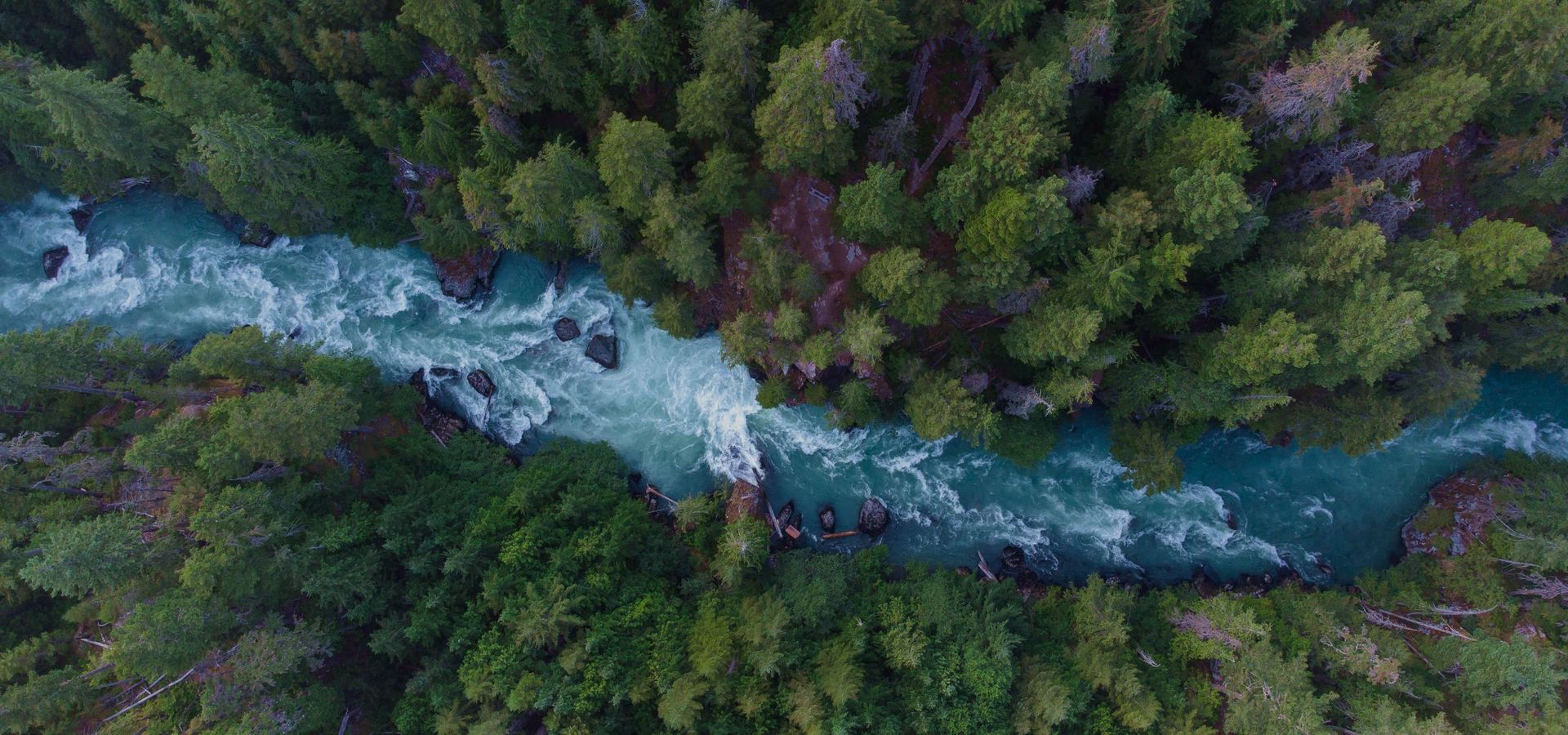
Machine learning in manufacturing: key applications, examples & adoption guidelines
March 21, 2024
Machine learning
- Services
- Use cases by industry
- Insights
Machine learning is now one of the main drivers of manufacturing digital transformation, poised to transform the majority of labor- and data-intensive manufacturing processes and improve companies’ operational efficiency. Here, we discuss the ten most prominent machine learning use cases and the technology’s impact on the industry, while our machine learning consultants outline a step-by-step ML implementation roadmap and explore the common adoption pitfalls.
