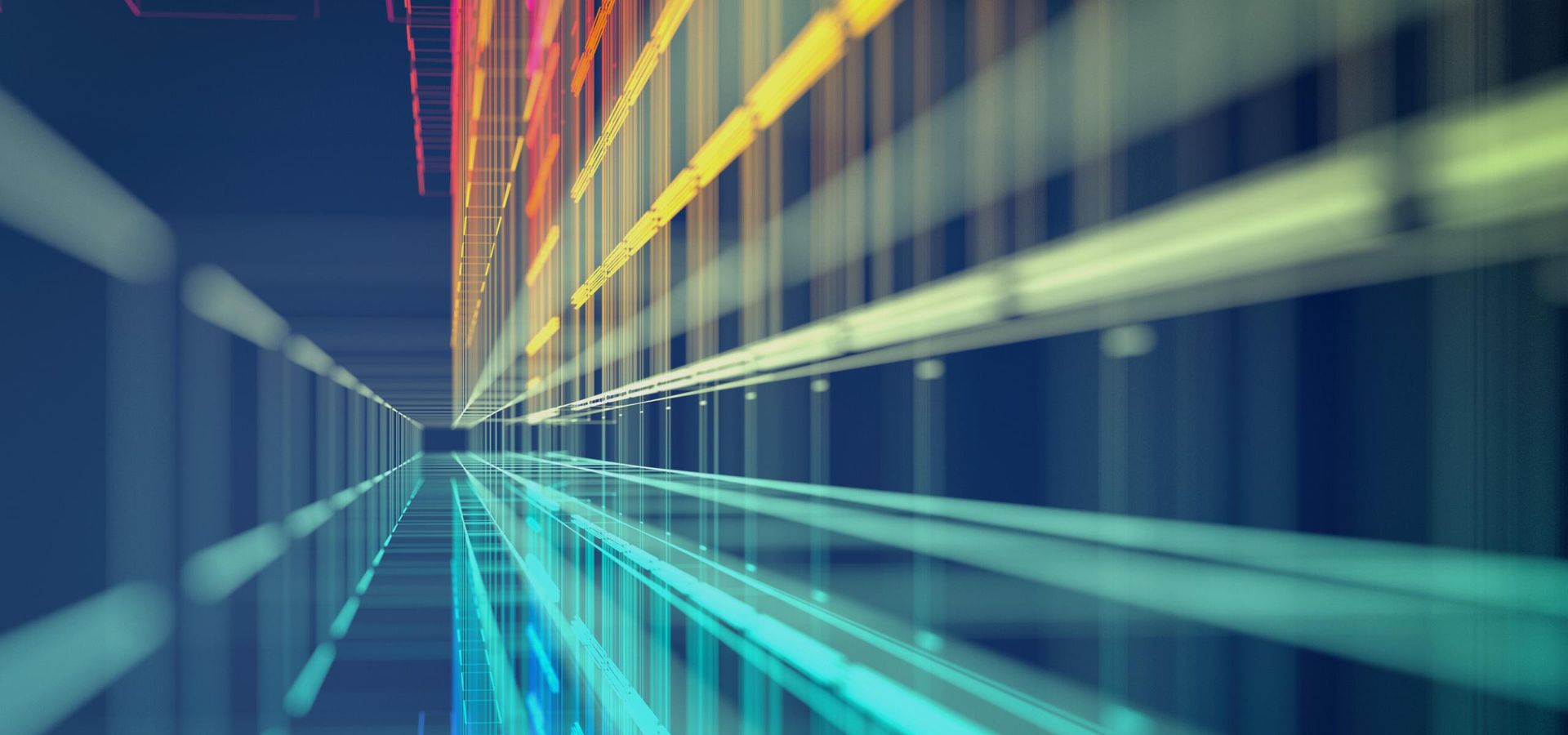
Computer vision in manufacturing: 9 use cases, examples, and best practices
May 23, 2023
- Home
- Computer vision in manufacturing
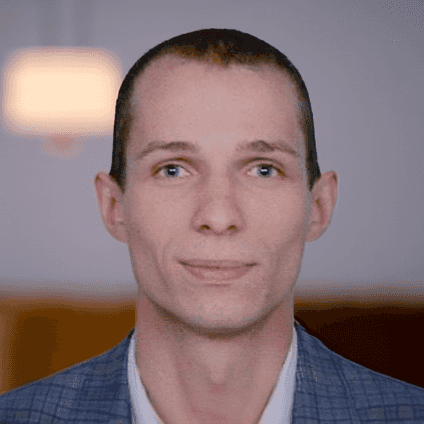
Head of AI/ML Center of Excellence
Computer vision (CV) in manufacturing involves AI-powered systems that can capture, analyze, and understand visual inputs from the physical world (such as factories and other industrial sites) to help humans take proper actions and assist them in various production processes.
