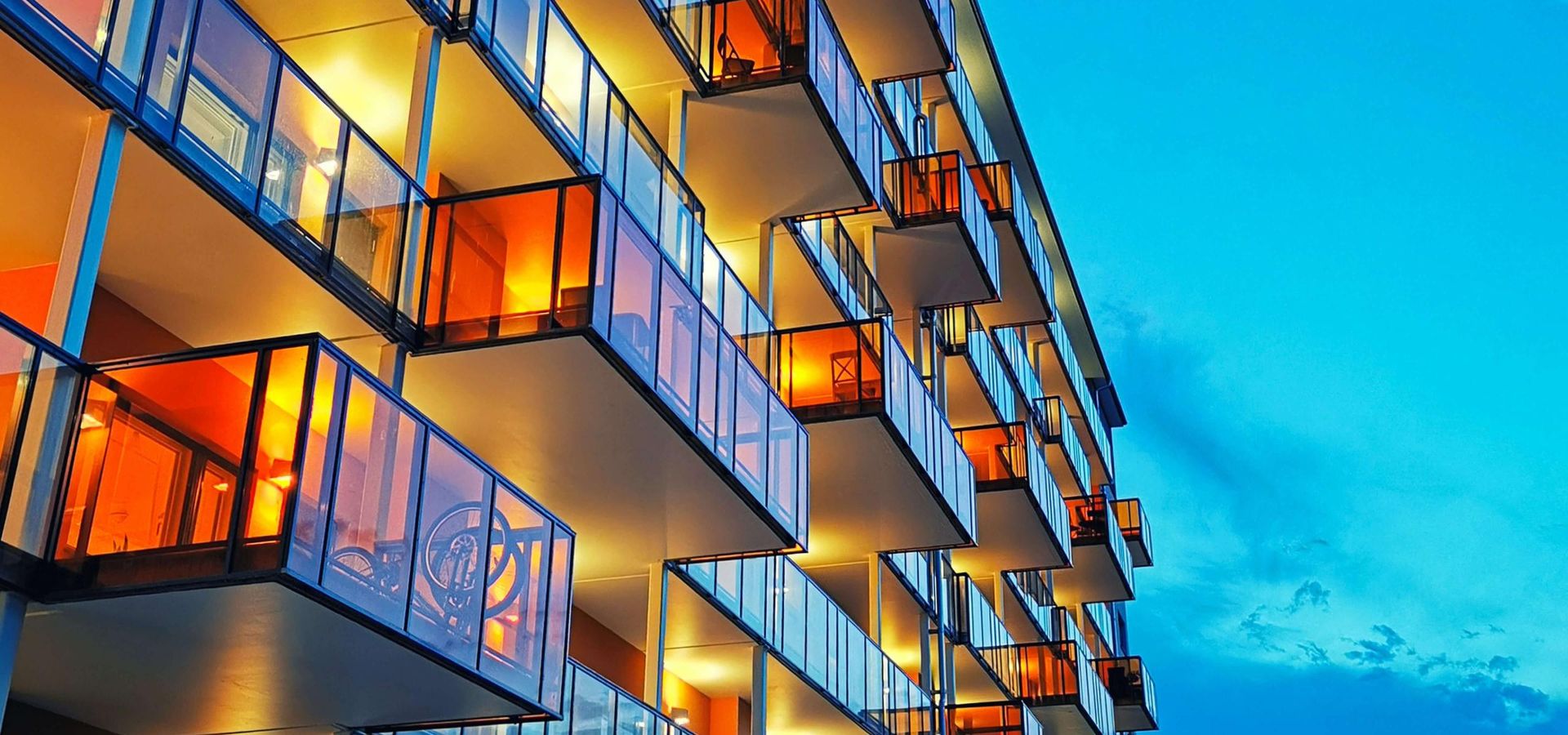
Machine learning in real estate: use cases, examples, and adoption guidelines
August 31, 2023
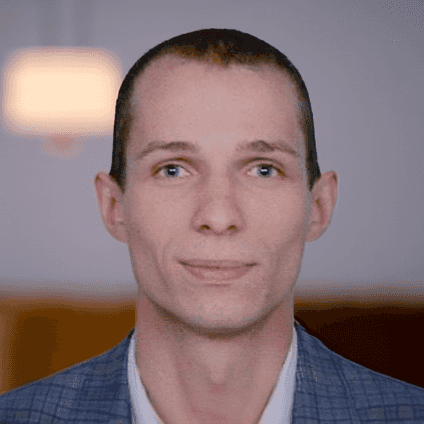
Head of AI/ML Center of Excellence
Learn how an experienced machine learning development company can help your organization embrace data-driven decision-making, support real estate agents, and drive sales
ML trends in the commercial real estate market
of CRE firms employ AI and machine learning to support decision-making
Altus Group
of CRE firms plan to invest in AI and ML capabilities in the next 2-3 years
Altus Group
of CRE firms are investing in ML, data science, and related tech to gain a competitive edge
Altus Group
Scheme title: Real estate disruptors by importance
Data source: pwc.com — Emerging trends in real estate 2023
8 ML use cases in real estate
Nowadays, AI-based technologies are a staple for most real estate platforms, apps, and services, supporting most corporate functions and business processes in this industry.
