Over the last couple of years, retail and other related sectors, such as manufacturing and logistics, have faced a global pandemic, a massive ship obstructing the Suez Canal, international crises of all sorts, and many other things that, allow us to quote from Blade Runner, "you people wouldn't believe".
Some effects of these events on retail were relatively predictable, including the skyrocketing growth in online sales due to social distancing and a severe supply chain disruption. Others, on the other hand, proved once again the inscrutability of the market and the human mind, such as the toilet paper panic-buying and resulting shortages.
Fortunately, retailers didn't get lost in despair “like tears in rain” (yes, another Blade Runner quote) and called for a solution. Technology's answer to their prayers and, above all, to their investments, was machine learning. Let's find out how with the help of machine learning consultants the retail industry to reinvent itself and find a more optimized way of functioning.
What is machine learning in retail
Machine learning in retail involves the adoption of self-learning computer algorithms designed to process huge datasets, identify relevant metrics, recurring patterns, anomalies, or cause-effect relations among variables, and therefore get a deeper understanding of the dynamics driving this industry and the contexts where retailers operate. The more retail data machine learning systems process, the more they fine-tune their performance as they detect new correlations and better frame the business scenario they're analyzing.
Such capabilities are typically leveraged in two ways. First, machine learning can power augmented analytics solutions that, compared with traditional methods of statistical analysis, will peer way deeper into data, spot even the subtlest interconnections between data points, and better cope with new trends and ever-evolving phenomena.
Second, machine learning's pattern recognition paves the way for relevant developments in the AI field of so-called cognitive technologies, which allow machines to replicate some of the innate skills of human beings. This includes natural language processing systems leveraging machine learning to recognize the linguistic patterns of human communication to understand and mimic them, along with computer vision solutions tapping into algorithms to detect visual patterns and relate them to specific objects.
Business opportunities of machine learning in retail
In practical terms, the above translates into the ability for retailers to implement machine learning and take advantage of it in a variety of business functions and scenarios, which include:
- Market and customer analytics to predict upcoming retail trends such as product demand fluctuations and set up suitable marketing, pricing and restocking strategies.
- A fully personalized shopping experience with recommendation engines, targeted advertising, dynamic pricing, and tailored promotions based on customers’ needs.
- Interactive solutions for digital stores to replicate the typical in-store experience in a virtual scenario through chatbots, virtual assistants, and contextual shopping.
- Machine learning-augmented logistics to streamline product delivery via anticipatory shipping, smart route planning, and self-driving vehicles or drones.
- Retail security measures to protect your assets, staff, and customers with video surveillance and spot signs of fraud via machine learning-based anomaly detection.
These applications of machine learning in retail can be complemented with a full spectrum of additional use cases unlocked by artificial intelligence and all its sub-branches and varying both in business impact and feasibility, as detailed by Gartner in its 2021 AI Use Case Prism for Retail.
The state of the market
Nowadays, most AI-powered retail software solutions rely one way or another on machine learning algorithms' capabilities. As highlighted by Mordor Intelligence in its 2021 Artificial Intelligence in Retail Market report, in fact, machine learning represents one of the major drivers of the global AI market growth in this industry, as it allows market players to provide end-users with a more personalized and interactive purchase experience.
Fortune Business Insight's 2020 study covering the same topic, on the other hand, pointed out machine learning's role in building forecasting models and offering valuable insights into ever-changing customer preferences. This makes machine learning the leading segment of the global AI market in retail, which seems set to grow from $3.75 billion in 2020 to $31.18 billion by 2028.
In this regard, it’s worth noting that the growing implementation of machine learning and related technologies in retail hasn't just been a reactive measure to better address the challenges mentioned in our introduction. Indeed, it has also represented a proactive initiative aimed at seizing the possibilities opened up by artificial intelligence, which ended up catalyzing general dynamics already undergone by the retail sector in previous years, such as the shift from a purely brick-and-mortar model to the coexistence of in-store shopping and ecommerce.
Let's explore these trends by better defining some of the AI use cases that harness the potential of machine learning in retail.