Machine learning adoption in manufacturing: market statistics
Take a look at the most telling statistics of machine learning in the manufacturing industry:
growth rate of AI in manufacturing from 2019 to 2027
Fortune Business Insights
KPIs for companies which actively adopt machine learning in manufacturing
McKinsey & Company
of companies expect to increase their efficiency with digital technologies
PwC
The importance of ML adoption in manufacturing
Chart 1: Average improvement through machine intelligence, by KPI
Data source: mckinsey.com-Toward smart production: Machine intelligence in business operations, 2022
Chart 2: Nine out of ten companies are investing in digital factories
Data source: pwc.de-Digital Factories 2020: Shaping the future of manufacturing
Machine learning-based technologies used in manufacturing
Predictive analytics
Intelligence process automation (IPA)
Computer vision
Computer vision is a field of artificial intelligence that allows computers to draw insights from visual information. Computer vision in manufacturing is used to automatically conduct a quality inspection of products at every stage of the production process, guide robots during product assembly, and ensure staff safety by proactively monitoring production facilities.Â
Neural networks
Looking for a reliable ML consulting partner?
10 use cases of machine learning in manufacturing
Quality control
Demand forecasting
Contract management
Product development
Production optimization
Cybersecurity
Robotics
Order management
Digital twin
Machine learning across manufacturing sectors
While many machine learning use cases are similar from one manufacturing sector to another (predictive maintenance, product quality control, inventory management), some of them have sector-specific use cases, which we outline below:
Food
- Sorting food with the help of computer vision
- Quickly analyzing the ripeness of fruits and vegetables
- Cleaning equipment that doesn’t need disassembling
Automotive
- Self-driving features
- Voice assistants and emotion recognition features
- Classifying and detecting defects
- Verifying proper assembly of the model
Furniture
- Cost-effective generative design
- Visual inspection of finished products
Semiconductors and computers
- Visual inspection of wafers
- Chip development and design
Plastic products
- Autonomous sorting of recyclable materials
- Optimizing injection molding
Manufacturing companies successfully using machine learning
Signs you need to adopt machine learning
Unsafe working environments
Unsafe working environments
Robots embedded with advanced computer vision systems can differentiate between objects and people and make intelligent decisions about pathfinding and movement in general, which significantly increases on-site employee safety.
Inefficient equipment maintenance
Inefficient equipment maintenance
Assisted with IoT sensors and machine learning, technicians can save valuable time and resources by conducting maintenance checks only when equipment has a high probability of failure in the near future instead of routinely checking machinery conditions.
Inefficient visual inspection
Inefficient visual inspection
Conventional visual inspection systems rely on high-resolution optical cameras that capture visual data, which is then compared to a template to identify defects. Slight variabilities in the surrounding environment or product itself cause a high number of false positives. Machine learning-enabled visual inspection tools, on the other hand, aren't thrown off by environmental changes and can continuously learn, which results in improved visual inspection precision.
Limited design options
Limited design options
While creativity is an exclusively human capability, machines can boost and scale it up. When a machine learning-based generative design system is given a very narrowly-defined set of parameters and limitations to adhere to, the number of design options usually far exceeds human outputs.
Inaccurate demand forecasting
Inaccurate demand forecasting
Instead of relying on a gut feeling and industry experience, organizations can introduce machine learning-enabled systems to streamline demand forecasting. Thanks to its ability to process enormous amounts of data in real time, demand predictions can become much more accurate.
