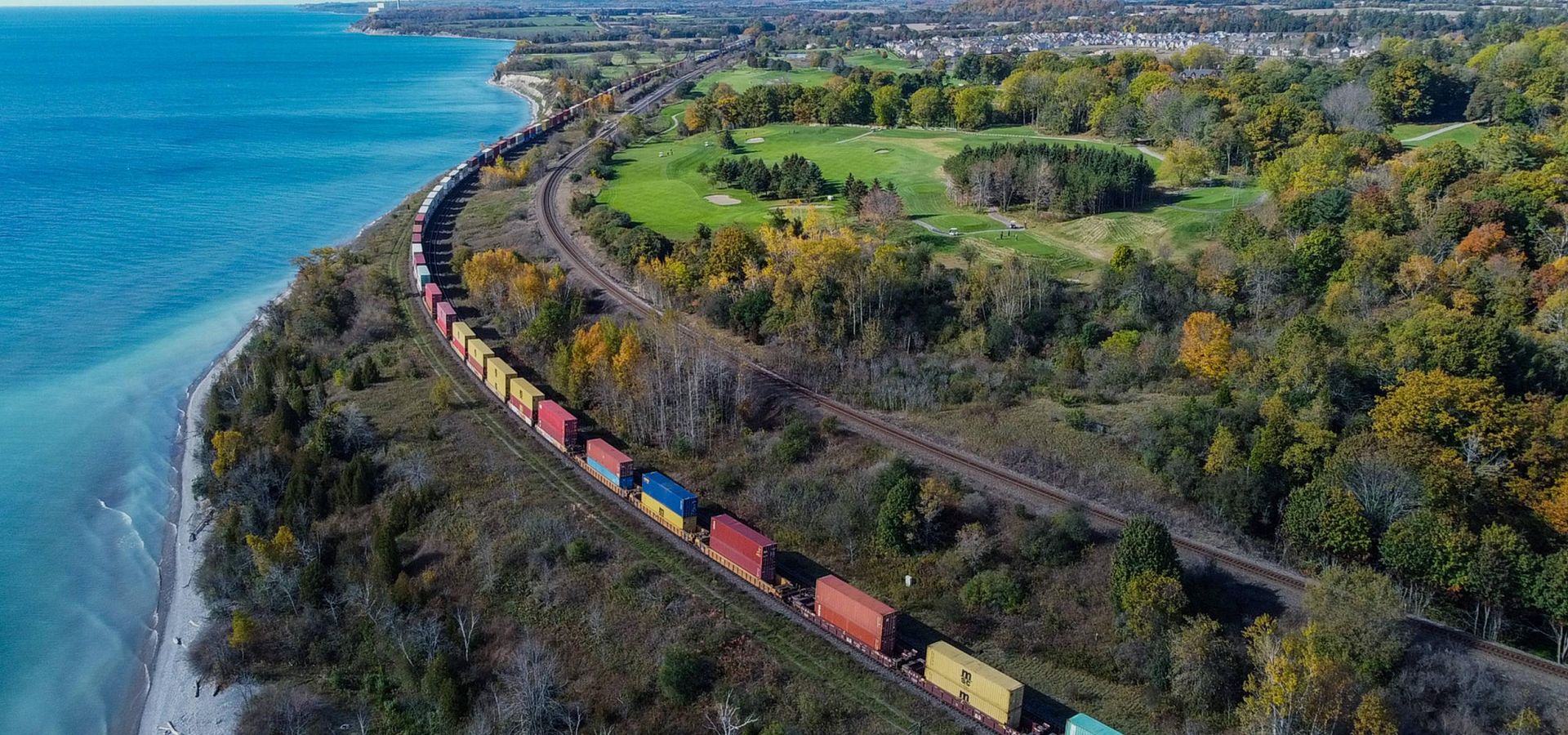
Machine learning in logistics: technology breakdown & 10 use cases
January 25, 2023
- Home
- Machine learning
- Logistics
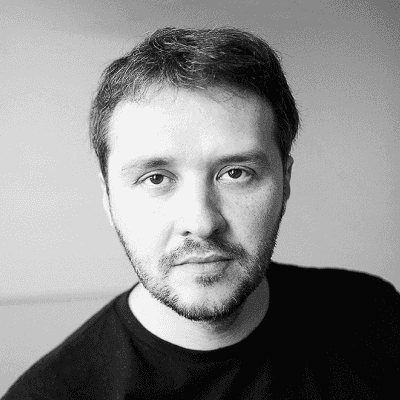
by Aleksei Shinkarenko,
BI Architect
With the rapid increase of globalization, the development of international logistics has never been more important. As our reliance on international freight transportation continues to grow, effective and technology-driven supply chain management becomes the backbone of staying on top of the competition. That is why more and more logistic companies are seeking machine learning advisory.