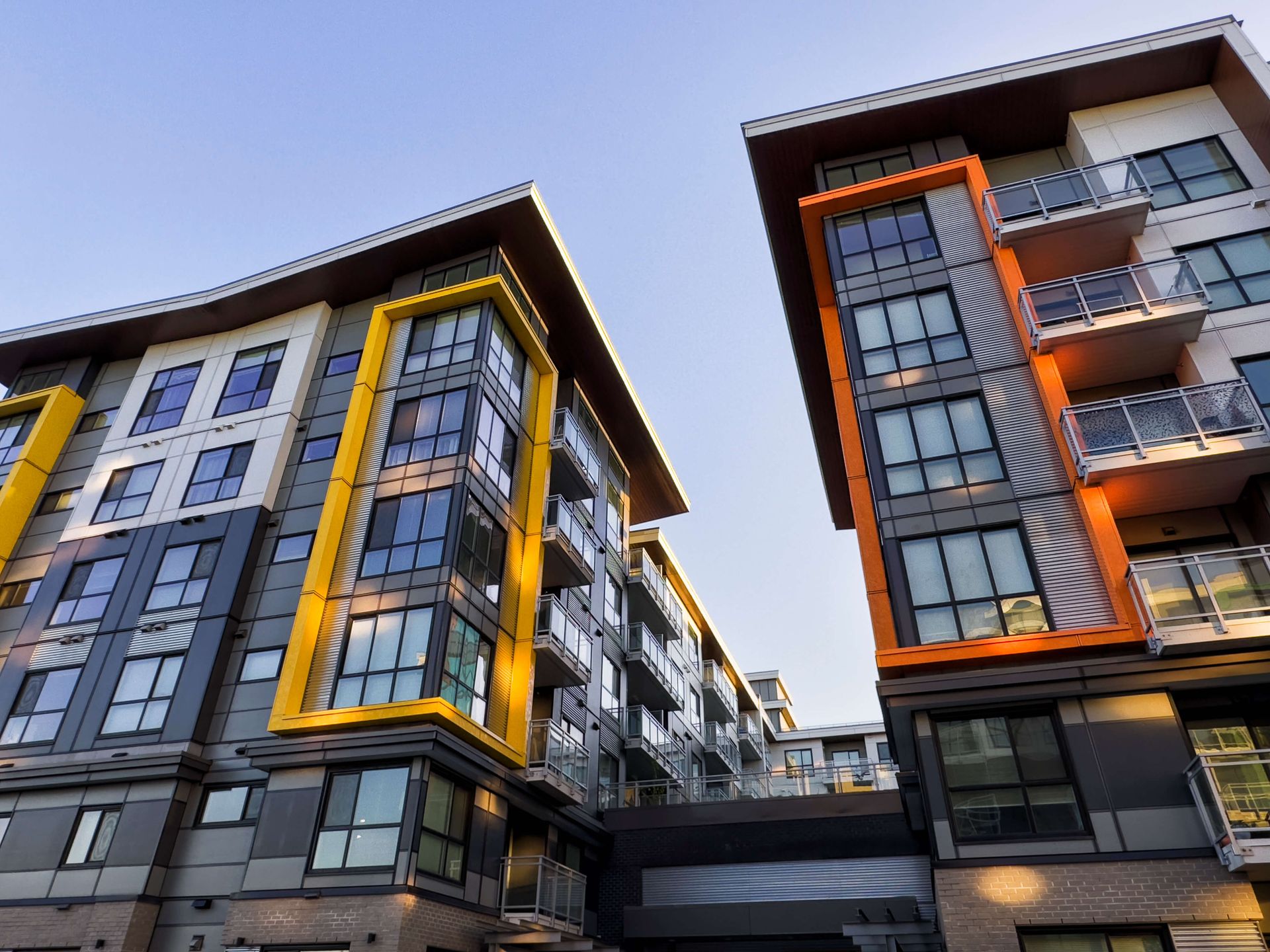
1 Â Real estate appraisal
2 Â Portfolio management
3 Â Targeted advertising
4 Â Recommendation systems
ML-based recommender systems implemented on major real estate platforms provide customers with tailored suggestions based on past online interactions, including search history. Similar to their counterparts deployed in retail, the aim of these engines is to narrow down property search and route users to assets matching their needs and requirements.
5 Â Chatbots
6 Â Asset monitoring
Coupled with IoT devices, ML solutions for anomaly detection can monitor key components of a property, including the electrical system, to identify any outliers, forecast failures, and enable predictive maintenance. These tools also optimize power consumption by spotting any shift from ideal conditions, such as suspicious spikes in energy usage.
7 Â Fraud detection
Real estate enterprises can leverage ML to safeguard their businesses from fraudulent activities and streamline KYC procedures. Powered by machine learning algorithms, fraud detection systems cross-check financial transactions, criminal records, creditworthiness, and other data on potential partners, tenants, or customers to identify inconsistencies.
8 Â Finance and accounting
Enhanced with AI-based NLP capabilities and superior context understanding, RPA bots in real estate can replace or assist managers and accountants in performing a wide range of clerical tasks. Automatable processes include tax billing and reporting, lease contract processing, tenant onboarding, bank reconciliation, AR/AP, and NAV calculations.
Ready to enhance your business with machine learning?
Real-life examples of ML in real estate
Redfin implemented an ML-powered real estate appraisal system enabling users to estimate the market value of their properties based on over 500 metrics, including buyer demand and neighboring home prices. The tool estimates around 92 million properties across the US, showing a valuation accuracy of 98% for on-market homes and 93% for off-market assets.
Zillow recently equipped its real estate platform with an advanced NLP feature that leverages ML algorithms to answer search queries more efficiently. This solution can understand human-like communication, sift through millions of listings, and recommend properties with features matching the descriptions provided by users in the search bar.