Rely on Itransition's computer vision consulting and implementation services to fully seize the benefits of this technology in your business scenario.
Computer vision in manufacturing: a market overview
Computer vision technology and the manufacturing sector look set to develop closer ties in the years to come.
of manufacturers consider computer vision important for meeting their business goals
IBM, 2021
of the global computer vision market is covered by its industrial segment alone
Grand View Research, 2021
CV market CAGR 2023-2030, with manufacturing as one of its fastest-growing segments
Mordor Intelligence, 2023
Cybersecurity
Advanced data analytics including predictive/prescriptive analytics
Automation/robotics
IIoT/IoT data from devices
Artificial intelligence (AI) and machine learning (ML)
Computer vision
Autonomous systems
Augmented/virtual/mixed reality
Next generation ERP systems
Track and trace for supply chain visibility
Scheme title: Top 10 technologies helping manufacturers meet their business goals
Data source: ibm.com — 2021 Digital transformation assessment Covid-19: a catalyst for change
Step up your productivity with Itransition's computer vision solutions
An example of a manufacturing-oriented computer vision system
A study by the Institute of Electrical and Electronics Engineers suggested a potential system framework to integrate computer vision into your existing manufacturing environment. These are its key components and related processes:
Scheme title: Main components of a computer vision system for manufacturing
Data source: IEEE — Computer vision techniques in manufacturing, 2022
Lighting module
Provides the sensing module with uniform illumination and enables it to capture clear images of the manufacturing system.
Manufacturing system
Encompasses assembly lines, assembly robots, and automated guided vehicles.
Sensing module
Comprises cameras that collect visual data from the manufacturing system and send it to the CV system via the Internet of Things (IoT).
Computer vision system
Typically powered by machine learning (ML) algorithms, it can perform different tasks from product counting to execution quality control with defect (anomaly) detection.
Decision-making module
Processes the previous results with rule-based or more complex artificial intelligence algorithms to trigger a suitable course of action.
Actuator
A set of robots that interact with the manufacturing system according to the strategies defined by the decision-making module.
9 use cases of computer vision in manufacturing
Computer vision systems can be deployed in many use cases and operational scenarios within manufacturing processes.
1 Quality inspection
Combined with ML-powered anomaly detection, computer vision enables automated visual inspection to identify defective products with any type of anomaly (e.g. scratches or dents).