Machine learning in logistics: a summary
In this article, we will discuss how machine learning solutions can revolutionize the logistics industry and maximize operational performance. We’ll also explore the different ways logistics companies are applying machine learning, provide implementation advice, and look at the technology’s potential for years to come in the logistics industry.
Machine learning in logistics market statistics
of logistics industry leaders consider technology as a competitive advantage
Gartner
of commercial supply chain management vendors will use AI and data science by 2026
Gartner
projected CAGR of AI in the supply chain market from 2021 to 2028
BlueWave Consulting
Best 10 ML use cases in logistics
Route optimization
ML and AI tools for transportation can analyze data such as traffic patterns and distances between locations and work out the most efficient routes for freight delivery in real-time. Combined with predictive analytics, ML can also help logistics companies save time and money by alerting them about costly traffic jams or delays caused by weather or other unforeseen circumstances.
Workforce planning
Fraud detection in payment systems
By analyzing patterns and data across multiple sources, ML models can detect suspicious activity and fraudulent payments more accurately and quickly than humans. Additionally, these models can help identify new types of fraudulent activities that may have gone undetected before. With ML-based fraud detection technology in place, companies can also craft new, more efficient fraud prevention strategies to prevent financial losses as well as potential customer data breaches.
Demand prediction
Predictive maintenance for vehicles
Self-driving delivery vehicles
Equipped with modern-day ML-powered sensors, autonomous vehicles can navigate complex cityscapes and rural areas with little to no human intervention. Their implementation for delivery can reduce labor costs, optimize fuel usage, help drivers handle unexpected road conditions, improve drivers’ security, plan optimal routes for deliveries, and even predict traffic patterns to avoid delays.
Autonomous drones for package delivery
Supplier relationship management
Dynamic pricing
Looking for reliable machine learning experts?
Real-life examples of ML in logistics
As the trucking industry faces a continued driver shortage, the demand for autonomous trucks that enhance the safety, efficiency, and sustainability of long-haul trucking is increasing.
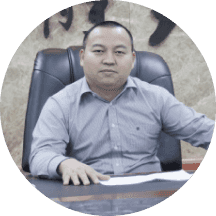
Liu Zhiyuan
CEO and Founder of Guangzhou Zhihong
Image title: Coupa supply chain demand modeler
Data source: coupa.com — Demand Modeler
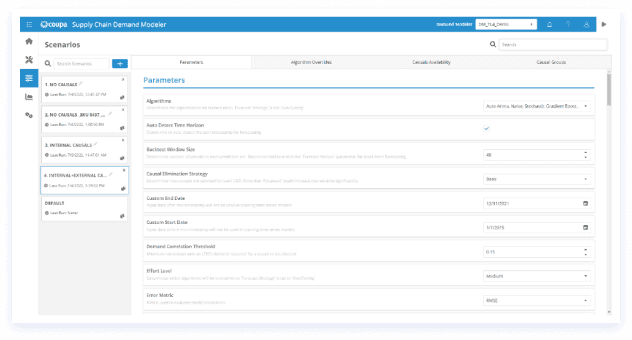
Image title: Controlant’s app in action
Data source: controlant.com — Controlant now providing monitoring and Supply Chain Visibility for Pfizer-BioNTech COVID-19 Vaccine distribution and storage
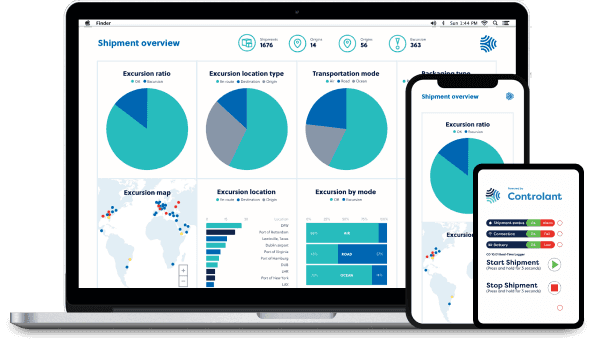
Image title: NHSBT supply and demand planning app
Data source: kortical.com — AI supply chain optimisation for platelets to reduce costs
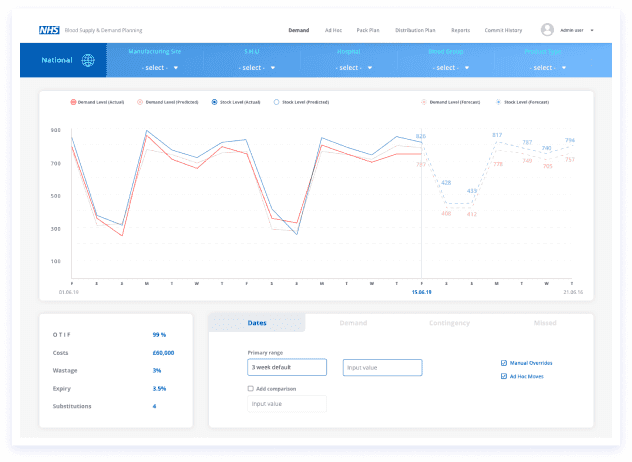
Machine learning technologies used in logistics
Predictive analytics
Predictive analytics is a data mining technique that uses statistical models and algorithms to analyze current and historical data sets and make predictions about future outcomes. Applied in logistics and supply chain, predictive analytics tools use data from customer orders, delivery times, transportation costs, and other data to identify patterns, help anticipate problems before they arise, and take provisional actions.