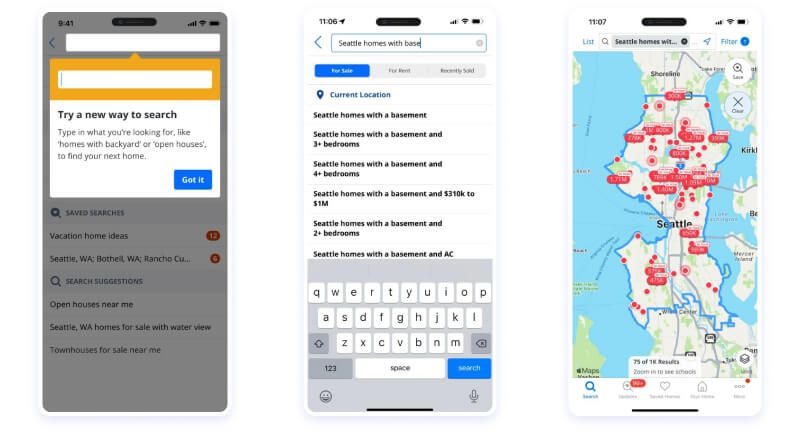
Image title: Zillow’s search feature interface
Data source: Zillow
This Israeli-based PropTech company created a commercial real estate analytics platform capable of processing data gathered from hundreds of sources and covering over 400,000 assets to provide actionable insights. Skyline's ML-driven solution can also identify emerging market trends, pinpoint untapped opportunities, and help firms make profitable investments.
Gridium developed an ML-based data analytics solution to optimize properties' energy consumption, reduce their environmental footprint, and streamline maintenance operations. By adopting this tool, real estate investor KBS saved $47,000 in electricity costs and other operating expenses at the Premier Office Towers in Emeryville, California.
ML adoption roadmap for real estate companies
The path to implementing machine learning can vary depending on the use case and the type of solution selected. However, these are some typical steps you may go through.
1
Business analysis
2
Initial data analysis
3
Product design
4
Building the ML solution
5
Model integration and deployment
The coding phase encompasses front-end and back-end development, followed by embedding the ML model into the software architecture to enable its AI-powered functionalities. You should also configure all necessary API- or ESB-based integrations to fuel your solution with data from other applications and IoT sensors. Cloud data integration tools can help in this regard. Then, you can deploy the ML solution on-premise or in a cloud-based environment.
6
Support
Benefits of ML in real estate
Wider data pool
ML-powered cognitive technologies like NLP and computer vision can harvest real estate and market data from multiple sources, providing analysts with a wider set of real estate-related metrics to better interpret trends or phenomena.
Superior valuation accuracy
According to Forbes, ML-powered Automated Valuation Models (AVM) can assess the value of an asset with an absolute error of less than 4% for homes and under 6% for commercial properties, offering accurate insights for better investment decisions.
Increased sales performance
ML solutions encompass powerful tools, such as recommendation engines, to route customers toward suitable listings, along with features and techniques to drive sales, including user segmentation, targeted advertising, and lead scoring.
Cost reduction
From the automation of time-consuming clerical processes via software bots to power consumption monitoring through data analytics platforms, machine learning solutions help real estate organizations to optimize their budgets and resources.
Improved customer experience
Thanks to machine learning, your customers and tenants can benefit from instant and convenient support via chatbots and virtual assistants. Furthermore, real estate platform users can enjoy easier property searching, tailored recommendations, and virtual tours.
Risk mitigation
ML systems' anomaly detection and predictive analytics capabilities safeguard your business from various financial risks, including fraud and market volatility. They also help ensure safer living environments through predictive maintenance.
Partner with Itransition to bring your machine learning project to life
Current challenges of using ML in real estate
While machine learning benefits your business, implementing it can involve various business and technical complexities. Here are some tips to overcome typical obstacles and streamline ML adoption.
Issue
A common obstacle to machine learning implementation you can face in the early assessment stage involves selecting a use case where it makes sense to opt for a ML solution over more conventional technologies. In this regard, O'Reilly’s 2020 survey ranks use case identification second among the major AI adoption challenges. Still, this choice is crucial, as it will determine the ROI of your ML project and impact executives’ and stakeholders’ buy-in.
Recommendations
Given ML's requirements in terms of model training, architecture, and processing power, solutions based on this technology can be more costly than "standard" software. So, it's worth investing in ML to enhance the most impactful and profitable business domains in your industry or key corporate functions suffering from major inefficiencies. According to Altus Group, for instance, most real estate firms adopt ML to target markets and assets for investments, streamline internal operations, enhance portfolio management, facilitate property valuation, and select partners.
Scheme title: Top reasons CRE firms are investing in ML and data science

Data source: Altus Group — The state of data science in commercial real estate investing
Data collection
Issue
Alongside evident parameters, such as square footage, number of rooms, days on the market, and cost of homes in the same area, many not-so-obvious variables still influence the attractiveness of a real estate asset. These should be taken into account to assess the value of a property. The problem is where to find this information and how to sift through such a vast array of data types and sources.
Recommendations
Realtors have started considering a broader ensemble of non-traditional parameters, such as the quality of local services, employment opportunities, and crime rate. Applications of ML-based cognitive technologies for automated data collection, such as NLP and computer vision, help gather this information from various sources, including social media, market research, sociodemographic reports, satellite imagery, and review sites. These insights can be combined with information from public real estate databases and corporate systems like ERP or CRM.