A step-by-step implementation guide
1
Use case definition
As with any technology implementation, it all starts with the definition of use cases, and feasible and value-adding use cases stem from real business needs. For example, if there are too many customer complaints about scrapped products, investing in a machine learning-augmented quality control system might be a good idea.
2
Data gathering
After you've defined a use case, it's time to determine what data you need and where you can source it from. For example, for predictive maintenance, it's data generated by machinery sensors, and for visual inspection systems, it's thousands of images of faulty products.
3
Data cleaning and formatting
Machine learning models are as good as the data you provide them with. For machine learning tools to operate effectively, you need to clean, format, contextualize, and organize the initially gathered unstructured, raw data. Ideally, this process should be performed for every data set you gather, as it can have a significant impact on your future ML applications.
4
Data visualization
While it may seem not mandatory to visualize data for your specific use case, data visualization is a sure way to realize the full potential of machine learning and advanced analytics in general. Easily understandable dashboards can be extremely useful in unlocking valuable insights about manufacturing processes.
5
Model training
After the data is in the desired state, you are all set to train your machine learning model. By running historical and live data through the algorithm, you can identify patterns that lead to inefficiencies in a given process. While choosing the algorithm goes way beyond the scope of this guide, the importance of opting for the right algorithm should never be underestimated.
6
Model validation and deployment
To ensure that your machine learning model can be useful in a real-world scenario, it’s crucial to test it against previously unseen real data. In the majority of cases, these tests reveal the need for sufficient model adjustments and improvement. If after multiple tests, the model works as intended, you can safely deploy it into the production environment.
7
Continuous model retraining
When it comes to machine learning, implementation is never a one-time effort. As the production environment, products, processes, data, and people change and evolve, machine learning models become obsolete quite fast. To maintain model effectiveness, it's critical to constantly retrain, update, and deploy them.
Looking to streamline manufacturing with ML?
Machine learning adoption challenges and how to resolve them
Given an avalanche of potential use cases and benefits that AI and machine learning can bring to manufacturing organizations, one may wonder why this technology is not adopted by more companies. In their 2021 report AI Adoption in the Enterprise, O’Reilly Media found out what aspects of the technology’s implementation proved particularly challenging.
Talent shortage
Lack of data
Finding a starting point
In the manufacturing context, seemingly every use case is the right one for ML implementation. Every manufacturer would love to know the exact reasons for machinery failure and offload routine tasks like inventory management to the algorithms. Successful use case definition boils down to addressing real business needs. In other words, machine learning use cases have to bring tangible financial impact. Then, when you think that you've selected the right use case, it's always a good idea to start small. Deploy that machine learning tool on a single factory floor in a single production line before expanding to other facilities to see if the juice is worth the squeeze.
Boost your production performance with ML
Machine learning is an essential technology for realizing the Industry 4.0 transformation of the manufacturing field, and it's not surprising that companies are actively investing into it. In the meantime, ML solutions are becoming more commonplace among factories as a result of rapid technological advancements. The benefits of machine learning are numerous, including the production of high-quality products, downtime reduction, productivity and efficiency increase, resources optimization, and safety improvement. If are looking to improve your operational efficiency with machine learning, don’t hesitate to get in touch with Itransition’s experts and discuss your project.
