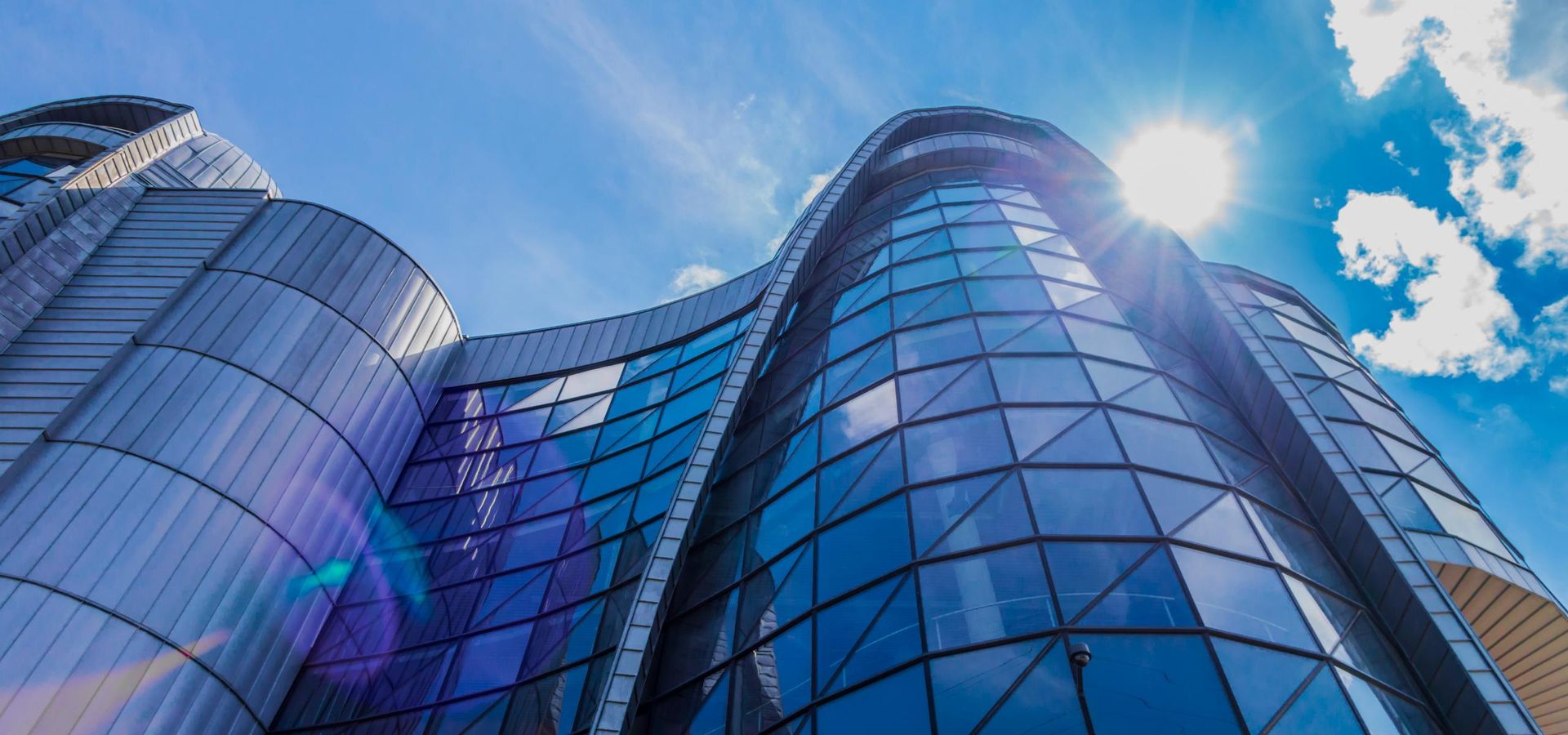
Top machine learning use cases and industry applications
June 9, 2023
- Home
- Machine learning
- Machine learning use cases
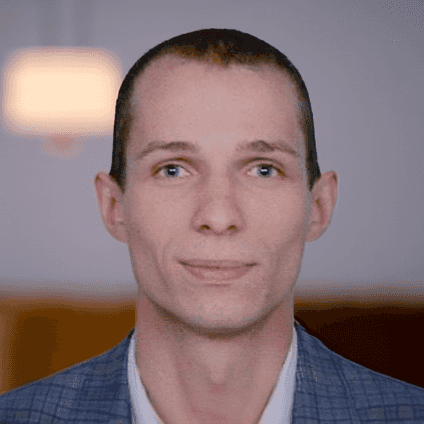
Head of AI/ML Center of Excellence
ML technologies are fundamentally transforming the way businesses work. With the advancement of artificial intelligence and ML algorithms, computers can process big data sets quickly and efficiently, enabling organizations to process large amounts of information faster. In addition, the advancements in machine learning solutions and expertise have allowed for more accurate predictions of customer behavior and market trends. So it is only natural that the global ML spending is expected to grow from $21.17 billion in 2022 to $209.91 billion by 2029, at a CAGR of 38.8%, according to Fortune Business Insights.