Computer vision
The Internet of Things
Similar to the combination of IoT and AI in the architectural domain, the mix of ML and IoT technologies has the potential to revolutionize the logistics industry. Together, they can provide a powerful solution that collects, processes and analyzes vast amounts of data from connected devices and makes decisions automatically. In logistics, ML and IoT can be applied for optimizing supply chain routes, predicting demand for goods and services, anticipating disruptions in the flow of goods, and improving customer experience.
Implementation guidelines
1
Define the problem
ML implementation should always be driven by a real business need. It’s paramount for technology leaders to collaborate with supply chain experts to figure out which problems prove to be most disruptive to their company and if emerging technologies like ML can help solve these issues.
2
Assess your ML readiness
Next, companies should check their readiness for integrating ML. This includes the impact of ML adoption on workforce and workflows, identifying personnel gaps, machine learning ROI in the long term, and implementation expectations.
3
Establish data governance standards
Given that machine learning shines only when fed enough data, logistics companies need to sort out where and how they acquire data. After determining what issue they want to address with ML, companies should identify relevant data sets, the more the better, for model training.
4
Collaborate with other companies
The key to reaping maximum benefit from ML lies in building an ecosystem of partners that mutually benefit from exchanging region- and niche-specific information. With an abundance of actionable data, ML models have the highest chances to add value.
5
Involve industry experts
Explaining the variability in data with the help of ML is a complex initiative. The development of accurate forecasting models requires not only data science and programming expertise but also industry-specific knowledge. This is why it's critical to involve supply chain professionals early in the process and during model development in particular.
Benefits of machine learning in logistics
Increased visibility
Improved customer service
Enhanced decision- making
Automated processes
Increased scalability
Improved delivery times
Automated data analysis
Improved forecasting
Reduced logistics risks
Faster response times
ML in logistics
Provides greater transparency into the supply chain by tracking data throughout the entire shipment journey.
Personalizes the customer experience by automatically predicting customers’ needs and resolving their common inquiries.
Helps to make better decisions faster and more efficiently based on large volumes of disparate data.
Automates manual tasks such as route optimization, task allocation, and inventory management, reducing the need for manual labor.
Improves operational scalability by automating processes that usually require human input.
Reduces delivery times by optimizing routes, managing inventory levels, and providing accurate customer information in real time.
Generates detailed reports on customer behaviors and trends, used to optimize logistics operations.
Accurately forecast demand to help companies manage inventory levels and improve supply chain performance.
Minimizes logistics risks by tracking shipments and providing accurate customer information in real time.
Speeds up response times by automatically detecting problems and offering solutions to them much faster than humans.
Increased visibility
Provides greater transparency into the supply chain by tracking data throughout the entire shipment journey.
Solving key logistics challenges using ML techniques
Challenge
Solution
Inefficiency due to manual processes
High transportation costs
Limited visibility
Keeping track of shipments is essential for effective shipping management. ML can make supply chain smarter and more transparent, providing logistics companies with real-time insights into their operations.
