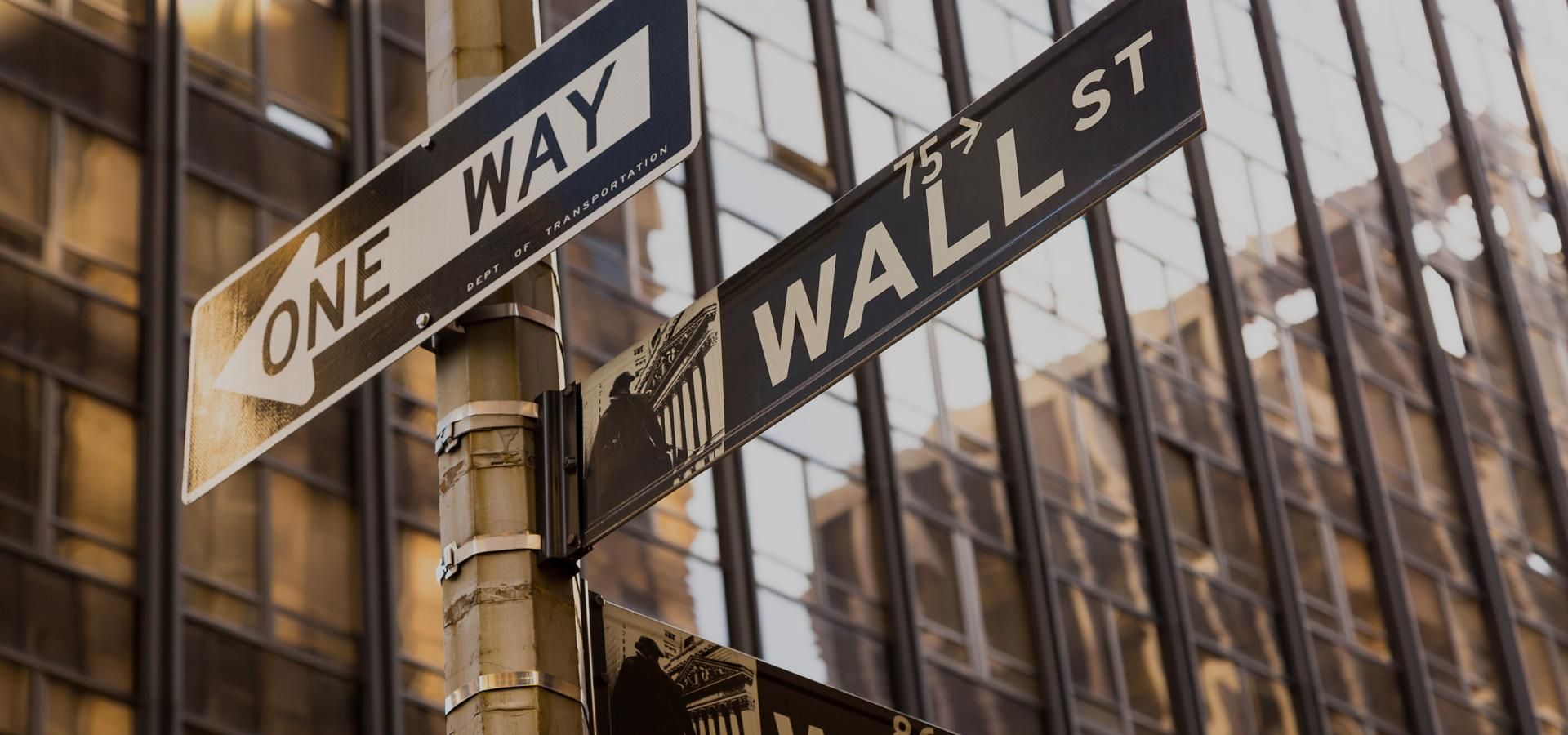
Machine learning for stock market prediction: applications & technical overview
January 14, 2025
- Home
- Machine learning
- Stock market prediction
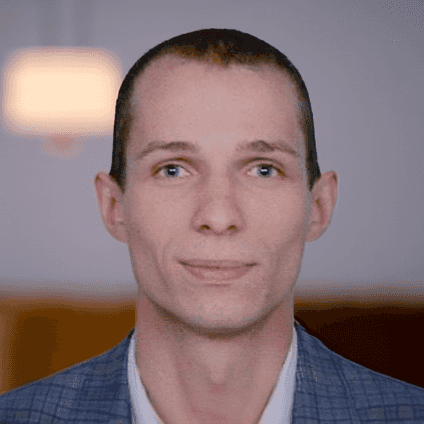
Head of AI/ML Center of Excellence
Machine learning for stock market prediction involves the use of advanced algorithms to forecast the future value of stocks or other financial instruments and provide insights into trading and investment opportunities.
An experienced ML services provider, Itransition delivers financial solutions equipped with predictive analytics capabilities to help retail and institutional traders and investors optimize their trades and portfolios, maximizing profitability while mitigating risk.
ML-powered stock market prediction use cases
Stock trading
Integrated into stock trading software and financial data analytics tools, ML algorithms can forecast stock price fluctuations and market volatility to recommend the most promising stock selection strategies, helping users execute buy and sell orders for maximum short-term profit. ML solutions analyze numerous factors, including global financial trends, corporate earnings, and investor sentiment on social media, to derive these predictions.
Financial firms can also integrate machine learning tools for stock market prediction into algorithmic and high-frequency trading software to automate trades based on stock price forecasts.
Portfolio management
ML-based predictive analytics tools integrated into wealth management platforms can help investors better assess potential asset allocations and build a well-balanced and diversified portfolio comprising stocks, bonds, and commodities that are highly likely to increase in value in the long run. These tools, which can include advanced recommendation engines or AI agents, take into account individual users’ investment goals and risk tolerance, along with multiple financial indicators, to suggest investment options balancing expected returns and risk.
Portfolio management platforms can also incorporate roboadvisors, conversational interfaces that interact with users in natural language to identify their financial goals and provide investment advisory or make investments autonomously based on ML stock price predictions.
Real-life examples of ML-based stock price prediction
Itransition created a portfolio management platform with a custom ML algorithm that can process historical and real-time stock market data to forecast stock prices and trends, helping users optimize their trades and investments. According to our regular comparisons, the algorithm we developed outperforms the S&P 500 index. The solution also sends trading strategy alerts to investors, including notifications on the best time to close a position. The platform currently serves thousands of users, who manage over $20 billion in investments.
