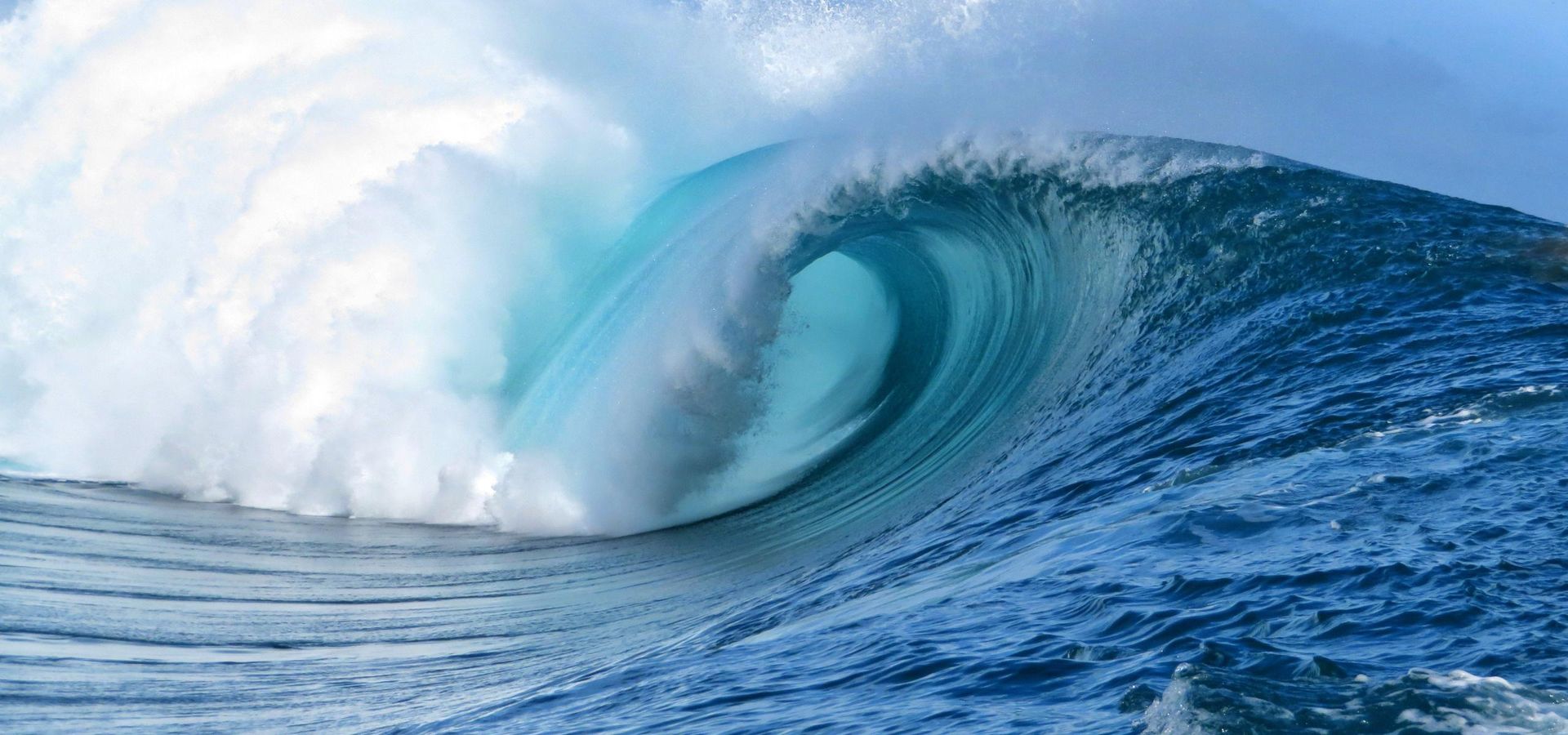
Machine learning for fraud detection: essentials, use cases, and guidelines
September 26, 2023
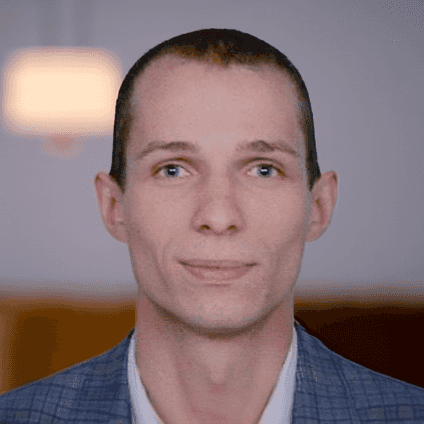
Head of AI/ML Center of Excellence
Explore the nature, payoffs, and applications of this technology in common fraud scenarios, along with some guidelines to streamline its adoption. Finally, find out how machine learning development experts can help you protect your company and customers from ever-evolving fraud threats.