2 Assembly process automation
3 Packaging inspection
4 Object detection and recognition
5 Compliance monitoring
6 Sorting and counting
7 Dimensional measurement
8 3D vision and design
9 Supply chain traceability
Seize the business opportunities unlocked by computer vision
Examples of computer vision in manufacturing
Here are a few real-life examples of manufacturing companies leveraging computer vision in a variety of processes:
Dow Chemical
Volvo
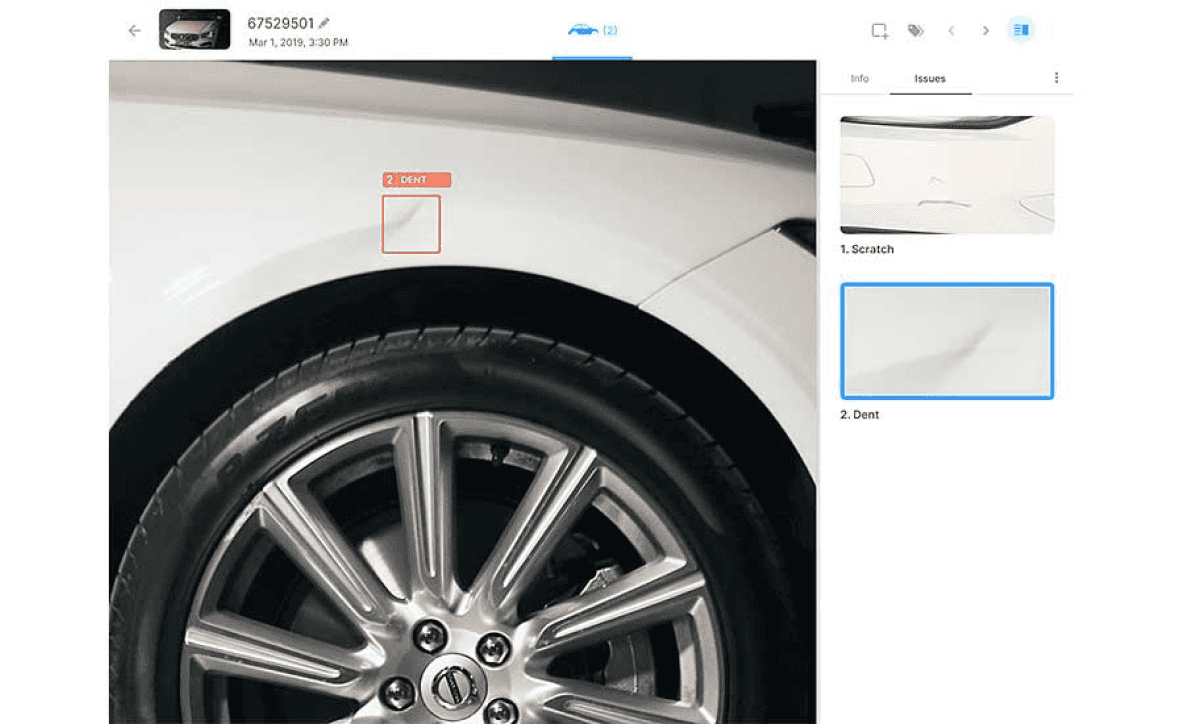
Image title: Volvo Cars’ visual inspection system Atlas
Data source: assemblymag.com—AI-based vision technology aids vehicle inspection
Komatsu
Tennplasco
Benefits of computer vision in manufacturing
The adoption of computer vision systems in industrial processes can strengthen the manufacturing sector in terms of:
Greater productivity
According to Deloitte, adopting computer vision, automation, and other smart factory initiatives accelerates manufacturing cycles, resulting in a 12% growth in labor productivity and 10% in total production output.
Cost optimization
Increased productivity and minimized machine downtime via automation and computer vision-based maintenance (up to 50%, based on McKinsey's estimates) translate into a general reduction in operating costs.
Improved quality
Computer vision-driven robots operate with surgical precision, ensuring better product quality, minimized human error, and an overall reduction of 10-20% in QA operations cost, as reported by McKinsey.
Workforce safety
Deloitte highlighted that computer vision systems in manufacturing enable organizations to identify dangerous malfunctions, monitor workers' conditions, and detect any sign of fatigue or discomfort.
Computer vision adoption roadmap for manufacturers
Implementing an ML-based computer vision solution in a manufacturing scenario will involve business-oriented and technical steps. Here’s a brief rundown of the main adoption stages.
1
Business needs analysis
Analyze customer business needs and expectationsÂ
Assess the current tech ecosystem
Frame corporate needs and goals
Define the solution’s scope and requirements
2
Initial data analysis
Map and assess corporate data sources
Identify external data sources (public databases, etc.)