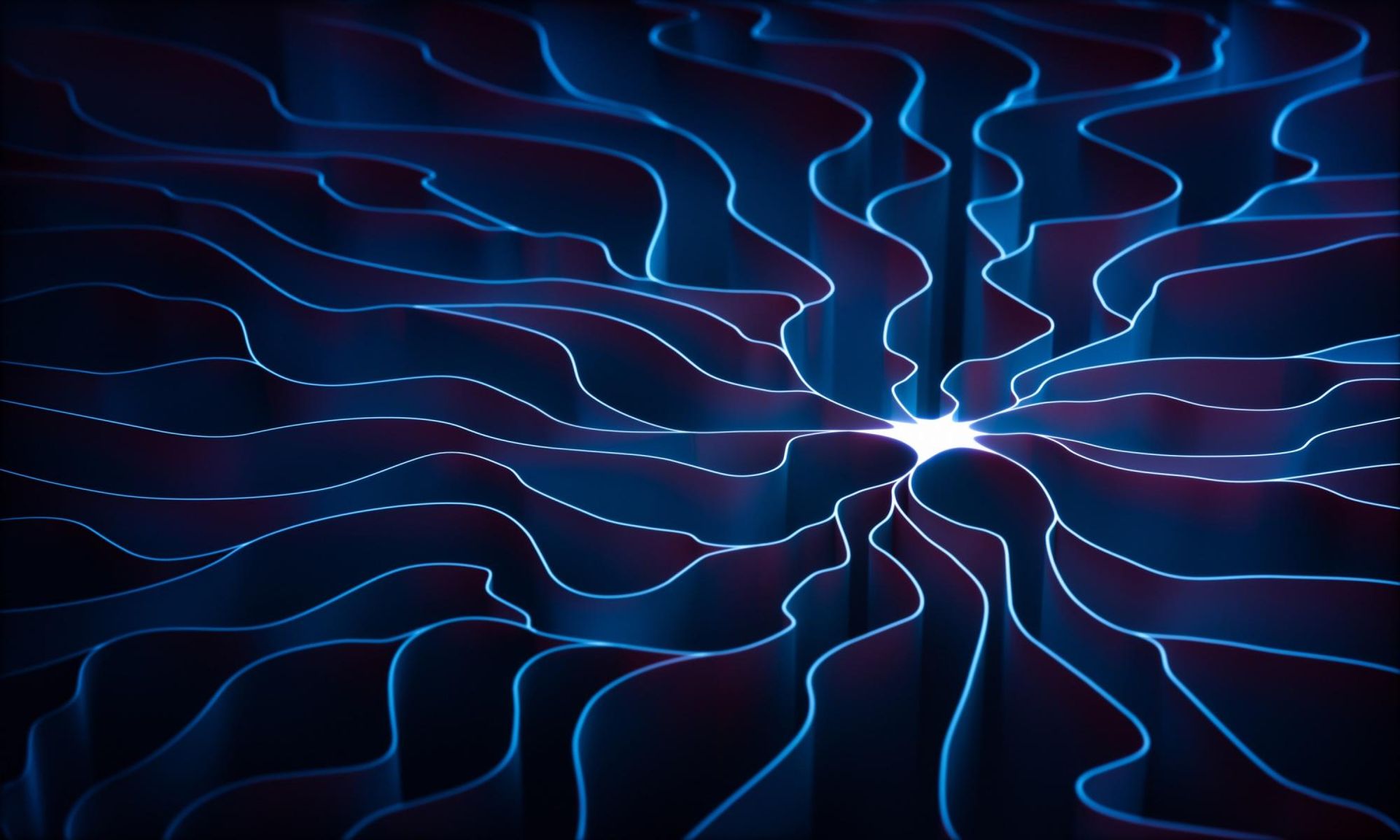
Service
Machine learning consulting services & solutions we deliver
Explore our range of machine learning consulting services, along with related technologies, use cases, implementation roadmap, and payoffs.