Machine learning in fraud detection: trends and stats
of organizations experienced some form of fraud over the previous 24 months
PwC
of organizations already leverage AI and ML to detect and deter fraud
ACFE
of organizations plan to adopt AI and ML for fraud detection in the next 2 years
ACFE
ML-based vs rule-based fraud detection
ML-enabled anomaly detection applications differ from traditional software in terms of detection technique:
ML-based fraud detection
- ML solutions autonomously identify and use more complex and variable rules than traditional systems. To do so, ML algorithms process data on past fraud cases, discover patterns and relationships between data points, and build models trained to identify those patterns once they recur in future datasets.
- ML systems can predict imminent criminal actions by identifying anomalies, namely subtle and unconventional behavioral patterns that humans would probably overlook but that still deviate from the norm, which could be clues to upcoming fraud.
- ML-powered solutions improve with experience, refining their models over time as they process new data, including unmapped data points. So, if they encounter new fraud scenarios, machine learning-based anomaly detection systems will quickly adapt to such threats, automatically integrating and updating the existing rules without human intervention.
Example
Rule-based fraud detection
- Traditional rule-based solutions follow an "if/then" logic and trigger a response when a predefined condition is violated.
- These tools must be manually instructed to detect fraud cases by compiling libraries with hundreds of rules.
Example
Consult our experts to implement machine learning the right way
Technical overview of ML for fraud detection
Supervised learning
ML-based fraud detection systems are trained with large amounts of labeled data, previously annotated with certain labels describing its key features. This can be data from legitimate and fraudulent transactions described with "fraud" or "non-fraud" labels, respectively. These labeled datasets, which require rather time-consuming manual tagging, provide the system with both the input (transaction data) and the desired output (groups of classified examples), allowing algorithms to identify which patterns and relationships connect them and apply such findings to classify future cases.
Unsupervised learning
These algorithms are fueled with unlabeled transaction data and have to autonomously group these transactions into different clusters based on their similarities (shared behavioral patterns) and differences (typical vs unusual patterns which can correspond to fraudulent activity). This approach, typically associated with deep learning, is computationally demanding but can be the only choice when facing fraud attempts that have never been met before and therefore unlabelled.
Reinforcement learning
This trial-and-error approach involves multiple training iterations in which the algorithm performs a fraud detection task in different ways several times until it can accurately identify fraudulent and non-fraudulent attempts. Since it does not require labeled inputs, reinforcement learning can be applied without prior knowledge of the current fraud scenario. However, it requires considerable computing power.
Scheme title: Supervised vs unsupervised learning
Data source: medium.com — An Executive’s View: Introduction to Machine Learning
Machine learning vs deep learning
Machine learning
A subset of AI focusing on systems that can learn and improve with experience. Identifying patterns and anomalies is one of their most common tasks and a key enabler for fraud detection. “Traditional” ML solutions can be trained with a relatively small amount of data and don’t need substantial computational power, at least compared to deep learning-based software.
Deep learning
A machine learning method typically associated with complex sets of algorithms known as deep neural networks. Such structures, comprising connected layers of artificial neurons that mimic the human brain, can process data flowing from one layer to the next and spot the most subtle patterns and features hidden within an immense amount of data points. This particular process makes neural networks very effective in detecting fraud, but it also requires enormous computational power and large data sets for proper training.
ML algorithms
Logistic regression
A supervised learning algorithm which calculates the probability of one event out of two alternatives, such as "fraud" and "non-fraud" based on a set of relevant parameters.
Decision tree
Another algorithm of the supervised learning subset is a tree-like decision-making model in which every bifurcation represents the analysis of a certain metric or condition (spending threshold, location, etc.) to determine whether an operation is fraudulent.
Random forest
A combination of several decision trees to further expand the amount of data types and conditions examined and identify non-linear relations among multiple variables.
Support vector machine
Several systems rely on this supervised learning algorithm for credit card fraud detection because of its excellent performance with large datasets despite it being computationally demanding.
