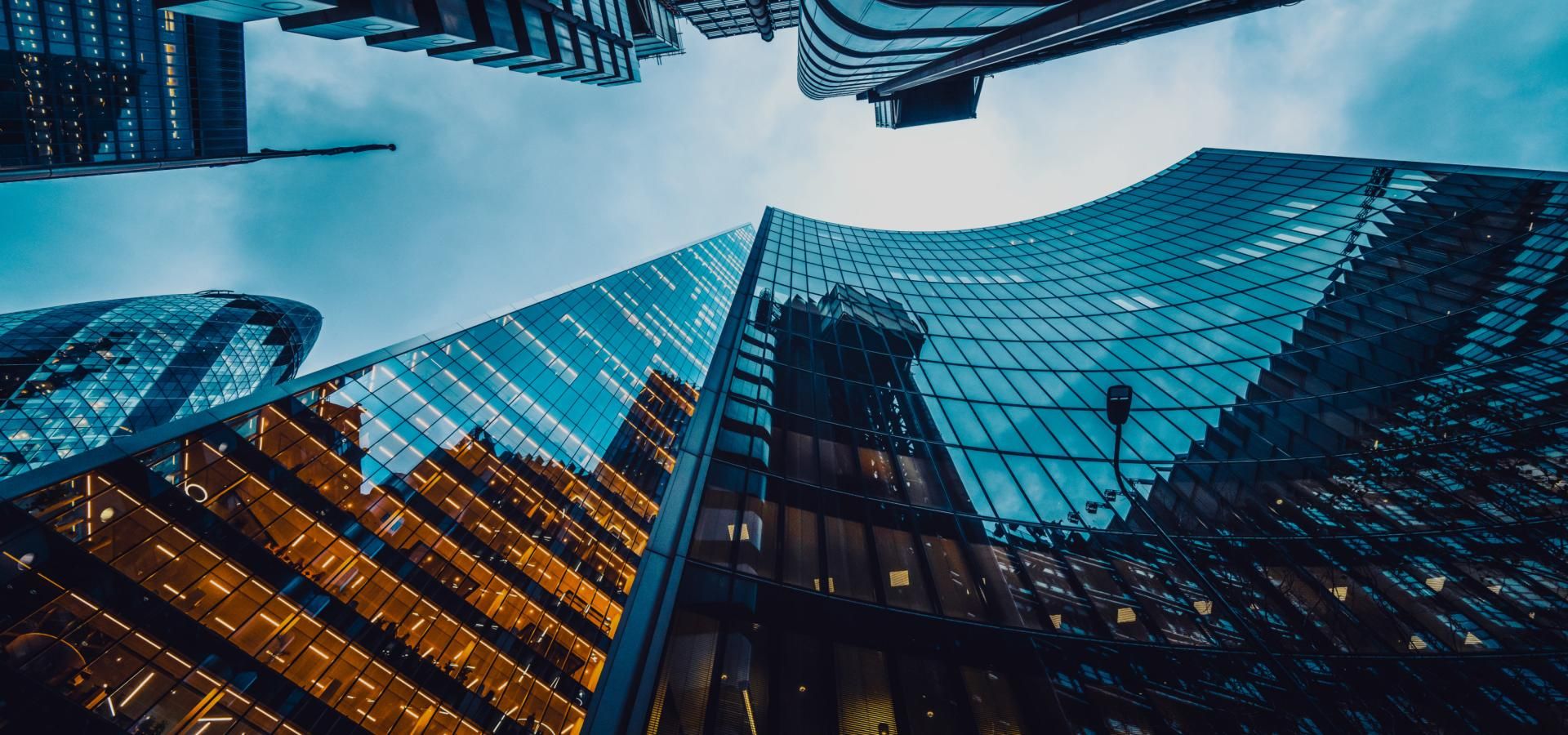
Predictive analytics in finance: use cases, platforms & adoption guidelines
November 12, 2024
- Home
- Predictive analytics
- Predictive analytics in finance
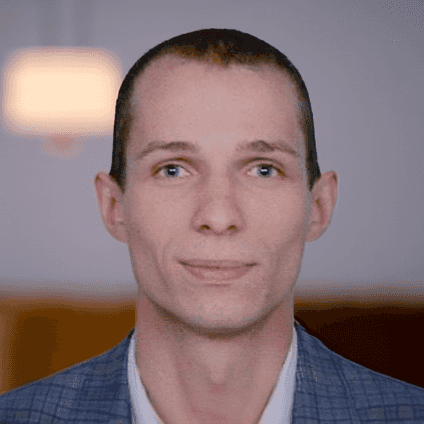
Head of AI/ML Center of Excellence
With holistic expertise in AI, predictive analytics consultants from Itransition can help you build and implement advanced software solutions to predict future financial outcomes and make more informed decisions for your business.
Table of contents
Financial data analytics market trends
the estimated size of the financial analytics market in 2024
Mordor Intelligence
AI and analytics’ potential annual value in the global banking industry
McKinsey
of CFOs plan to invest in AI and predictive analytics accounting tools by 2025
Roland Berger
Key predictive analytics use cases in finance
Brokerages, hedge funds, and other firms use AI-powered wealth management tools, stock trading software and financial analytics platforms featuring predictive capabilities to optimize their trades and portfolio investments. Powered by machine learning decision trees based algorithms and neural networks, these tools can process financial data in real time to predict changes in stock prices and other market trends based on various economic indicators. This enables investment and trading companies to identify the most promising stocks, bonds, and commodities and build more balanced portfolios. Financial firms can also combine algorithmic trading with predictive analytics for smarter trade order automation.
