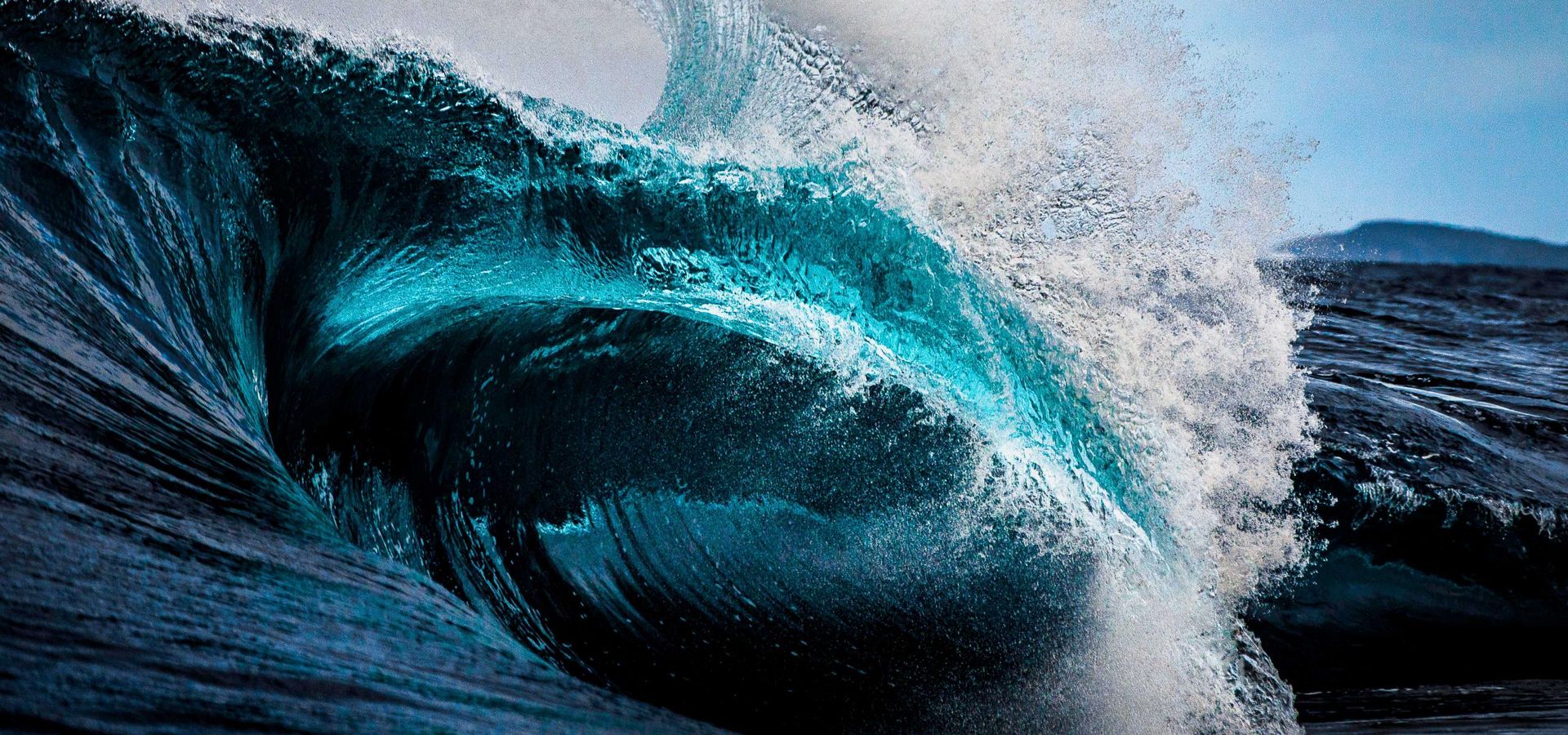
ML PoC for aquatic environment analysis
Itransition delivered a PoC solution for plankton detection and classification, proving the feasibility of the suggested ML approach.