K-nearest neighbor
This supervised learning algorithm, which proves quite accurate but difficult to interpret when making a certain decision, can frame the nature of an event (be it fraud or non-fraud) by comparing it with similar occurrences recorded in the past.
Neural networks
Complex, multi-layered architecture and superior big data analysis capabilities make neural networks the go-to algorithms when it comes to spotting non-linear relations and dealing with unprecedented fraud scenarios through supervised, unsupervised and reinforcement learning.
Machine learning in top fraud scenarios
Machine learning can be deployed to keep fraudsters and cybercriminals at bay in a variety of scenarios. Let’s take a look at some popular use cases of this technology.
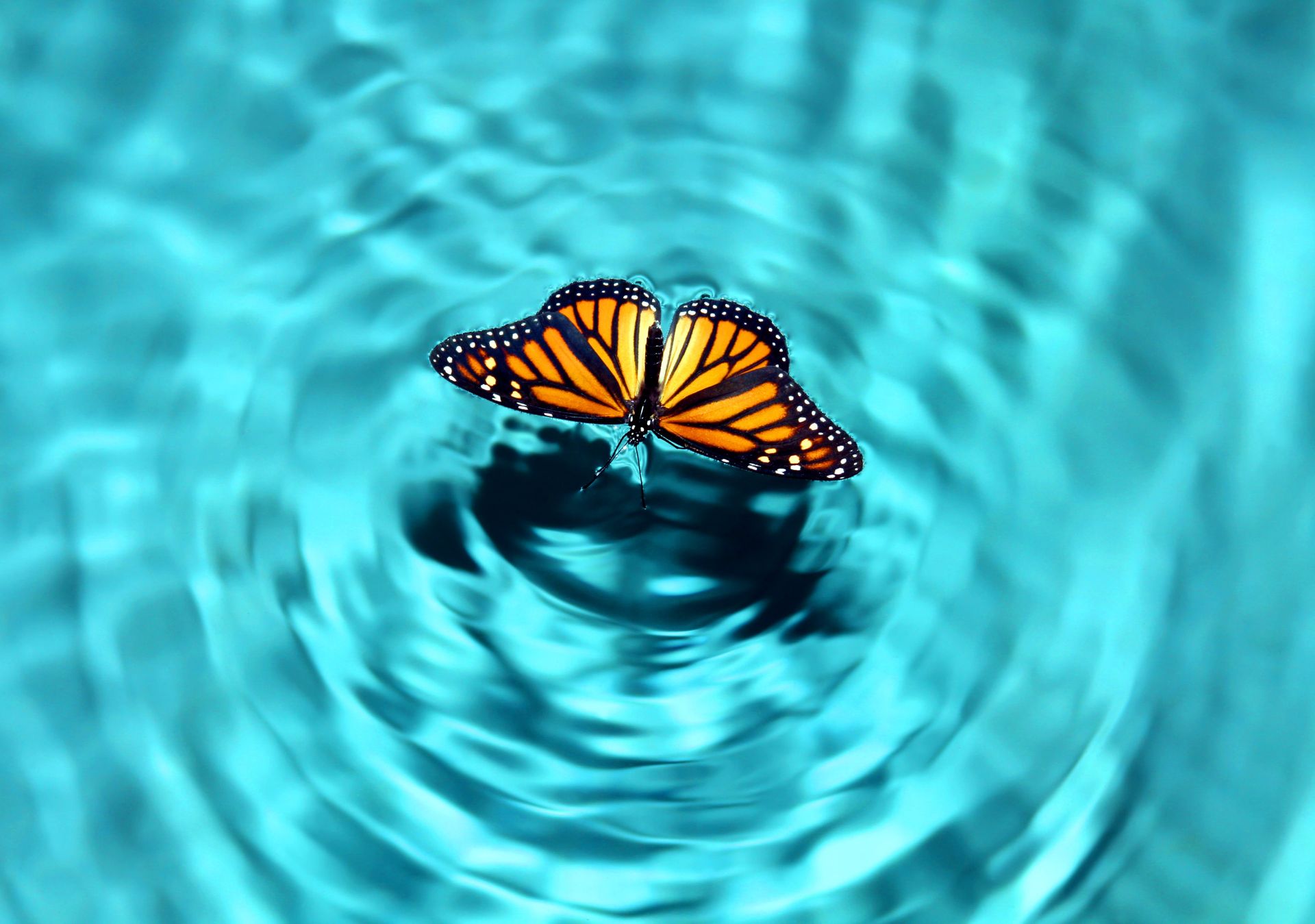
1 Market manipulation
Financial institutions have come to understand the synergistic potential between the stock market and machine learning and the benefits of predictive analytics in finance, considering the large sums involved and the required compliance with increasingly strict regulations.