The applications of machine learning have been constantly expanding. Сompanies from healthcare, retail, finance, and other industries turn to machine learning development services to unlock new insights and improve their operational efficiency. With so much potential and opportunities to leverage ML, let’s explore some of the most common examples of its use cases and applications across industries.
Scheme title: Worldwide AI software by category value, 2020-2021
Data source: idc.com — Artificial Intelligence Spending Grew 20.7% Worldwide in 2021, According to IDC
Table of contents
Key ML applications
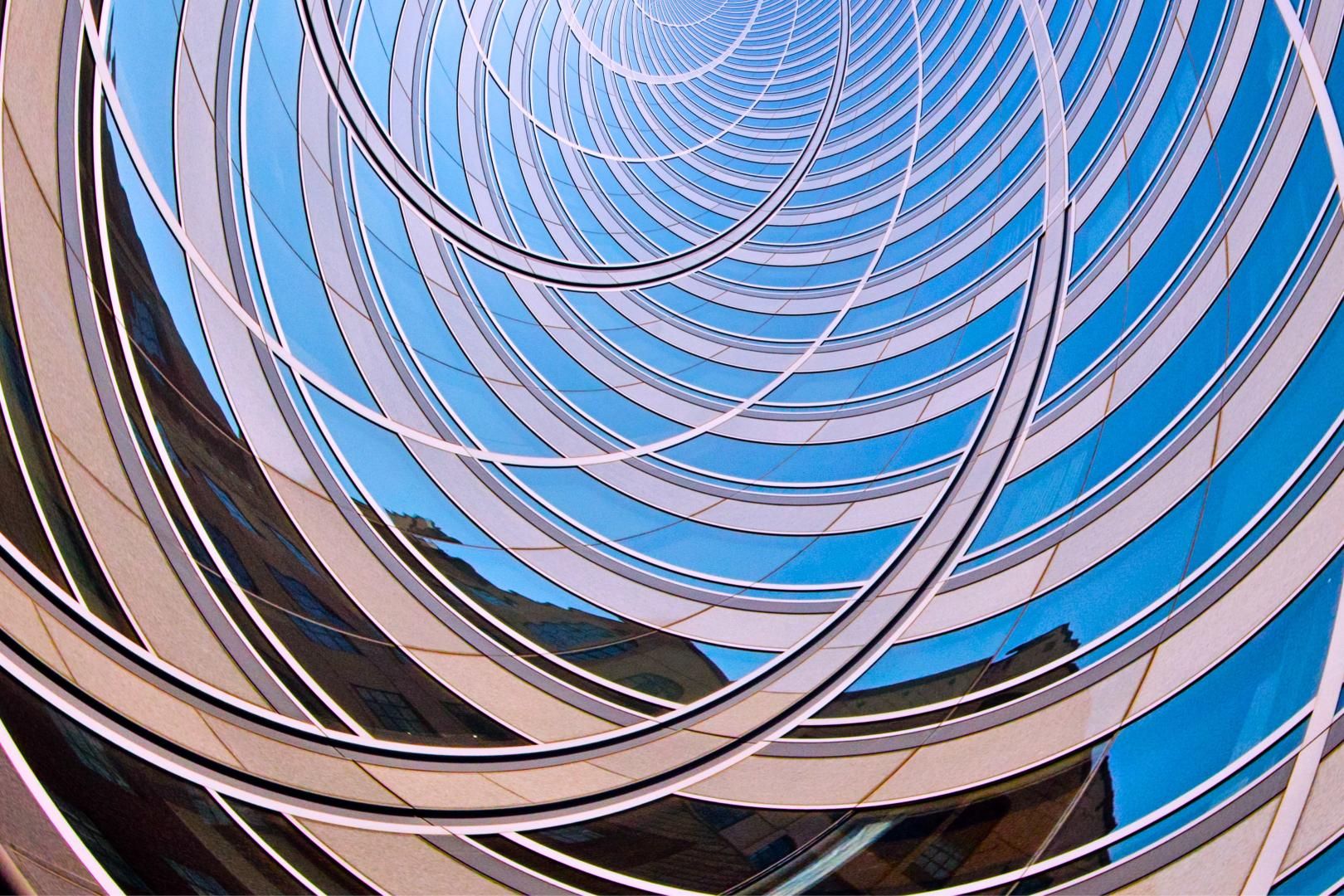
Computer vision is a subfield of ML that focuses on enabling computers to understand the content of digital images, videos, and other visual data. Computer vision use cases include facial recognition, object detection/classification, image recognition and segmentation, etc. and is relied upon across retail, healthcare, cybersecurity, and other sectors.
- Automated visual inspection and defect detection in manufacturing
- Biometric authentication for security
- MRI analysis for the detection of tumors, internal bleeding, and other diseases
- Social distancing tracking
- Real-time traffic monitoring
- Object detection for autonomous vehicles and robotics
NLP
Natural Language Processing (NLP) is a subset of machine learning that allows computers to comprehend and interpret spoken and written human language. It is increasingly implemented in various sectors, including customer service, healthcare, and marketing automation, for tasks such as sentiment analysis, speech recognition, text classification, document summarization, and more.