Context
Our customer is a US-based company, created by a group of scientists and engineers to design and build advanced underwater measurement and observation systems that can operate in the most severe ocean environments. Striving to advance the knowledge of our planet’s aquatic environments, they built integrated instrumentation platforms to observe all kinds of physical and chemical parameters in real-time. From this data, scientists and companies from various industries get a better understanding of complex dependencies in ecosystems and make informed decisions.
One of the issues the customer addresses is understanding plankton composition in ocean water through acquiring data on plankton quantity and types. The detection, extraction, and classification activities were based on a supervised learning model involving convolutional neural networks (CNN). The activities happened in real time on their NVIDIA Jetson embedded AI computing device, which is coupled with a machine vision camera. Their self-developed plankton detection and recognition software based on C++ limited the image processing speed to 8-10 FPS, though the camera's maximum possible speed was 30 FPS. Therefore, the accuracy of plankton detection and recognition, important to the business, was unsatisfying, as the system employed older versions of CNN algorithms.
Considering the challenges the customer faced, they wanted to make the following changes:
- Improve or redesign the detection and classification approach to meet the hardware needs, ensuring the app’s full compatibility with their computing device and camera and align image processing speed to the camera’s speed
- Take full advantage of the hardware’s possibilities, improving the accuracy of the detection and classification processes
The customer was searching for a trusted and competent partner that would take up the project and deliver a robust solution to improve the company’s digital performance. In the end, they turned to Itransition, as we proved to be the right match considering our strong ML consulting expertise and experience with solving similar challenges.
Solution
Analytics
Based on the provided documentation and the code file, our experts concluded that there were two possible ways to achieve the customer’s goal:
- Improve the existing solution
- Come up with a more efficient approach and develop a new solution from scratch
As for improvements to the current solution, our experts prepared a list of recommendations to speed up code and algorithm execution. Still, we and the customer eventually decided that developing a completely new system with a new architecture and selected technology stack was a much faster and easier-to-maintain option. It would also bring the following positive changes:
- Perform most of the operations using the solution’s RAM instead of working with files, reducing execution time and accelerating the solution’s performance
- Enable multi-thread processing (i.e. separating each process into separate threads working independently from other functionalities), which would also speed up the execution time
- Apply modern ML algorithms to improve the accuracy of the customer’s detection and classification process
New solution vision and investigation
According to the proposed approach, the solution detects objects in images using the YOLO object detection system. Then the EfficientNet CNN is applied to the obtained results, creating a vector for each detected object. After that, the solution compares the created vectors to the detected objects against the reference ones, leveraging the Faiss or Annoy algorithm, and chooses based on the minimum distance between them.
We opted for Python as the main programming language because of its popularity and quicker and easier development compared to previously used C++, which would still deliver comparable performance results.
For training the detection and classification models, we considered using Tensorflow, PyTorch, and Darknet, an open-source neural network framework written in C and CUDA. Initially, we wanted to ensure the uniformity of the leveraged libraries and utilize Tensorflow for both cases, especially considering that Darknet could be easily converted to Tensorflow. This would ensure model type consistency because using one framework reduces possible risks. Both Tensorflow and Darknet could work with Jetson CPU, but you can get the maximum performance boost when they work with the device’s GPU.
However, after testing the frameworks on the customer’s Jetson module, we found out that Tensorflow was unable to work with GPU properly given the module’s non-standard ARM architecture. As it was impossible to make the most out of Tensorflow capabilities, we discarded it in favor of Darknet for the detection process. For training the classification model, we chose PyTorch as it performed well on the customer’s machine and, unlike Tensorflow, could leverage its GPU resources.
When deciding which algorithm to apply for the Nearest Neighbor Search (NNS) process, we were choosing between Annoy and Faiss. We tested both options and decided that Faiss would be a much better solution considering the customer’s company specifics and a relatively small number of values (30+ classes with 100 images for each class).
PoC development
To evaluate the new plankton detection and recognition process and fine-tune the solution before development, Itransition suggested creating a Proof of Concept (PoC). A PoC would have allowed us to elaborate on implementation details, solve potential issues, meet the customer’s specific technology requirements, and agree on the most suitable technical approach and budget. Before the start of PoC development, the customer:
- Provided two marked-up datasets for detection and classification to use for a vectorization reference model creation. We will then further use this reference to analyze and compare data with the Faiss algorithm.