ML-driven systems can help prevent financial fraud, such as churning, spoofing, and wash trading, by spotting anomalies in stock traders' activity and cross-checking transactions and brokers' data to detect inconsistencies in the information provided.
2 Money laundering
Applying machine learning in banking has proved useful to track anomalous transactions that could be a sign of criminal activity, such as large sums of money exchanged among a group of newly-established companies registered in tax havens.
Machine learning models can be trained with data relating to three possible scenarios: lawful transactions, money transfers flagged as suspicious by bank alert systems, and potential money laundering cases reported to the authorities. For each case, machine learning systems analyze the senders’ and receivers' background or their previous transaction histories. This way, they can spot the same patterns once they recur in future scenarios and therefore distinguish between legitimate activities and criminal actions.
3 Credit card fraud
Electronic payment fraud represents a widespread crime taking many forms and encompassing almost any industry, from banking to ecommerce. However, machine learning systems in retail have a good chance of containing this escalation by detecting abnormal account behavior that could be a sign of fraud. Some of the factors to consider are a growing transaction frequency (especially to purchase premium goods), payment on a card carried out significantly before the due date, associations with different high-risk accounts, and multiple payment methods added in a short time.
Machine learning-driven systems for payment fraud detection can also update card users' behavioral profiles after each transaction, making future predictions more precise and avoiding false positives.
4 Identity theft
Another type of crime strictly connected to payment card fraud and remarkably widespread in many scenarios, including fraudulent loan applications and ecommerce scams, is identity theft. This involves a scammer exploiting the personal information of another individual, including their name and credit card number, without their consent to commit a crime. To deal with this kind of fraud, we can count once again on machine learning-driven analysis of users' habits and transaction data.
Furthermore, we can leverage machine learning-powered computer vision to analyze identity documents or add additional verification mechanisms such as face recognition and biometrics.
5 Fraudulent insurance claims
Machine learning solutions can also strengthen fraud detection in the insurance sector, especially in health insurance. Algorithms can identify false and duplicate claims, pointing out a customer who reported an incorrect diagnosis or exaggerated medical coverage costs.
One of the most valuable machine learning techniques in healthcare is natural language processing, which ensures an in-depth analysis of unstructured data such as medical reports. These solutions leverage machine learning to scan documents written by doctors, insurers, or clients, searching for suspicious inconsistencies.
6 Tax fraud
Public authorities can use machine learning capabilities in identifying unusual patterns to enhance audit and tax compliance. For example, ML can examine the general ledger for anomalous entries which could be the signs of attempted fraud.
Algorithms can spot a wide range of clues easier and faster than human auditors, taking into account various parameters. These include monthly variations in companies' gross sales, relations among different taxpayers, inconsistencies among purchases, or the itemized deductions in an income peer group.
Get these ML benefits with Itransition’s guidance
Real-world examples of ML for fraud detection
Scheme title: The scope of Capgemini’s fraud detection solution
Data source: capgemini.com — Next generation fraud management solutions
Scheme title: Danske Bank’s fraud detection system operation
Data source: assets.teradata.com — Danske Bank Fights Fraud with Machine Learning and AI
Scheme title: Amazon Fraud Detector’s architecture
Data source: aws.amazon.com — Amazon Fraud Detector
Nasdaq adopted a deep learning-based solution to monitor over 17.5 million trades per day, recognize fraudulent equity orders, report them to the authorities, and thus ensure transparent markets. By probing unfamiliar trading patterns, it can even shed light on suspicious events which can end up being previously unknown types of fraud. This system operates in combination with human analysts and the preexisting system based on rules and statistical techniques.
It [points] out what we call an ‘interesting event’. It’s not necessarily a prohibited activity, but it’s what the model has deemed to be interesting because it’s not normal market behavior.