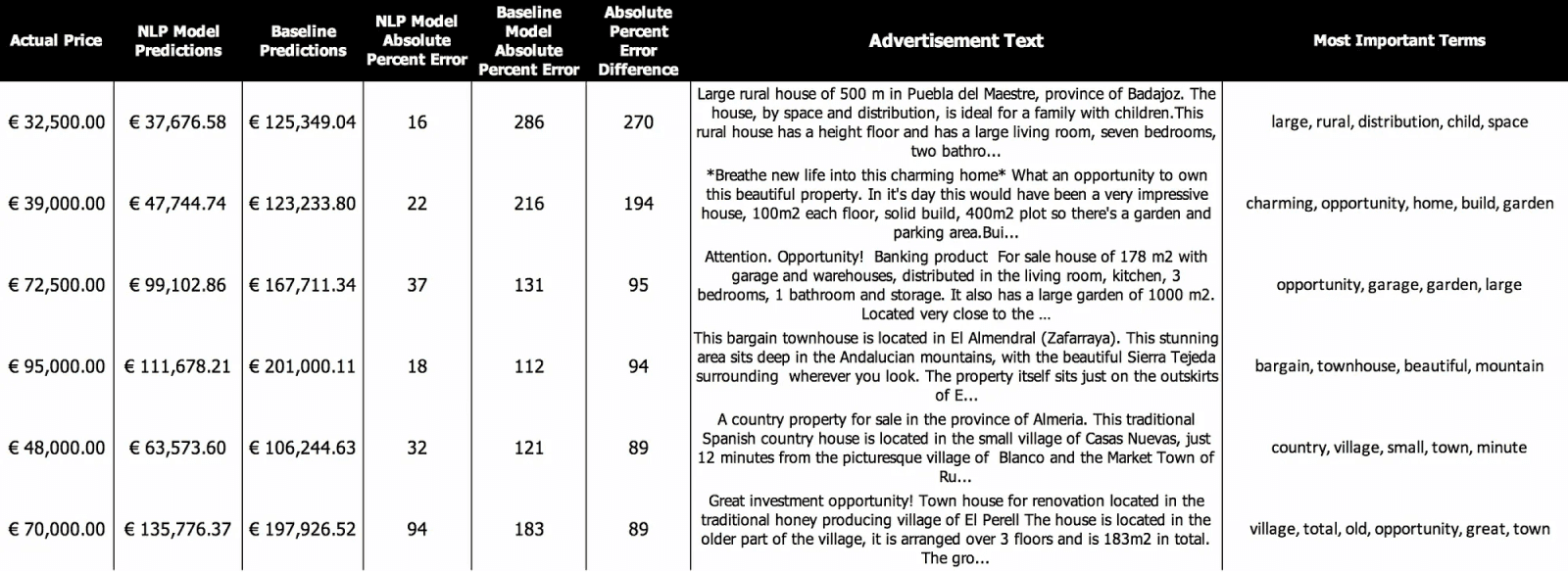
Image title: Example of NLP in real estate advertisement analysis

Note: Advertisement predictions that saw some of the highest improvements in performance with including NLP features

Data source: becominghuman.ai — NLP with Real Estate Advertisements, part 2
ML model reliability
Issue
ML systems operating without supervision can and will deliver inaccurate forecasts, resulting in huge financial disasters (see Zillow's 2021 algorithm failure). This can happen because the ML model was trained on outdated, low-quality data sets. An ML system can also turn out inaccurate due to overfitting, i.e., when a model is trained too long on a specific data set and performs poorly with new data points, or on ML model drift, namely when its performance degrades over time due to input changes.
Recommendations
No ML model or algorithm achieves 100% accuracy. That said, adopting a data management strategy encompassing data cleansing and transformation through ETL pipelines, metadata management, and data warehousing can help ensure the reliability of the data you'll train your model with. Also, splitting such data into training, validation, and test sets will mitigate overfitting issues. As for preventing model drifts, ongoing monitoring and fine-tuning with new datasets through multiple iterations (as recommended by MLOps best practices) can do the trick.
Scheme title: AWS-based training pipeline for an ML-powered real estate platform

Data source:  AWS — Machine Learning Infrastructure for Commercial Real Estate Insights Platform
Data privacy and security
Issue
Given the amount of sensitive information, including financial data, that real estate artificial intelligence software needs to handle, organizations are likely to face hacking, breaches, and leaks. Integrations with external systems and IoT devices further expand the range of points of vulnerability. Therefore, poor data security can adversely affect business operations and corporate reputation and draw the attention of financial authorities and regulators.
Recommendations
Your AI solution should comply with applicable standards and regulations (HIPAA, GDPR, etc.) and be equipped with cybersecurity features encompassing identity and access management, multi-factor authentication, event management, and user activity monitoring. You can also rely on encrypted data exchange via cryptographic protocols (such as Transport Layer Security, X.509 certificates, or symmetric keys) and device authentication. Furthermore, consider adopting a data governance strategy and related internal policies.