- Allowed us to remotely and securely (via SSH access) run the new software on their machine to reduce communication and data exchange time and ensure the solution’s compatibility with both their computing device and the camera.
The main purpose of the PoC was to ensure satisfactory interoperability of the new app and the customer’s hardware. We also wanted to try out the selected CNN algorithms and determine whether the level of accuracy they provide would be sufficient. To achieve the set PoC phase goals, our team performed the following activities:
- Design the architecture suitable for the customer’s hardware, particularly the modules that the solution should include to carry out the expected detection and classification processes
- Prepare reference data for models training, remove the duplicate, broken, or blurry images from the reference dataset, and convert images to a unified format for the frameworks
- Implement solution modules and install new software on the customer’s machine
- Set up a camera connection using the PyCapture library and enable the solution to get frames from it and save them in RAM
- Check and enable running new software on the customer's machine GPU
- Train the YOLO CNN using Darknet for plankton detection based on around 1500 images
- Train the EfficientNet CNN using PyTorch to encode the images with detected planktons and feature vectors based on the reference images for classification (around 8000 images grouped into 30+ classes)
- Choose and implement the plankton classification algorithm based on the NNS approach using the Faiss algorithm
- Create functionality for saving final plankton images as BMPs and statistics in the format approved by the customer
- Test the solution on the customer's hardware and take necessary quality and performance measurements at the runtime and find ways to improve the maximum processing speed and models accuracy in the current setup given a tight timeframe and limited budget
Process
Our project team consisted of a project manager and backend and ML engineers. Also, a developer from the customer’s side got involved if we had blockers while working with the customer’s machine. We had weekly meetings where we made go/no-go decisions, sent the customer weekly updates, timely notified them about blockers, and invited them to demos.
Since the PoC development project had many unknowns, we defined and decomposed the project scope, identified the main milestones, and determined their deadlines for easier, more efficient and manageable activities planning. Before the development began, we also created an architecture diagram and approved it with the customer.
Throughout the project, we carried out app testing on the customer’s equipment to make sure the solution’s modules worked properly locally as well as deployed on their machine. For this, we launched the solution, viewed logs, got performance metrics for each module, collected statistics, and made changes and improvements if needed. At first, we tested the solution on our systems with images from the folder and later connected to the camera and tested the system using a live video stream.
During PoC development, we adapted our work pace in line with the encountered complexities, such as selecting the necessary libraries for deploying the app on the customer’s machine and connecting it to the camera or choosing the frameworks for model training. We also measured the speed of the solution’s various components to identify bottlenecks and optimize them.
Challenges
Challenge |
Description |
Solution |
---|---|---|
New software installation and environment setup on the customer's machine |
To run the application, suitable libraries and packages had to be installed, since NVIDIA Jetson is of a non-standard ARM architecture. We faced multiple obstacles while installing libraries because not all versions were supported. |
We researched and installed the corresponding libraries (Numpy and Pillow for processing multidimensional arrays, OpenCV for resizing images, etc.) suitable for the customer’s machine. We set up the environment so that we could use these libraries, as they were crucial for image processing. We tested different versions of libraries to identify which version works best on the customer’s device and ensured optimal configurations of the libraries and the device itself. |
Establishing camera connection |
We needed to figure out which libraries and their versions were supported by the camera. |
We researched libraries and found the versions suitable for the FLIR Grasshopper camera and the developed solution. To enable reading frames from the camera, we applied the PyCapture library. |
Performance optimization |
While measuring necessary performance metrics, we found several bottlenecks:
|
We found alternative ways to convert frames to a suitable format and improve the solution’s performance.