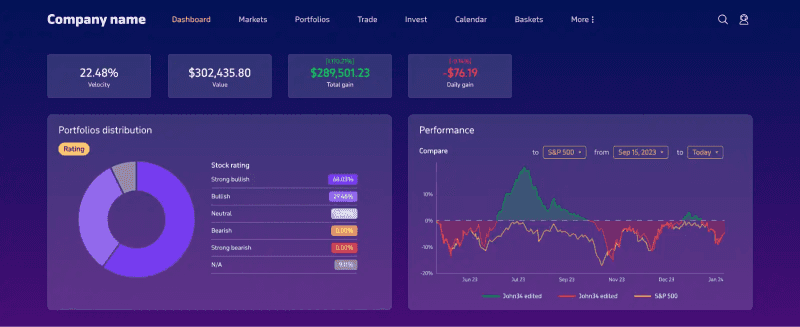
Image title: Portfolio distribution dashboard
Numerai is a San Francisco-based hedge fund whose trades are driven by ML-based stock price predictions. The fund follows an unconventional crowdsourcing model, with thousands of data scientists building algorithms for Numerai and then being rewarded with its native cryptocurrency. Backed by major investors like Paul Tudor Jones and Howard Morgan, it has returned 49% of its initial investments since its founding in 2019.
eToro is a popular social trading platform that recently partnered with InvestorAi to create ML-driven equity portfolios. eToro's models use a mix of technical, fundamental, and sentiment analysis to identify trade signals and allocate assets accordingly. Asset allocation also complements bottom-up, ML-based stock selection with predefined top-down constraints, which can exclude, for example, companies with significant sales declines.
Looking for a reliable ML consulting and development partner?
Top platforms for stock market prediction
Nowadays, both commercial stock trading platforms and custom tools incorporate predictive analytics capabilities to help traders navigate volatile markets and make better investment decisions. Here are some options worth considering for market analysis:
Tableau is a well-known BI and analytics platform by Salesforce that facilitates data analysis through intuitive features like self-service data preparation, no-code machine learning model building, and interactive dashboards. The solution relies on linear regression algorithms to build forecasting models for predicting stock market price fluctuations, sales by segment, and other financial trends. Thanks to the Tableau-Python integration, users can also build complex ML models with Python and then use Tableau to visualize the model’s results.
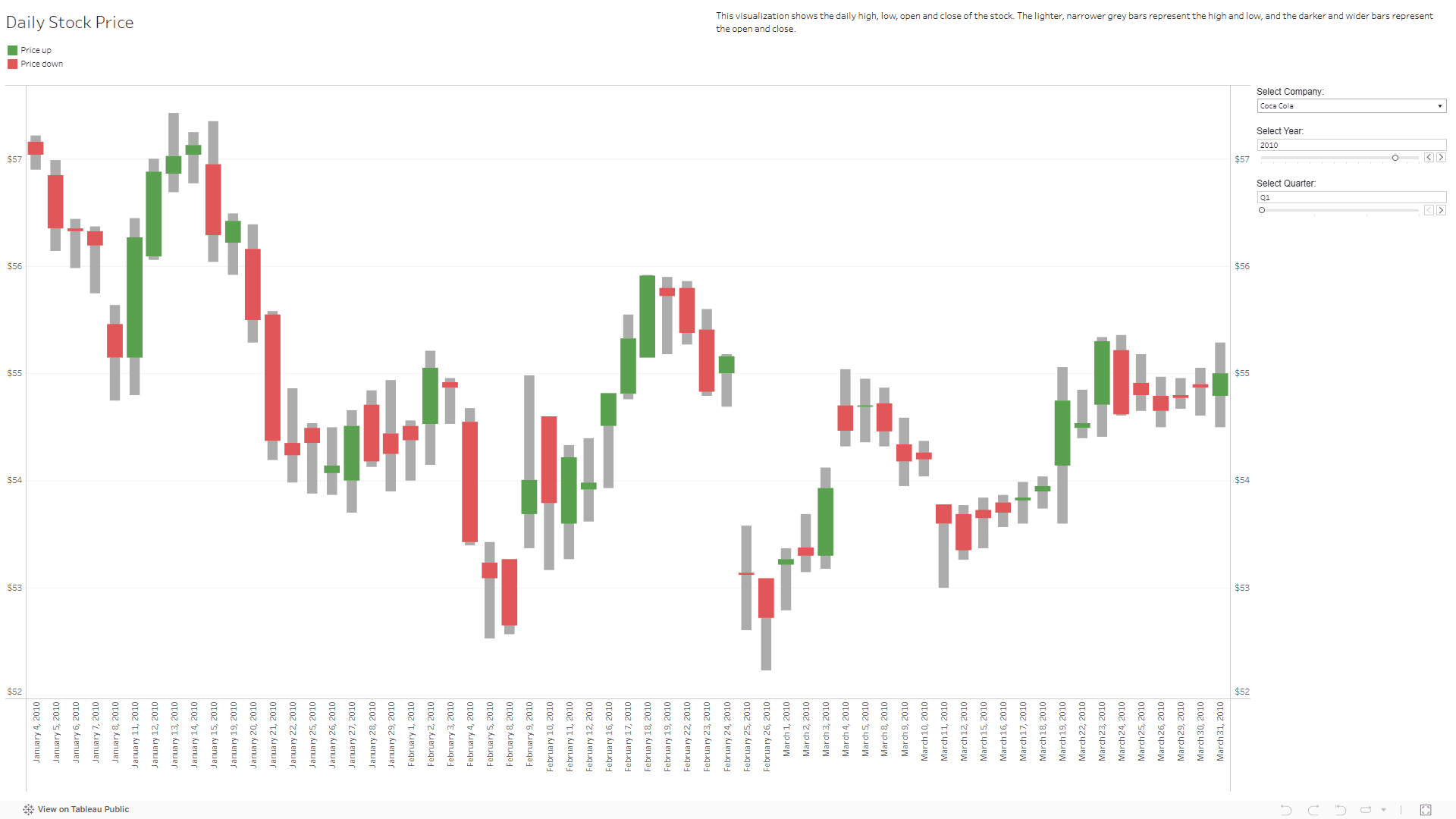
Image title: Tableau’s dashboard for financial analysis
Image source: tableau.com — Determine stock volatility using candlestick charts
TradingView is a financial platform for traders and investors combining data analytics and social media functionality. It provides users with a vast selection of built-in indicators powered by machine learning, including moving averages and oscillators, to forecast market trends based on opening and closing prices, minimum and maximum prices, trading volumes, and other metrics.