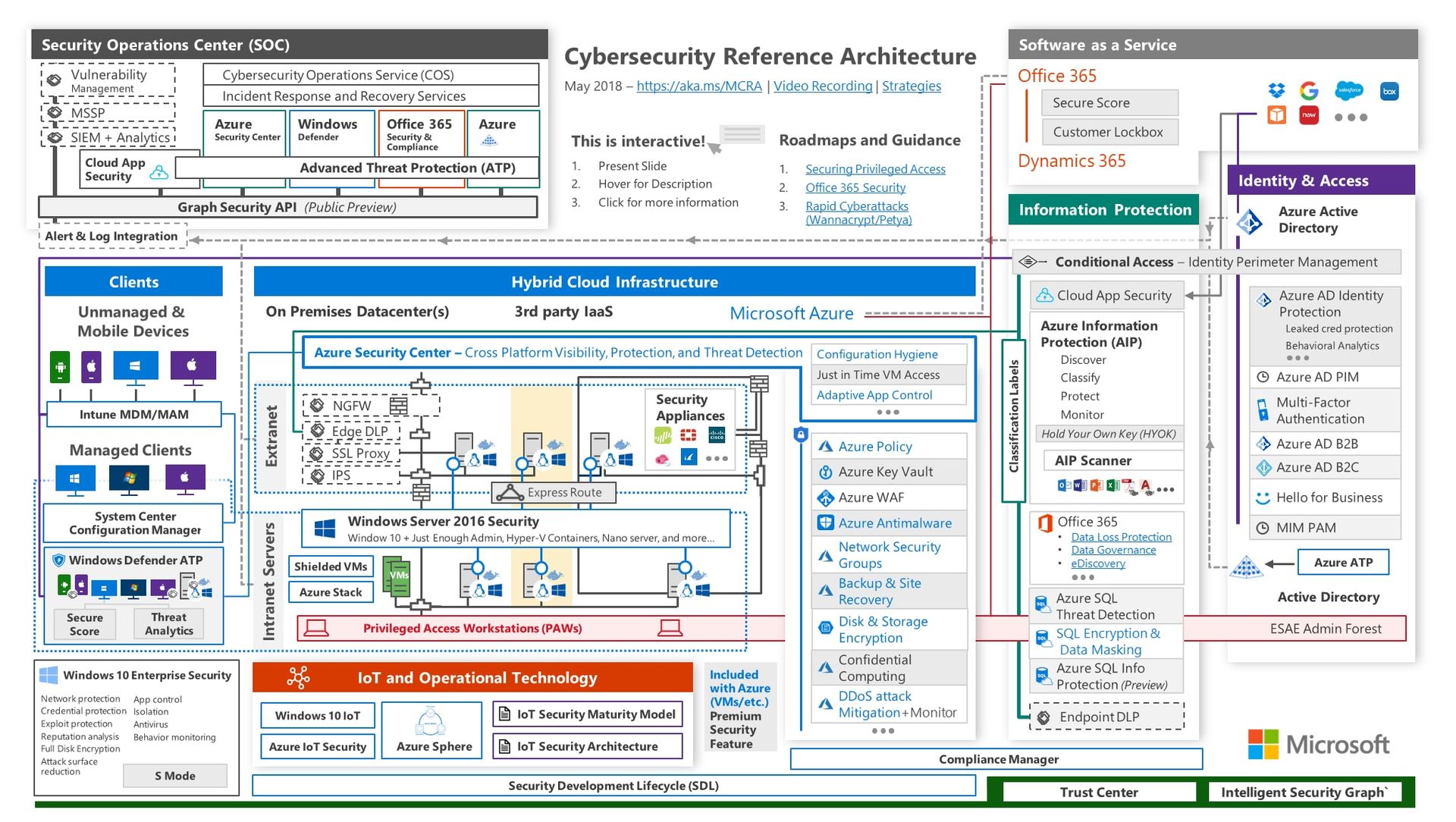
Image title: Microsoft’s cybersecurity reference architecture

Data source: Microsoft — Cybersecurity Reference Architecture: Security for a Hybrid Enterprise
A new tech momentum for real estate?
Just a few years ago, financial organizations such as Morgan Stanley defined real estate as one of the least digitized sectors. More recently, however, this industry has come a long way in implementing the most advanced applications of artificial intelligence and machine learning to improve decision-making, automate processes, and provide better services.
However, real estate companies embracing this technology can face a variety of adoption barriers due to the black-box, data-driven nature of machine learning. To better address such challenges, consider relying on Itransition's experience in ML consulting.
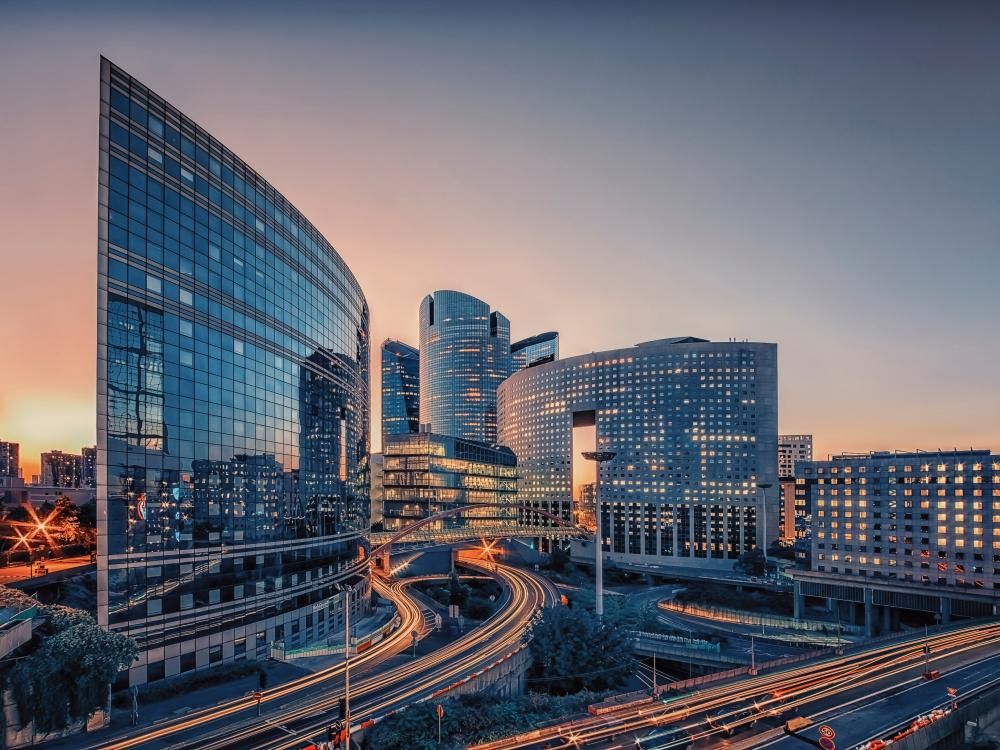
Insights
Predictive analytics in real estate: applications and limitations
Learn from real-life examples how predictive analytics helps real estate professionals make better decisions.