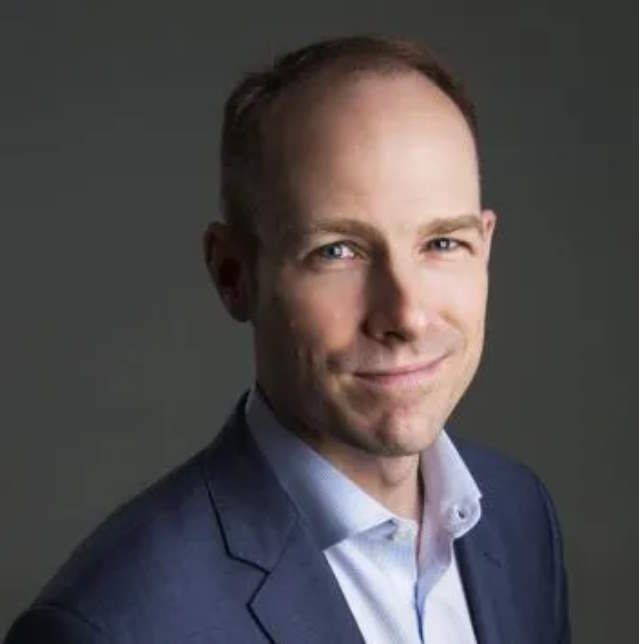
Mike O'Rourke
Senior Vice President, Head of Artificial Intelligence and Investment Intelligence Technology, Nasdaq
How to set up an ML system for fraud detection
1
Business analysis
Identify fraud prevention-related needs and challenges via discovery workshops and process observations
Evaluate your current tech ecosystem
Understand if it makes sense to opt for ML-based fraud detection software over conventional solutions
Define the solution’s functional and non-functional requirements
Set up a project roadmap, including scope, deliverables, and timeframes
2
Initial data analysis
Perform an exploratory analysis to map available data sources (corporate databases and connected devices such as ATMs or POSs)
Identify external data sources (public records, law enforcement, or government watch lists)
3
Product design
Draw up a specification detailing the solution’s architecture, modules, core features, UI/UX, and integrations with other software
Identify a suitable tech stack to build your software
Optionally, deliver a proof of concept to ensure the project’s feasibility and financial viability while pointing out potential adoption challenges
4
Building the CV solution
Perform data pre-processing, including data cleansing, annotation, and transformation
Extract the most relevant features (customer's IP, preferred payment methods, number of failed transactions, average order value, fraud rate of the issuing bank)
Outline the fraud detection system’s evaluation criteria
Process your datasets with ML algorithms to train the model to recognize patterns and anomalies, or build multiple models until you achieve the desired output
5
Model integration and deployment
Integrate the ML model into the solution to power its fraud detection capabilities with the model’s output
Deploy the system to the target environment (on-premise or cloud-based)
Configure all necessary API- or ESB-based integrations with other corporate systems and data sources
6
Support
Closely monitor the system’s operation and perform ongoing maintenance with the help of experienced ML engineers
Provide your staff with user training and support
Following MLOps' best practices, retrain the ML model with new datasets across multiple iterations to fine-tune its output and address model drift issues
The benefits of ML in fraud detection
The distinct mechanisms driving ML-based fraud detection and fraud prevention solutions make them superior to more traditional, rule-based systems in several respects.
1 Higher flexibility and reactivity
The rule-based approach is not flexible enough to deal with rapidly evolving fraud patterns. After all, the rule sets are manually coded and built on previous fraud scenarios, so they should be continuously adjusted according to new types of events. Thanks to self-learning abilities and sheer processing speed, ML-driven systems can deal with this process far quicker than rule-based tools and their human reviewers by adjusting machine learning models on their own based on new kinds of threats.
2 Wider data pool for analysis
Statistical and rule-based fraud detection methods can process structured data, such as transaction figures, but can struggle when handling unstructured data, for example, written reports, insurance claims, and pictures from IDs and other documents. In the meantime, machine learning and its related technologies, such as natural language processing and computer vision, can deal well with any kind of data, enabling companies to analyze more criteria and variables across data points.
3 Lower rate of incorrect outcomes
The traditional approach tends to follow a "black or white" mindset. This results in a massive amount of false positives that don’t represent a real threat but still need to be double-checked through an expensive and time-consuming manual review. Machine learning-based systems, instead, are far more accurate and cost-efficient even in complex scenarios, as they don’t rely on strict rules but search for patterns and dependencies among a wider amount of variables. This helps them to grasp some nuances, understand the logic behind a suspicious case, and avoid false alarms.
4 Superior compliance
The need for constant human intervention and interaction with data of rule-based systems can clash with the increasingly strict trading, fiscal, and data management regulations. With less reliance on manual operations, the adoption of machine learning systems can minimize human errors and mitigate compliance-related business risk.