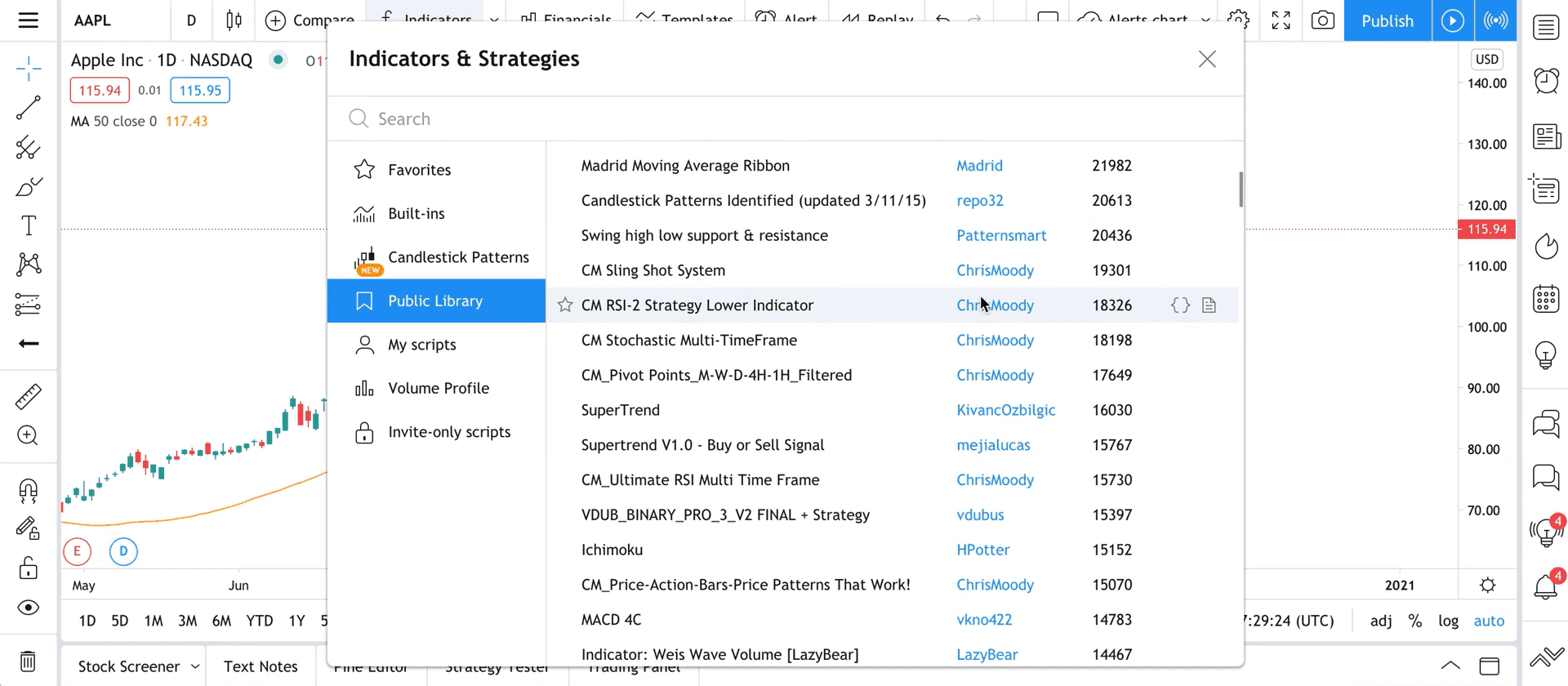
Image title: TradingView’s indicators library
Image source: tradingview.com — What is an indicator?
QuantumStreet is a wealth management platform powered by IBM Watson that helps professional investors identify market opportunities while mitigating financial risk. The solution analyzes news, macroeconomic data, and other information that can impact users' holdings and generates portfolio risk and return projections, along with a confidence score defining the spread between low and high forecasts and therefore the overall forecasting accuracy.
Best ML algorithms for stock price prediction
Ranking machine learning algorithms for stock market forecasts can be a complex task for two main reasons:Â
- Research is still ongoing and far from achieving universally accepted results, since the range of algorithms suitable for this purpose is pretty wide and evaluating their accuracy in a variety of different scenarios can be challenging.
- FinTech corporations and investment firms are typically reluctant to reveal their trump cards in order to maintain a competitive edge. This is why most performance data on different ML-based stock price forecasting methodologies and information regarding their actual deployment maturity is kept off the radar of independent researchers.
Traditional machine learning
The term "traditional" refers to all those ML algorithms not belonging to the deep learning subset of machine learning.
Pros
ML algorithms have shown relatively high accuracy, especially when processing wide datasets, and even more when combined with each other to produce ensemble models. This fusion among different ML algorithms can easily amplify their potential, since some of them are better at handling stock price data, while others give their best when applied to sentiment data.
Cons
These algorithms can be hypersensitive to outliers and fail to properly detect anomalies and exceptional scenarios.
Among the machine learning techniques and algorithms tested by researchers, we can mention:
- Random forest
This powerful algorithm ensures optimal accuracy for large datasets and is widely used to build regression models, which can identify relationships among multiple variables for stock price prediction.
- Naive Bayesian classifier
This represents an efficient and relatively simple option for investigating smaller financial datasets and determining the likelihood of one event affecting the occurrence of another.
- Support vector machine(SVM)
This is a machine learning algorithm based on supervised learning (trained by providing actual examples of inputs and outputs). It can be particularly accurate with massive datasets but not so good when handling complex and dynamic scenarios.
- K-nearest neighbor
This ML algorithm uses a rather time-consuming, distance-based technique to forecast the outcome of a certain event according to the records of its most similar historical situations called "neighbors".
