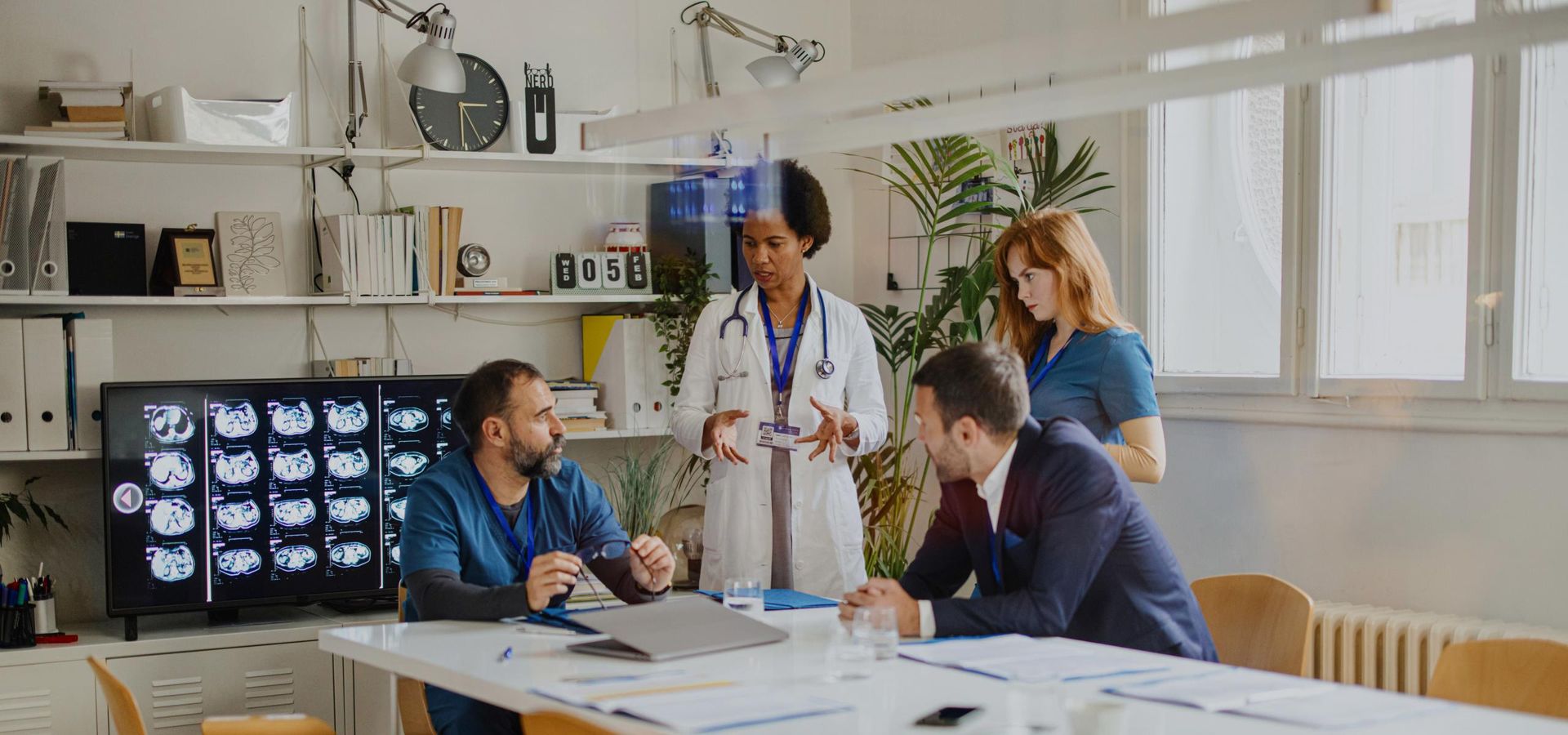
AI in radiology: top ten use cases & best practices
March 6, 2025
- Home
- Artificial intelligence
- AI in radiology
Owing to recent advancements in artificial intelligence and computational capabilities, AI-powered radiology tools are showing great promise in the field of diagnostic imaging. Able to quickly process vast amounts of image data and detect subtle patterns and abnormalities in it, AI solutions promise to enhance diagnostic accuracy, streamline clinical workflows, and ultimately lead to better patient population outcomes.
AI in radiology market statistics
of radiologists use AI in their clinical practices
American College of Radiology
plan to purchase AI tools in the near future
American College of Radiology
AI in the global radiology market CAGR from 2021 to 2030
Vision Research Reports
Ten AI use cases in radiology
The number of use cases of artificial intelligence software in clinical data science and radiology practice is growing. Here, we share a list of the most common applications of AI in radiology.
Classifying brain tumors
Spotting vertebral fractures
Spinal fractures, being the most common fragility fractures, can be an early sign of osteoporosis. They can go unnoticed since 54% of vertebral fractures remain undetected by radiologists in CT scans. The researchers at UCB Pharma attempted to solve this issue by training a deep-learning algorithm to detect and grade vertebral fractures. The algorithm was trained on real-world images containing 969 vertebrae coming from three different types of scanners. The algorithm produced an area under the curve (AUC) of 0.93, meaning that it can be potentially used in examination rooms.
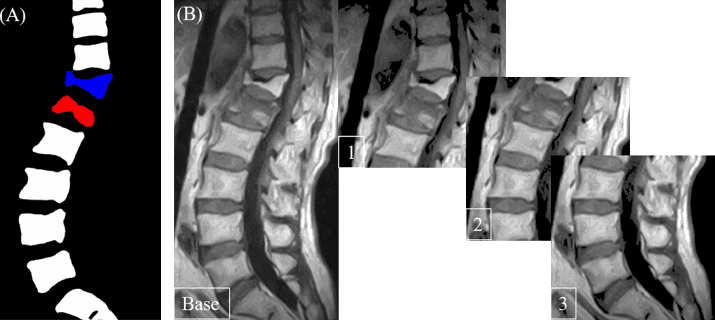
Detecting Alzheimer’s disease
Another challenging neurological disease to diagnose is Alzheimer’s. To boost radiologists’ confidence in diagnosing, researchers at the University of California have developed an algorithm that can detect Alzheimer's based on a fluorodeoxyglucose (FDG)-positron emission tomography (PET) scan. This algorithm looks for subtle processes and global changes in the brain (such as changes in glucose uptake), which is not visible to the naked eye. After training the algorithm, researchers tested its performance on 188 new images. AI correctly identified 92% of patients with Alzheimer's.
A critical point of using computer-aided detection in Alzheimer’s diagnostics is that the algorithm can pick up very early indications of the disease, which radiologists would not be able to detect. If diagnosed early enough, the progression of the disease can be delayed or even stopped.