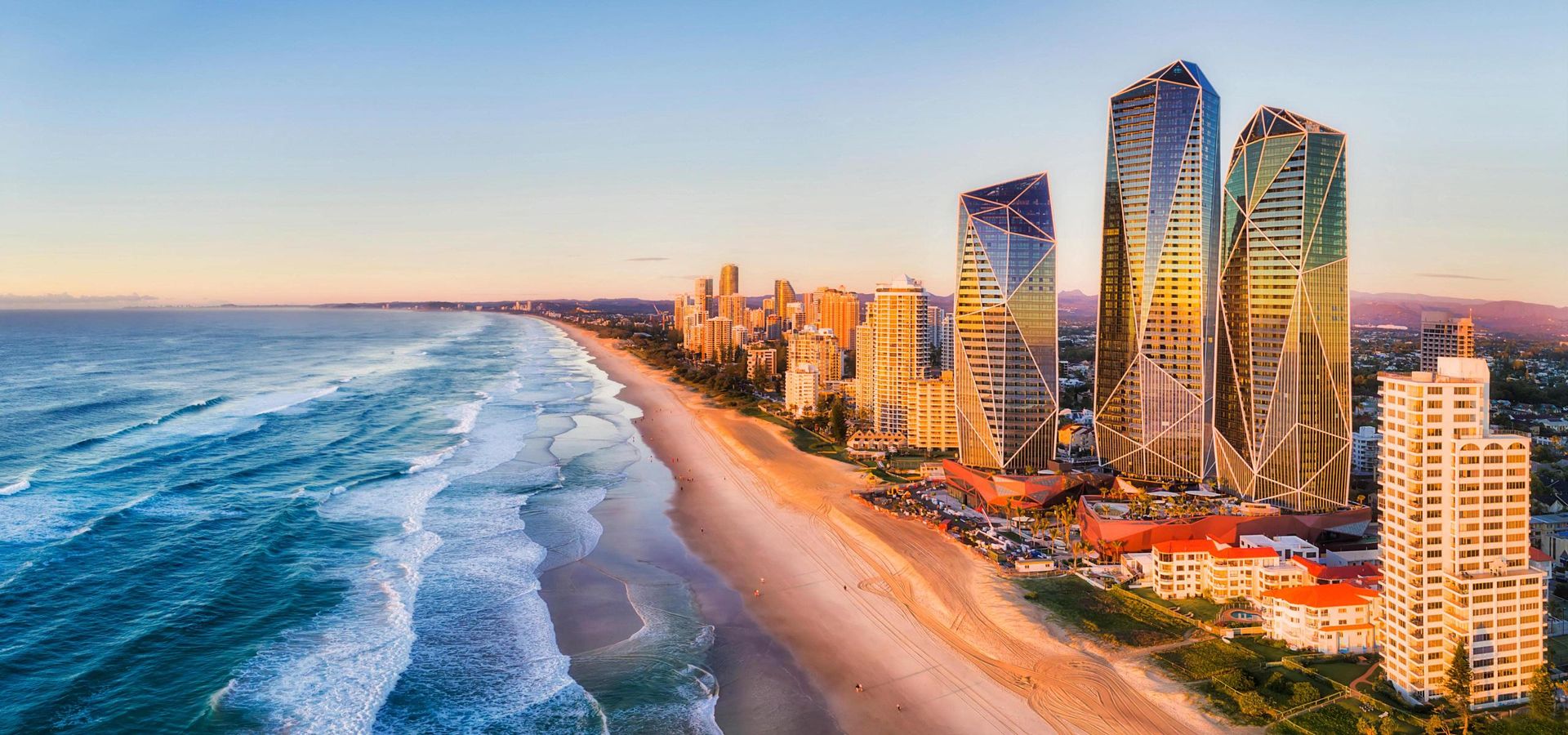
Service
Real estate data analytics
Learn about real estate data analytics use cases, metrics, and development essentials as you explore Itransition's range of data-centric services.
