Alongside other artificial intelligence technologies such as computer vision and machine learning, natural language processing (NLP) has made its way into the healthcare industry by ensuring medical devices can read, listen, and interact with humans.
Does that mean we've finally found a high-tech solution to doctors' age-old problem of indecipherable handwriting? The use of artificial intelligence services in healthcare, NLP in particular, addresses various tasks:
- Harvesting clinical data spread across thousands of medical records.
- Scanning research results faster than a human eye might ever do.
- Powering virtual nurses and other interactive solutions.
NLP unlocks these and many other possibilities, but before delving into such AI use cases, let's dedicate a few words to the essence of this technology and the actual stage of its implementation.
Good machines, lend me your ears!
Oversimplifying the subject, we may say that computers have always been very talkative when it came to speaking in binary, but they could have a real hard time grasping concepts expressed in the human language. After all, we often don't understand each other, so why should we have expected computers to understand us?
Fortunately, NLP has made great strides in terms of human/machine interaction, specifically in turning the unstructured data typical of our daily communication into structured information usable by computers. Today this fascinating technology at the intersection of computer science, AI, and linguistics has evolved enough to solve these issues brilliantly.
So brilliantly, actually, as to end up turning the communication between us and machines into something taken for granted. Nowadays, we're so used to interacting with a bunch of electronic devices, including smartphones, domestic virtual assistants, and in-car infotainment hardware, that we don’t even notice when we do.
What is not so well-known to the general public, however, is the role played by NLP in healthcare software development. A rapidly growing role, as the statistics show. According to MarketsandMarkets' 2020 Natural Language Processing in Healthcare and Life Sciences Market report, the global NLP market was valued at $1.5 billion in 2020 and is expected to reach $3.7 billion by 2025, with a CAGR of 20.5%.
Speaking of geography, North America is likely to maintain its position as the largest contributor to the global market revenue. On the other hand, the Asia Pacific share may be the fastest-growing one.
The aforementioned study also suggests that the main factors driving this growth will be the spread of predictive modeling in healthcare and the increasing adoption of electronic health records (EHR). In this respect, NLP powers electronic records management software. Regarding data, the implementation of cloud-based solutions combined with NLP tools looks set to grow rapidly, thanks to their ability to ensure easy data maintenance along with the well-known cost-effectiveness and scalability.
The real game-changer: neural networks
How was it possible to achieve such promising results in terms of performance, market revenues, and impact on the modern society? The first significant breakthrough in NLP's evolution was the shift from the old-fashioned rule-based methods (which followed pre-defined sets of rules) to machine learning models relying on statistical inference to autonomously learn human-language rules.
This learning process was carried out by analyzing millions of typical real-world examples collected into large text corpora and searching for recurrent language patterns. Once the machine learning system had been properly trained, it could make probabilistic decisions based on its previous experience regarding, for example, the most suitable answer to a question or the best way to translate a sentence into a different language.
A further step forward was the replacement of statistical methods with neural networks, interconnected layers of nodes called artificial neurons, designed to replicate the structure and functions of the human brain when sending information to each other. Neural networks serve as the foundation of modern machine learning, specifically its latest evolution known as deep learning, and are widely leveraged in NLP solutions nowadays.
Their core strength over statistical techniques is their capacity of predicting the likelihood of word sequences and processing entire sentences in a single integrated model, while statistical approaches relied on strings of separate intermediate tasks.
This makes neural networks a valuable tool for performing all the typical NLP functions, including image recognition powered by OCR algorithms, speech recognition, sentiment analysis, natural language generation (NLG), and many more. Let’s see how some of these top NLP techniques can be applied in the healthcare domain, starting from those related to collecting and managing data.
Have an NLP project in mind?
Partner with Itransition to carry it out
A deeper look into data
When it comes to collecting, processing, and managing massive amounts of data to get precious insight into medical phenomena, NLP is definitely one of the first candidates. Typically combined with computer vision, machine learning, and deep learning technologies, NLP solutions can gather information from different sources such as medical records, clinical case reports, or public health forums.