- Sentiment analysis of customer feedback, reviews, and social media posts
- Text translation
- Generation of product information for ecommerce
- Speech to text, text to speech
- Automatic extraction of relevant information from text
- Chatbot and voice assistants for customer support
- Meeting notes generation
Data mining
Data mining uncovers patterns and discovers correlations, trends, and outliers in large data sets. Data mining use cases can be seen in various industries such as finance, healthcare, and retail for tasks like fraud detection, medical diagnostics, customer segmentation, and more.
- Financial fraud detection
- Demand forecasting in retail and logistics
- Anomaly detection in cybersecurity
- Medical treatment recommendations
- Risk estimation in banking
- Purchase trend analysis
- Consumer sentiment analysis from social media data
Looking to implement AI into your business processes?
38 ML use cases by industry
Retail
1 Inventory management
Processing customer purchase patterns and a range of external factors like seasonality and market trends, machine learning in retail helps companies optimize and streamline their inventory management, preventing stockouts and overstocking.
2 Shelf and store optimization
ML can be used to optimize the layout of stores and shelves and improve customer experience and maximize sales. The algorithm can do it by analyzing customer traffic patterns, product placement, and signage.
3 Workforce optimization
Retail-specific ML solutions can help more efficiently distribute shifts and workload by analyzing customer traffic patterns, sales data, and employee availability.
Ecommerce
Recommendation engines provide personalized product and content recommendations to customers on ecommerce sites. Machine learning algorithms can analyze a customer’s past purchases, browsing behavior, and reviews to create tailored recommendations.
5 A/B testing
Machine learning can be used to analyze the results of A/B tests and determine what changes are most effective for improving customer experience, increasing conversions, and preventing customer churn.
6 Pricing optimization
Embedded into ecommerce sites, machine learning algorithms can automatically optimize the prices of products and services. By analyzing data on sales trends, industry benchmarks, and customer demographics, companies can adjust their pricing models to maximize revenue.
7 Virtual chatbots
Using machine learning and natural language processing, companies can create chatbots to act as shopping assistants and create more life-like shopping experiences for customers. These chatbots can understand customer queries, generate product recommendations, and even process orders.
Healthcare
8 Medical imaging
Machine learning algorithms can analyze medical images such as X-rays, CT scans, and MRI scans. These algorithms identify anomalies in images and provide diagnostic feedback to physicians, significantly reducing diagnosis time and improving its accuracy.
9 Medical transcription and documentation
Machine learning-based tools can be assigned to transcribe medical notes and documentation, thus reducing the time and cost associated with manual data entry and preventing human error.
10 Hospital management
Machine learning can help optimize hospital operations, such as patient scheduling and better resource allocation. By analyzing historical data, ML algorithms can automate routine administrative processes and help hospitals run more efficiently.
11 Diagnostics
Machine learning can detect diseases and anomalies in patient data, from genetic sequences to electronic health records. By leveraging large datasets, deep learning models identify patterns in the data and detect diseases at early stages before they become too severe.
Banking
12 Credit underwriting
Machine learning algorithms can analyze customer data such as credit score, income, and spending history and accurately determine a customer’s creditworthiness to make better underwriting decisions.
Banks can use machine learning algorithms to analyze large sets of customer data and transaction patterns and detect anomalies as well as fraudulent activities and behavior with high probability.
14 Personalized recommendations
Machine learning can be used to analyze customer data and provide personalized recommendations for financial products and services, improving customer engagement and loyalty.
Education
15 Personalization/adaptive learning
Adaptive learning leverages machine learning algorithms to customize the learning experience for each student, offering personalized courses based on a student’s performance, interests, and goals. Thus, educational institutions can use machine learning to help students learn more effectively and reach their academic goals faster.
16 Dropout mitigation
Machine learning can detect patterns in students’ performance data and identify those at risk of dropping out. By leveraging predictive models, educators can intervene before it is too late and provide the necessary support for those in need.
17 AI teachers
Using machine learning and natural language processing, companies can create AI tutors and chatbots to help students learn more effectively. These AI tutors can understand student queries and give answers to them, generate personalized lessons and advice, and even grade assignments autonomously.
Stock market
18 Sentiment analysis
Machine learning algorithms can analyze news and social media data in order to identify market sentiment. ML models can detect patterns in the data and provide insights into upcoming market trends.
19 Algorithmic trading
Algorithmic trading leverages machine learning algorithms to automate buying and selling financial instruments. By analyzing large amounts of data, ML algorithms can identify potential trading opportunities and execute them faster than humans.
20 Stock ranking
Machine learning algorithms can be used to rank stocks based on various factors such as historical data, market trends, and economic indicators and generate values for K, quality, growth, and other score types.