3
Solution design
Design the solution’s architecture
Set up a project plan, including budget and timeline
Identify a suitable tech stack
Define the scope of a PoC, if required
4
Building the solution
Perform data cleansing, annotation, and transformation
Define the solution’s assessment criteria
Process data with algorithms
Build a CV model trained to recognize patterns
5
Integration and rollout
Integrate the CV model into the solution
Deploy the product in the target environment
6
Support
Fine-tune the CV model with new data
Perform ongoing maintenance, updates, and fixes
Computer vision adoption challenges and best practices
When implementing computer vision in manufacturing, you can face some technical challenges. Keep in mind the following recommendations to streamline its adoption.
Integration
Integration
Processing
Processing
Security
Security
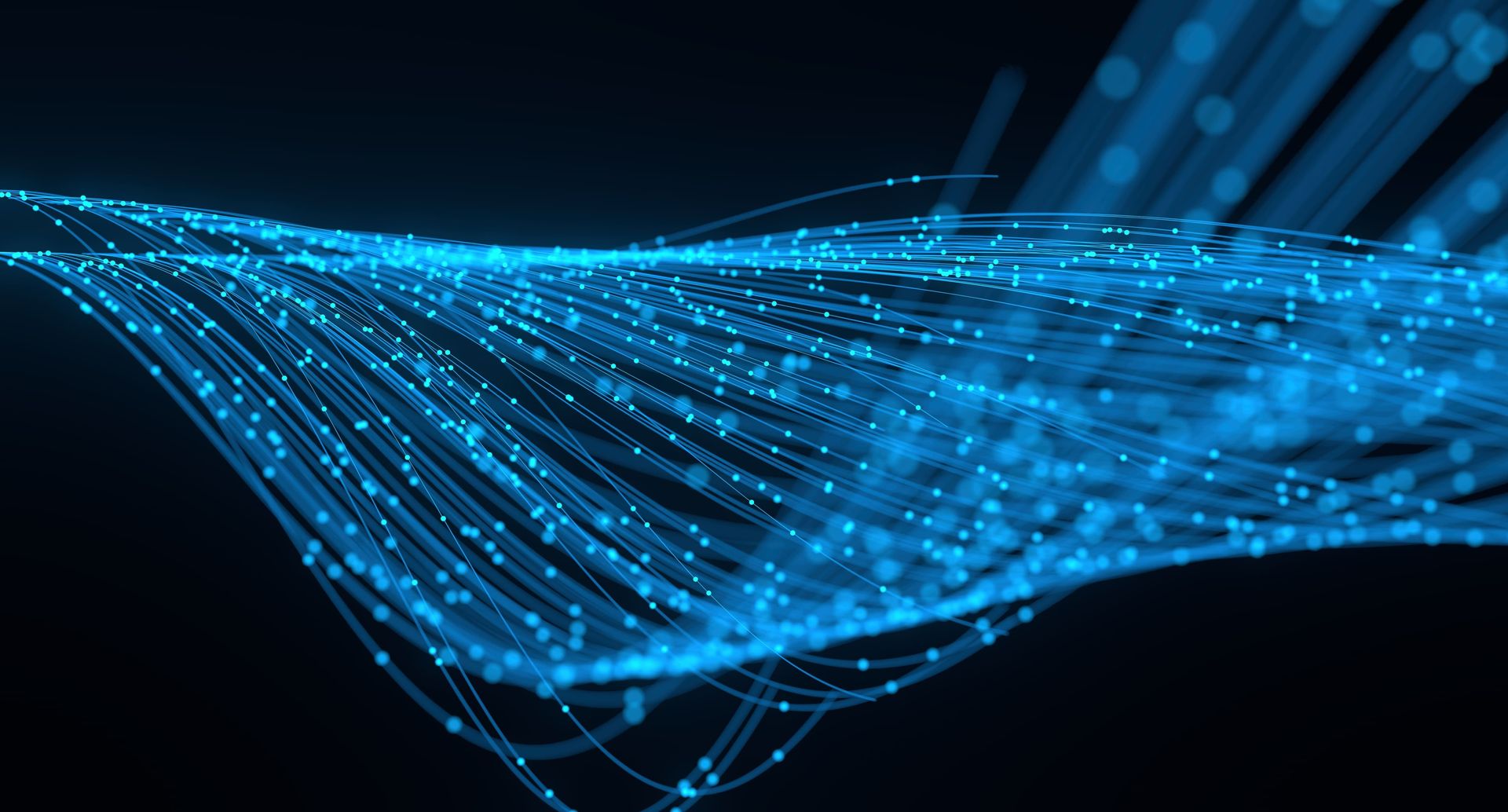
A journey towards Industry 4.0
Along with other technologies driving digital transformation in manufacturing and the shift towards the Industry 4.0 model, computer vision proved to be a valuable ally for this sector. Indeed, its implementation has often resulted in significant cost cuts, superior quality control, higher production output, and increased worker safety.