Budgeting & accounting
Â
provide finance teams with capabilities like sales and expense analysis and cash flow forecasting for more accurate financial planning and resource allocation. For instance, these solutions can monitor the cash conversion cycle, internal rate of return by region, and other indicators to provide accountants with detailed reports on the company’s future financial performance and recommend budgeting strategies to achieve specific financial goals.
Marketing & sales personalization
Predictive analytics is changing the way financial organizations and, more specifically, their marketing and sales teams engage with prospects or clients, enabling a hyper-personalized customer experience. This means, for instance, helping monitor customer behavior on social media and other digital channels to identify their needs and interests, segment them accordingly, and target them with tailored financial product offers and recommendations.
Credit scoring
Mortgages, credit cards, and other types of loans always involve some default risk. Predictive analytics solutions can mitigate it by calculating clients’ creditworthiness based on their credit inquiries, available liquidity, disposable income, tax returns, payment history, and other factors and suggesting high-value solvent customers. These tools can also complement individual customer assessments with data on current market conditions and competitor offerings to help banks and mortgage companies estimate an appropriate credit limit and interest rate.
Fraud detection & prevention
Preventing fraud is one of the main goals of artificial intelligence implementation for financial organizations. Indeed, predictive anomaly detection systems powered by machine learning algorithms can spot suspicious trading patterns or transactions to help prevent market manipulation and money laundering. Furthermore, ML-based fraud detection solutions can identify anomalous credit card account behaviors to mitigate the risk of payment fraud.
Turn data into valuable insights with Itransition’s predictive analytics solutions
Real-life examples of predictive analytics in finance
A major financial institution partnered with PwC to incorporate a predictive analytics tool into their budget forecasting framework. The solution helped the client forecast the impact of internal or external events on cash flows, from high-level economic trends to individual disbursements. As a result, the client was able to extend the forecast period from 3 to 12 months, free up employee time for value-added activities, and make more accurate budget decisions.
One of the largest banks in the United States implemented a fraud detection engine with predictive capabilities from DataVisor to accurately assess the likelihood of fraud across a range of transactions and other operations, from card purchases to loan applications. After deployment, DataVisor’s solution improved successful interceptions of fraud attempts in online loan applications by 30% and achieved a false-positive rate of just 1.3%.
Carbon, an African digital bank, chose DataRobot’s cloud-based AI platform to automatically assess customers’ credit risk. The ML-based system collects data from first-, second-, and third-party sources to create a credit score, allowing customers with higher scores to access better rates. The solution also estimates default risk for each customer, which is then used to adjust loan terms. Carbon reported that their team would need 25% more members to perform the same tasks manually.
A multinational bank adopted Teradata's cloud data analytics platform to foster customer acquisition and engagement. The bank can now identify potential clients with a high level of interest in their services prior to starting an application based on their web page visit durations and other website KPIs. It can also target high-value prospects with personalized messages based on past transactions and interactions across digital channels. As a result, the institution increased the click-through rate of their personalized messages by 50 times.
Best predictive analytics platforms for finance
Tableau is a popular BI, analytics, and visualization tool by Salesforce providing self-service data preparation, guided ML model building, and other user-friendly features for seamless analysis. The platform also offers predictive analytics capabilities powered by linear regression algorithms to help forecast multiple financial scenarios, from sales trends to stock market price fluctuations.
Pricing
- Free trial
- Multiple plans, price upon request
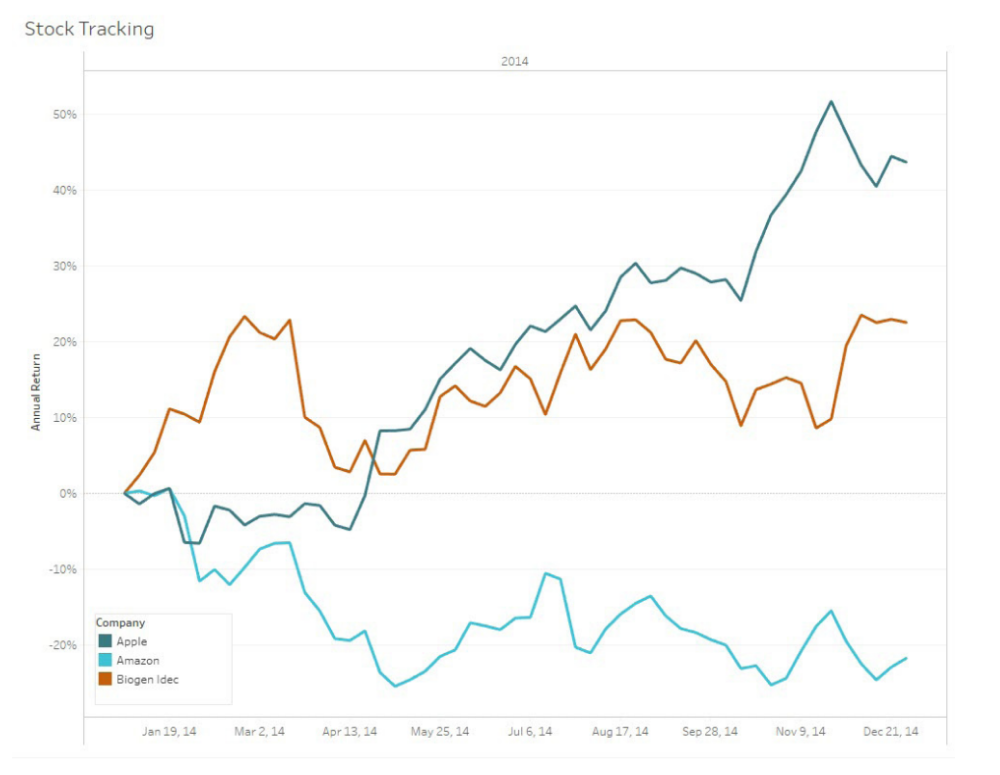
Image title: Tableau’s feature for time series analysis of stock prices
Image source: tableau.com — Advanced Analytics with Tableau
Zoho Analytics is a self-service BI and analytics platform powered by forecasting algorithms and natively integrated with the Zoho Finance suite of business apps. The solution enables companies to analyze their financial and accounting data, predict business performance indicators like sales and revenues, and visualize these insights through interactive dashboards.