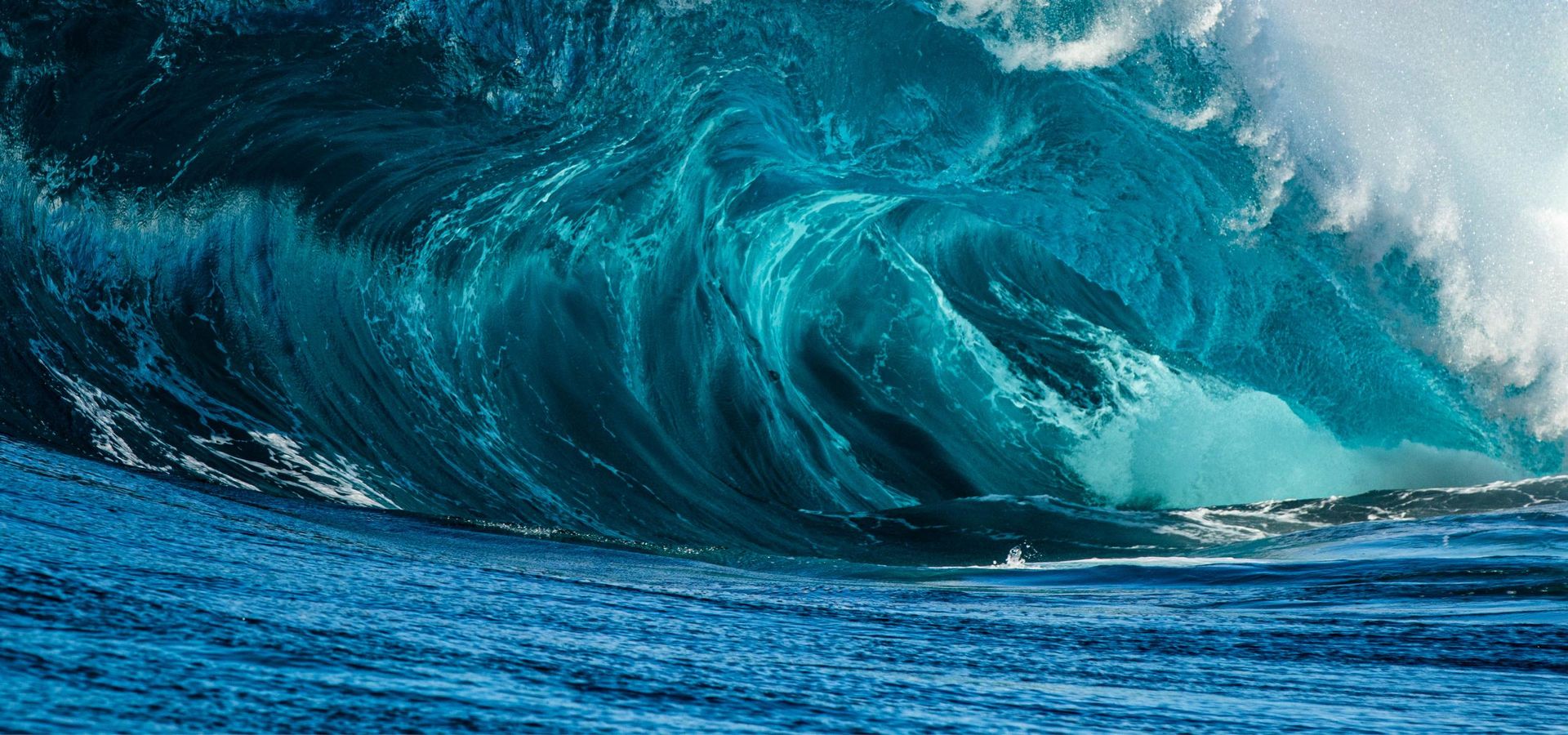
Service
Predictive analytics consulting
Predictive analytics consulting will help you foresee customer behavior and market demand, predict fraud and customer churn, forecast sales and financial risks.