To reap the full benefits of this technology, however, companies will need an upgraded tech ecosystem, a solid data management and integration strategy, and the expert guidance of a trusted partner like Itransition.
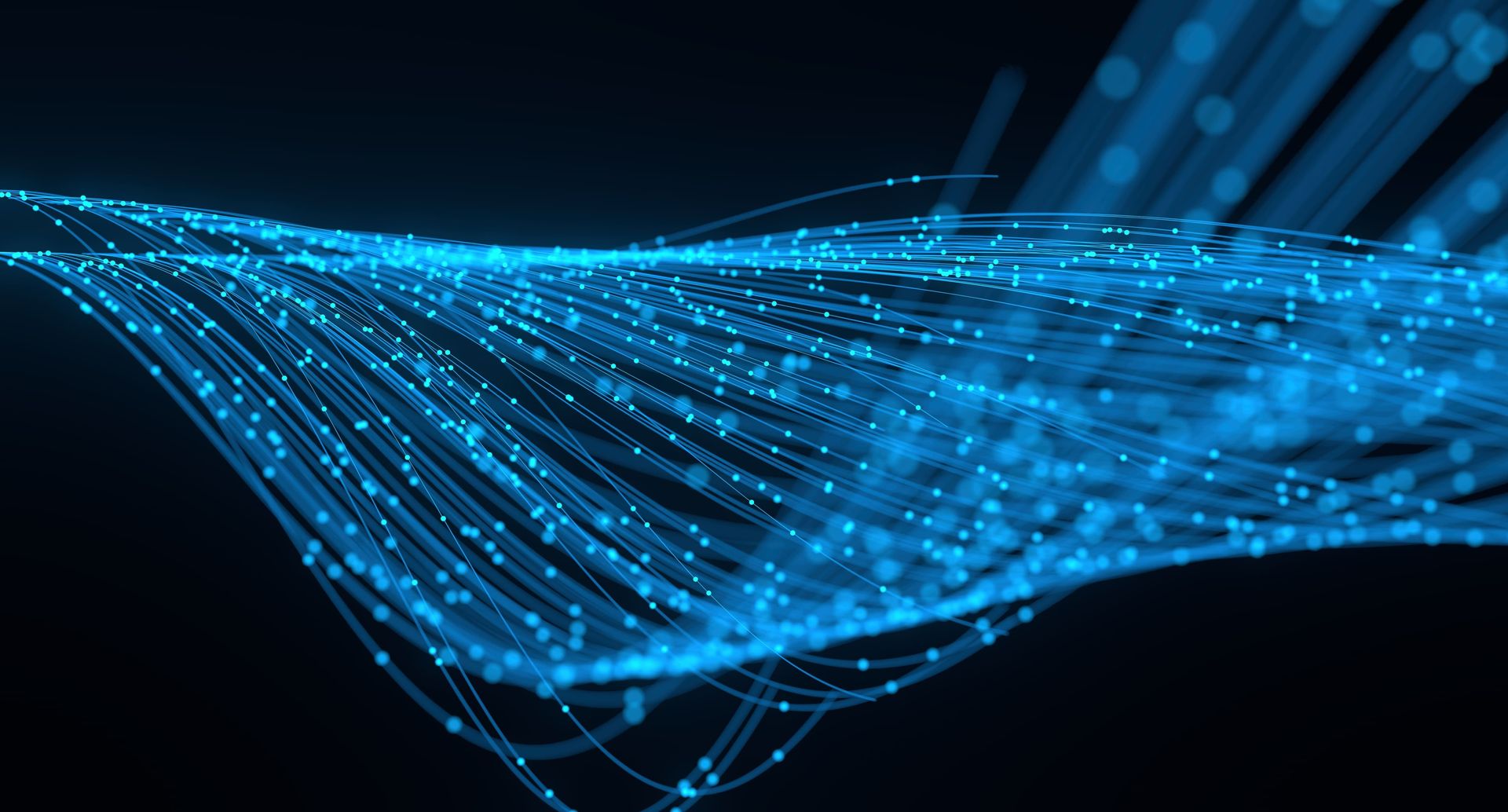
Need help with implementing a CV-driven solution?Â
FAQ
How is computer vision used in manufacturing?