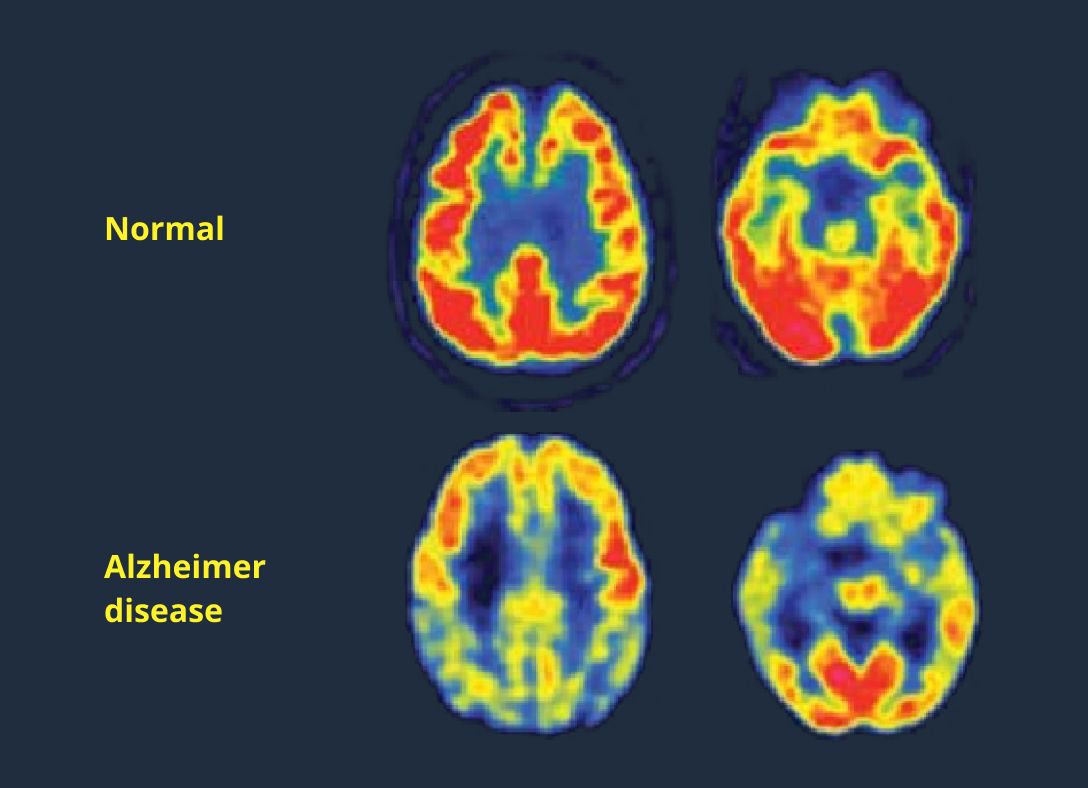
Diagnosing ALS
Assisting with radiology reporting & data-related tasks
Detecting breast cancer
In 2022, 2.3 million women were diagnosed with breast cancer, making it the most common cancer in women in 157 countries out of 185, according to WHO. Recent studies point out that AI shows increasingly promising results in oncology for detecting breast cancer in particular.
For example, German AI startup Vara in collaboration with The Mammography Reference Centre North in Oldenburg recently conducted a study where researchers assessed 2,396 screening mammograms from women who were later diagnosed with interval cancer. AI correctly detected and localized 27.5% of false negatives and 12.2% of the minimal sign cancers. This means that AI can detect breast cancer signs that aren't visible to many radiologists at the earliest stages of the disease.
Dose optimization
Detecting pneumonia
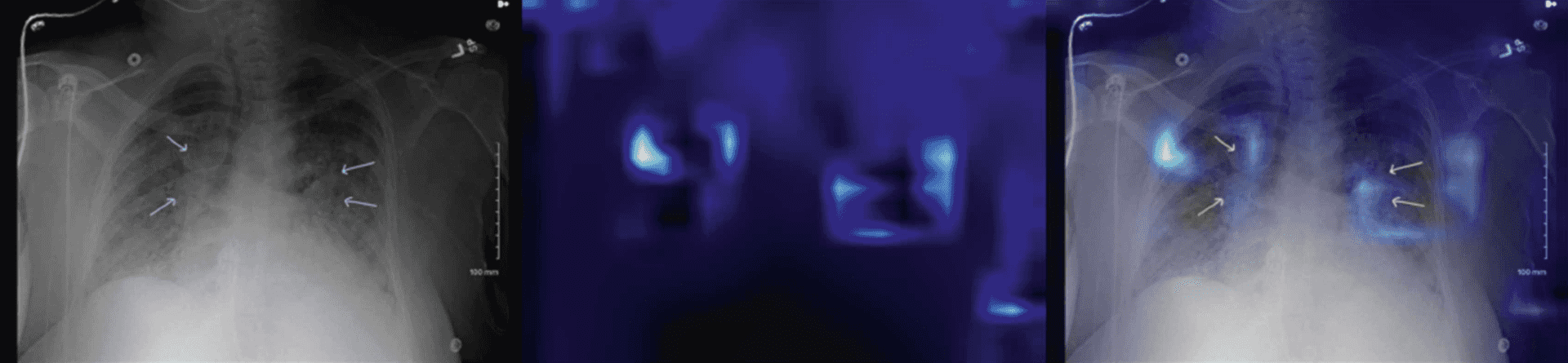
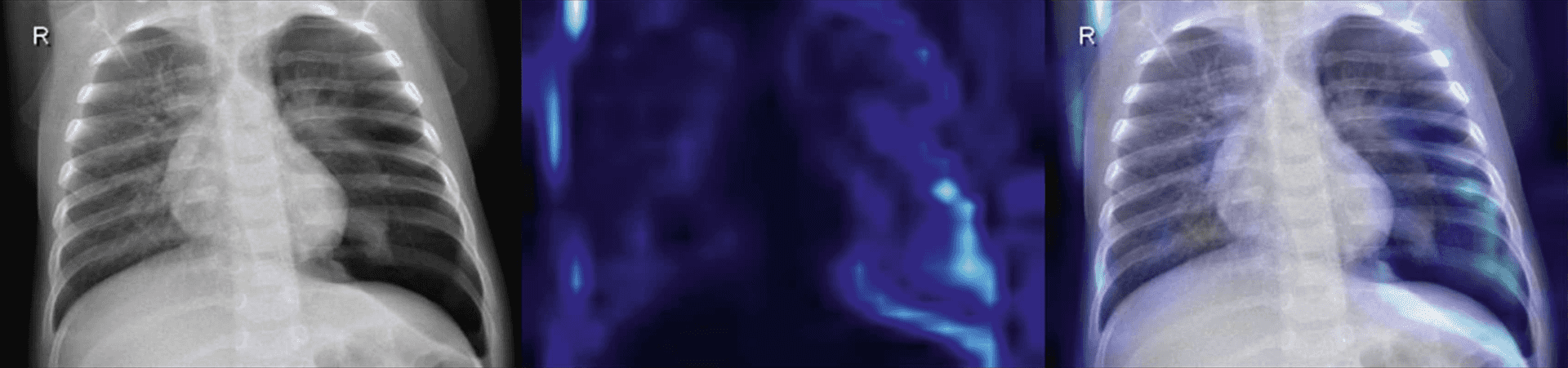
Detecting LVO
Looking for reliable AI consultants?