|
Setting up detection and vectorization on the customer's machine GPU |
We found out that TensorFlow utilized GPU poorly and needed too much RAM. |
We decided to stick to Darknet and PyTorch for detection and vectorization correspondingly, as they run faster and utilize resources better. |
Architectural design |
The available RAM caused app queue limitations and required a thoughtful solution architecture design. |
We experimented with the number of queue entities and their size to define whether the solution would work properly without freezing and crashing. We also designed the architecture that allows the app to optimize RAM use. |
Detection and classification
The delivered solution includes the following modules:
- Object detection – the YOLO model enables searching and finding all object types on an image
- Vectorization – EfficientNet executes the vectorization process for all detected objects
- Class search (NNS) – the Faiss search algorithm allows for distinguishing the most similar vectors to a given vector based on the reference dataset
- Statistics – collecting all the information necessary for outcomes analysis
Index creation
This step is performed before the main classification step because the solution receives the class of the found plankton using the Faiss index and the JSON file during the “Class search” step:
- Creating the Faiss index, which contains ID and vector bundles for each image from the reference dataset.
- Creating a JSON file, which contains ID and class bundles for each image from the reference dataset.
Object detection
Frames grabbing
There are two sources to get frames from – the folder with images and the camera:
- The folder with pictures
The system opens an image from the specified folder and adds it to the detection queue. - The camera video stream
The system finds the camera, connects to it, and prepares it according to the defined settings. Then, frame capture starts and approximately every 100 ms (10 FPS) a callback function is triggered, receiving an image in the PyCapture image format. The system converts the image into numpy.ndarray (a three-dimensional array type) to enable working with it later and adds it to the detection queue.
Detection
- Getting a frame from the detection queue and scaling it up to 416x416 as per model requirements to increase execution speed by using less RAM without compromising accuracy.
- Detecting plankton on the compressed image, getting a list of bounding box values specifying the position of the found object (x and y coordinates of the center, width, and height).
- Adding the images with the found plankton to the vectorization queue.
Classification
Vectorization
- Getting an image with a plankton object from the vectorization queue and scaling it to the predetermined 224x224 format
- Image normalization to the compatible format to allow EfficientNet to correctly process the image
- Converting the image to the vector format, representing the list of 672 float elements
- Adding the vector with a plankton object to the class search queue
Class search
- Getting an image vector with the identified plankton object from the class search queue.
- Searching for the most similar five vectors in the Faiss index queue utilizing the NNS algorithm. As a result, there is a list of neighbor IDs and a list of distances from them to the given vector.
- Selecting the most frequent IDs from the list of nearest neighbors and choosing the reference vector, the distance to which is minimal because it will be the most similar to the detected object.
- Determining the plankton class name using the JSON file from the Faiss index that stores ID and class bundles.
- Adding the bounding box with an identified plankton object class to the statistics collection queue.
Statistics
The statistics module is responsible for gathering all the information collected at the runtime based on the data found. After the thread is initialized, the following actions take place:
- Getting a bounding box with a plankton object from the statistics collection queue.
- Recording results in the TXT file, including the ID, timestamp, bounding box (x_min, y_min, x_max, y_max), detection confidence, and a class of each found plankton.
- Saving the found plankton as a BMP image.
Improvements roadmaps
Itransition defined the next improvement steps for the PoC solution to increase the solution’s performance and the accuracy of detection and classification models. For model accuracy, we suggested the following:
- Using one dataset instead of two marked up for both detection and classification. This would unify the variety of reference examples for both processes and help better understand the location of possible bottlenecks and drawbacks.
- Training the detection model on a larger dataset and implementing a focus calculation algorithm, i.e. a strict algorithm that defines whether an object is in focus or out of focus (currently decided by the CNN). We proposed to do research, come up with the most optimal options, and implement the algorithm.
- Implementing a new approach to speed up the detection process, according to which all plankton, whether in focus or not, is detected. After that, a filter based on a predefined rule defines whether objects are in focus or not, with the latter removed afterward.
- Training the classification model on a dataset containing more classes and more images per class.
To increase processing speed, we suggested:
- Exploring the possibilities of running Faiss on GPU.
- Redesigning the detection and classification model architectures so that they can use a batch of images at the same time and give a 10-15% speed boost without affecting RAM.