Pricing
- 30-day free trial
- Multiple plans starting at $24/month, billed annually
- Free basic plan for the on-premises version
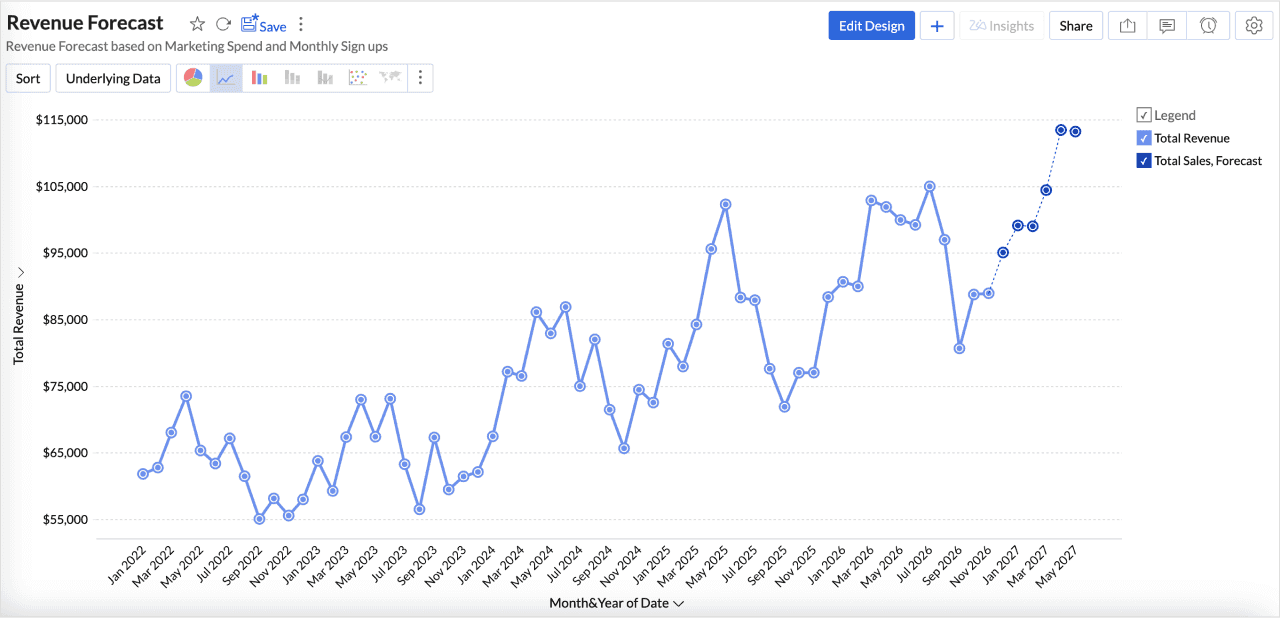
Image title: Zoho’s revenue forecast dashboard
Image source: zoho.com — Forecasting
Qlik Sense is an augmented analytics platform featuring AI-assisted data preparation, natural language querying, and predictive analytics capabilities. This advanced solution enables decision-makers to generate forecasts and run simulations via what-if scenarios, facilitating financial planning, revenue and profitability management, and expense management.