- ARIMA
This is a statistical technique for time series forecasting that can rely on ML algorithms. It works best with linear (arranged in a sequential manner) data to forecast short-term stock price fluctuation based on past stock trends like seasonality, but cannot handle non-linear (randomly ordered) data and make accurate long-term predictions.
Deep learning
Deep learning leverages more mathematically complex algorithms to derive deeper insights and connections from large sets of data. You can also use hyperparameter tuning techniques to maximize deep learning algorithms’ accuracy.
Pros
Deep learning algorithms outperform traditional ML algorithms in terms of stock price prediction capabilities.
Cons
Deep learning systems need a lot of data for training and generally require significant data storage capacity and computing power.
Most researchers show an increasing interest in the potential applications of deep learning algorithms and models for stock price prediction, including:
- Transformers
This neural network architecture can easily identify relationships across sequential data points, making it a top choice for time series analysis, such as stock price forecasting, which previously relied primarily on long short-term memory (LSTM) networks. Major pre-trained transformer models include TimeGPT, TimesFM, and Chronos.
- N-Beats
This is another popular neural network model for time series forecasting across various industries, including finance. N-Beats combines excellent interpretability (highlighting which data components impact its predictions) and generalization capabilities (handling different types of time series data).
- Recurrent neural networks(RNN)
These are a particular type of artificial neural network (ANN) in which each processing node also acts as a "memory cell", allowing it to store relevant information for future use and send it back to previous layers to refine its output.
Essentials of ML-based stock market prediction adoption
Ensuring data quality & availability
You need to feed ML-based stock market prediction systems with large volumes of high-quality data to ensure they deliver accurate results.Â
- Identify rich and reliable data sources for algorithm training and data analysis. You can choose from a vast selection of financial databases and market intelligence platforms, such as Bloomberg, Reuters, Nasdaq, S&P Global, Polygon.io, MarketWatch, Alpha Vantage, Financial Times, and Marketstack.
- Complement purely economic datasets with information collected from sources like social media posts or financial news articles. This can help you monitor investor perceptions of key stock market trends through sentiment analysis.
- Since data from such sources is typically heterogeneous, you’ll need to integrate and transform it via ETL/ELT pipelines and consolidate it into a suitable repository. This can be, for instance, a data lake to effectively store large volumes of structured and unstructured data, a data warehouse to keep cleansed data ready for analysis, or a database optimized for time series data. ETL/ELT pipeline setup can be facilitated via cloud data integration services.
Improving ML model training for optimal performance