Examples of AI in radiology
Viz LVO
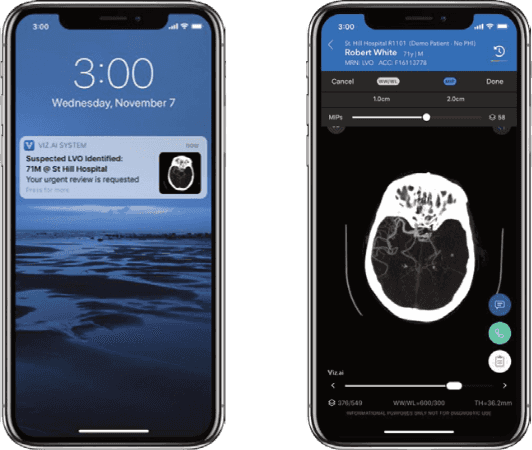
Lunit INSIGHT MMG
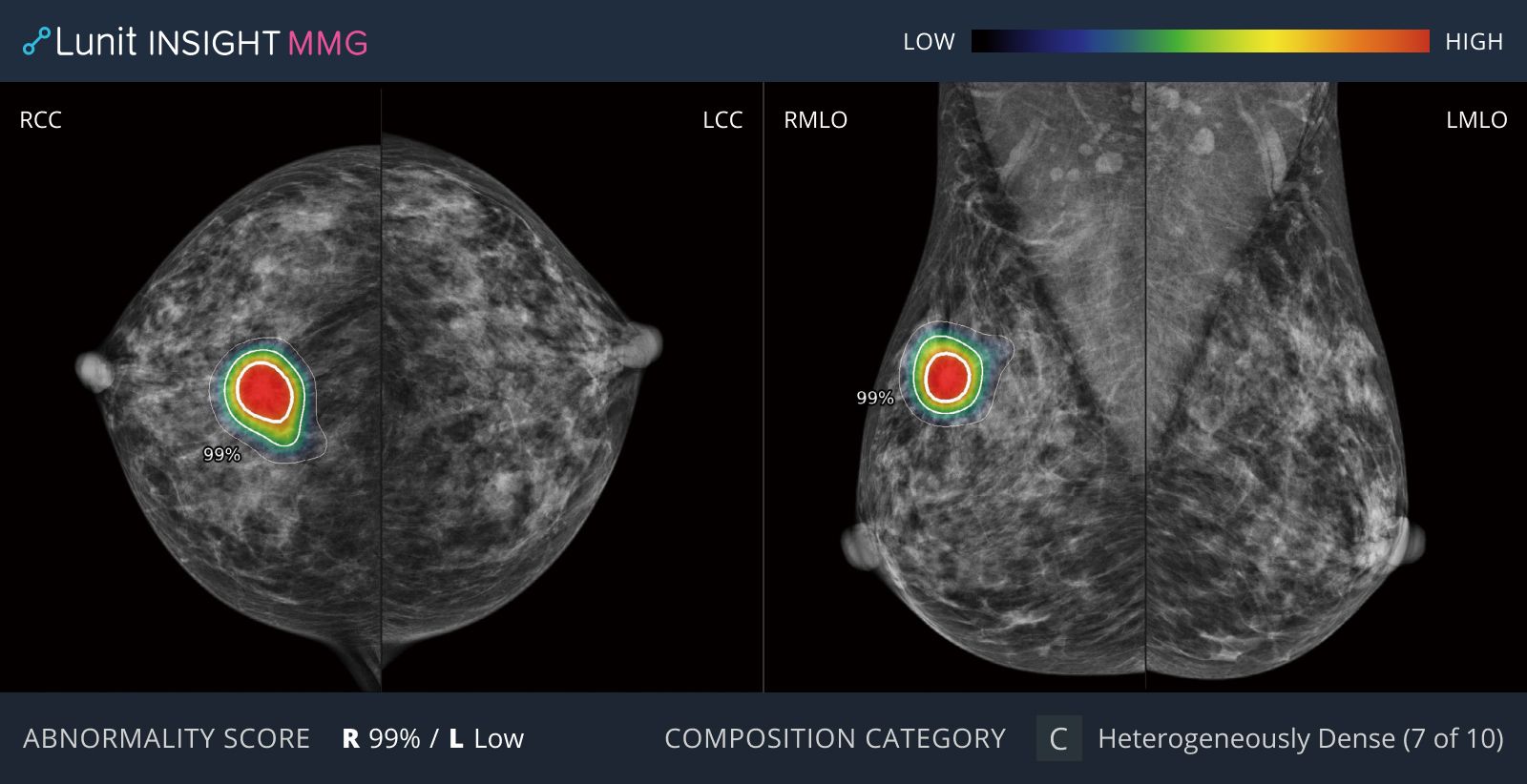
Lunit's AI tool is particularly effective in helping diagnose dense breasts, which are often more likely to be misdiagnosed among East Asian women, and has helped improve communication between radiologists and breast surgeons.

Yeh Wei Cheng
director of the Department of Radiology Nantou Hospital
Rapid ICH
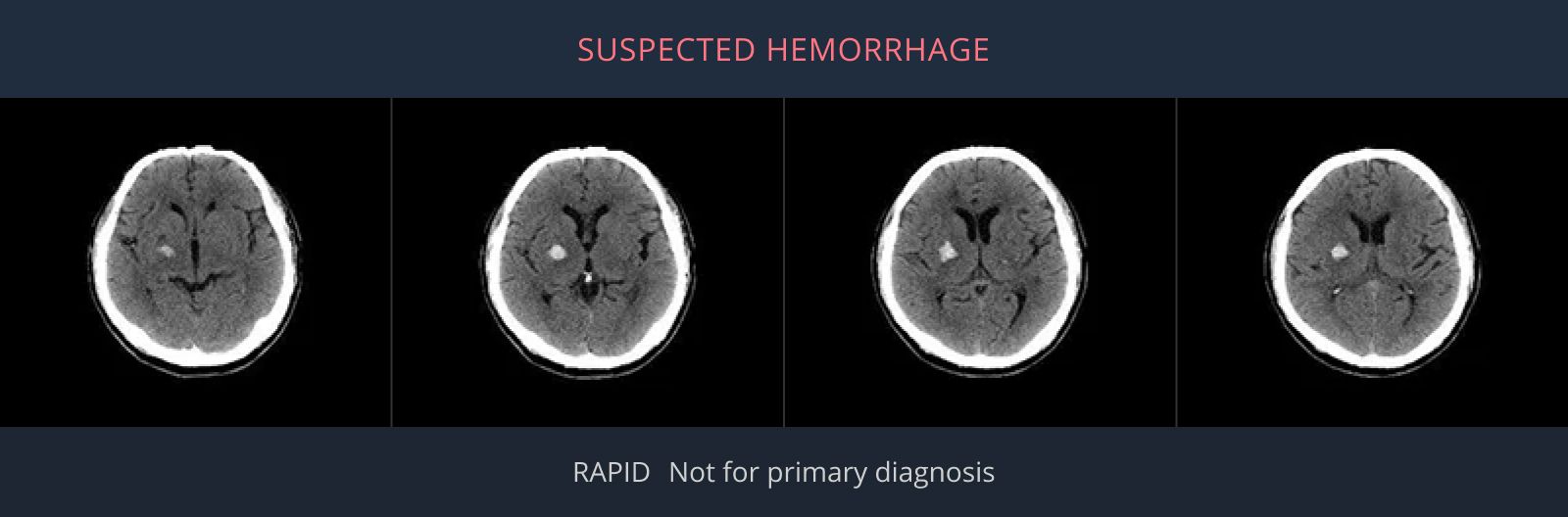
qXR
qXR, developed by Indian AI startup Qure, is a tool that helps radiologists detect chest pathologies indicative of lung cancer in radiography imaging. With the help of a proprietary deep learning model trained on more than 3.5 million X-rays, xQR can reliably identify abnormalities in the lungs, pleura, mediastinum, and bones in under 1 minute for each scan. A recent peer-reviewed study revealed xQR’s sensitivity of 99% and specificity ranged from 87% to 92%, making it a reliable assistant in detecting malignant and non-malignant lung nodules, tuberculosis, and other lung diseases.