1. Recommendation engines
Since we've just mentioned the rapid transition from in-store to online shopping, we’ll start our roundup from what we may consider the deus ex machina of major ecommerce platforms such as Amazon, namely machine learning-powered recommendation systems.
The role of these powerful engines in digital marketplaces represents the virtual reflection of a human sales assistants' duties in a physical shop, which is to understand what kind of customer we are dealing with and to connect them to the right product. Recommendation systems do so by:
- Segmenting users according to their personal data, including behavioral patterns and previous purchases on the platform, social media comments, browsing history, product reviews, and more.
- Complementing this analysis with product information and contextual parameters such as broader market trends or geographic location.
- Providing users with tailored suggestions matching their customer archetype and subsequently fine-tuning such recommendations based on user feedback, instead of letting them wander endlessly in a sprawling maze of products.
This process, however, can vary depending on the approach taken by each recommendation system:
- Recommendation systems based on collaborative filtering focus on users' perception of products and suggest merchandise already purchased by other customers with similar tastes.
- Recommendation systems adopting content-based filtering mostly consider the product's specific features and will offer items similar to those already bought by the customer in the past.
- Hybrid systems represent the fastest growing segment in the recommendation system market as they combine the mechanisms and pros of both approaches with proper techniques.
Scale up your sales with Itransition's machine learning solutions
2. Targeted marketing
Another tool leveraging the potential of machine learning in ecommerce but easily capable of driving in-store sales as well as targeted advertising. Its underlying mechanism is relatively similar to that behind recommendation systems. A machine learning-based predictive analytics system can gather and process user data from social media or ecommerce platforms to probe relevant metrics and shed light on their correlations. These may include:
- Behavioral factors, such as time on site, bounce rate and conversion rate
- Demographic variables, like age and gender
- Psychographic metrics relating to interests and personality
- Geographic data like user city and region
Based on such parameters and how they interact (such as the recurring relationship between age or cultural interests and favorite brands), the system will forecast customer interests and attitude towards certain products to target specific buyer personas with fully personalized content and ads. An example of successful targeted marketing comes from the American department store chain Macy's, which implemented a predictive analytics solution to fine-tune its email and website marketing campaigns, leading to a 10% increase in online sales in just three months after its introduction.
3. Contextual shopping
The opportunities for marketers unlocked by machine learning in retail don't end with targeted advertising. Another trick to shorten the virtual path connecting customers with the products they are looking for is contextual shopping. This highly interactive software solution, powered by machine learning algorithms and computer vision, can recognize and highlight the merchandise appearing in online content of major social media platforms, allowing users to reach your digital store and purchase the item they want with a simple click.
The video ecommerce platform provider AiBUY, for example, relied on Itransition's machine learning consulting and development services to create a similar tool. Take a look:
4. Chatbots and virtual shopping assistants
Chatbots, as contextual shopping, represent an embodiment of machine learning in retail focusing on interactivity. However, their capabilities come from a different cognitive technology, namely natural language processing, which allows them to assist customers 24/7 in a variety of tasks. This may include sending notifications regarding new collections, helping users find the product they need, suggesting similar items based on recommendation engines’ predictions, and so on.
Such versatile and tireless virtual assistants can be found all around the internet as they've already been deployed on the websites of several brands such as Burberry and Victoria’s Secret. They've also been implemented on social media platforms like Facebook, which launched its Messenger bots in 2016, allowing businesses to partially automate their customer service operations.
5. Dynamic pricing
The clairvoyant capabilities of machine learning are not just about predicting which products customers would like to buy, but also how much they are willing to pay for them. In this regard, one of the techniques that has benefited most from implementing machine learning algorithms is dynamic pricing, which involves, as the name suggests, dynamic adjustments to the price of products based on changing circumstances.
To accurately predict the potential impact of such fluctuations on demand (the so-called price elasticity) and suggest price changes or promotions which may maximize profit while minimizing the risk of customer churn, machine learning systems can assess a wide spectrum of parameters which would be difficult to consider by performing this task manually. For example, they can crawl the web searching for prices of similar products offered by competitors, their hot deals and promotional initiatives in place, and the pricing history of particular products.