You need to properly approach ML model training since it greatly impacts the output of your stock market prediction solution.
- Massive training datasets imply an enormous range of variables and extremely long training times. You can mitigate this issue by selecting the most relevant variables (trading volume, open price, etc.) to shorten the training phase and make the resulting data models easier to interpret.
- Overfitting can affect stock market prediction models when algorithms are trained too long on a certain financial dataset and the model they produce performs well on that specific set but can't handle new data samples. To address this problem, divide model training data into training, validation, and test sets and cross-validate their outputs. However, you may still encounter this issue in production. That’s why, in the pilot phase, SMEs should validate every action taken by the algorithm. A post- or pre-check approach can be implemented depending on the algorithm.
- Assess model performance with suitable metrics based on your algorithm’s task. Mean squared error, mean absolute error, root mean square deviation, and mean absolute percentage error are popular metrics for financial predictions. Precision and Recall or F1 can also be useful for certain tasks.
- Model performance can degrade over time due to progressive changes in input variables. This phenomenon, known as model drift, can be addressed by performing multiple post-deployment retraining iterations to adjust model output. Set up proper model drift monitoring to understand when to execute such iterations.
Combining fundamental & technical analysis
Training datasets for ML and deep learning algorithms are typically large and include a variety of data types. As for the latter point, there are two major research methods prioritizing different types of data:
Fundamental analysis
tries to determine the intrinsic value of a stock and its future fluctuations by monitoring market and industry parameters and corporate-related metrics, such as market capitalization, dividend, deliverable volume, net profit and loss, P/E ratio, and total debt.
Technical analysis
focuses on stock price and volume trends over time to spot recurring patterns and predict future price movements, especially in the short term. These may include the well-known head and shoulders, triangles, cup and handles, and so on.
Your ML system for stock price prediction should include both methods and be fueled with a full spectrum of data types, encompassing corporate data and stock price patterns, to better frame the financial scenario under consideration.
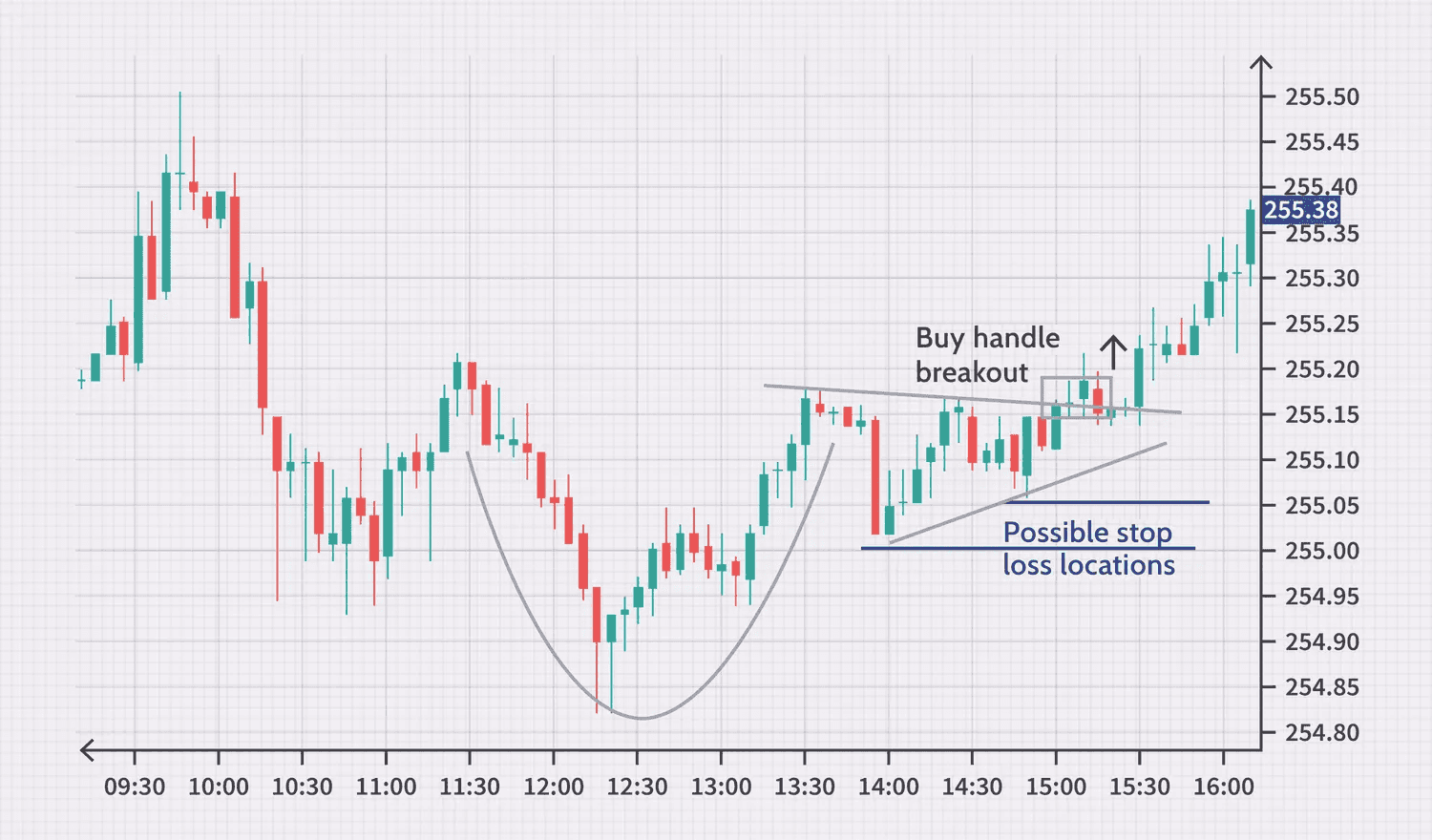
Image title: Examples of stock price patterns. Cup and handles
Image source: investopedia.com — How to Spot Key Stock Chart Patterns
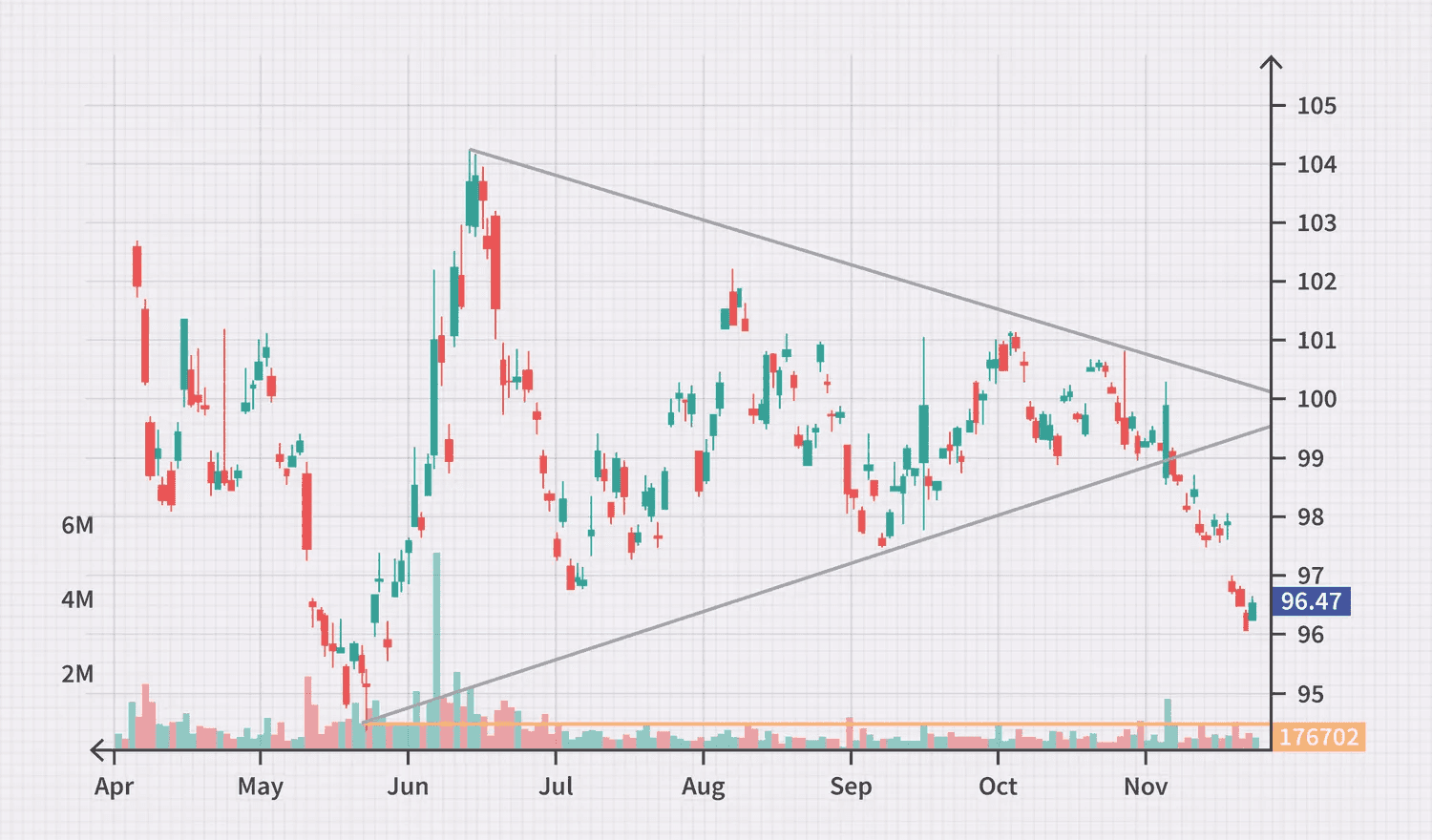
Image title: Examples of stock price patterns. Symmetrical triangle
Image source: investopedia.com — How to Spot Key Stock Chart Patterns
Complexities of ML-powered stock market forecasting to consider
Despite the potential of ML-based stock price prediction systems, their implementation in real-world scenarios can be hampered because of their data-driven nature or even lead to unexpected outcomes.