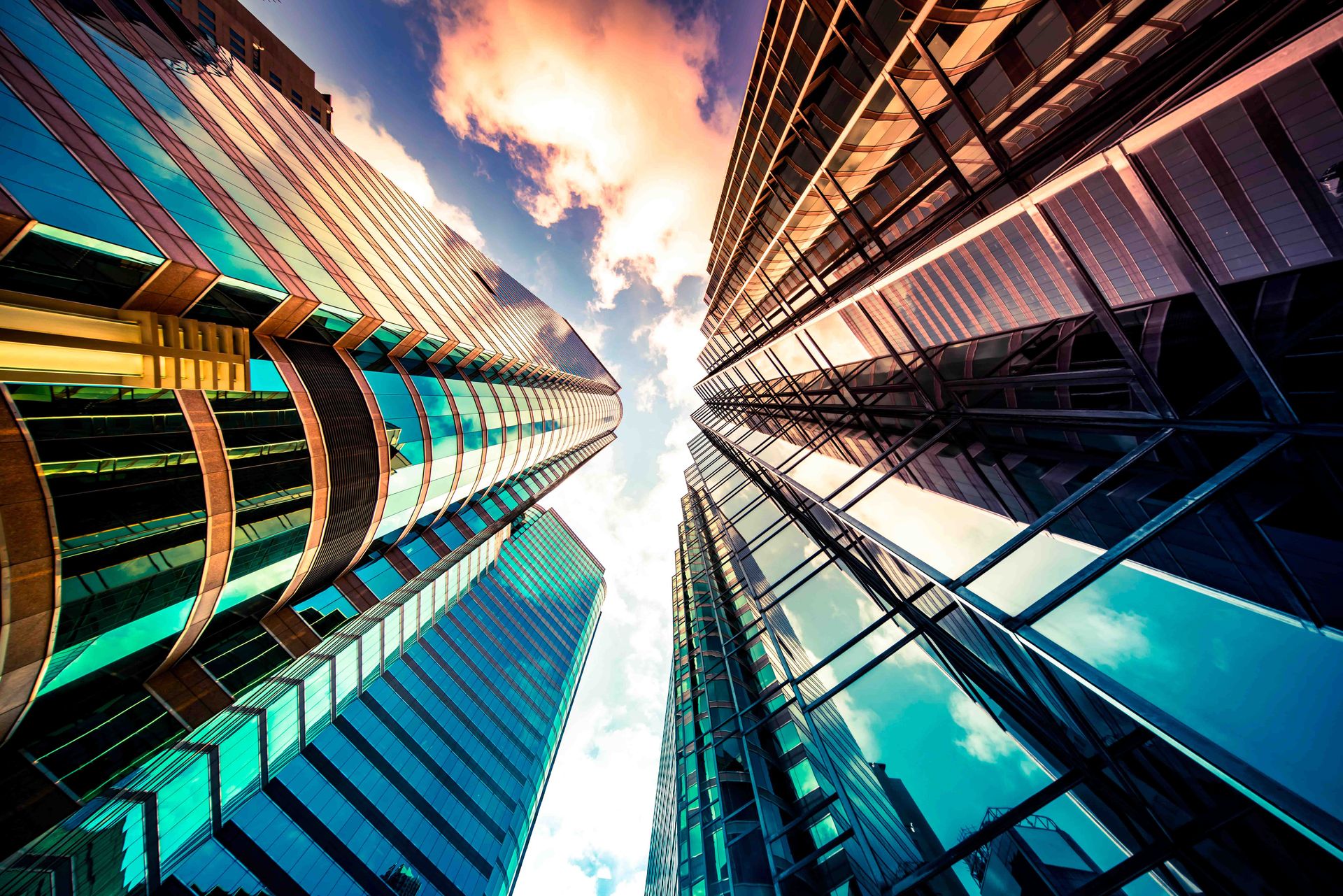
Streamline supply chain management with ML
A growing number of customers who demand swiftly and error-free delivery is forcing supply chain companies to rethink their approach to operations. Due to ML’s ability to quickly process large amounts of data from a myriad of sources and suggest solutions for emerging operational challenges within minutes, the technology will inevitably become a staple in logistics. If you want to optimize your supply chain operations with the help of machine learning, contact us to make their logistics operations more efficient.