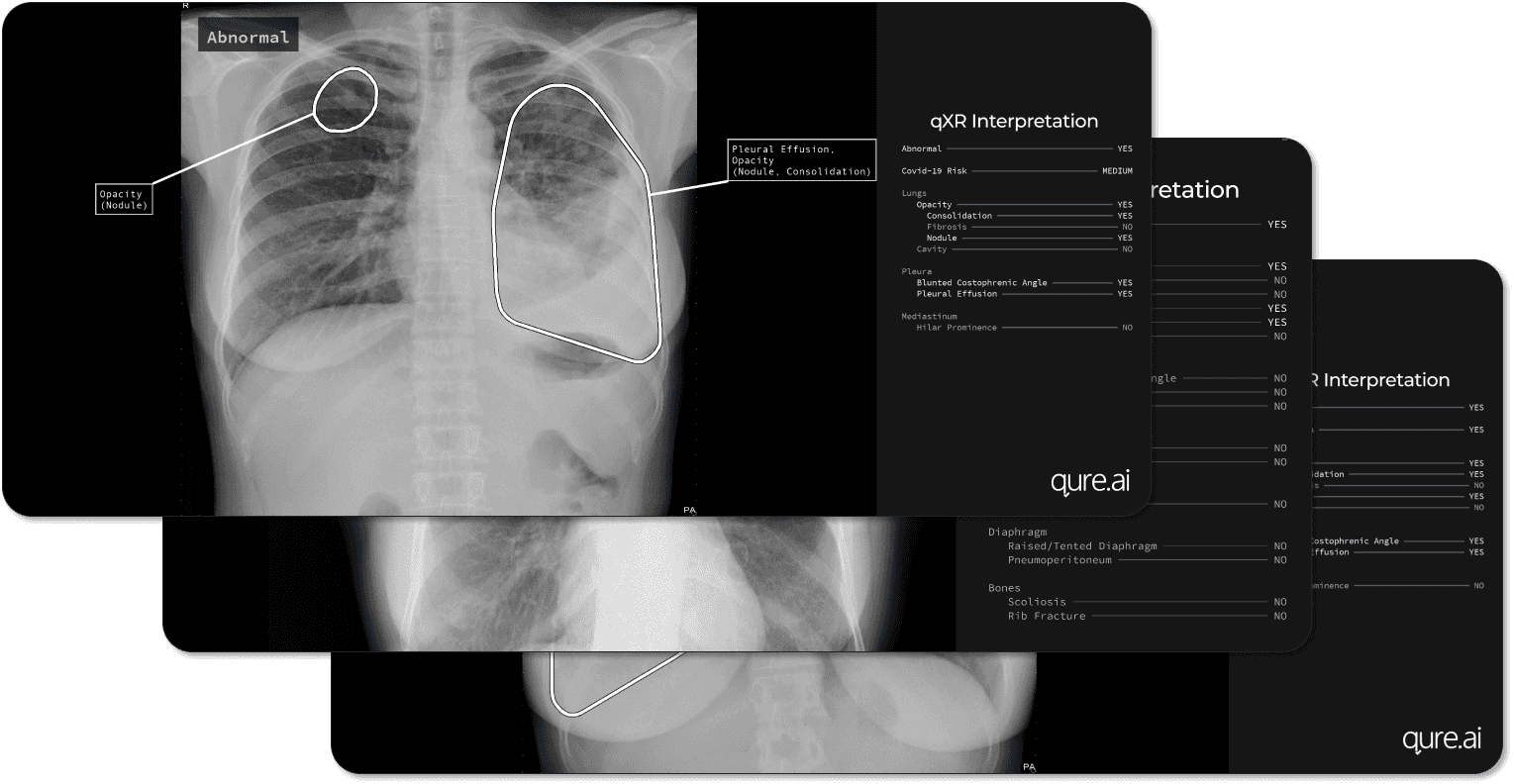
Ten benefits of AI in radiology
Early detection
Improved prioritization
Improved accuracy
Optimized radiology dosing
Reduced radiation exposure
Enhanced image quality
Improved satisfaction
Faster diagnosis
Improved access to care
Improved reporting
AI in radiology
Early detection
AI can more reliably detect diseases at early stages, preventing complications and dramatically improving patient outcomes
Common challenges with AI in radiology & potential solutions
Aligning medical guidelines with AI outputs
Challenge
Solution
Most current AI applications in radiology provide estimates of how likely a certain patient is to have complications based on radiological imaging. For example, an AI system concludes that a breast lesion of a certain patient has a 10% chance of being malignant. A radiologist could opt for a biopsy, but the AI system may not understand the severity of the problem and deem a 10% chance of cancer insignificant for conducting a biopsy.
Developers and medical professionals must work in close collaboration. Insights from medical professionals can improve the performance of AI-based image acquisition and processing and decision-support solutions.
Human reluctancy
Challenge
Solution
Designing accurate AI algorithms is as challenging as understanding how to integrate AI systems in daily radiology workflows. The roles and responsibilities of radiologists can change. Still, it is unlikely that algorithms will make fully autonomous clinical decisions, regardless of their implied accuracy and effectiveness.
Healthcare providers should ensure that human-machine collaboration is effective and human professionals keep the lead in the decision-making.
Poor IT infrastructure
Challenge
Solution
Despite many use-cases of AI in radiology, many healthcare providers haven’t even started the digital transformation. Their research and patient data is siloed, security measures are outdated, hardware requires upgrades, and systems lack interoperability. Incorporating AI in such a setting can create more hurdles.
Combating these problems comes down to gradual changes in IT infrastructure, preferably with support from an experienced third-party vendor. Healthcare organizations can start their AI adoption journey with the adoption of image management and PACS systems for improved image quality and easier retrieval.
Data quality
Challenge
Solution
The lack of high-quality labeled datasets is a universal problem across sectors and industries, and radiology is no exception. Gaining access to clear and labeled imaging data for training medical AI is not easy.
Given that many healthcare providers are currently in the midst of digital transformation and the demand for quality datasets is growing every day, it is only a matter of time before the majority of datasets will adhere to high-quality standards.
Want to add AI-based technologies into your radiology practice?
AI methods in radiology
AI has become synonymous with machine learning (ML), which encompasses many techniques used to create predictive models, including deep learning models. Today, the most popular way of mimicking human decision-making is artificial neural networks (ANN), which is a certain type of very flexible deep learning model.
ANN is inspired by the design of biological neural networks. In other words, this model tries to mimic the network of neurons that comprise the human brain so that it can solve problems similar to the way a human would.
Currently, the convolutional neural network (CNN), which is a class of ANN, is the most popular model for analyzing visual data, making it the most important component of AI in radiology.
AI in radiology: implementation tips
Build a multidisciplinary team
Build a multidisciplinary team
While input from field experts is always critical to the development of AI solutions in virtually any industry, medical imaging calls for radiologists to have a much more decisive and significant role in the development of AI-based radiology solutions. Engineers and data scientists lack an understanding of essential radiological and anatomical concepts, which is crucial to creating AI systems applicable in radiology.
Gain support from leadership
Gain support from leadership
Healthcare providers should get key decision-makers and executive leadership on board with the implementation of AI tools. According to the latest report by Deloitte, healthcare providers who succeeded in AI initiatives put leaders at the core of AI transformation. These leaders should clearly communicate with the radiology department on how new tools will impact their daily workflows.
Prioritize AI explainability
Prioritize AI explainability
In healthcare, where decisions can directly influence one's well-being, it is paramount for radiologists to understand the underlying decision-making of AI systems. Most AI radiology tools use deep learning models, and system interpretability and transparency become crucial. While deciphering deep learning models is a universal task across many AI applications, developers are responsible for minimizing bias and variability and making models as explainable as possible. It comes down to continuous algorithm validation, dataset audit, evaluation of its performance metrics, standardized documentation, and routine bias assessment.