Challenge
Considerations
Market anomalies
Algorithms build their models on historical data to predict financial trends but can struggle to face anomalous scenarios, such as a global pandemic and its tremendous impact on the markets. Moreover, stock exchange data is rather limited, as the modern financial market is relatively young and a good portion of human history is unknown to algorithms.
Algorithms build their models on historical data to predict financial trends but can struggle to face anomalous scenarios, such as a global pandemic and its tremendous impact on the markets. Moreover, stock exchange data is rather limited, as the modern financial market is relatively young and a good portion of human history is unknown to algorithms.
While these issues can’t be completely solved, their effects can be mitigated by adopting a separate anomaly detection system to identify outliers and plan your trading activities and business strategies accordingly.
Over-reliance on predictive models
If all traders followed identical ML-prompted investment strategies, they’d end up buying and selling the same equity securities and vaporizing any potential gain. A similar episode, known as the Quant Meltdown, happened in 2007 when a bunch of relevant hedge funds guided by similar quantitative models sold their stock simultaneously and suffered massive losses.
If all traders followed identical ML-prompted investment strategies, they’d end up buying and selling the same equity securities and vaporizing any potential gain. A similar episode, known as the Quant Meltdown, happened in 2007 when a bunch of relevant hedge funds guided by similar quantitative models sold their stock simultaneously and suffered massive losses.
Businesses should implement a system with different levels of trust in ML-based solutions, ranging from full reliance on their autonomous operation to the submission of their findings for a designated specialist’s review.
Our machine learning services
.jpg)
Our consultants offer expert guidance for your ML project, helping organizations speed up the solution’s implementation, overcome technical roadblocks, and maximize the adoption benefits of this technology.
- Use case identification
- Data mapping and quality assessment
- Current solution audit
- Initial project setup advisory
- Development process overview
- ROI analysis
- Solution architecture design
- Tech stack selection
- Project budgeting
- MVP conceptualization
- Risk management strategy definition
- User training (demos, tutorials, etc.) and support
We deliver ML solutions aligned with your business requirements and financial industry’s specifics or enhance your existing software to meet evolving needs and embrace emerging tech trends.
- ETL pipeline setup
- Data preprocessing, including cleansing, annotation, and transformation
- Data protection and cybersecurity elaboration
- Selection of suitable machine learning techniques and algorithms
- ML and deep learning model training
- Software integrations and APIs creation
- UX/UI and data visualization setup
- Deployment to production
- End-to-end testing
- Post-launch support, optimization, and upgrades
Team up with Itransition to implement your ML solution
Predicting stock prices with ML-powered oracles
With an increasingly interconnected global market, financial institutions are facing an amplified butterfly effect where seemingly minor variables can project their effects further and stronger than expected. Machine learning-powered systems can help these organizations forecast future events, providing them with valuable insights to address market volatility.
To take full advantage of artificial intelligence, ML, data science, and other advanced technologies, consider relying on an experienced IT partner like Itransition.
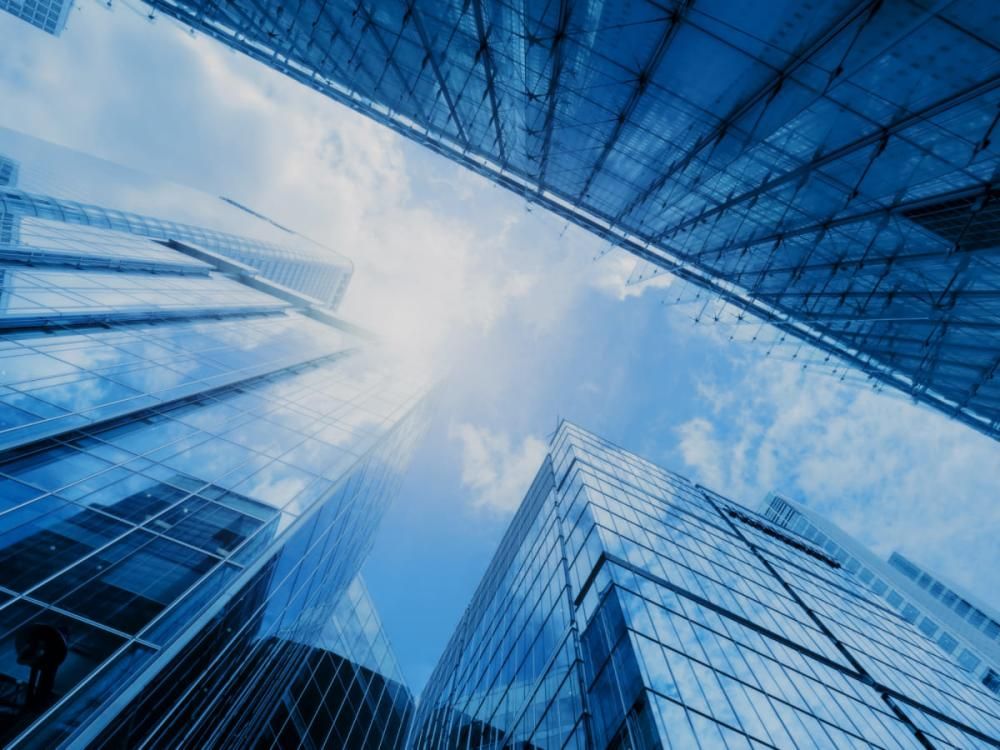
Insights
AI in fintech: use cases,
 solutions & implementation challenges
Explore the top use cases, real-life examples, and branches of AI in fintech, along with common adoption challenges and guidelines to address them.