Machine learning algorithms can also take into account general market trends, product demand compared to supply, and the items with the lowest price in their respective product category, which typically results in a disproportionate share of demand. All these insights can be incredibly useful for setting a markdown pricing strategy and getting rid of obsolete merchandise at the end of a season.
Rely on Itransition's expertise to get value from your data
6. Demand prediction for inventory management
As we've just pointed out, prices obviously affect product demand and the adoption of machine learning in retail can help us figure out how this relationship works in order to optimize our pricing strategies. But things are more complicated than that, since demand trends on a large scale are driven by a vast range of variables:
- Business initiatives such as the aforementioned pricing decisions, promotions, and the product assortment and display inside a store.
- Purchase patterns relating to weekdays, seasonality, or celebrations (think about chocolate hearts for Valentine's Day).
- External motivators that encourage customers to leave home, like good weather or local events.
Monitoring such factors to predict fluctuations in product demand is an essential component of proper inventory and supply chain management, as it allows retailers to keep stocks replenished. In this regard, Deloitte highlighted that stockouts may cost retailers up to $1 trillion a year in lost sales and reported that a major German sportswear retailer successfully leveraged machine learning algorithms to streamline restocking operations in its online and in-store branches.
To keep track of all these parameters, however, retailers should fuel machine learning-based predictive analytics systems with a constant stream of information coming from multiple data channels, which include "virtual sources" like social media and ecommerce platforms, along with data collected from physical stores. These may include "virtual sources" such as social media, ecommerce platforms, and the integration of voice commerce along with data collected from physical stores.
Besides traditional data sources, it's worth mentioning new, more unconventional ways of harvesting data to enhance inventory management. A striking example comes from Amazon Go, a chain of convenience stores relying on machine learning-powered cameras and computer vision to update product stock data in real time based on the products customers grab off the shelves. Furthermore, these computer vision systems are able to:
- Monitor customers’ behavior, including walking and gazing patterns, to help retailers understand people’s interests and restructure stores accordingly.
- Identify the most popular merchandise for each buyer archetype. For example, if elderly people tend to frequent the store on weekdays rather than weekends, then the products specific to this age group can be promoted during those days.
- Suggest specific marketing actions to store managers. For example, if a particular brand of milk is selling better than the competition, it can be positioned more prominently.
- Turn stores into smart, cashier-free points of sale where fully automated camera-based checkout replaces long queues at the cash desks.
7. Delivery optimization
Products come and go and optimizing how they leave your store is as important as perfecting their replenishment. Machine learning-powered route planning represents a major use case of AI in transportation as it leverages sensors, cameras and other devices interconnected through the Internet of things to collect real-time weather and traffic data and calculate the fastest route for deliveries.
Anheuser-Busch, for example, adopted machine learning for route optimization in a pilot program covering two American cities and aimed at speeding up daily deliveries. The algorithms considered factors such as weather conditions and the driver’s experience to suggest the best delivery time for each consumer. After a few months of running the pilot program, Anheuser-Busch reviewed the success metrics, including driver satisfaction and working hours, and decided to extend this navigation approach to all of its US wholesalers.
But what if we could reduce delivery times by sending products even before customers hit the "buy" button? This is the approach adopted by Amazon, which implemented an "anticipatory shipping" system based on machine learning and able to predict what its users are likely to order in the future based on their previous purchases, wishlist, and other parameters. This solution enables Amazon to send merchandise to a closer hub while waiting for the customer to actually order it and then dispatch it via standard shipping, which is significantly less expensive than same-day delivery.
8. Self-driving vehicles
Optimizing delivery routes doesn't change the fact that drivers still get tired and can't operate a vehicle 24 hours a day. Robots, instead, don't need a nap and can work around the clock. That's the idea behind deploying self-driving vehicles powered by machine learning and computer vision for logistics and retail.
Different retailers are already experimenting with self-driving vehicles for their last-mile deliveries. Since 2019, Amazon has been testing Amazon Scout, a self-driving electric device the size of a cooler. Amazon started with six of these devices delivering packages on weekdays in daylight and operating under human supervision to ensure they safely navigated around pets and pedestrians.
