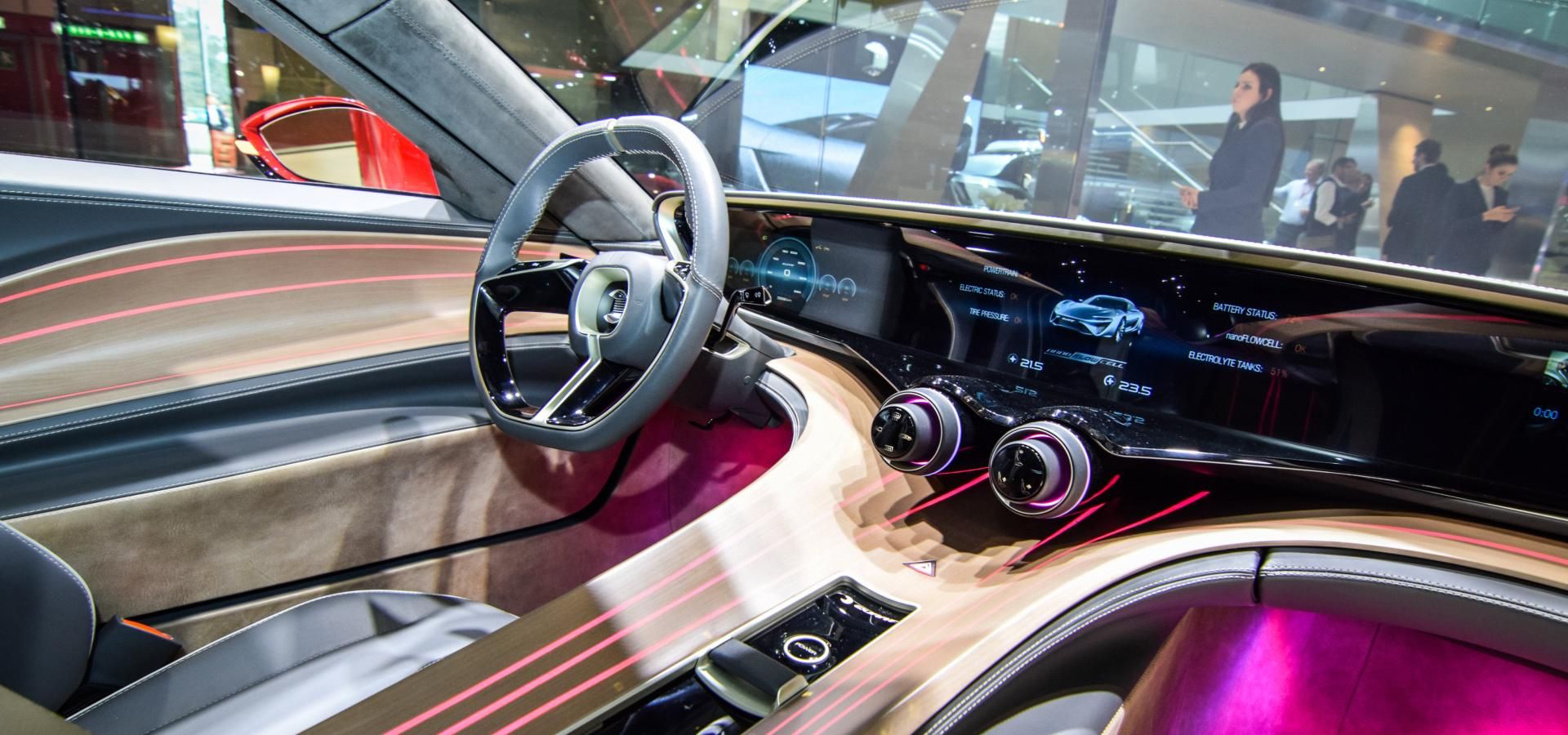
AI in the automotive industry: use cases, success stories & adoption guidelines
March 5, 2025
- Home
- Artificial intelligence
- AI in the automotive industry
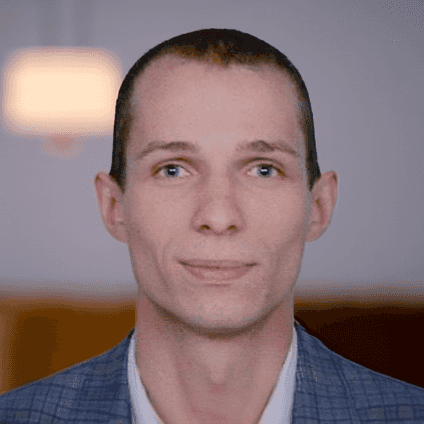
Head of AI/ML Center of Excellence
With solid experience in AI consulting, Itransition develops advanced solutions to help companies overcome the unique challenges of this industry.