Computer vision systems cover various use cases and operational scenarios. These include, for example, product design, automated product assembly, quality inspection, predictive maintenance, inventory management, supply chain traceability, and safety monitoring.
How reliable are computer vision systems in manufacturing?
Thanks to modern deep learning algorithms and neural networks, computer vision systems for object or anomaly detection and other manufacturing-related tasks have achieved close to 99% accuracy, as reported by SAS.
What is the difference between computer vision and machine vision?
While they partially overlap, computer and machine vision diverge in terms of focus.
- Computer vision
represents a broader field that includes tools and techniques to capture, process, and understand visual inputs for both analytical and practical purposes. However, the emphasis is on advanced cognitive skills, data analytics, and decision-making. - Machine vision
is a more specific term referring to the use of vision systems for industrial or manufacturing applications, including inspection, quality control, and automation. Machine vision uses specialized hardware and software to capture and analyze visual data in real time, such as measuring dimensions, detecting defects, and identifying patterns.
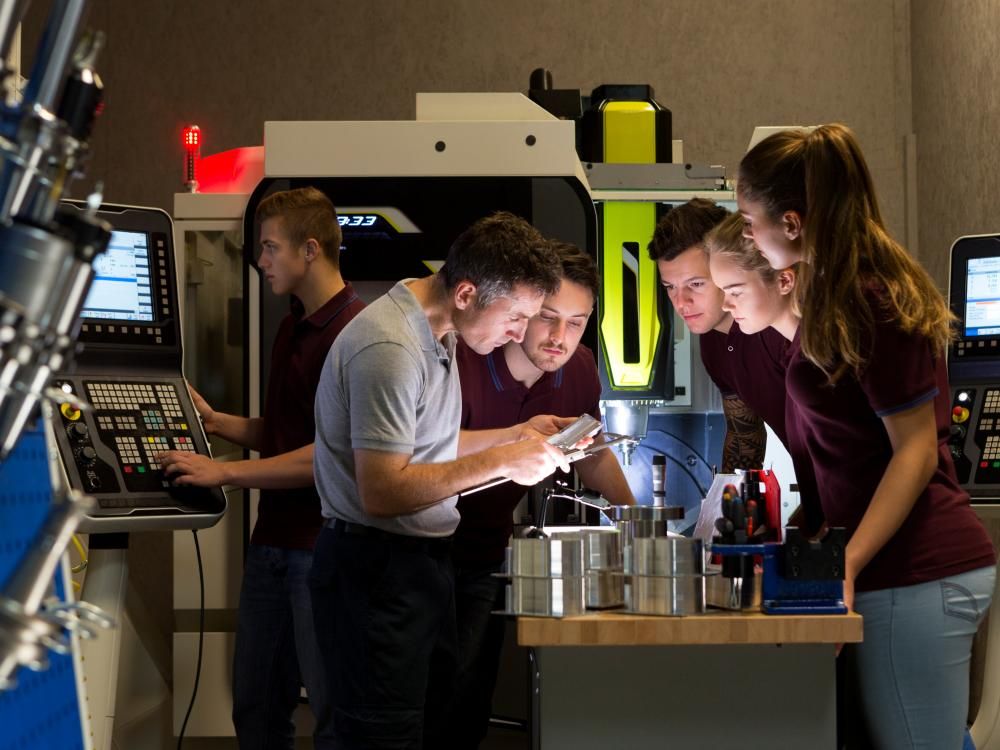
Insights
Digital twin in manufacturing: a must-have in Industry 4.0
Explore how manufacturing companies implement digital twins to increase efficiency, encourage innovation, and drive more value.