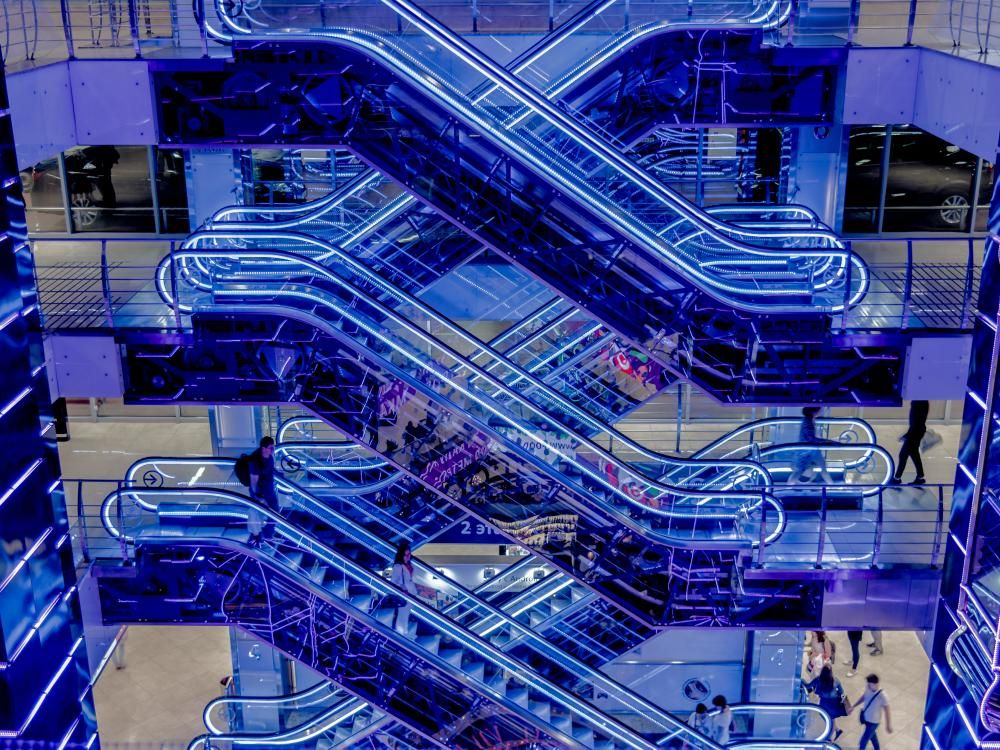
Insights
Machine learning in retail: 10 ways to upgrade your store
Explore the top use cases of machine learning in retail and find out what benefits this technology can bring to your business.

Insights
Using AI to scale wealth management
Discover how wealth management companies use AI to generate more leads, automate back-office tasks, improve customer relationships, and improve bottom lines
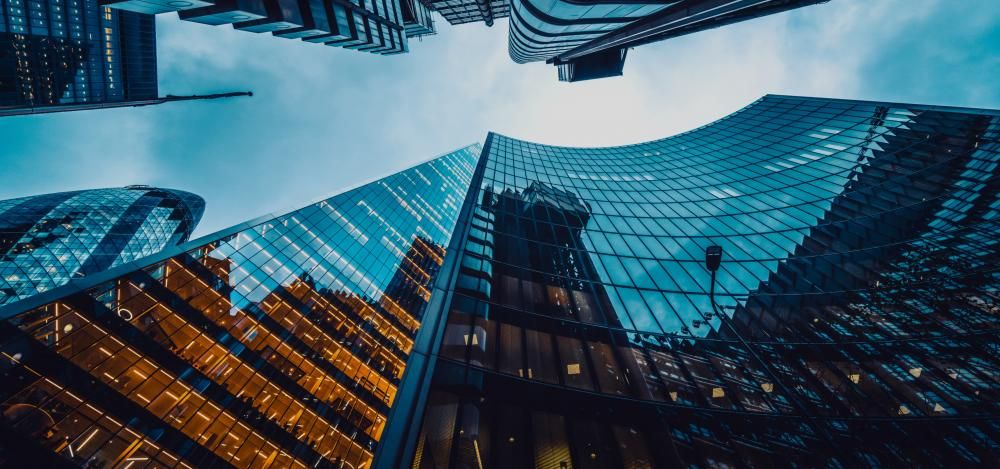
Insights
A guide to predictive analytics in finance
Learn how financial institutions can apply predictive analytics in finance to minimize risks, decrease operational costs, and improve their bottom lines.