Pricing
- Free trial
- Three plans starting at $825/month
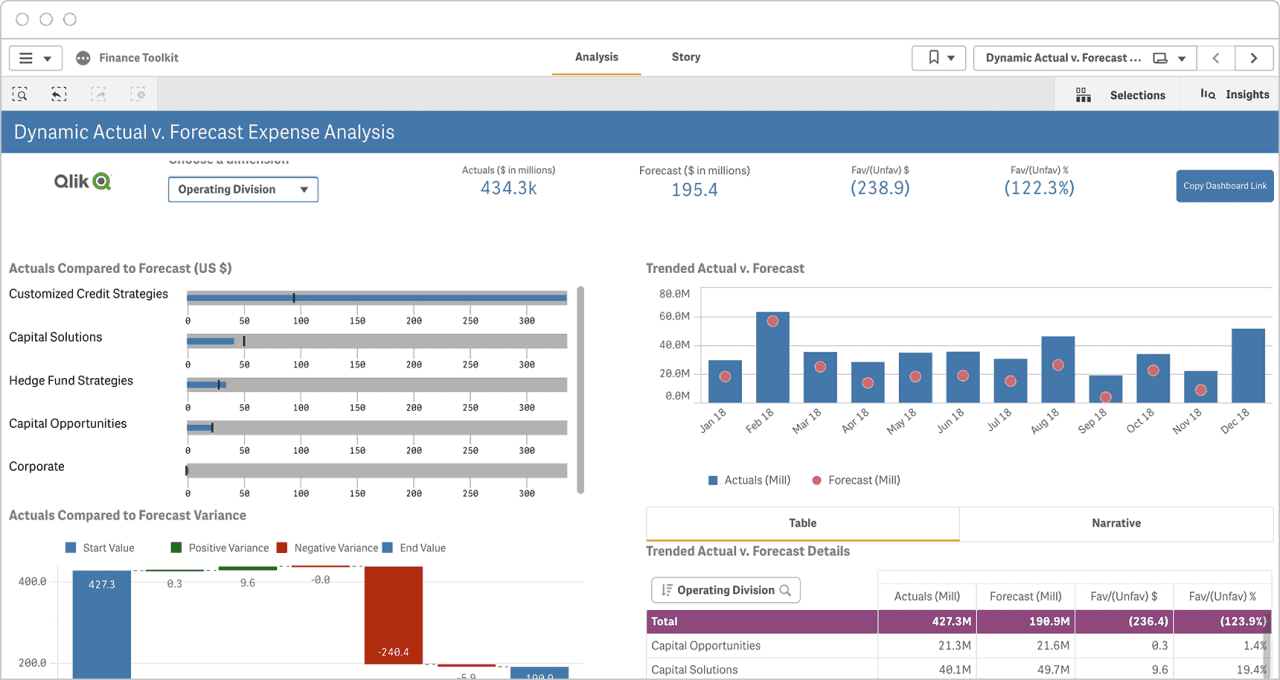
Image title: Qlik’s expense forecasting tool
Image source: qlik.com — Financial Analytics
TradingView is a financial data analytics and social media platform for traders and investors, available as both a desktop and mobile app. Users can access an extensive set of functions provided by the platform or developed by its community to predict market trends based on metrics like opening and closing prices or trading volumes.
Pricing
- Free basic plan
- Five paid plans starting at $12.95/month
- 30-day free trial for certain plans
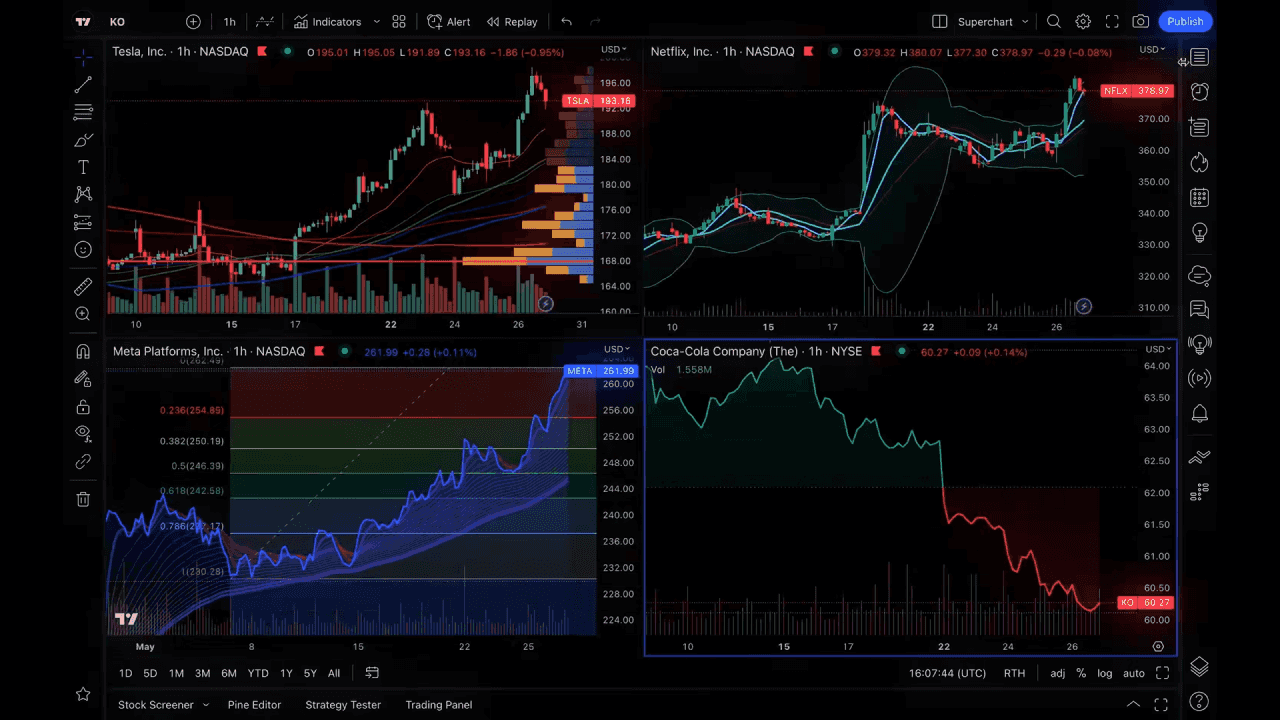
Image title: TradingView’s market data dashboard
Image source: tradingview.com — Features
Top 5 types of models used in finance
Financial institutions rely on different types of ML and statistical models to make predictions. These can be classified based on the task they perform and how they produce a certain output.