- Considering the replacement of the current YOLOv4 model with a newer YOLOv7 released on PyTorch. It would allow the customer to significantly improve the solution’s speed and models’ accuracy on the current hardware with the same amount of RAM and computing resources. The company would also unify the leveraged libraries by replacing Darknet with PyTorch.
At the end of PoC development and after the final demo with the customer, we provided executable files and instructions on how to run the app. After the customer has sufficiently used the solution in the real environment and collected data, we will develop a full-scale software suite.
Results
Itransition delivered a PoC solution for plankton detection and classification. The PoC allowed the customer to test the quality and performance of the approach we suggested without investing in a full-scale solution. By implementing the PoC, we proved the feasibility of the suggested approach and achieved the set goals:
- Ensured the compatibility of the customer’s computing device and camera with the new software
- Increased image processing speed from 8 FPS to about 15 FPS
- Achieved a 98% accuracy of the detection and classification models
- Compiled a list of steps to improve the accuracy of detection and recognition models and overall solution performance
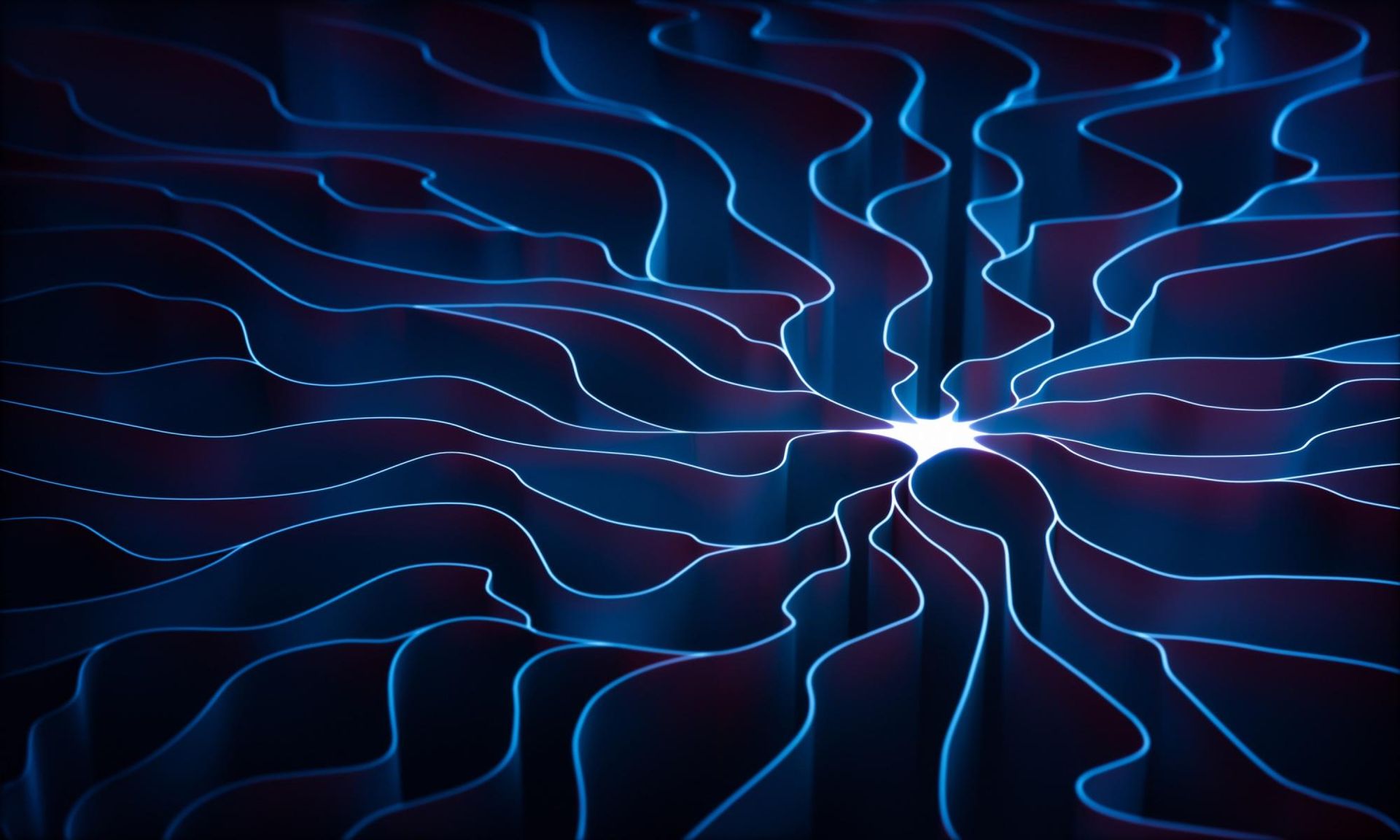
Services
Machine learning consulting services & solutions we deliver
Explore our range of machine learning consulting services, along with related technologies, use cases, implementation roadmap, and payoffs.