The ultimate goal? Streamlining the clinical workflow and speeding up pharmaceutical research. Here are some examples in this regard.
Digitalization of clinical documents via OCR
This is the standard method of converting printed or handwritten text into a digital format so that it's made "digestible" by machines. In a sector like healthcare, where many physicians rely on pen and paper to draft various documents (including medical charts, test results, and prescriptions), optical character recognition aka OCR has proved useful for digitizing all this critical information and storing it into clinical databases for future consultation or statistical surveys.
A relevant example comes from the Australian e-Health Research Centre, which is developing an NLP solution to digitalize free-text medical data from pathology reports. The main objective of this initiative is to extract information on cancer cases from paper-based records (which are still the majority in this area of the Australian healthcare system). Such a procedure will allow the national health service to constantly monitor the trends in cancer incidence and enhance its data-driven decision-making.
However, this digitization process will take some time, as most of the global healthcare documentation is still unstructured (around 80%, according to PwC’s estimates) and therefore would remain unused if we did not turn it into a structured format that is recognizable by computers.
Clinical named entity recognition
NLP tools don't just act as scribes copying handwritten documents into virtual databases. Instead, they can also recognize different types of concepts in a text and label them based on their semantic nature. This specific technique is known as named entity recognition (NER) and is commonly adopted in the healthcare field to scan clinical documents or research papers and categorize entities such as treatments, drugs, diagnoses, and so on.
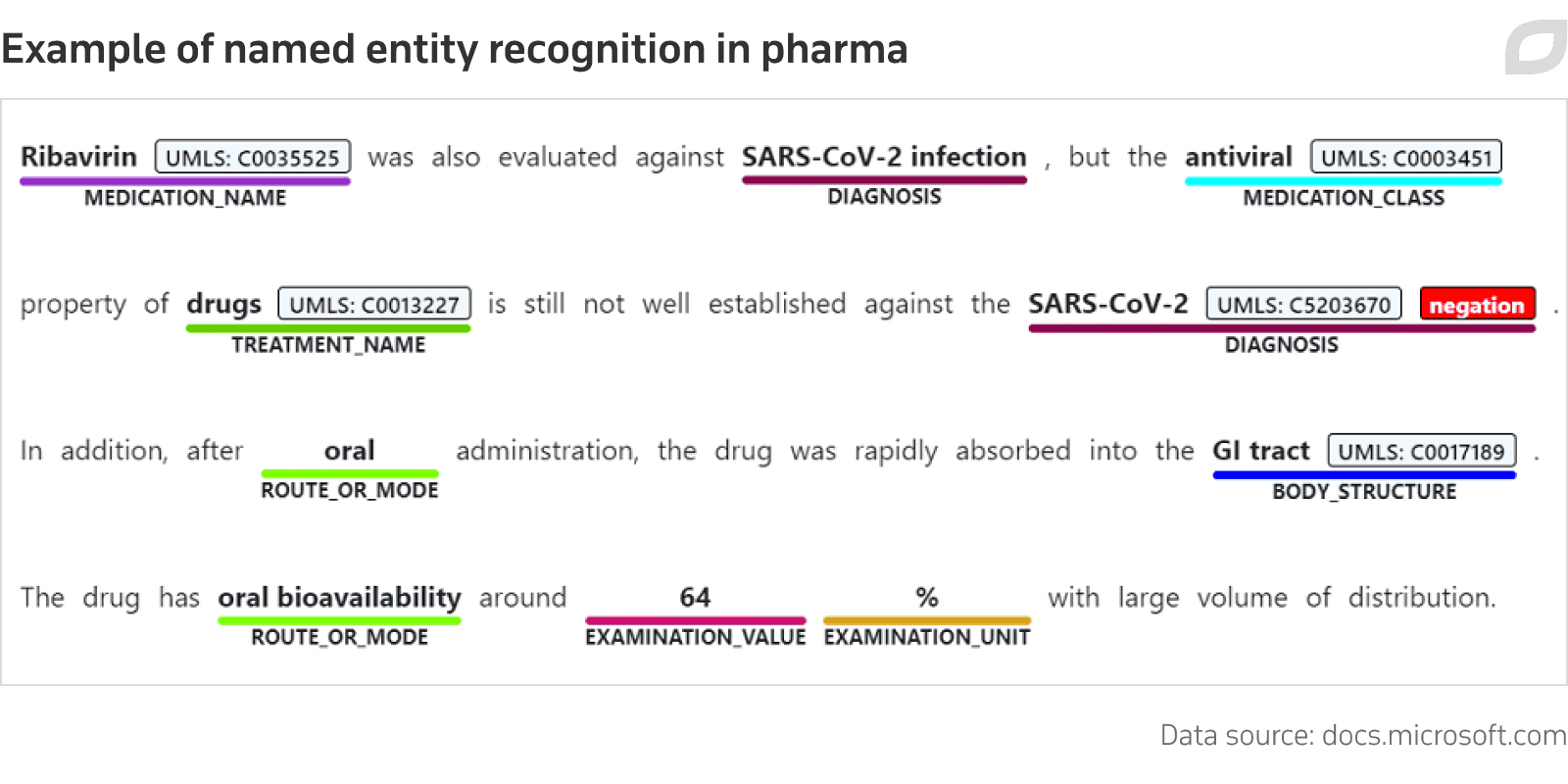
Implementing NER solutions can dramatically enhance patient care by enabling medical staff to streamline the cataloging and cross-search of relevant data across medical records according to associated keywords. A similar procedure can also be followed in research. In a 2018 case study by McKinsey, clinical terms have been classified into ICD-10 diagnosis codes to synthesize clinical guidelines, speeding up the process by 60% compared to standard methods.
Clinical de-identification
The growing traffic of personal data has not gone unnoticed by the watchful eyes of governments and international institutions, which have reacted by imposing increasingly stringent regulations in terms of privacy and data protection—limits that apply even more rigorously in the healthcare industry, for obvious reasons of private data sensitivity.