Classification models
Classification models divide data points into two or multiple categories (binary and multiclass classification, respectively) based on their features and can be used to forecast future outcomes. In finance, for example, they can help predict whether a certain company's stock will go up or down.
Time series models
Time series models track a certain variable throughout a specific time period to predict how that variable will be affected in another interval of time. For instance, financial institutions can use time series models to predict how a given metric, such as securities prices or inflation rate, will change over time.
Anomaly detection models
These models identify significant outliers in a data set to predict unexpected events, which makes them popular for fraud detection. For example, if a credit card user purchases a luxury watch in a country where they don’t live, an anomaly detection model will flag the transaction as potentially fraudulent since this behavior deviates from the holder's typical buying patterns.
Clustering models
Clustering models group data points according to their shared features and differences. For instance, they can be a powerful tool for clustering similar customers into segments based on their purchasing or investment patterns and predicting which financial products and services would meet their preferences.
Regression models
These models identify correlations between dependent and independent variables to make predictions based on historical data. For instance, regression analysis can help estimate the returns for stocks based on the market risk premium or the revenues of a business according to the number of salespeople employed.
Benefits of predictive analytics adoption in finance
Increased revenues
Predictive models help financial firms make data-driven trading and investment decisions to maximize profits.
Easier financial planning
Predictive analytics enable companies to forecast cash flows and costs for more accurate budgeting.
Risk mitigation
Predictive analytics-powered capabilities like credit scoring and fraud detection ensure more effective risk management.
Superior customer experience
Organizations can analyze clients’ data to deliver personalized financial services and thus improve customer satisfaction and retention and minimize churn.
Predictive analytics challenges & guidelines
Concerns
Recommendations
Data quality & availability
Like any other data-driven technology, predictive analytics solutions deliver accurate insights and forecasts only when fueled with large high-quality data sets. These may encompass different types of data from multiple systems and external sources.
Like any other data-driven technology, predictive analytics solutions deliver accurate insights and forecasts only when fueled with large high-quality data sets. These may encompass different types of data from multiple systems and external sources.
Integrate heterogeneous data from selected sources (market data providers, credit rating agencies, etc.) via ETL/ELT pipelines and consolidate them into suitable repositories, such as data lakes for cost-effective storage of structured and unstructured data or data warehouses for quick access to cleansed data for analysis. At the same time, connect your predictive analytics solution to other systems and external services directly via APIs to enable seamless data exchange or via middleware architectures like ESB if they use different communication protocols that must be converted. Cloud data integration services can facilitate both tasks.
Model training & performance
ML systems require huge computing resources to process big data sets or streams of real-time financial data for predictive analytics model training. The same applies to the trained model processing real data for financial forecasting. This requires a complex and financially demanding technology infrastructure. Additionally, the model can perform poorly when overtrained on a certain data set (overfitting) and its performance can degrade over time due to progressive changes in input variables (model drift).
ML systems require huge computing resources to process big data sets or streams of real-time financial data for predictive analytics model training. The same applies to the trained model processing real data for financial forecasting. This requires a complex and financially demanding technology infrastructure. Additionally, the model can perform poorly when overtrained on a certain data set (overfitting) and its performance can degrade over time due to progressive changes in input variables (model drift).
Consider using cloud-based ML services like Amazon SageMaker or Azure Machine Learning to get access to scalable computing resources, as well as out-of-the-box algorithms and pre-trained AI models. As for model performance issues, you can mitigate overfitting by splitting model training data into training, validation, and test sets and cross-validate the outputs. At the same time, a common practice to address model drift involves performing multiple post-deployment retraining iterations to fine-tune its output.
Security & compliance
Financial companies operate in a highly regulated market with strict data protection standards and legislation. Furthermore, financial data is sensitive information that can easily become a target for fraudsters and cybercriminals, resulting in breaches and leaks.
Financial companies operate in a highly regulated market with strict data protection standards and legislation. Furthermore, financial data is sensitive information that can easily become a target for fraudsters and cybercriminals, resulting in breaches and leaks.
Use obfuscated data, namely data sets anonymized via data masking techniques, to train your model. Additionally, make sure to design and utilize your predictive analytics solution in full compliance with applicable data management and security regulations, such as GDPR and PCI-DSS. This includes protecting the solution with security measures like data exchange encryption, identity and access management, and multi-factor authentication.
Our predictive analytics services
.jpg)
Our consultants can guide your organization throughout the predictive analytics software implementation process, assisting with business analysis, data audit, solution conceptualization, project planning and supervision, and user adoption.
Implementation
We help you implement predictive analytics solutions tailored to your business needs, taking care of architecture design, custom solution development or platform customization, integration, testing, and deployment to the target environment.
Support & modernization
Our specialists provide user training to facilitate predictive analytics solution adoption, perform ongoing maintenance to ensure your solution operates seamlessly, and enhance it via functional improvements or other upgrades to align it with emerging business needs and tech trends.