Challenges of ML for fraud detection
Despite their proven efficiency, ML-based fraud detection systems can be complex to develop and adopt. Here are a few tips to streamline their implementation while overcoming potential drawbacks of this technology.
ML model interpretability
Issue
Recommendation
False positives/negatives tradeoff
Issue
Recommendation
Data privacy concerns
Issue
Recommendation
Development complexities
Issue
Recommendation
Fighting crime with algorithms
The more our society moves towards full digitalization, the more cybersecurity attacks grow in impact, frequency, and complexity, with scammers and cybercriminals leveraging the same technologies that legitimate institutions deploy to counter them. As a result, AI and ML crack passwords, power adaptive bots, and manipulate datasets on the one hand, and power highly effective fraud detection systems on the other. Fraud detection solutions have proved to live up to the hype, thanks to their adaptability to new threats, smart context-based data analysis, and real-time identification capabilities. At the same time, ML’s limited interpretability, appetite for sensitive data, and substantial training and computing requirements should be approached with proper expertise and compliance in mind. To smooth out the challenges of ML adoption, rely on an experienced partner like Itransition.
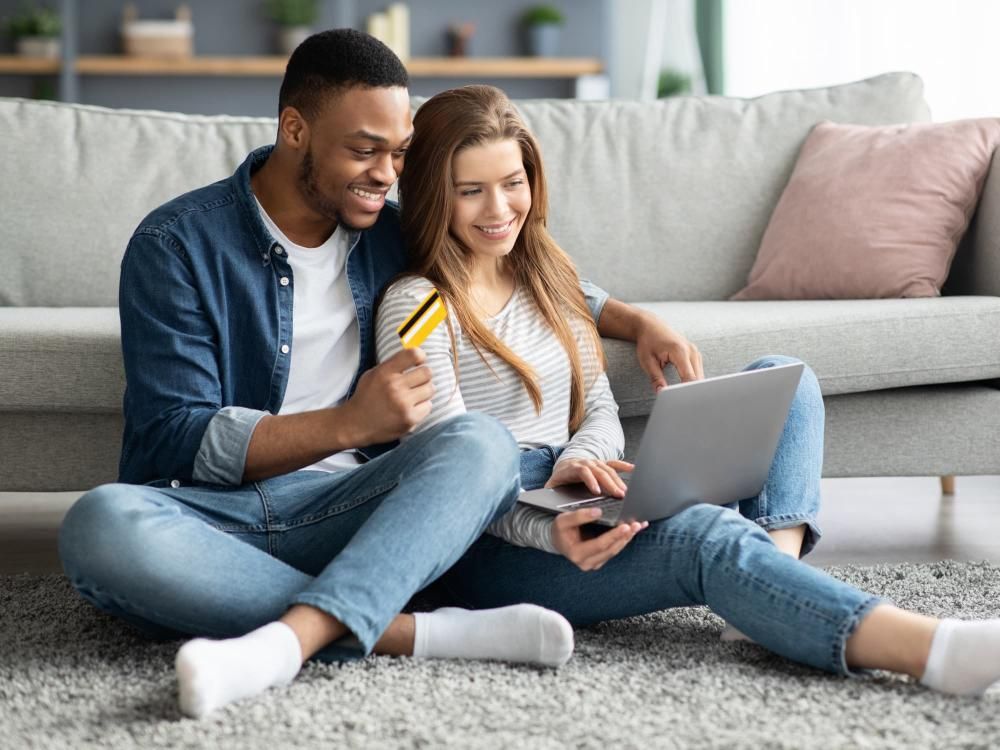
Insights
Machine learning in ecommerce: 10 use cases, examples, and best practices
Discover the key trends, use cases, payoffs, and examples of ML in ecommerce, along with some best practices to streamline its adoption.