In this regard, the HIPAA requires healthcare providers to protect patients' medical information from disclosure without their consent or knowledge, with the remarkable exception of data that has been de-identified.
With a mechanism similar to that described for named entity recognition, NLP systems can help solve this issue by scanning medical documents to spot protected health information (PHI) such as patient names and phone numbers. Hence, this sensitive data can be de-identified, namely obfuscated and replaced with tags, in order to avoid any risk of public exposure and privacy violation.
Sentiment analysis in healthcare services
Basically a blend of NLP, machine learning, computational linguistics, and biometrics, sentiment analysis is an advanced technique for evaluating the feelings and opinions expressed in a text, particularly on social media, blogs, and web articles. It’s one of the trending applications of machine learning in the stock market, marketing, and any other sector where it may be essential to get an idea of what customers and investors think about some brand or company.
Anyway, sentiment analysis can also be leveraged in a similar way in the healthcare industry to gather feedback from patients on the quality of medical services, perceived flaws, and potential improvements. This is typically done by looking for keywords with positive or negative overtones and weighing their relevance to the topic under consideration.
Bots, virtual nurses, and other wonders
The aforementioned applications of NLP essentially involve passive reception and processing of human inputs. However, NLP systems can be a lot more chatty than that. Just think of the sheer amount of bots and virtual assistants we interact with on a daily basis thanks to their NLG capabilities.
The healthcare sector makes no exception to this trend. Indeed, it represents one of the major growth drivers for the intelligent virtual assistant (IVA) market, which was valued at $3.4 billion in 2019 and may reach $23 billion by 2027 according to PwC:
Much of this success surely stems from the enormous advances in AI, deep learning, and neural networks, which have pushed the NLP discipline to new, unexplored horizons. By training on huge datasets and interacting with thousands of users in many different situations, bots can learn from experience and hone their communication skills over time, understanding the scenario in which they operate and grasping even the most hidden nuances of speech.
Chatbots and virtual assistants are extremely flexible tools, both in terms of ease of implementation and range of applications. Regarding the first point, they can be developed on the basis of already existing solutions or created from scratch. Moreover, they are easily scalable and can be "powered-up" by infusing them with different technologies according to their purpose.