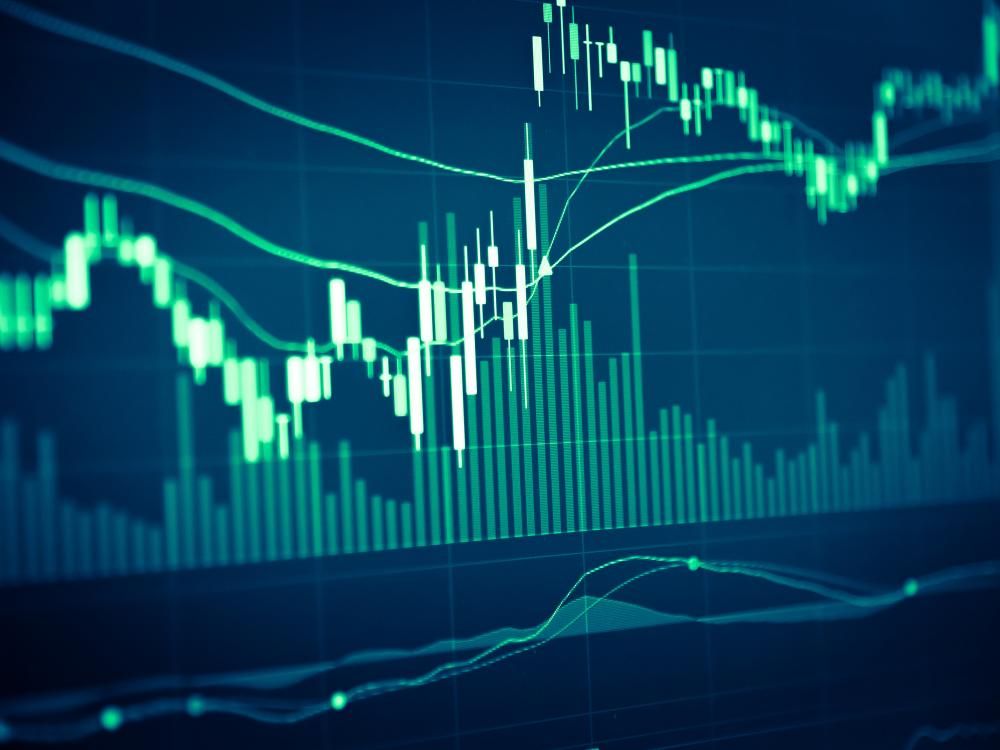
Case study
Trading platform software development
Learn how Itransition developed iOS and Android applications for a European bond trader with over $1 billion raised in funds for their clients.
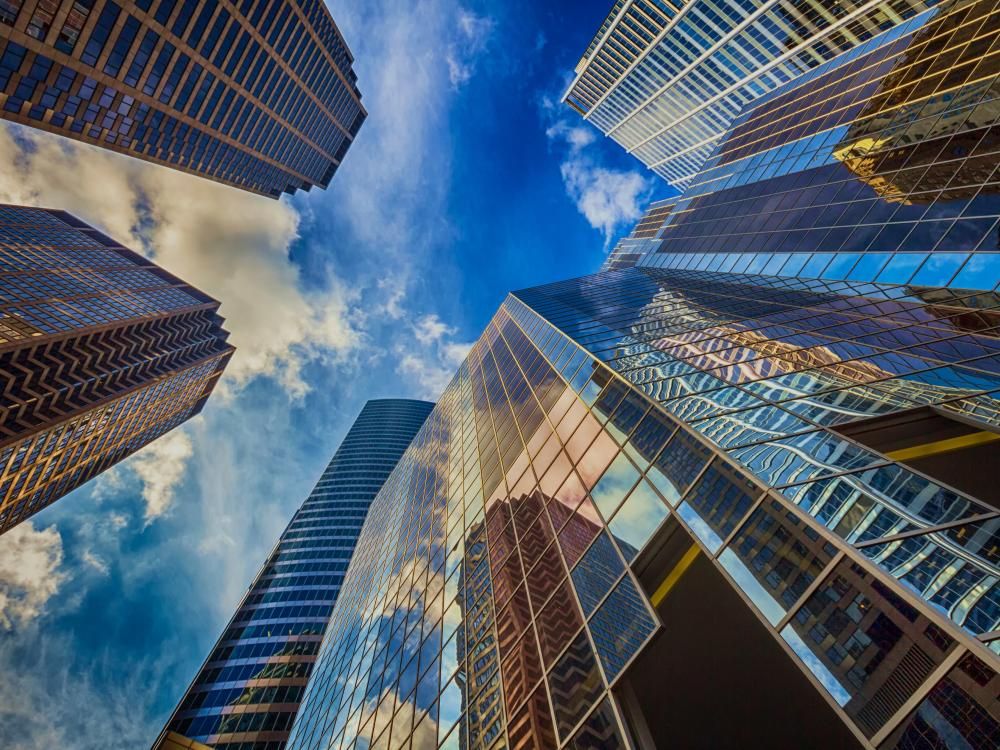
Insights
Business intelligence for finance: capabilities, tools & integrations
Explore how business intelligence and data analytics benefit the financial services sector and learn what capabilities, integrations, and tools make up a successful BI strategy.
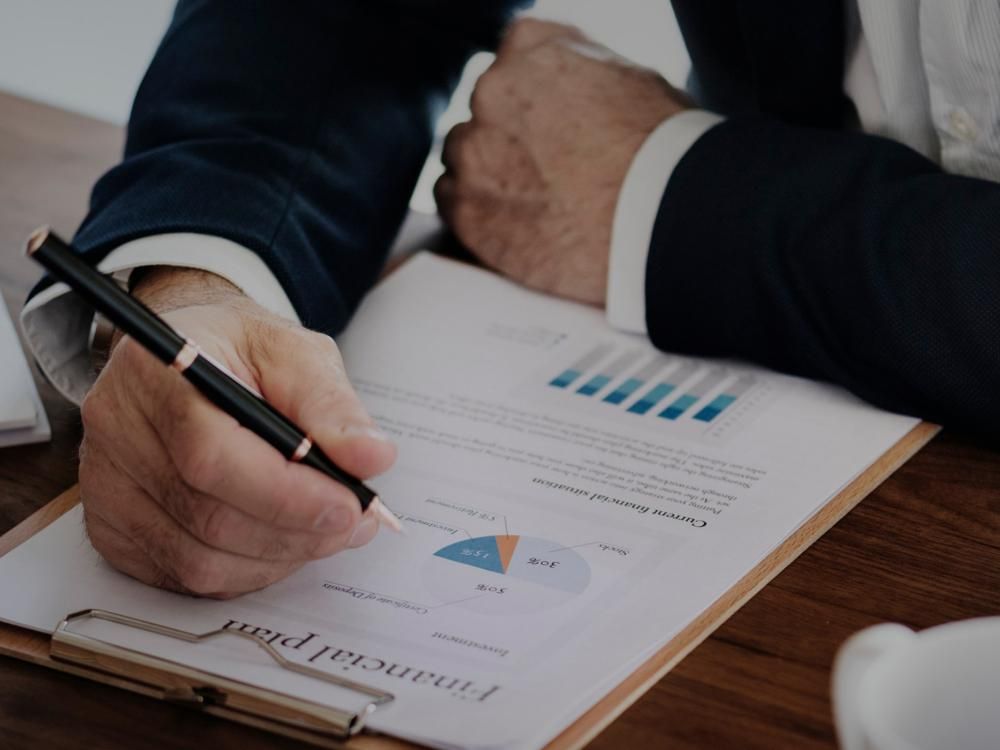
Case study
BI consulting and engineering for a commercial bank
Find out about Itransition's high-profile BI consulting for a Canadian bank, including data architecture analysis and a BI strategy.