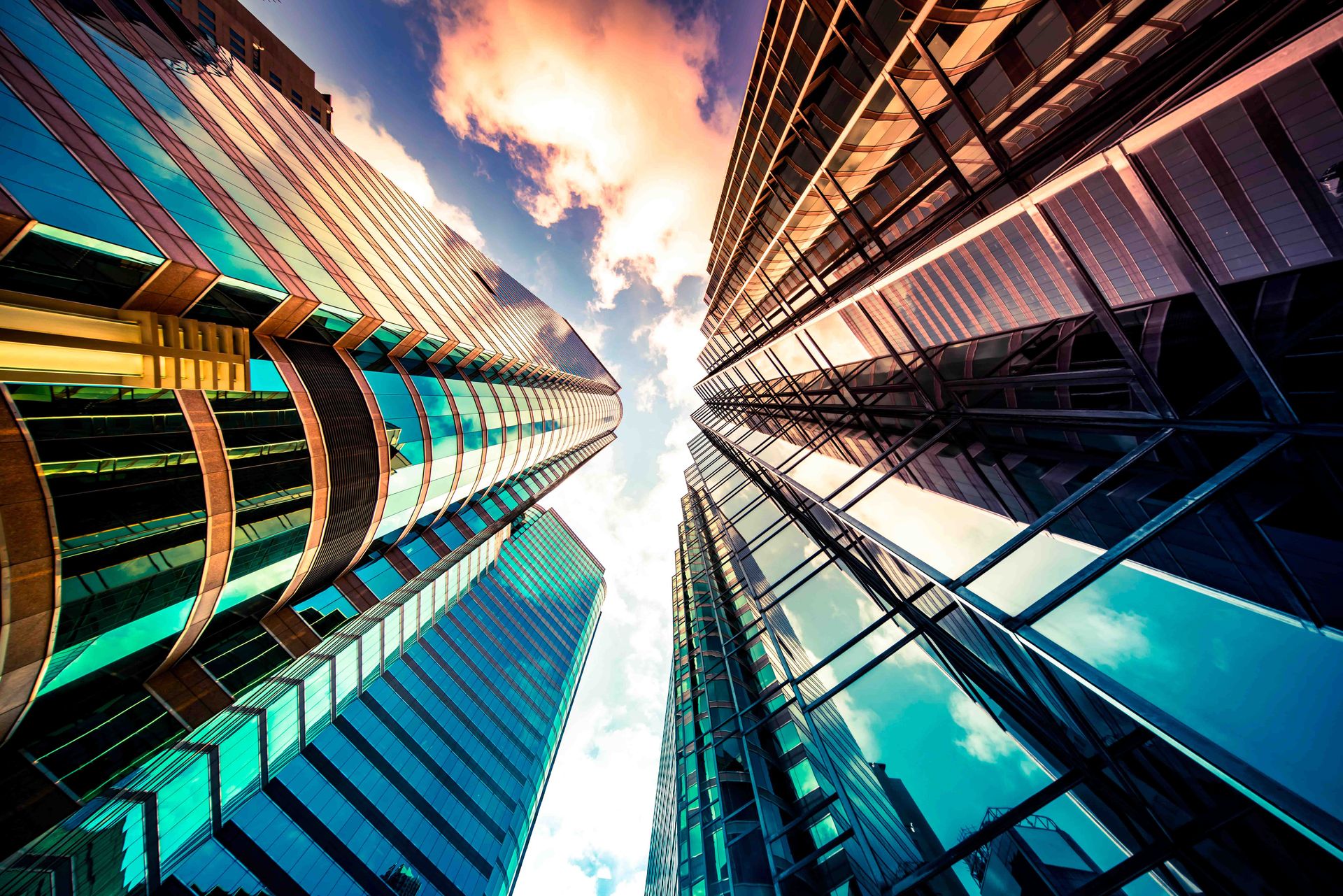
Want to streamline your logistics operations with machine learning?
FAQs
How machine learning is used in the supply chain?
Machine learning in the supply chain can be applied for demand forecasting, route optimization, inventory management, workforce and supply chain planning, and vehicle predictive maintenance.
How can machine learning be used in warehouses?
Machine learning can be used in warehouses to better manage inventory, track shipments, predict customer demands, and suggest optimal