As for the second point, these solutions can be deployed in a huge variety of AI use cases. However, we may classify all of these applications under two sub-groups representing the two main reasons for adopting NLP-powered chatbots.
1. Streamlining clinical processes
The pressure faced by healthcare professionals during the recent COVID-19 crisis has once again demonstrated the need to optimize workflows in hospitals and other medical facilities. In this regard, one of the most effective solutions is to delegate an increasing amount of tasks to bots, allowing healthcare professionals to focus on the human relationship with patients.
For example, the Providence St. Joseph Health system in Seattle has implemented an AI-powered bot with NLP capabilities to alleviate its hotline traffic volume during the pandemic. This tool can ask patients questions to define their symptoms and the actual urgency of each clinical picture. Then, it can reassure or advise those who don't need additional care and redirect riskier cases to the appropriate medical services, such as clinics and testing sites.
2. Enhancing the patient experience
"Tempus fugit," as the ancient Romans would say. But nowadays, time flies faster than ever and patients expect flexible and possibly 24/7 healthcare services. Luckily, bots can help speed up many bureaucratic procedures by unlocking self-service payments, online doctor bookings, and so on.
Furthermore, they can be used to tirelessly assist patients in need. The British National Health Service, for example, is testing a virtual nurse called Molly to monitor people with chronic diseases, answer their questions and assess their symptoms. According to the Harvard Business Review, these tools can save $20 billion annually by reducing the time nurses spend on patient management by 20%.
Consult our experts to implement NLP in healthcare the right way
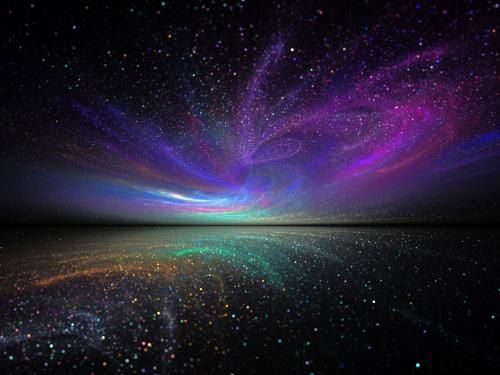
Artificial intelligence services
Our AI consultants can help you decide on the right AI solution for your use case, select suitable technologies, and implement it into your enterprise ecosystem.
Keeping an eye on the human side
NLP is likely to be a major factor in reshaping the healthcare sector. However, to unlock its full potential in enhancing patient experience and supporting healthcare professionals, this powerful tool should be in the right hands. Specifically, it should not replace human professionals but work alongside them and be properly supervised. This oversight, as you may expect, requires physicians and medical staff in general to know the technology they're dealing with.
That's why, alongside the mere deployment of new tech, it will be essential to promote the right professional culture (also via training and reskilling) and work on the integration between professionals, devices, and data. This last aspect, namely data, leads us to another potential pain point that is worth mentioning.
Knowledge is power, use it wisely
NLP, like any other AI-related technology, resembles a two-faced Janus when it comes to managing data. Because while it is true, as explained above, that NLP solutions can be implemented to hide sensitive patient information, at the same time we shouldn't forget that any machine learning-based system developed for healthcare must be trained on medical data.
Not to mention that this data may be processed in mysterious ways, as machine learning algorithms are typically affected by the well-known black box problem, when the way they come to their conclusions is obscured.
NLP and machine learning solutions should be developed with these aspects in mind, setting a general framework to collect and leverage healthcare data but also to secure it against cyberattacks. That's not just a question of compliance with personal data regulation but also a matter of ethics and respect for patients, who should always be the focal point of healthcare more than anything or anyone else.
After all, the ancient Hippocratic Oath recited "Whatsoever I shall see or hear in the course of my profession, I will never divulge, holding such things to be holy secrets." Centuries have passed, but this maxim still stands, even when we’re dealing with a technology like NLP that Hippocrates would have mistaken for magic.