Marketing
21 Content creation
Natural language processing and machine learning techniques can analyze customer profiles and generate relevant marketing messages and content to drive purchasing decisions and boost engagement.
22 In-depth consumer analysis
Machine learning algorithms can assess how consumers respond to advertisements and correlate these emotions to purchasing intentions. By understanding how users feel about the advertisement, marketers can optimize their ad campaigns to achieve the desired effect.
23 Content optimization
Marketers can apply machine learning tools to optimize content for search engines and social media, improving visibility and engagement and delivering more relevant content with less effort.
Real estate
24 Occupancy prediction
Real estate agencies can implement ML-based solutions to analyze data on property usage and predict occupancy rates, enabling their property managers to optimize leasing strategies and maximize occupancy.
25 Price prediction
ML-based pattern recognition techniques can predict the price of properties based on their features, location, surrounding infrastructure, and market trends. This helps real estate agents provide accurate estimates to their clients and streamline the negotiation process.
26 Loan risk assessment
ML algorithms can analyze customer credit history, financial data, and other factors and determine the likelihood of default. By leveraging predictive models, mortgage and loan lenders can assess the risks associated with each customer and make informed decisions on whether to approve or reject their application.
27 Home search personalization
ML algorithms can optimize the home search experience for potential home buyers by intelligently assessing a customer’s preferences based on their search history and web profiles, significantly narrowing down the number of offers and increasing engagement. ML-assisted search engines are a common feature of the world-leading real estate marketplaces.
Manufacturing
28 Equipment predictive maintenance
Using predictive analytics, manufacturers can detect patterns in equipment performance data to anticipate potential failures. This way, companies can timely identify and address emerging issues before they escalate, reducing downtime and maintenance costs.
29 Product development
With the help of ML, manufacturers can accelerate product development cycles and create a complex design optimized for both performance and cost. ML-driven generative design tools consider many parameters, including material properties and design constraints, to generate the most suitable design faster.
30 Demand forecasting
Machine learning algorithms can analyze large datasets and identify patterns in customer demand. This helps manufacturers accurately predict future demand, optimize production cycles, and reduce inventory costs.
31 Quality control
With the help of computer vision, machine learning models can automatically inspect products and detect defects. Such ML solutions can help manufacturers ensure that their products meet quality standards and reduce the need for manual inspections.
Logistics
32 Route optimization
By analyzing real-time and historical traffic data, machine learning models can identify the most efficient routes, reducing delivery times and saving transportation and labor costs. Logistics companies can also predict delivery times more accurately by taking into account loading and unloading times at each route point.
33 Warehouse management
ML can be used to optimize warehouse operations by analyzing data on inventory levels, order volumes, and other factors. By identifying opportunities for inventory management optimization, machine learning models can help logistics providers reduce costs and improve efficiency.
34 Carrier performance analytics
ML can be applied to analyze carrier performance by collecting data on on-time delivery, shipment damages, and other metrics. By analyzing this data, machine learning models can help logistics providers identify the best carriers for each shipment, negotiate better rates, and improve customer satisfaction.
Agriculture
35 Crop management
In combination with drones and computer vision, ML models can monitor crop health, detect pest infestations, and predict yield. Such a system can help farmers to optimize their irrigation and fertilization practices, reduce pesticide use, and improve yields.
36 Water management
ML models can analyze moisture data collected by sensors and predict water needs for different crops. Farmers can then use this information to optimize their irrigation schedules and reduce water waste.
37 Agricultural supply chain management
ML can analyze data on transportation, storage, and distribution and use it to optimize agricultural supply chain management. By providing real-time insights into supply chain operations, machine learning models can help companies reduce waste, improve efficiency, and increase profitability.
38 Harvest prediction
ML can be used to predict the timing and quantity of a crop harvest by assessing data on weather patterns, soil conditions, and other factors. Delivering accurate harvest predictions, machine learning models can help farmers plan their operations more efficiently, reduce waste, and optimize storage and distribution.
How to identify machine learning use cases for your business
To successfully implement ML, companies need to carefully consider a number of factors and have an in-depth understanding of the available options. To help with that, we have developed a step-by-step guide:
1
Pinpoint the improvement area
The idea to implement ML usually stems from problems or inefficiencies in established business processes or the desire to improve workflow speed or outcomes. So the beginners that have their sights on machine learning adoption should begin by answering a seemingly basic question “What are we adopting ML for?”. For this, they need to review the process intended for ML-based improvement and try to identify exact business pain points and areas for improvement.
2
Decide whether ML is the best solution
Machine learning can be a very effective solution, but its implementation isn’t reasonable in every case. ML algorithms require a lot of time, money and effort to build and train, as well as a significant revamp of the company’s IT infrastructure and ongoing maintenance post-deployment. So businesses need to make sure that no regular software solution is already able to deliver the expected results or solve the existing problem.
3
Review the existing ideas
If you’ve decided in favor of ML adoption, you next need to evaluate your rough ML solution ideas more closely in terms of implementation complexity, data availability and quality, and the value they can bring to your business. Moreover, companies should assess whether they can scale up the ML-powered application in the future and what resources they would need for its maintenance. Next, they should assess the regulatory, risk, and transparency aspects of the potential use cases.