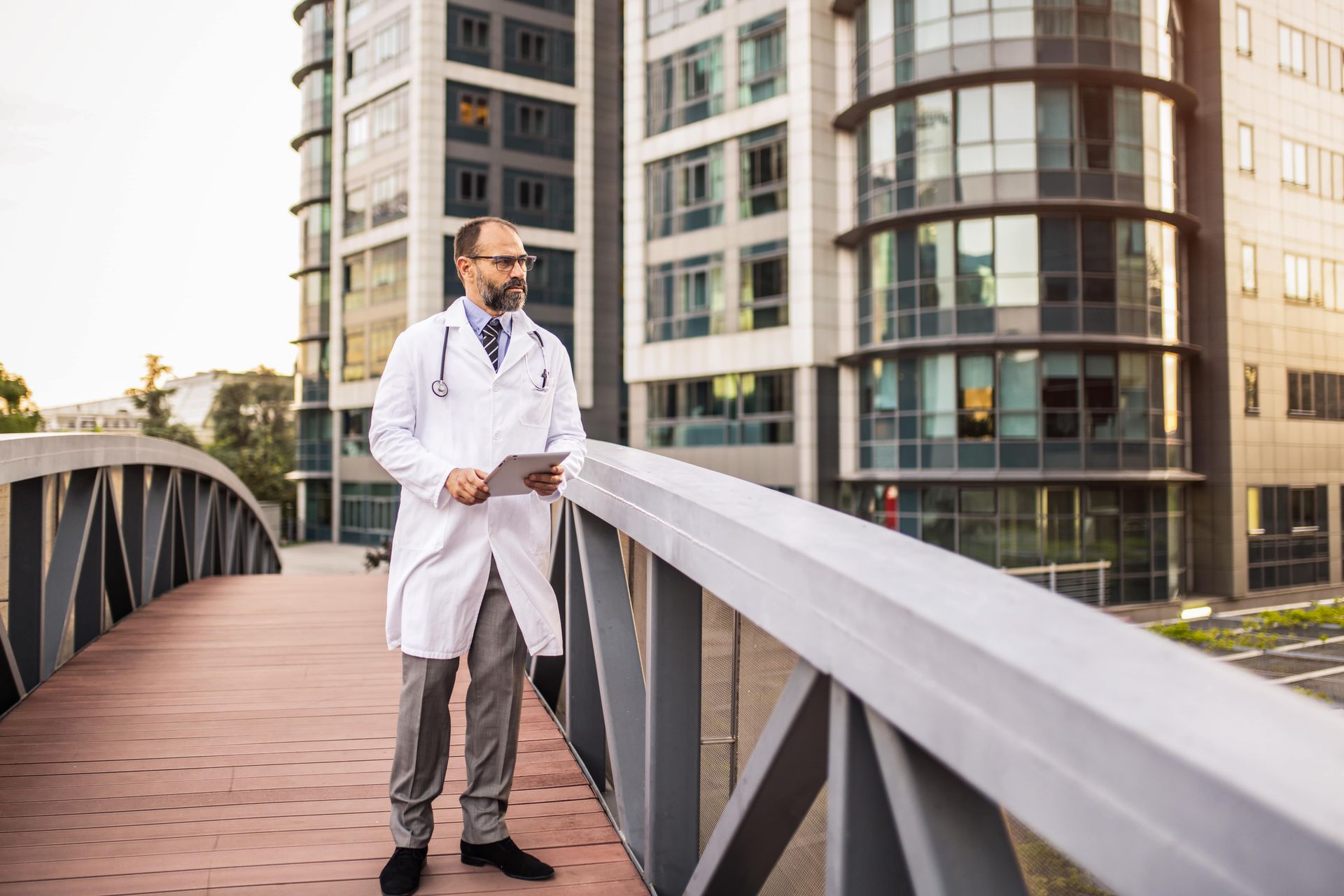
Add AI into your radiology practice
AI-based tools are already used in different fields of radiology, and their role will increase moving forward. The validation of existing AI applications and development of new ones for solving common radiological problems requires effective collaboration between radiologists, data scientists, and engineers.
AI will make the next two decades transformational for healthcare and radiology in particular. Our professional AI consultants can help you implement AI-based technology into your radiology practice.
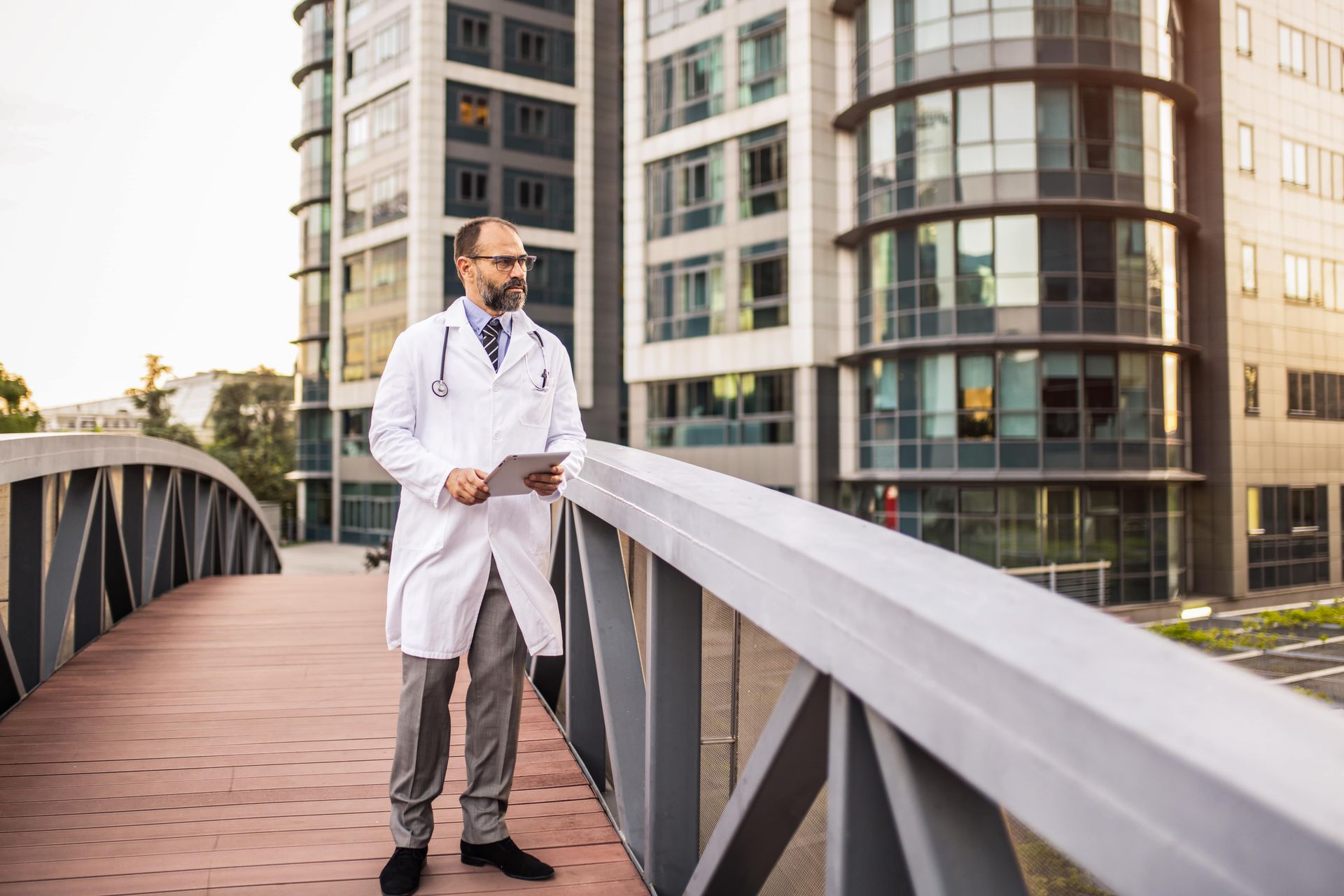
FAQ
Will AI take over radiology?