stocking levels for each item, which can result in reduced costs and improved delivery times.
What other techniques of artificial intelligence are used in logistics?
Other types of artificial intelligence commonly used in logistics include natural language processing, computer vision, and predictive analytics.
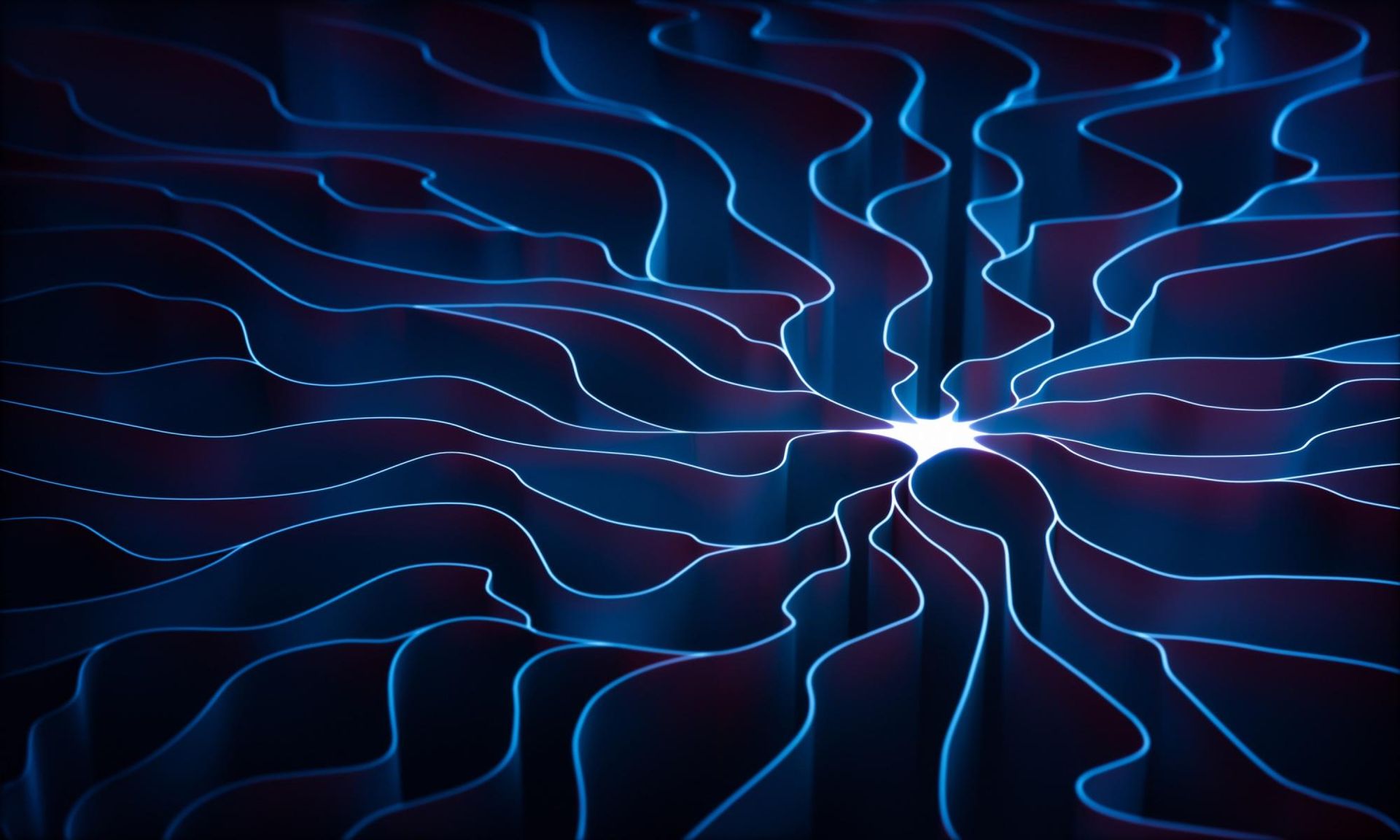
Service
Machine learning consulting services & solutions we deliver
Explore our range of machine learning consulting services, along with related technologies, use cases, implementation roadmap, and payoffs.