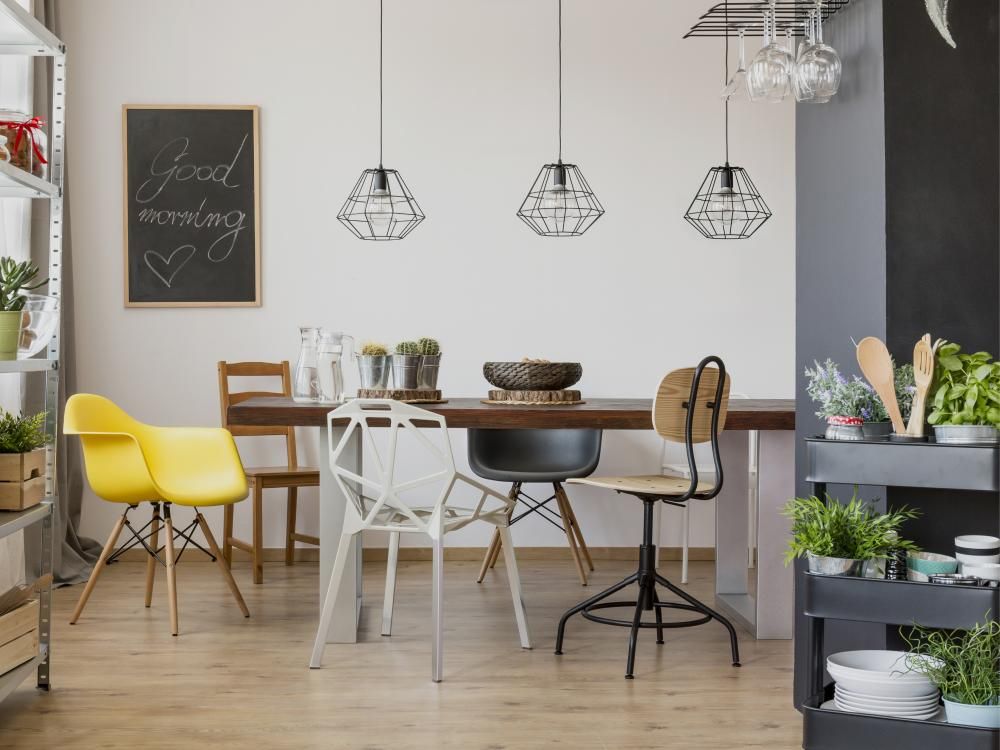
Case study
A furniture manufacturing software suite
Read the story behind Itransition’s 5-year collaboration with a leading UK furniture manufacturer on web, mobile and VR solutions.