AI can free radiologists from mundane tasks and improve their efficiency, but human involvement will remain critical to solving complex problems that call for rapid change. Similar to the advent of autopilot in aviation, AI will augment radiologists’ work rather than replace it. Curtis Langlotz, a radiologist at Stanford, said: “AI won’t replace radiologists, but radiologists who use AI will replace radiologists who don’t”.
What is the future of AI in radiology?
With the growing acceptance of AI among medical professionals and regulatory bodies, this technology will take over radiologists’ repetitive tasks and become their essential reference tool. The key question is how AI will further enhance diagnostic accuracy and efficiency while maintaining human oversight.
When was AI introduced in radiology?
The first use of AI in radiology dates back to 1992 and was used to detect microcalcifications in mammography. Arterys, the first AI-based FDA-approved medical imaging solution, was certified in 2017.
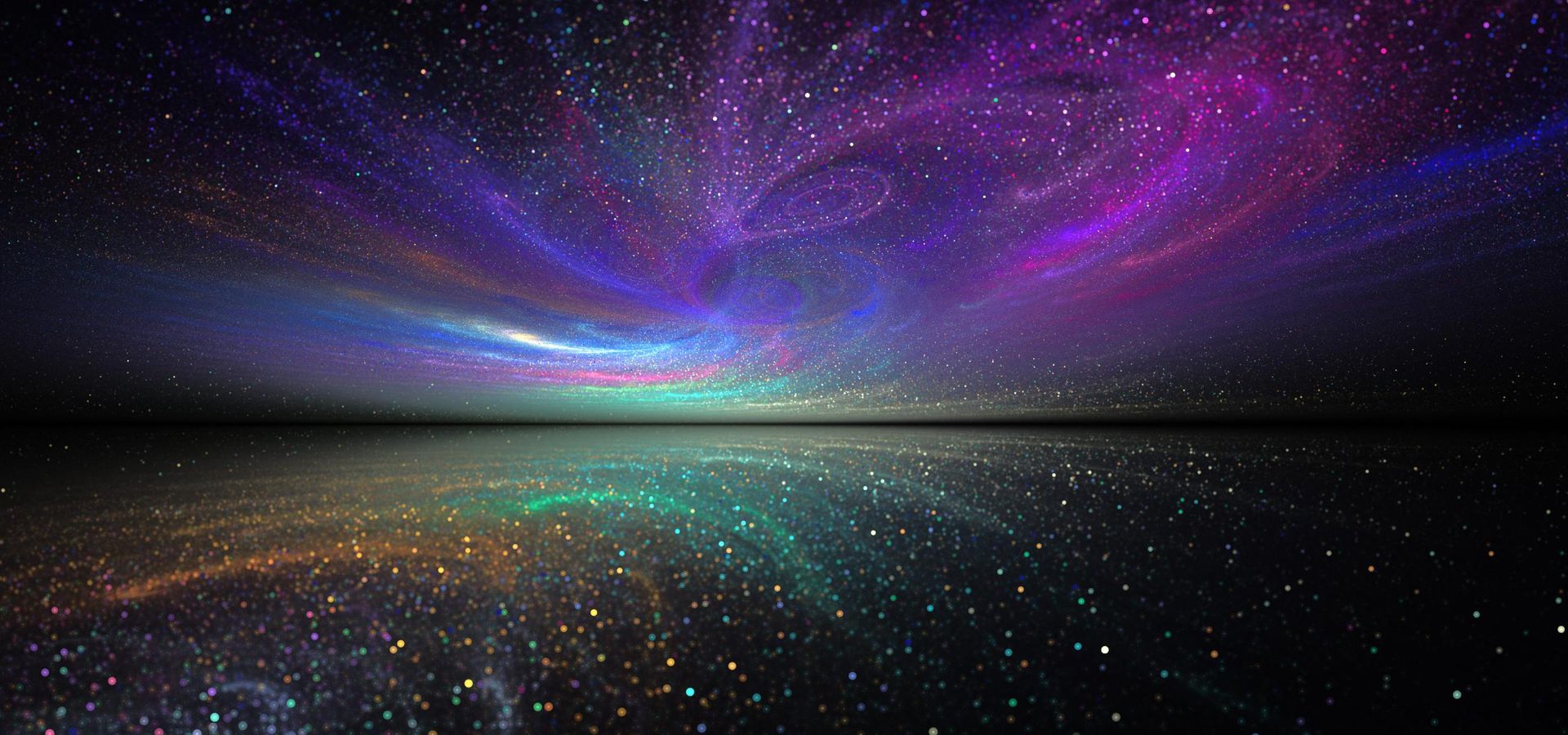
Service
AI services & solutions
Explore our range of AI services, along with key AI use cases, related technologies, and adoption guidelines.
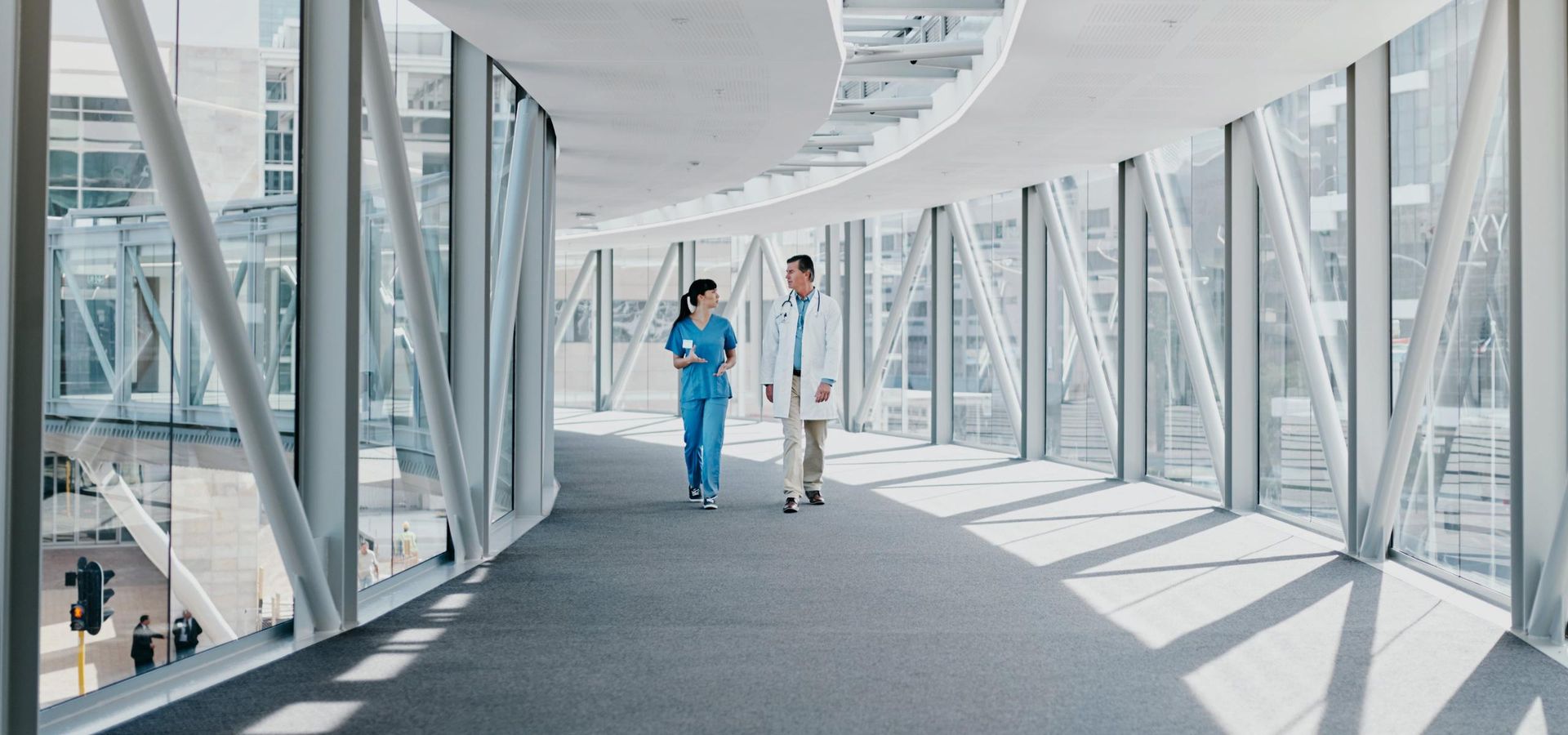
Service
EMR/EHR software development: a comprehensive guide
Discover the major capabilities and use cases of EHR systems and learn how we can support your organization with EMR/EHR software development services.