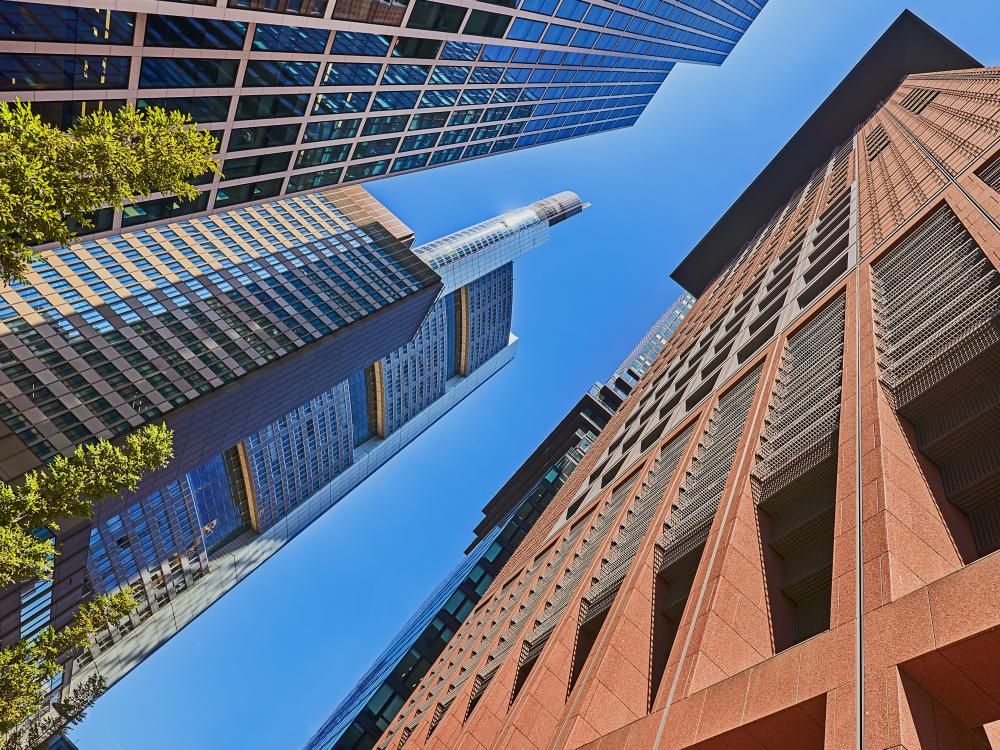
Insights
Machine learning in banking: 8 use cases and implementation guidelines
Learn how banks can apply machine learning to improve customer engagement, streamline compliance, and drive more revenue.
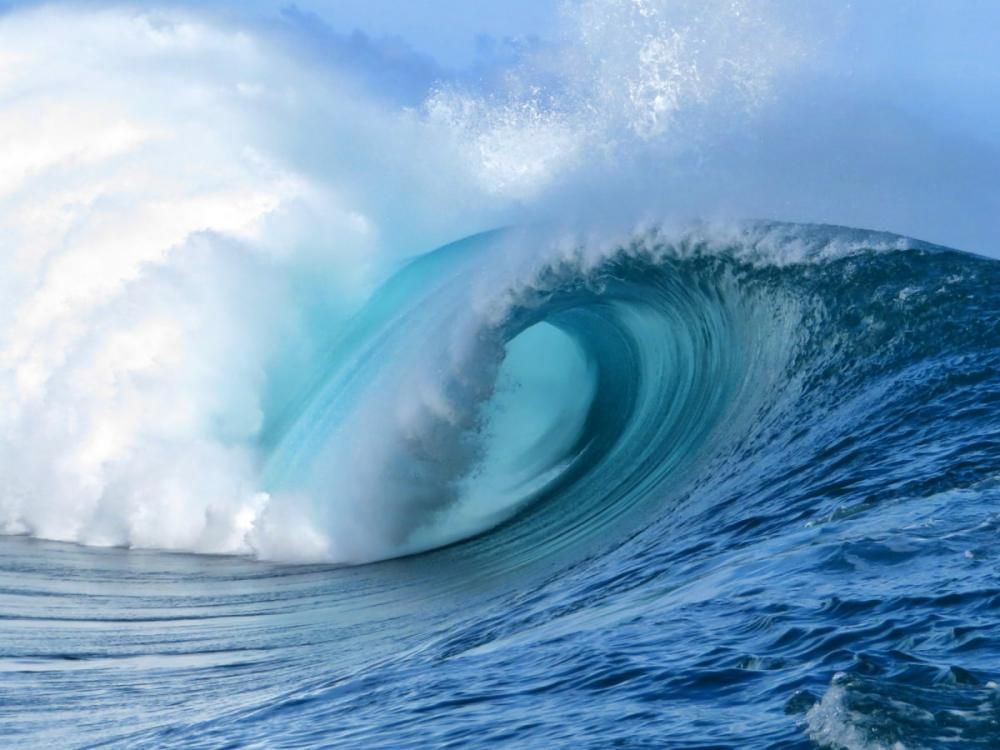
Insights
Machine learning for fraud detection: tech overview, use cases & challenges
Find out how ML for fraud detection works, along with key use cases, real-life examples, and the benefits and challenges of adopting this advanced technology.
More about machine learning services
Services