4
Assess your ML maturity
To identify suitable use cases for machine learning, one should also assess the organization’s current level of maturity with respect to ML implementation. Companies need to consider factors like the established degree of automation in processes and systems, the quantity and quality of data available for training ML models, and the availability of relevant talent. Assessing your ML readiness is critical to understand whether you can realistically implement complex use cases.
5
Prioritize use cases
At this final stage, companies single out specific tasks or issues the ML solution will be built for. More often than not, this stage can take multiple iterations due to the discovery of hidden implementation bottlenecks. For example, companies can come to the conclusion they should implement several smaller, more flexible ML tools than a single complex solution. This is why effective use case prioritization should be approached gradually, with each potential ML application re-evaluated at every step.
5 benefits of successful ML use cases
Successfully implemented machine learning use cases can bring a range of benefits to organizations, including cost savings, improved efficiency, and better decision-making:
1 Improved efficiency
When ML algorithms and models, including artificial neural networks, are properly applied, they can automate mundane tasks and reduce the amount of time and effort employees spend on a complex task. This way, ML allows companies to allocate resources on strategic initiatives and high-value projects.
2 Enhanced customer experience
By leveraging ML models such as decision trees to analyze customer data, businesses can gain unique insights into customer needs and preferences. With this data on hand, they can create more personalized experiences and sufficiently increase customer loyalty and satisfaction.
3 Streamlined decision-making
ML algorithms can help organizations make better decisions faster by processing data from multiple sources and providing valuable insights into complex and large-scale processes. By arming decision-makers with the right information in a timely manner, businesses can make smarter decisions that have far-reaching effects.