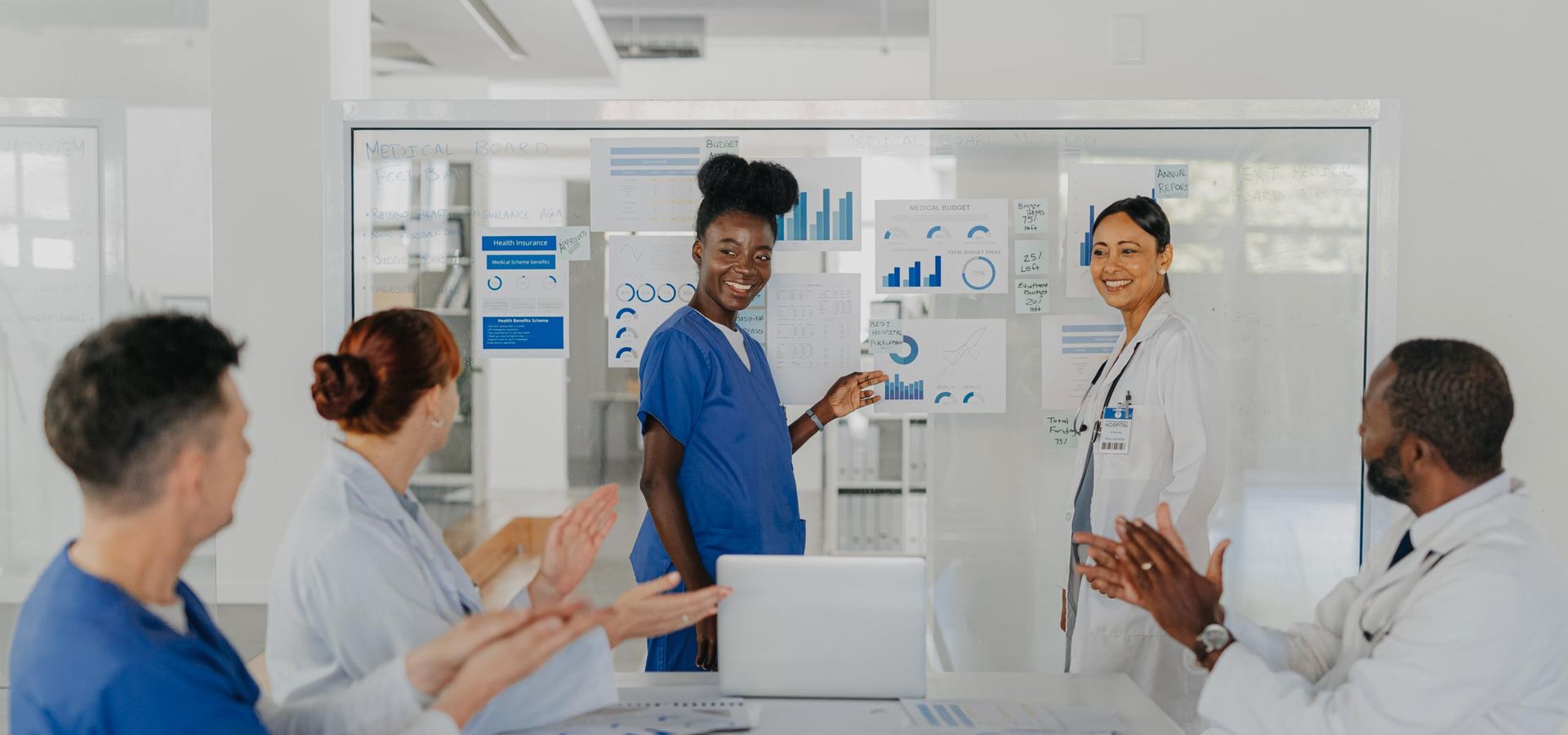
Service
Healthcare data analytics services: key types, features, and applications
Discover how our healthcare data analytics services can enhance patient loyalty while growing revenue and learn which solutions fit your business goals.