Leverage predictive analytics with Itransition’s guidance
Navigating volatile markets with predictive analytics
While predictive analytics systems can't see the future, they often highlight the correlations between certain variables or trends. This allows financial institutions and other organizations to better understand how past or current conditions may lead to future events and use these insights to address market uncertainty and refine their financial decisions.
Consider teaming up with an experienced IT partner like Itransition to take full advantage of predictive analytics and other advanced technologies and achieve better business outcomes.
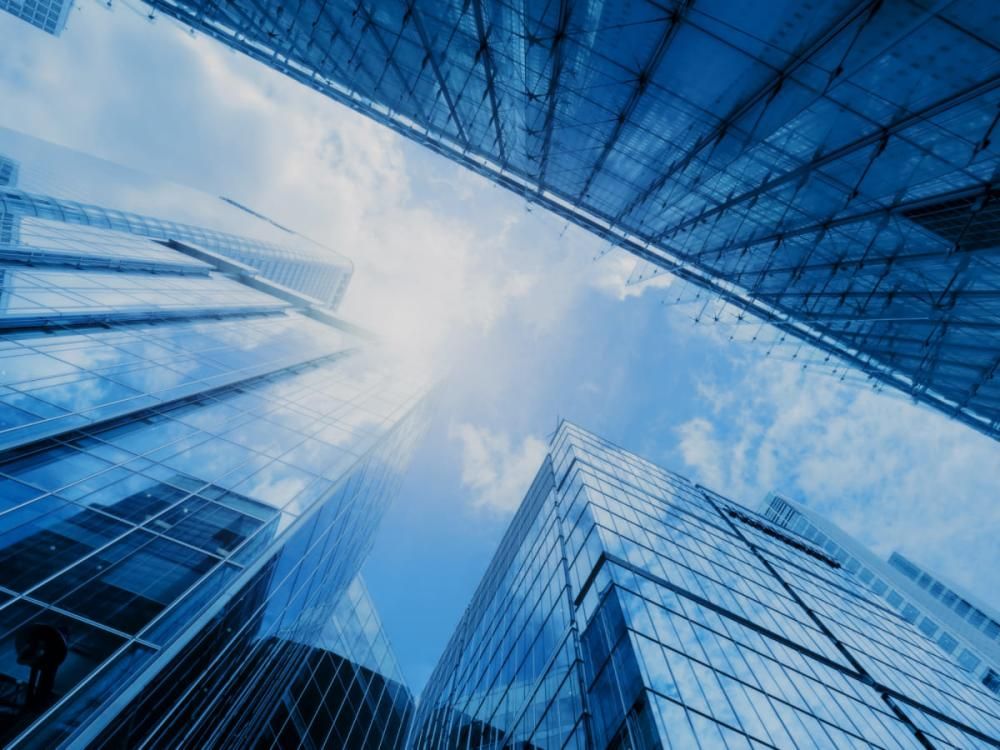
Insights
AI in fintech: use cases,
 solutions & implementation challenges
Explore the top use cases, real-life examples, and branches of AI in fintech, along with common adoption challenges and guidelines to address them.
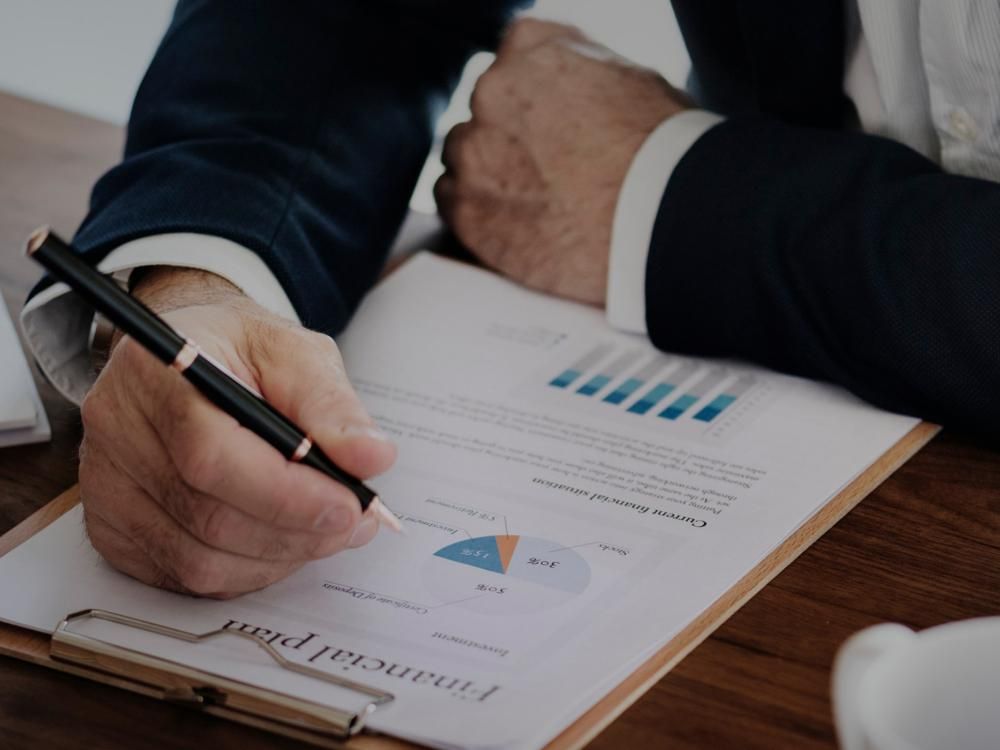
Case study
BI consulting and engineering for a commercial bank
Find out about Itransition's high-profile BI consulting for a Canadian bank, including data architecture analysis and a BI strategy.