4 Reduced costs
As ML technology automates mundane tasks and eliminates manual processes, it can significantly reduce operational costs and free up resources for other, more important projects. This can drive bottom-line savings and enable businesses to focus on higher-value activities.
5 Improved predictability
ML algorithms are particularly powerful when applied for predictive analytics, allowing businesses to gain wider insights into future trends and better anticipate customer behavior. This can enable companies to plan ahead with greater accuracy, ensuring they’re always one step ahead of the competition. For example, by utilizing ML models for forecasting sales or predicting customer preferences, organizations can develop more accurate strategies and increase their chances of success.
Our data scientists will help you with your ML project
Unlock the benefits of machine learning
Businesses worldwide have already unlocked the benefits of machine learning and proved its infinite potential. From enhanced customer experiences and improved efficiency to better decision-making and reduced costs, the potential of ML is unparalleled compared to other technologies currently on the market. As such, it is wise for businesses to start taking advantage of this technology sooner rather than later to gain a competitive edge. However, companies must remember that the success of an ML project heavily depends on the unique business case of each organization, its goals, and available data. By taking all these factors into account before launching an ML project, businesses can ensure they get the most out of their investment.
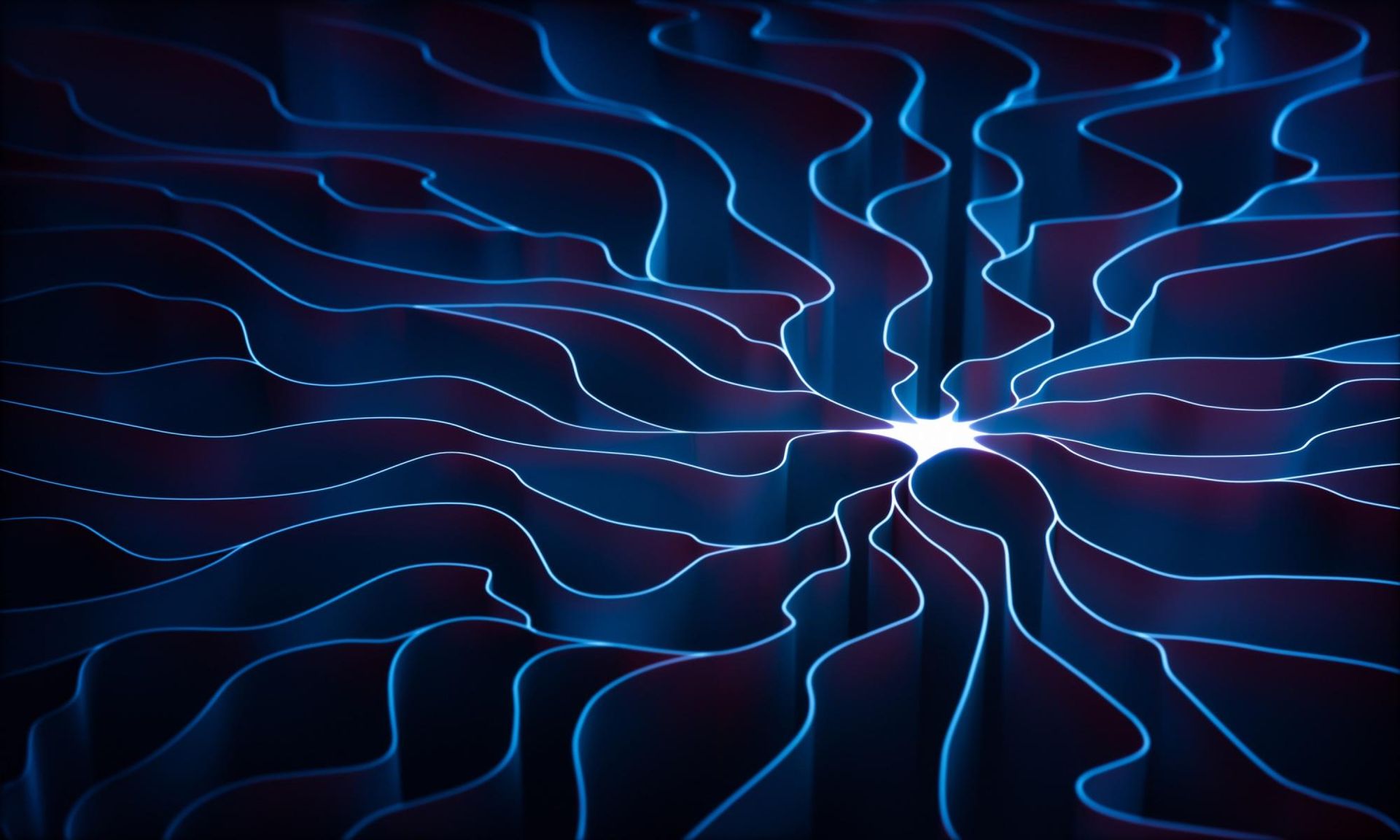
Service
Machine learning consulting services & solutions we deliver
Explore our range of machine learning consulting services, along with related technologies, use cases, implementation roadmap, and payoffs.
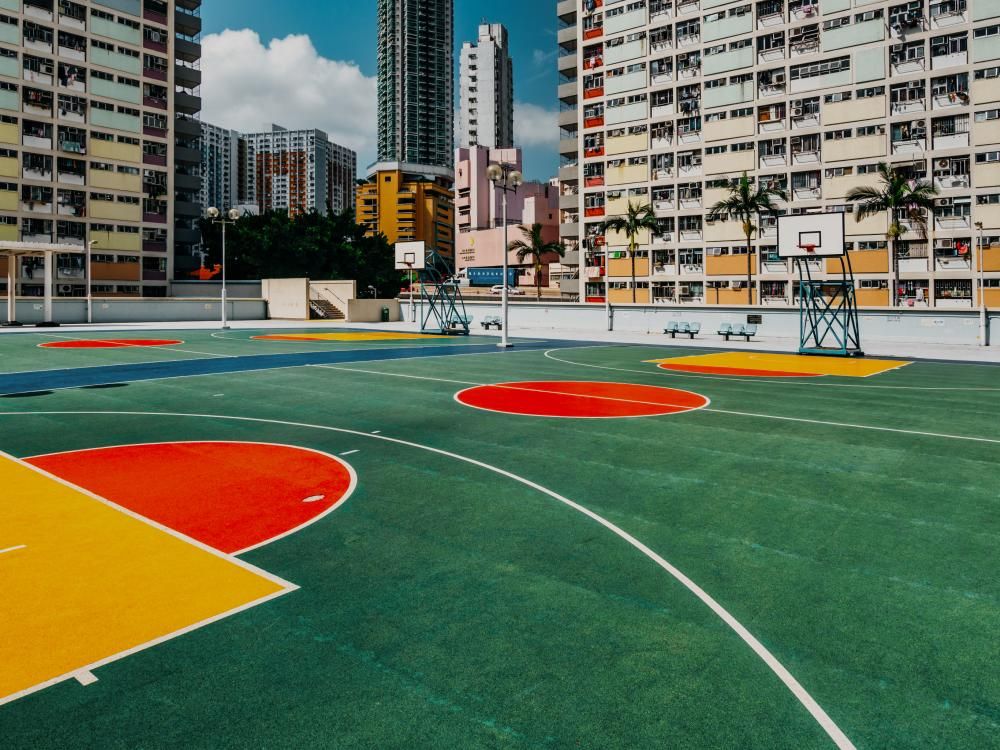
Case study
An ML solution for brand analytics and reporting
Find out how Itransition’s team designed and developed an ML tool for brand tracking and analytics that processes images 50% faster than the legacy solution.