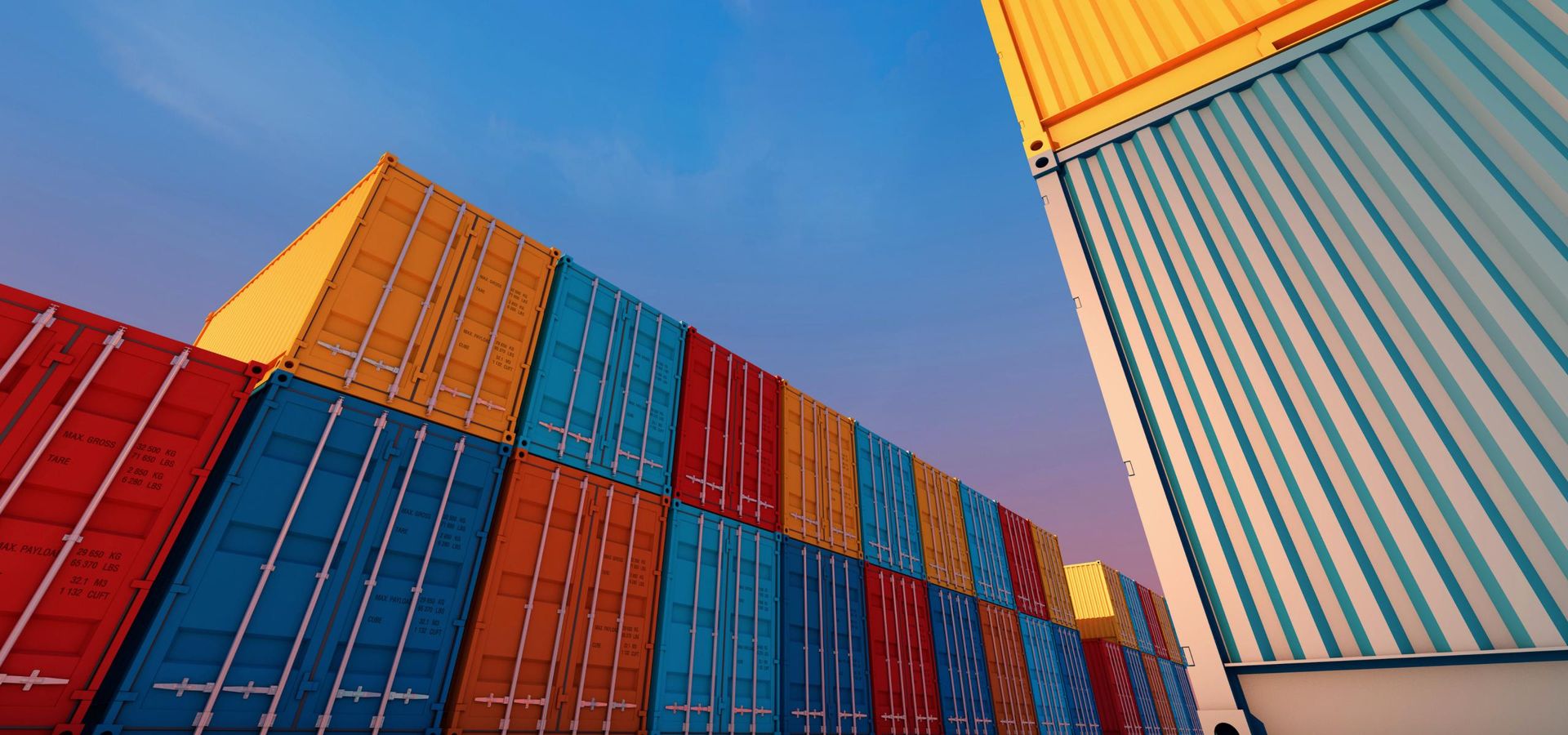
Service
Logistics software development
Explore Itransition’s range of logistics software development services, along with top solutions, technologies, and implementation guidelines.
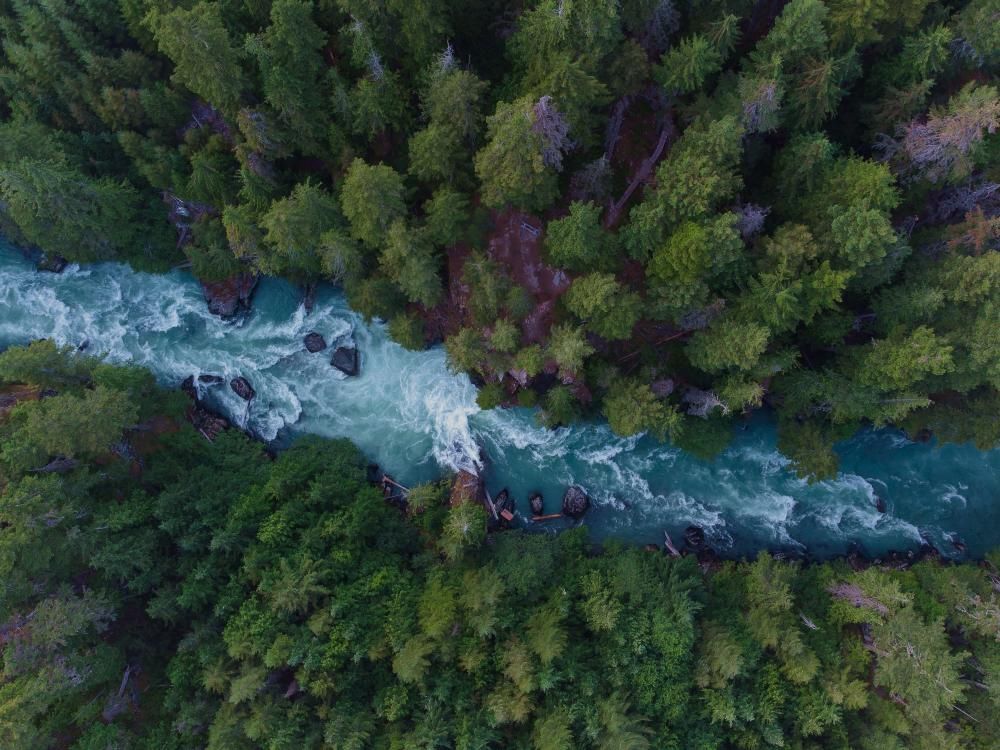
Insights
Machine learning in manufacturing: key applications, examples & adoption guidelines
Learn how machine learning can help manufacturers to improve operational efficiency, discover real-life examples, and learn when and how to implement it.