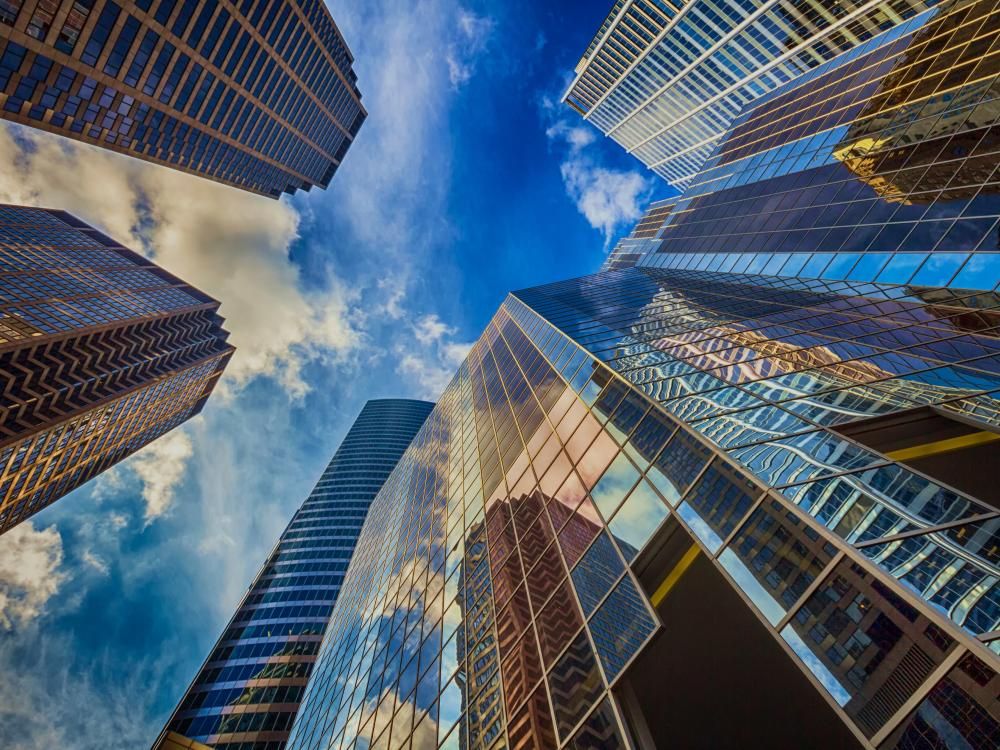
Insights
Business intelligence for finance: capabilities, tools & integrations
Explore how business intelligence and data analytics benefit the financial services sector and learn what capabilities, integrations, and tools make up a successful BI strategy.
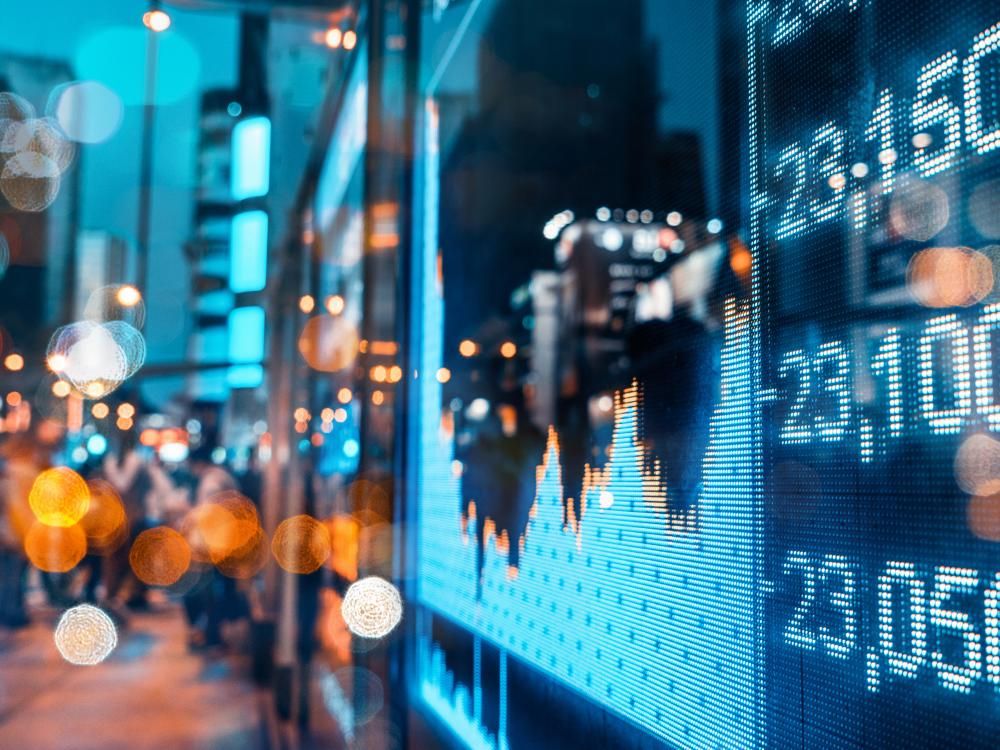
Case study
Dedicated team for investment portfolio management ecosystem
Learn how we developed a suite of investment portfolio management tools and custom algorithms now used by thousands of investors.