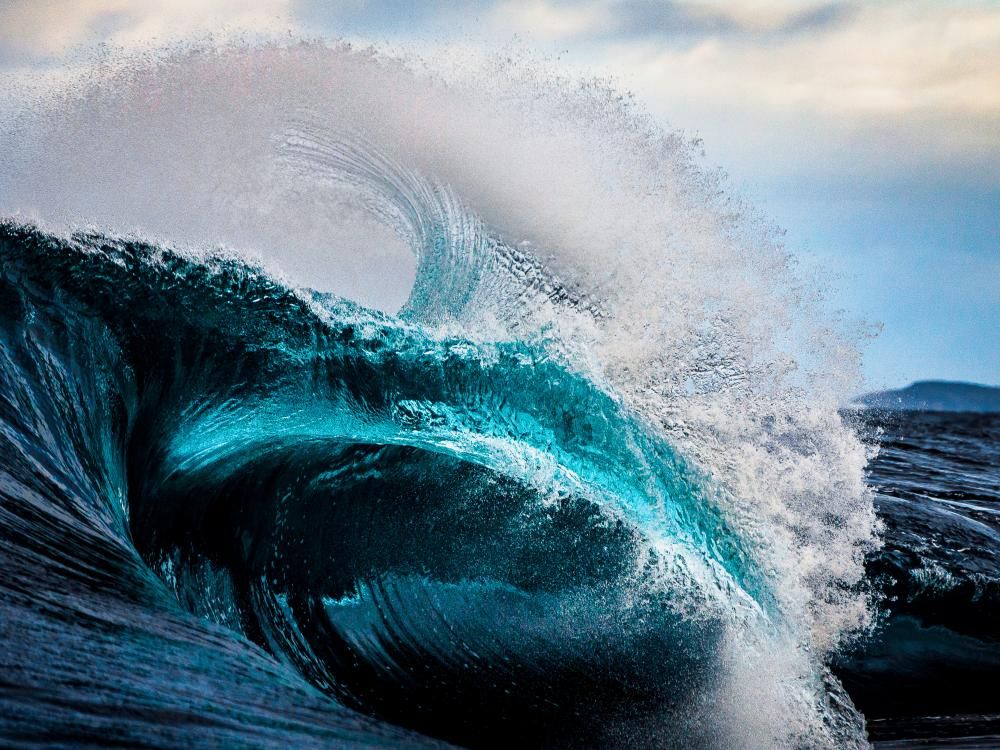
Case study
ML PoC for aquatic environment analysis
Learn more about a PoC of an ML-based plankton detection and classification solution we developed, proving the suggested approach.
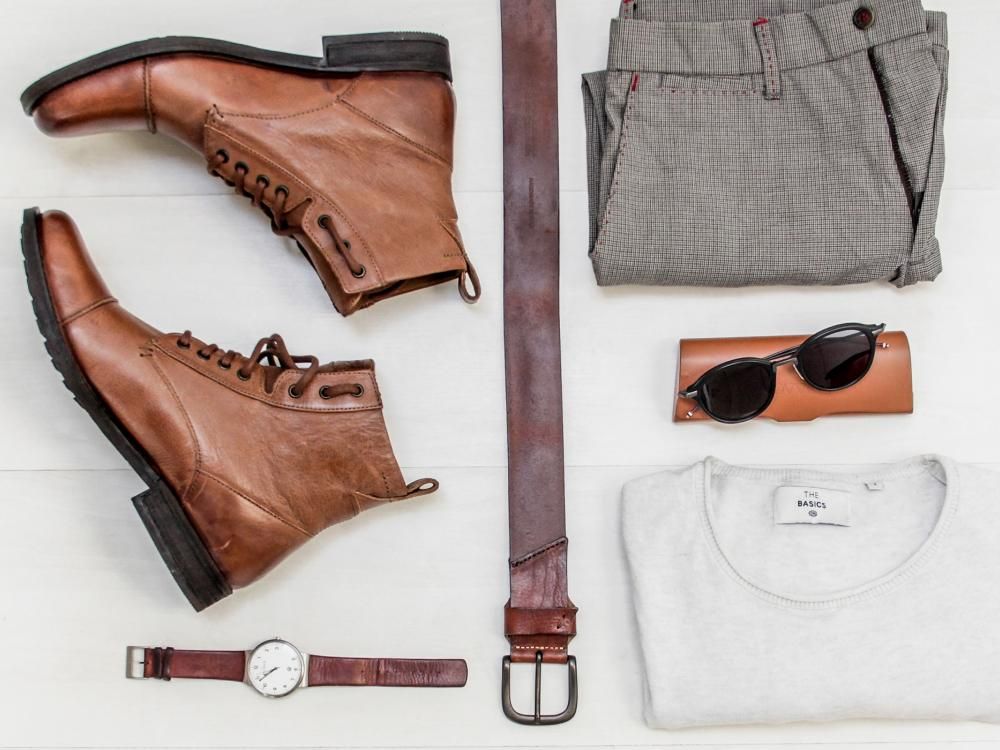
Case study
BI platform with AI and computer vision for a fashion retailer
Learn how Itransition delivered retail BI and deployed an ML-based customer analytics solution now processing 10TB of data.