Kroger, on the other hand, partnered with Google-backed robotics corporation Nuro to deliver groceries in self-driving vehicles seven days a week. The company just recently launched the 3rd-generation of its flagship model, designed to double the cargo volume and handle more deliveries.
However, this is only the beginning, as autonomous vehicles may soon take to the skies. Once again, Amazon is one of the pioneering operators in this field, as in the future it may be able to deliver packages in just 30 minutes with its "Prime Air" drone-based shipping system. On the other hand, the project is still far from gaining full momentum, as several incidents occurred over the course of a four-month test period, as reported by Bloomberg.
Get your custom machine learning solution from Itransition
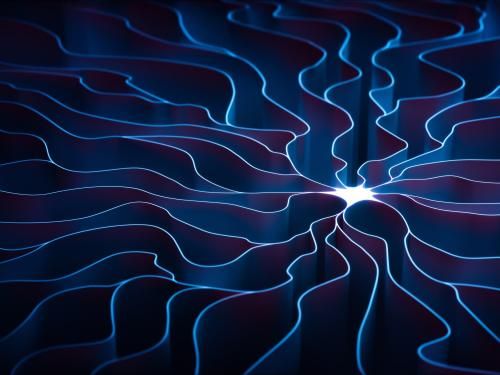
Tailored ML advisory
We offer machine learning consulting, modeling and implementation services, helping retailers with different business goals, needs, and budgets apply this innovative technology to their advantage.
9. Video surveillance
Having a Big Brother watching you can be the lesser evil when it comes to ensuring a safe retail environment. Video surveillance solutions harnessing the capabilities of machine learning and computer visions for retail can spot any other suspicious behavioral patterns which are common among thieves, for example, by detecting if someone picked an item and hid it in their pocket or backpack.
If retailers expect to reliably identify theft with computer vision, however, it will take more than "just" high-resolution cameras and algorithms, since they'll also need a huge amount of labeled examples of visual data representing distinct patterns of theft behavior. For example, the Japanese technology startup Vaak developed AI software designed to catch shoplifters in action and alert store managers. The CEO of Vaak admitted to training machine learning algorithms on 100,000 hours of video data depicting over 100 behavioral aspects, including hand movements, facial expressions, walking patterns, etc.
10. Fraud detection
In a world driven by technological progress and complex socioeconomic dynamics towards full digitalization, products disappearing from the shelves are neither the only nor the worst threat to retailers and their customers. As pointed out by the 2020 Nilson report, electronic payment fraud is a growing phenomenon affecting several industries and generating global losses of $28.65 billion in 2019 alone.
That's why many corporations, especially those operating in ecommerce, rely on machine learning for fraud detection. Indeed, algorithms can easily spot suspicious account operations breaking standard behavioral patterns, such as a spike in transaction frequency or money transfers with other high-risk accounts.
Machine learning systems also represent a powerful weapon to combat identity theft by monitoring users' purchase habits and transaction data. Furthermore, they can implement computer vision features to scan personal documents and verify user identity via face recognition and biometrics.
Lots of enthusiasm and a pinch of caution
AI and machine learning are swiftly invading the retail space, opening new opportunities for retailers to differentiate themselves from their competitors, including:
- Retaining current customers and acquiring new ones thanks to personalization
- Saving time and human effort by optimizing delivery routes
- Saving money through effective inventory management and theft prevention
- Gaining a reputation as an innovative and committed retailer who values its customers and goes the extra mile to please them
However, as amazing as it is, machine learning is still "just" a tool, and using it wisely is up to us. As highlighted by McKinsey in its 2021 The dos and don’ts of dynamic pricing in retail article, during the pandemic, several retailers massively hiked cleaning products' prices due to an apparent demand spike monitored by dynamic-pricing algorithms. The result was a significant backslash from customers which perceived their decision as an opportunistic move.
The first lesson we can draw from this episode is that people have an uncontrollable desire to clean their apartment when social distancing measures keep them stuck at home. The second, and most important one, is that proper human supervision of machine learning-driven strategies, at least for now, may be a necessity rather than a choice and should be performed with reason in mind.