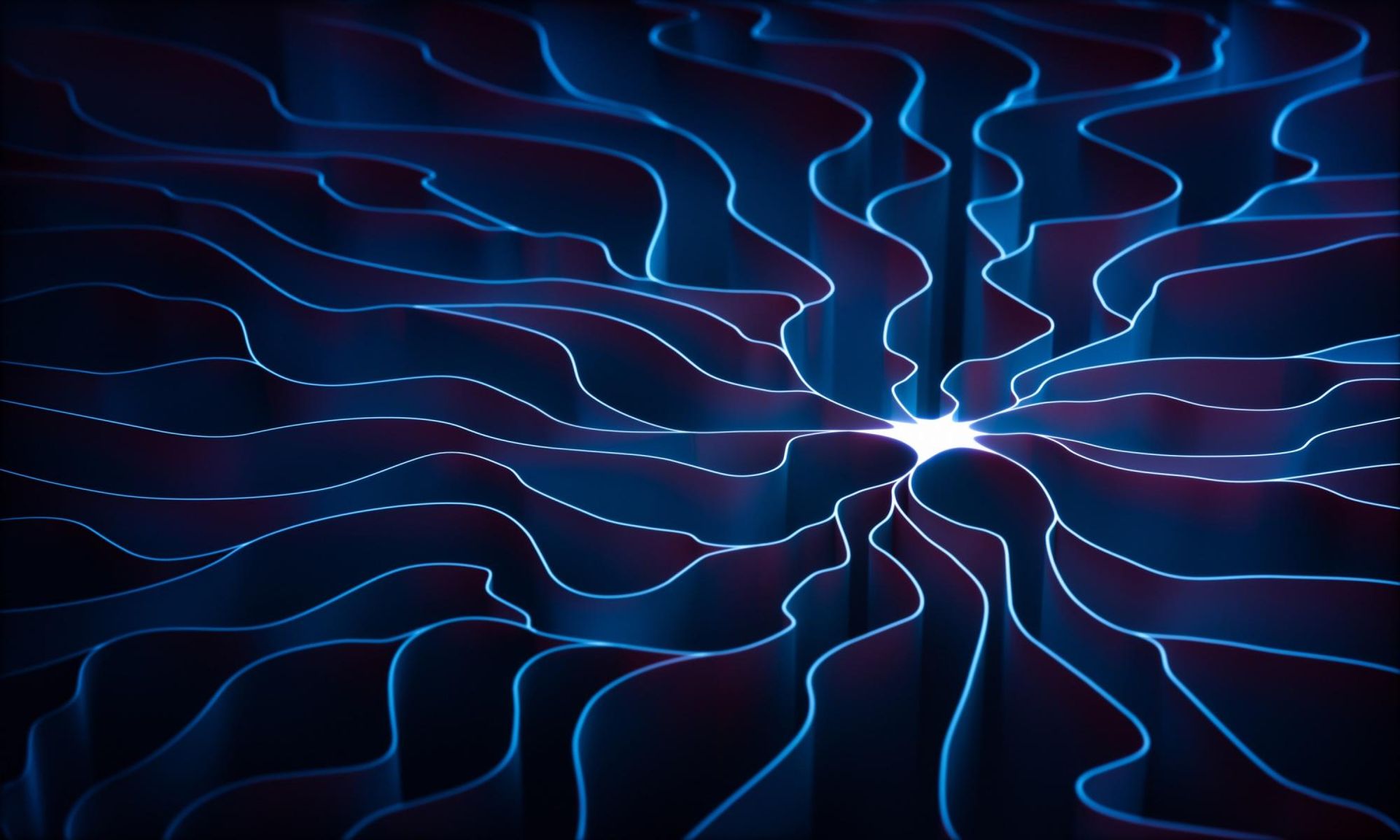
Service
Machine learning consulting
Explore our range of machine learning consulting services, along with related technologies, use cases, implementation roadmap, and payoffs.