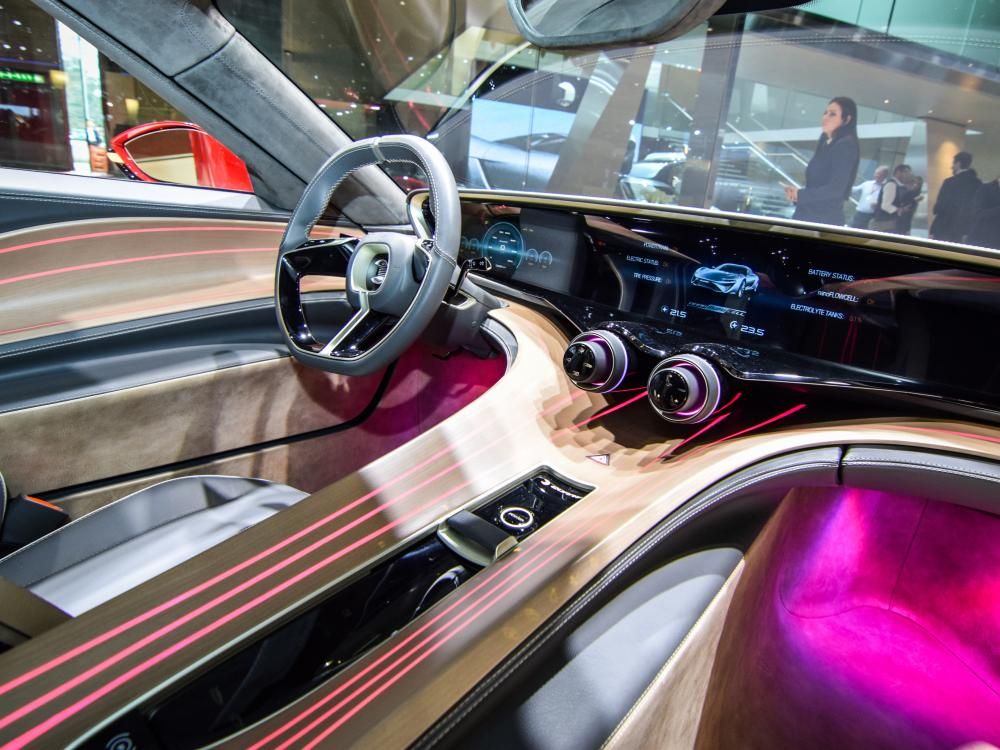
Insights
AI in the automotive industry: use cases, success stories & adoption guidelines
Explore key use cases, payoffs, and real-life examples of AI in the automotive industry, along with common adoption challenges and tips to address them.
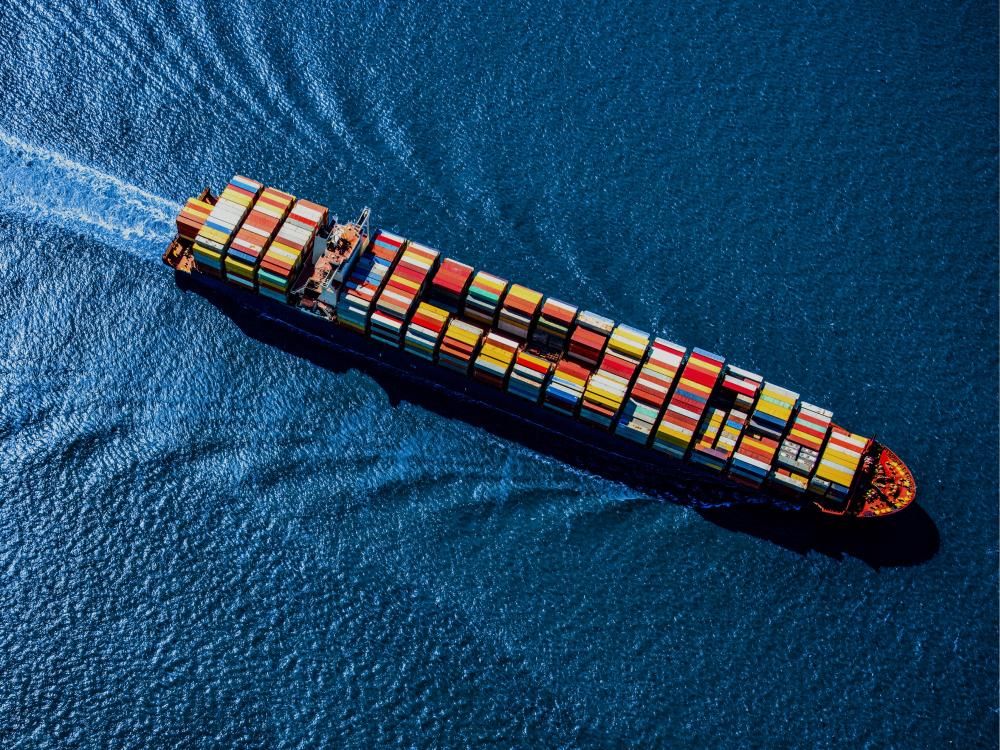
Case study
Robotic process automation for a global logistics company
Learn how Itransition automated three labor-intensive logistics processes using robotic process automation, improving the company's operational efficiency.
More about machine learning services
Services