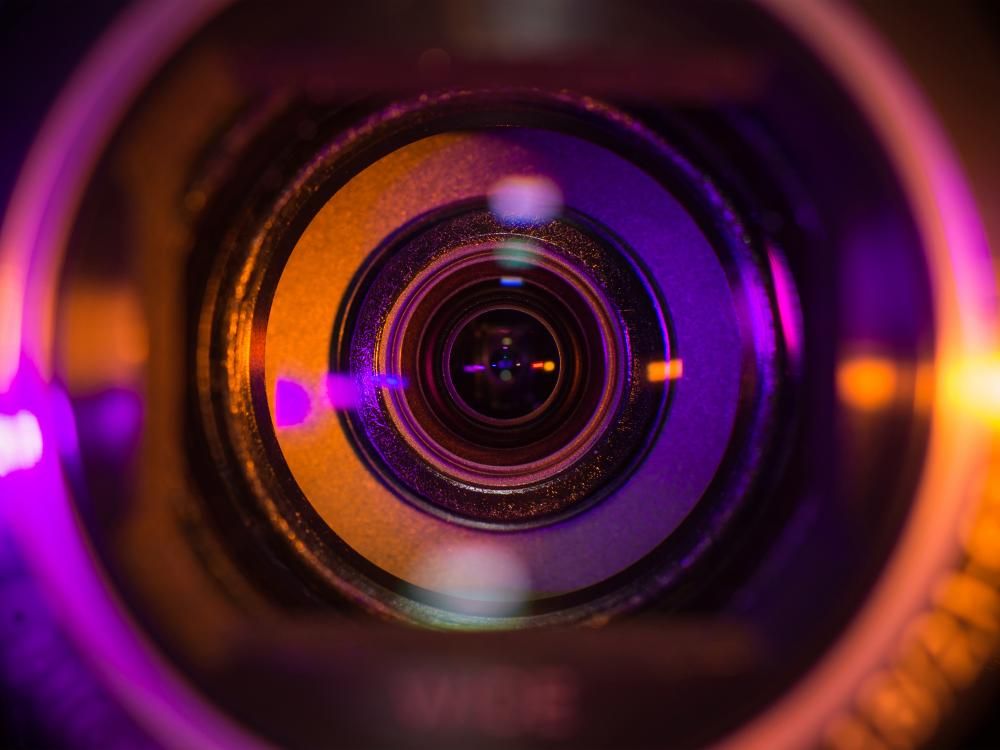
Case study
A shoppable video platform for AiBUY
Find out how Itransition’s dedicated team helped AiBUY release their innovative machine learning-driven shoppable video platform.