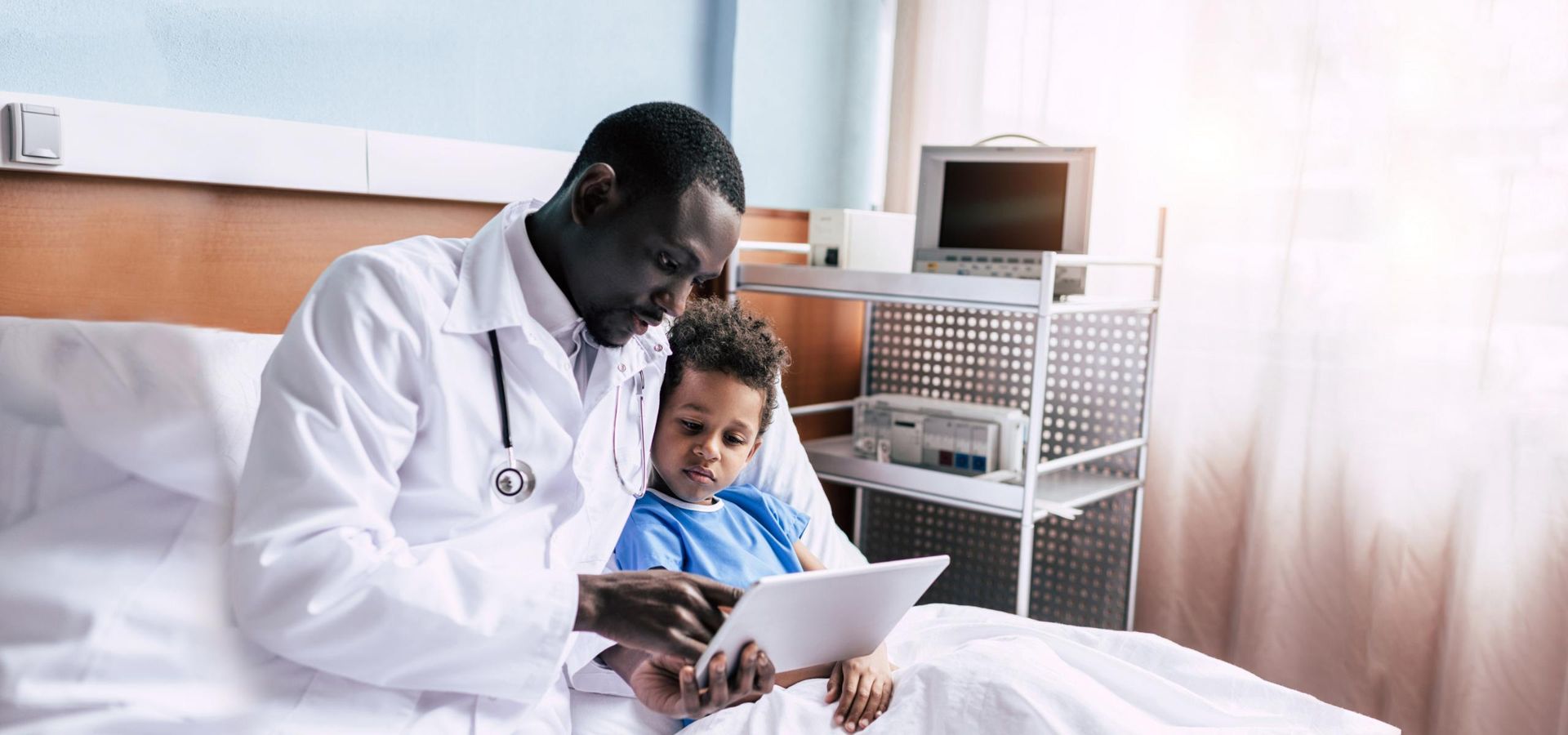
Service
Healthcare software development solutions & services
Entrust your medical software development to a company with 25+ years of experience in healthcare IT. Compliance and security guaranteed.
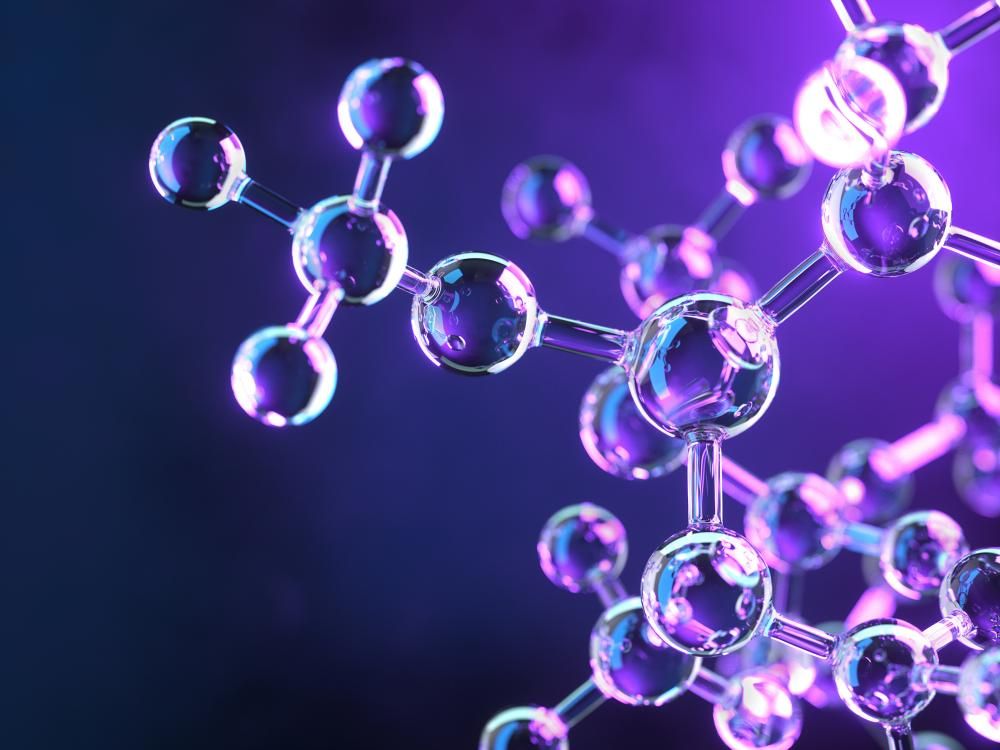
Insights
NLP in healthcare: fostering medical digitalization
NLP in healthcare provides a wide array of tools to assist medical professionals and improve patient experience. How? Let's find out.
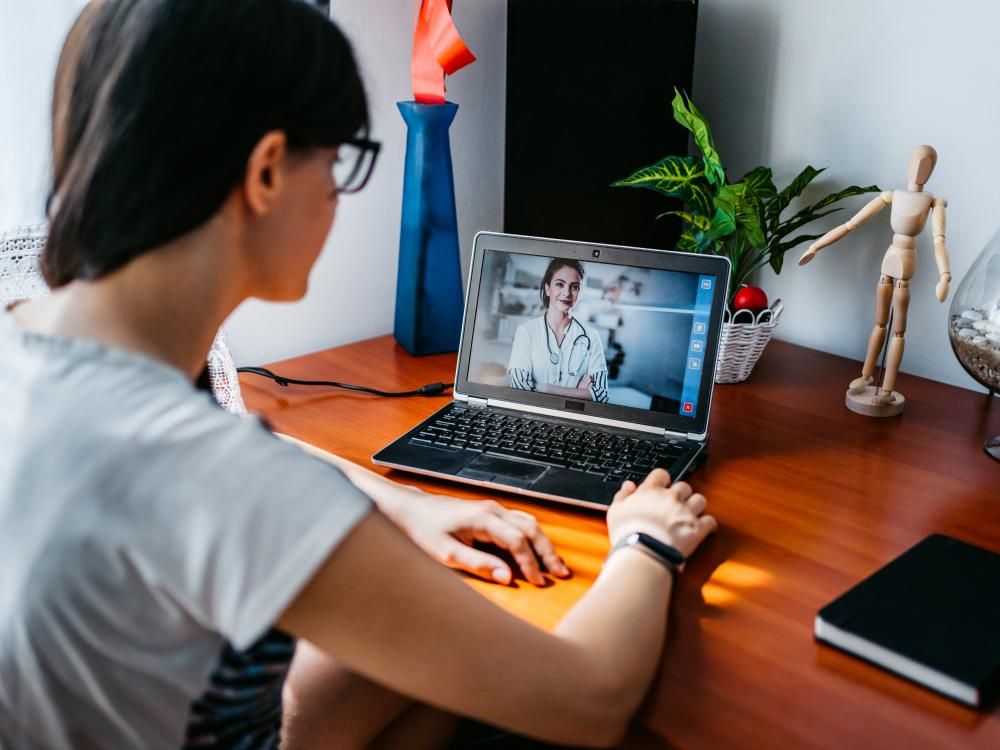
Insights
Digital transformation in healthcare: today’s trends and their positive effects
We look into digital transformation in healthcare and explore its positive impact on care delivery and waste reduction.