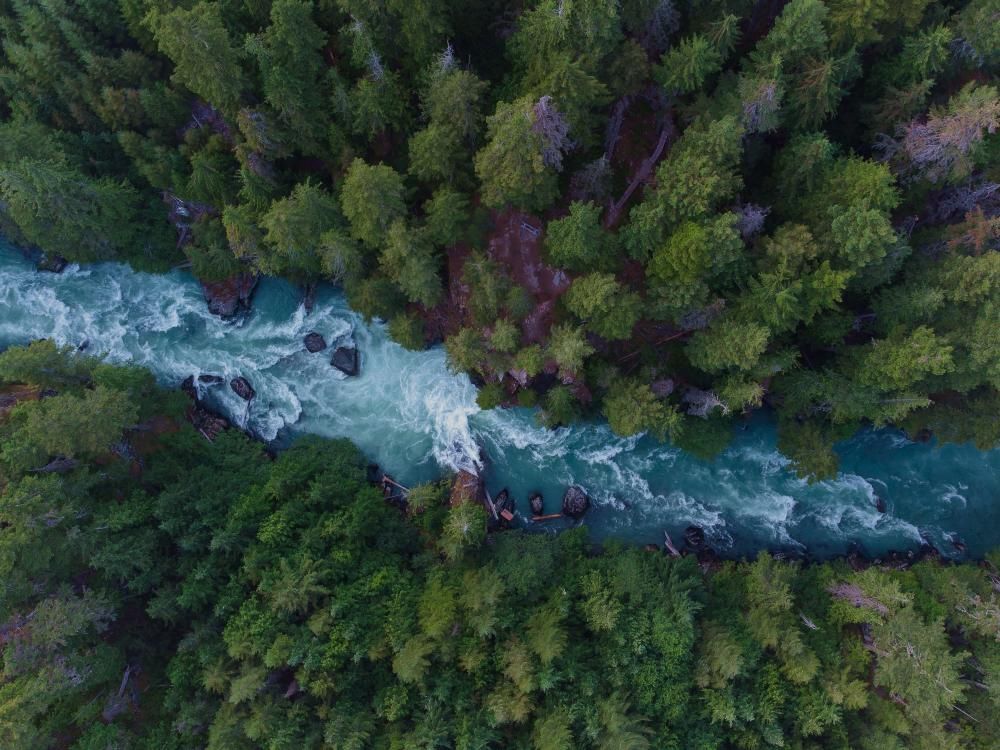
Insights
Machine learning in manufacturing: key applications, examples & adoption guidelines
Learn how machine learning can help manufacturers to improve operational efficiency, discover real-life examples, and learn when and how to implement it.
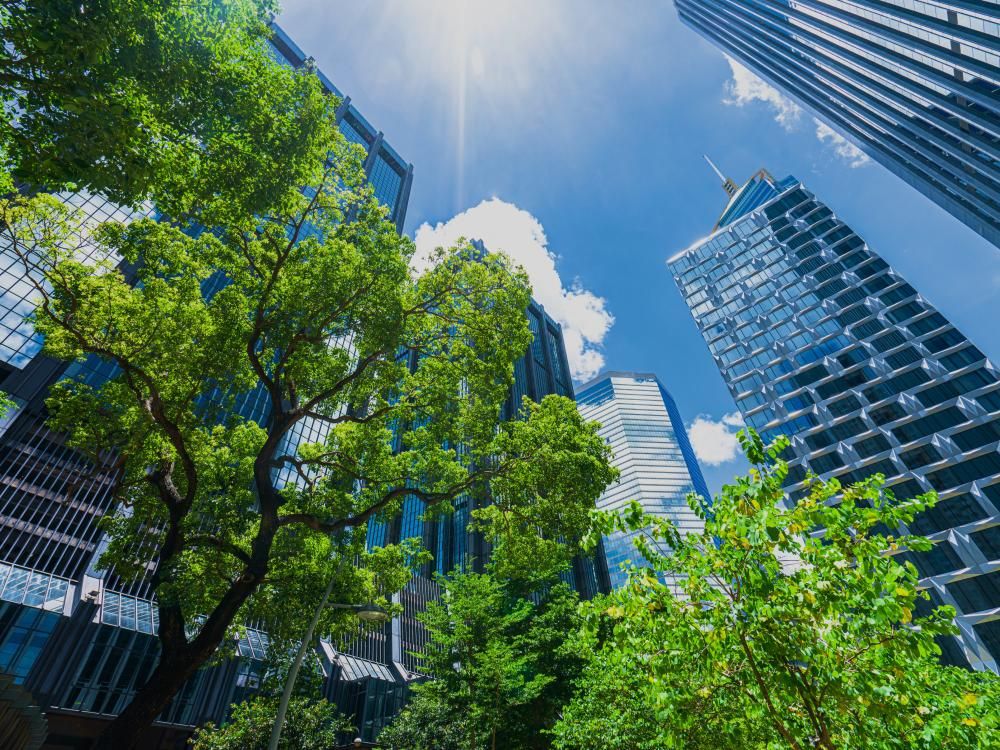
Insights
ERP for manufacturing: end-to-end solution overview
Explore top manufacturing ERP software on the market, as well as manufacturing ERP functionality, integrations, adoption roadmap, and business value.