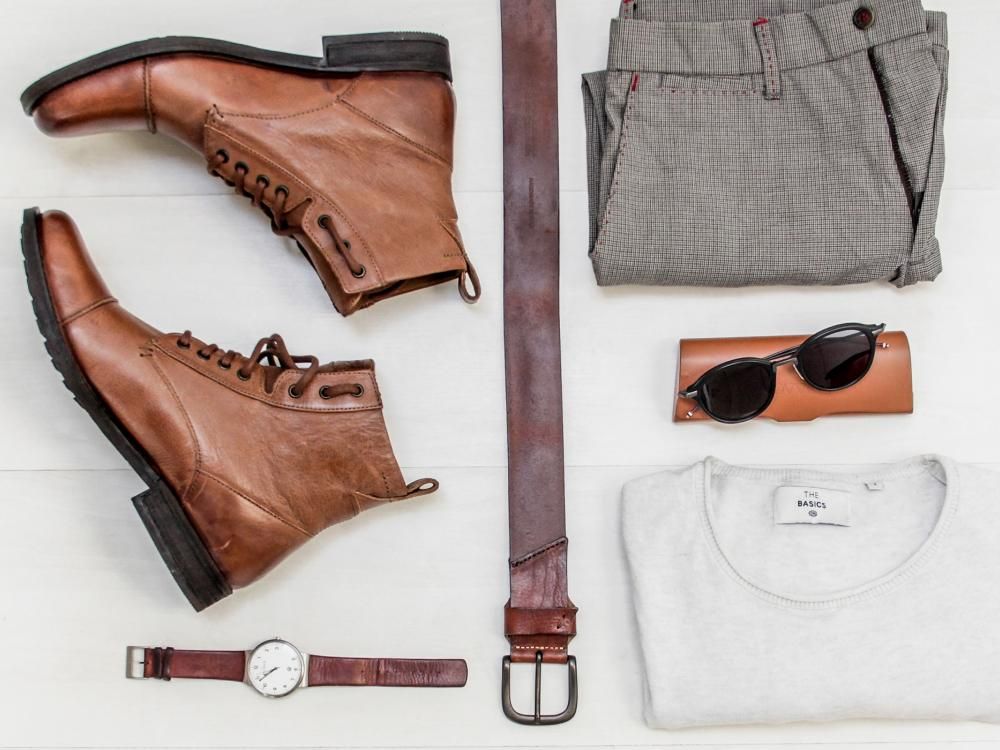
Case study
BI platform with AI and computer vision for a fashion retailer
Learn how Itransition delivered retail BI and deployed an ML-based customer analytics solution now processing 10TB of data.
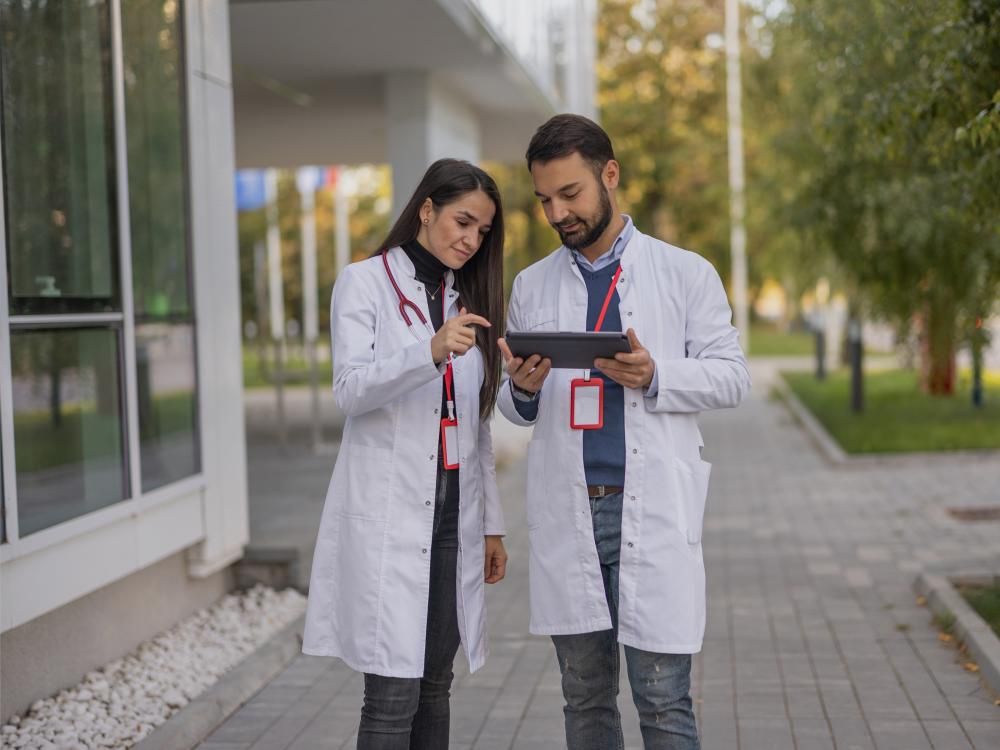
Case study
Clinical data exchange PoC
Learn how we developed a PoC of a secure clinical data exchange app for paramedics which has already passed beta testing and attracted 30+ new clients.