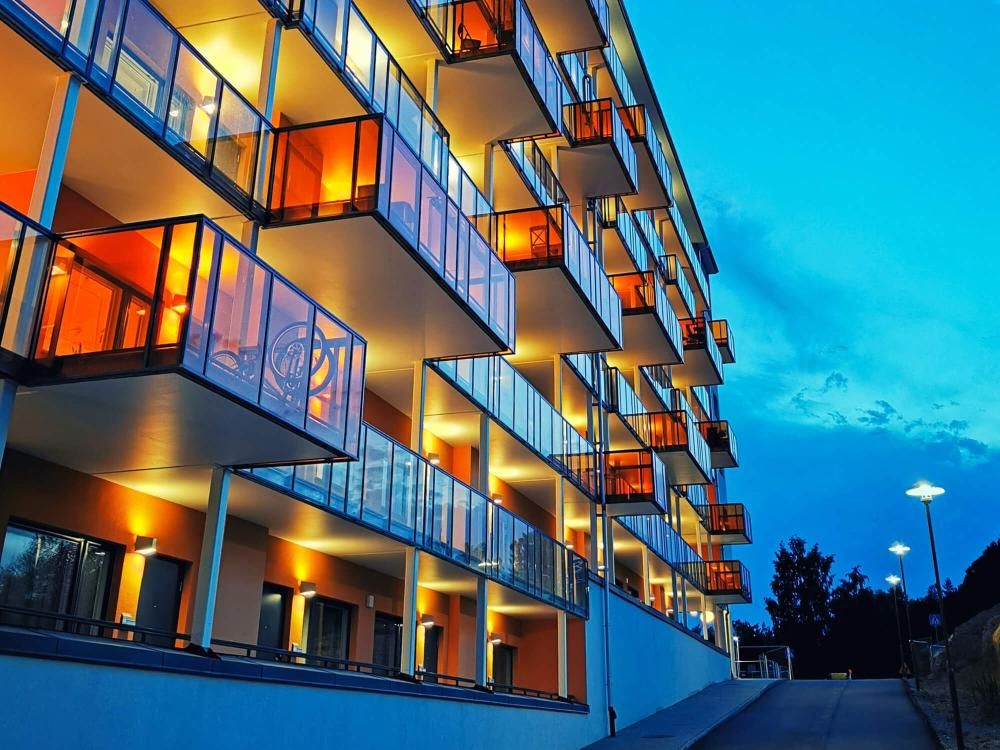
Insights
Machine learning in real estate: use cases, examples, and adoption guidelines
Explore the top trends, use cases, examples, and pros of ML in real estate, along with key adoption challenges and best practices for addressing them.

Insights
Machine learning statistics
Itransition presents the ultimate list of machine learning statistics: market data, ML adoption by industries, economic impact, investments, and other facts.
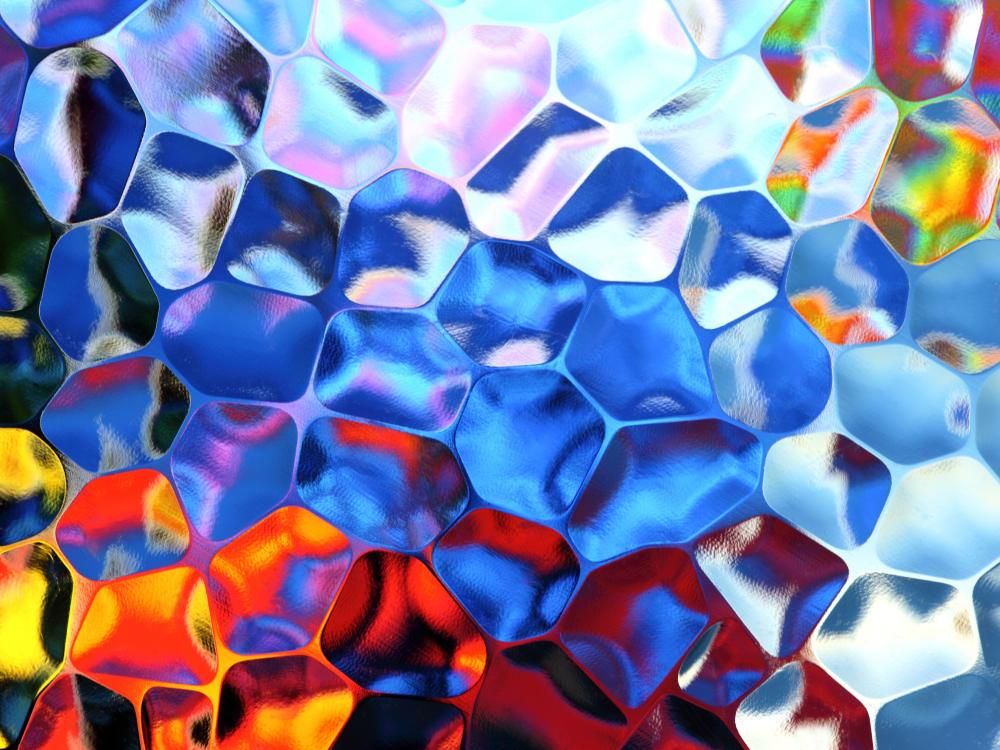
Insights
AI use cases: 3 rules to find the right one for your business
One of the main barriers to AI adoption is identifying the right AI use cases. Find out the best practices to make it less challenging.