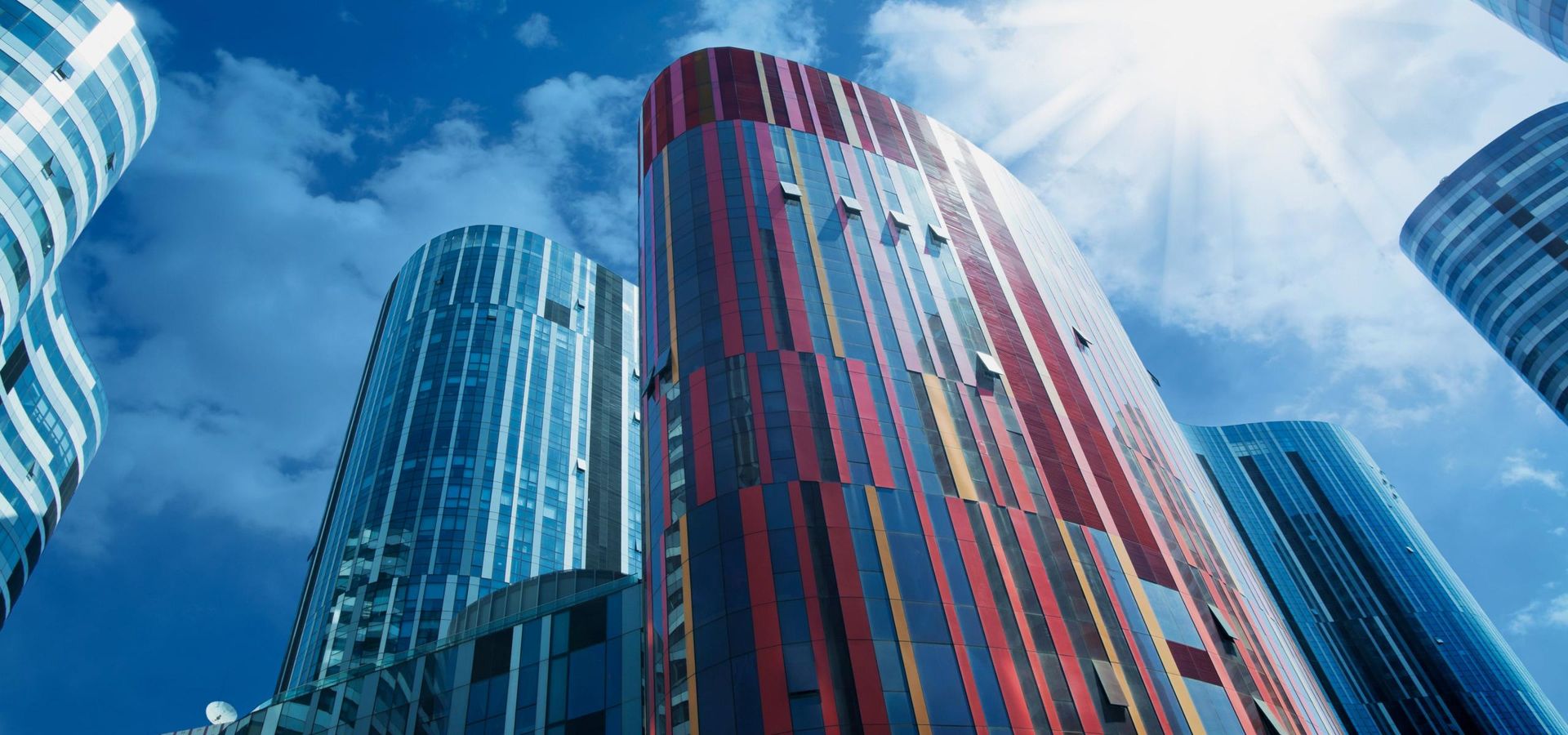
Service
Real estate portals: development services and successful examples
Ready to invest in real estate portal development? Check out portal types, essential features, and the best implementation examples.
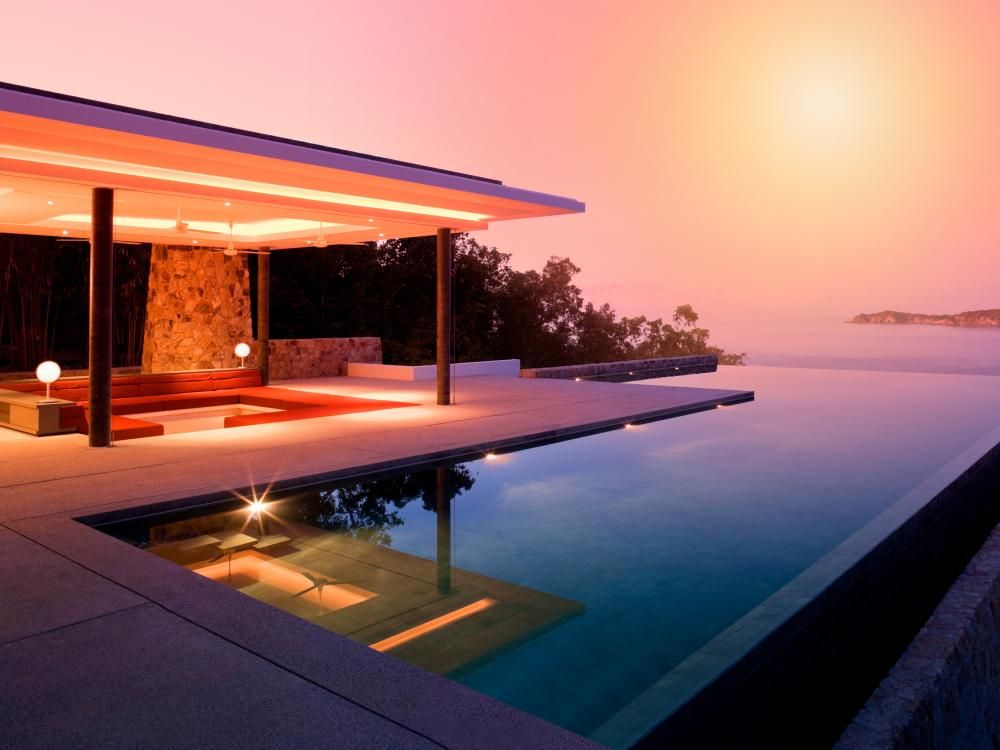
Case study
Real estate sales automation software development
Learn how Itransition built a real estate sales solution with a data autocompletion feature, an admin panel, and a mobile-friendly interface.