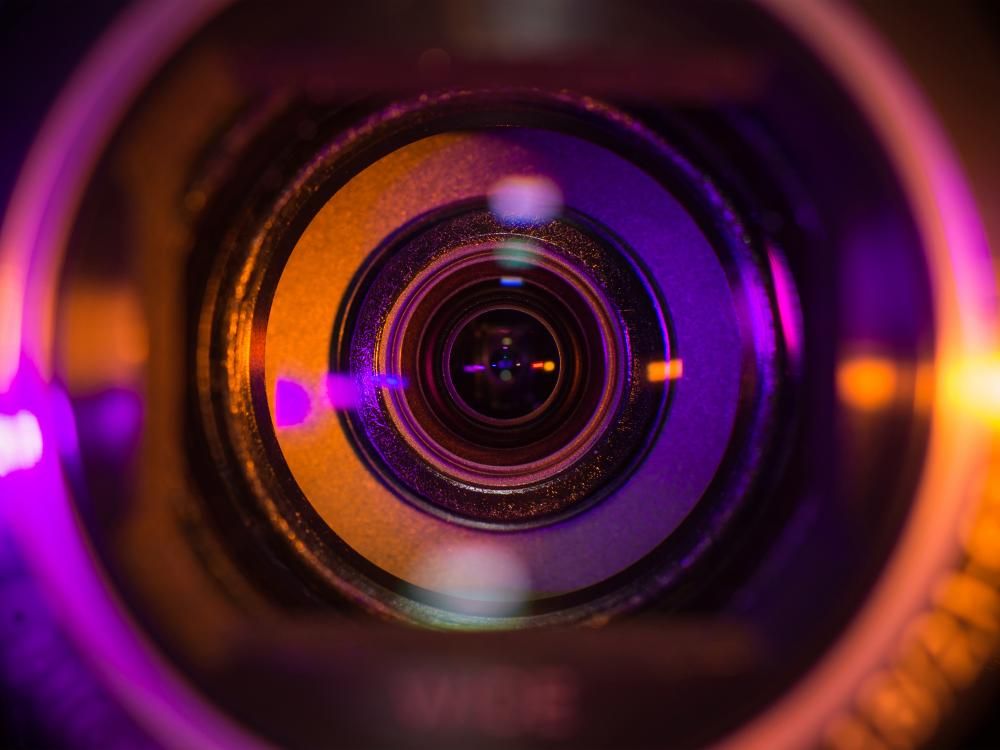
Case study
A shoppable video platform for AiBUY
Find out how Itransition’s dedicated team helped AiBUY release their innovative machine learning-driven shoppable video platform.
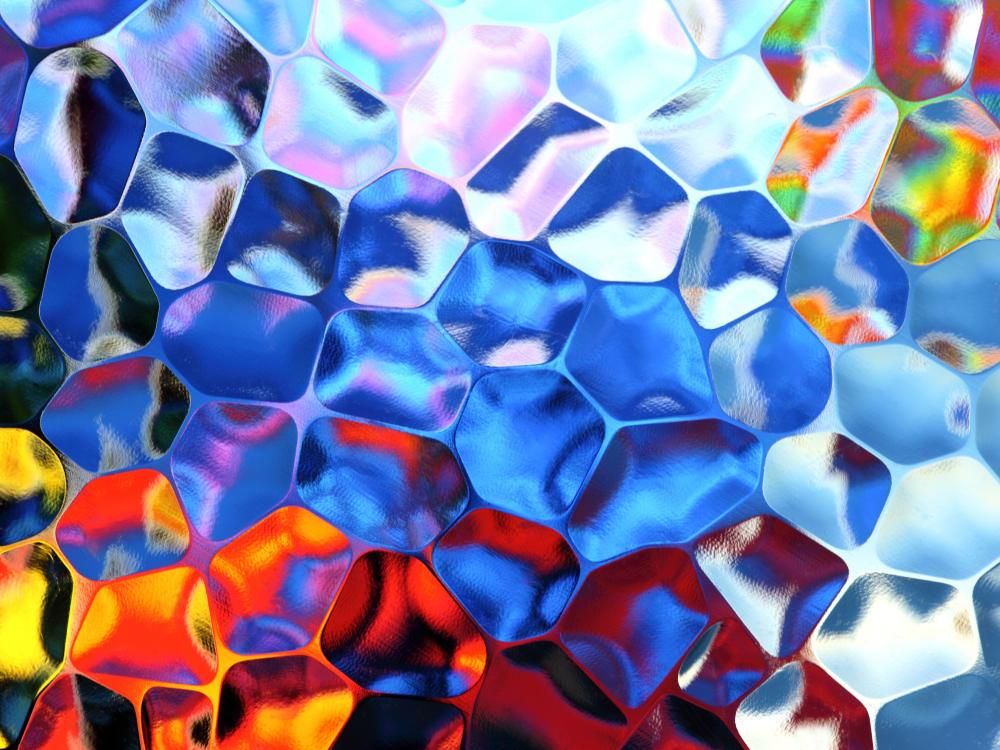
Insights
AI use cases: 3 rules to find the right one for your business
One of the main barriers to AI adoption is identifying the right AI use cases. Find out the best practices to make it less challenging.
More about machine learning services
Services
Industries