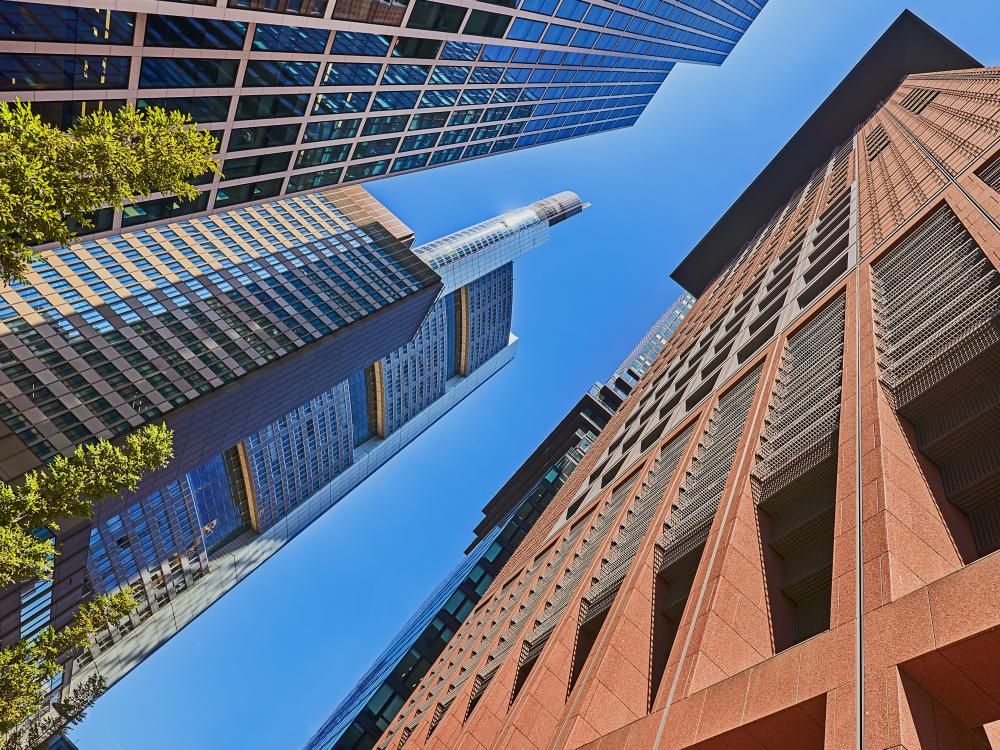
Insights
Machine learning in banking: 8 use cases and implementation guidelines
Learn how banks can apply machine learning to improve customer engagement, streamline compliance, and drive more revenue.
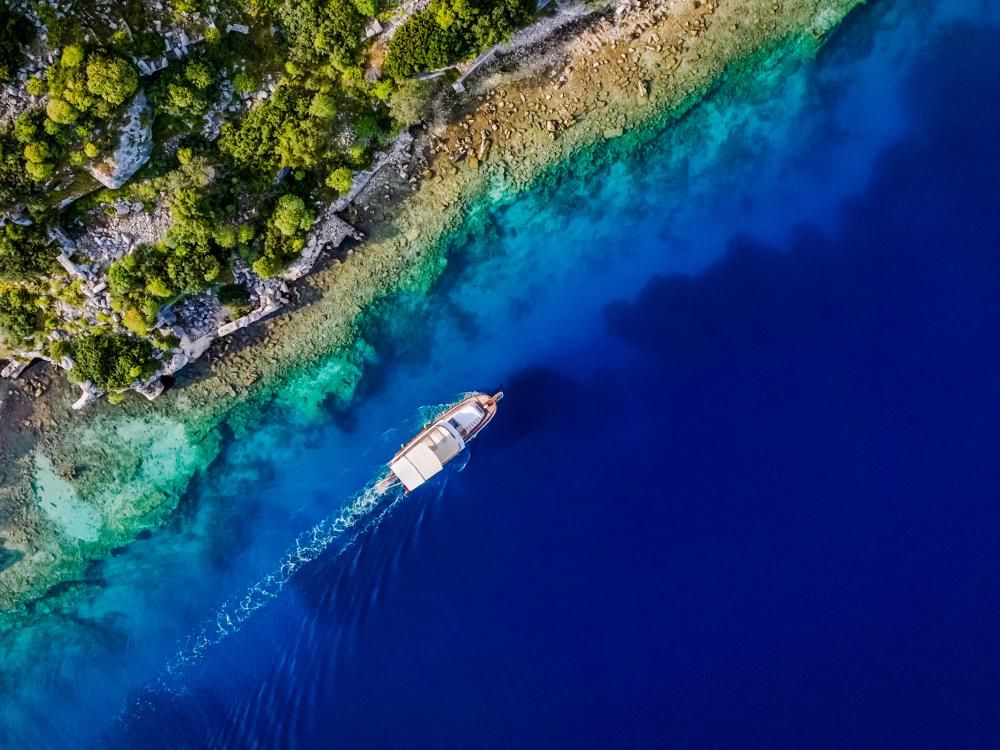
Insights
Wealth management software
Learn how wealth management software facilitates the provision of financial advice, improves asset management, and creates best-in-class investor experiences.