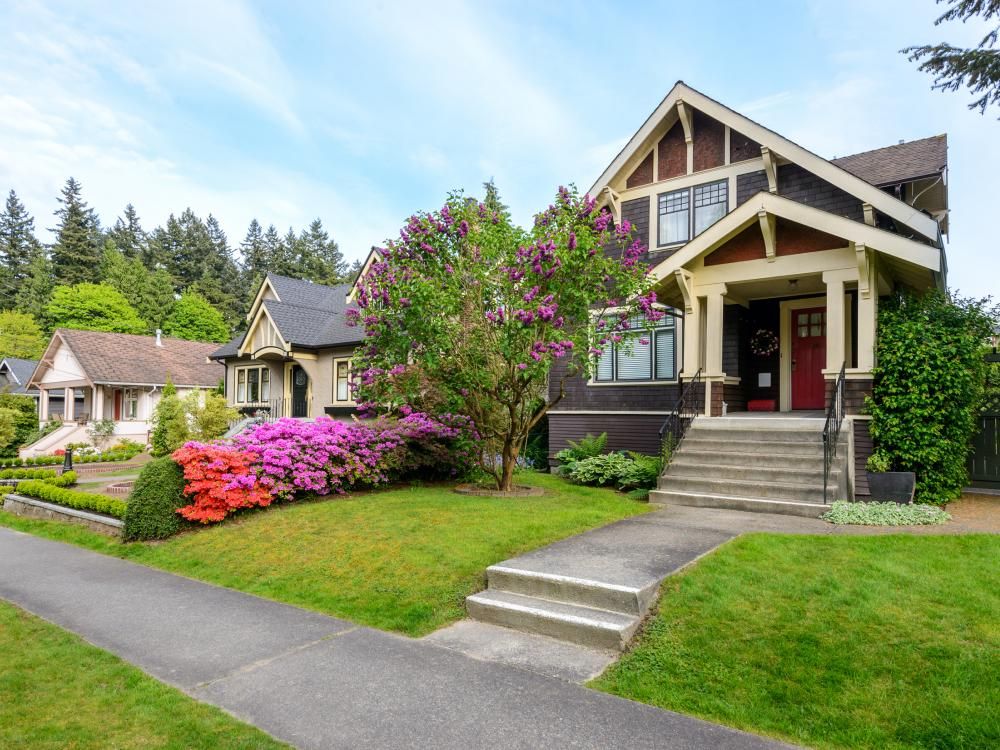
Insights
RPA in real estate: 10 use cases, platforms & best practices
We explore top robotic process automation use cases in real estate, review the best RPA platforms on the market, and examine adoption guidelines and challenges.
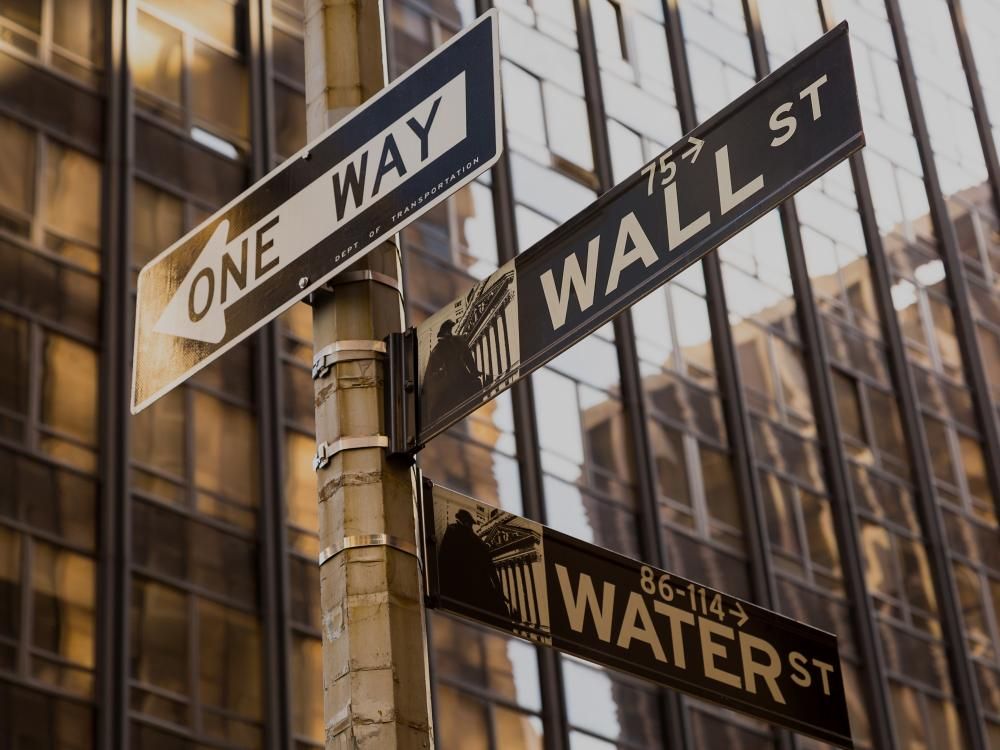
Insights
Machine learning for stock market prediction: a tech overview
Explore the trading opportunities, key algorithms, implementation guidelines, and challenges of machine learning for stock market prediction.