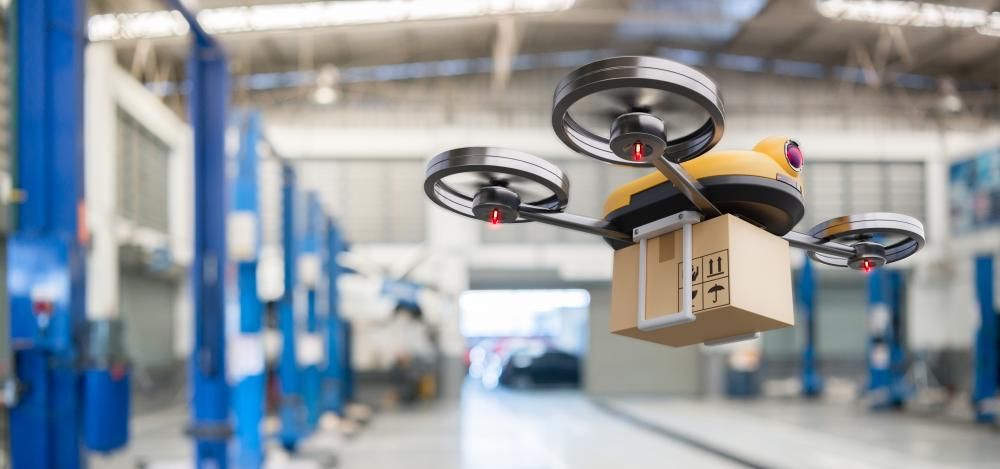
Case study
A POC application for a drone delivery startup
Read how we developed a POC Android mobile application that facilitates contactless drone delivery, helping the customer prove the product's feasibility.
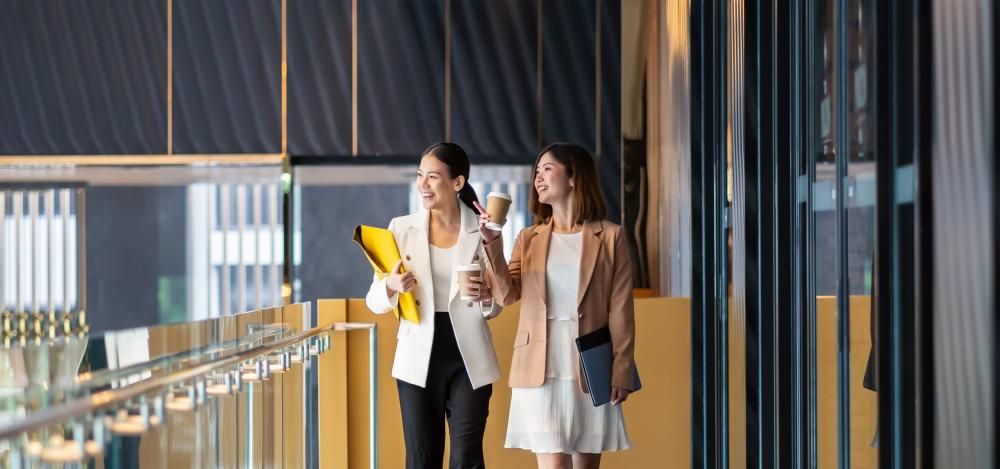
Case study
Robotic process automation HR PoC
Learn how Itransition developed an RPA bot that automates addition of new candidates to the HRM, significantly increasing process efficiency and reliability.