AI in the automotive industry: market statistics
the projected size of the global automotive AI market by 2032
Allied Market Research
of automotive enterprises surveyed are experimenting with at least one gen AI application
McKinsey & Company
In which of the following ways, if any, is your organization using AI and automation today? Please select all that apply.
[Among IT Professionals at companies currently exploring or deploying AI]
Scheme title: Use cases of AI and automation in the automotive sector
Data source: IBM Global AI Adoption Index Report
AI use cases in transportation
As an emerging tech trend in the transportation sector, AI promises to make driving less stressful and more secure and, on a larger scale, facilitates logistics operations involving large fleets of vehicles.
Personal voice assistants
Whether it’s a third-party personal assistant like Alexa and Siri or a proprietary solution, most automotive companies currently implement AI systems that use voice recognition to interact with drivers. These virtual assistants can adjust the interior temperature, provide information on fuel level and consumption, make calls, and change radio stations. Additionally, such tools offer high levels of personalization by remembering drivers' preferences and suggesting adjustments based on the context.
Fleet management
AI systems rely on connected vehicle technology and algorithms to process data on road conditions, traffic in a specific area, weather, and other environmental information and help fleet managers perform various tasks. These include identifying the most efficient routes, predicting potential delays, coordinating drivers, and rescheduling deliveries accordingly. Furthermore, logistics companies can use these solutions to optimize order distribution across their freight vehicles based on cargo weight, volume, and delivery points.
Advanced driver-assistance systems
ADAS solutions integrate AI-powered cameras and sensors to provide semi-autonomous driving functionality like adaptive cruise control, traffic sign recognition, forward-collision warning, and drowsiness detection. Previously found in premium cars, these systems are turning into a staple due to their ability to ensure better vehicle control even in challenging driving conditions, which helps minimize the risk of road accidents and boost vehicle safety.
Self-driving vehicles
Fully autonomous vehicles embody the highest level of driving autonomy according to the SAE six-level classification, as they leverage AI-powered ADAS systems that can control the vehicle under any conditions. These typically use multiple types of sensors (visual, radar, lidar, INS, and ultrasonic sensors) to gather data from the surrounding environment, which is then processed with AI algorithms to enable the operation of autonomous cars. Despite complex ethical implications, public concerns, and the need for further experimentation, we can already find promising examples of this technology, such as self-driving cars and taxis and truck platooning systems.
Develop robust AI solutions with Itransition
AI use cases in vehicle manufacturing
Generative design
Forward-looking automotive manufacturing companies are already using generative design software to create more durable and sustainable automotive parts. Generative AI-based systems can create hundreds of design variations for a specific component based on the set of parameters defined by designers and engineers, who then select the most suitable option.
Vehicle design simulation
Automakers extensively use digital twins to simulate how certain design decisions impact their final products. By feeding machine learning systems with historical and real-time sensor data on speed, acceleration, fuel efficiency, and other metrics, engineers and designers can understand how their ideas translate into vehicle performance without costly physical prototype testing. For example, this includes predicting how driving style and particular weather conditions impact battery performance in electric vehicles.
Automated vehicle assembly
Nowadays, AI-powered robots are increasingly present on the assembly lines of major car manufacturers. These manufacturing solutions can easily identify components via computer vision and manipulate them with pinpoint precision through mechanical arms, which makes them valuable tools for improving production outputs while mitigating physical workloads.
Quality control
While many automakers already use rule-based anomaly detection for quality control, this approach can fail to detect minor or new types of defects since rules must be updated regularly and can hardly cover the entire range of potential anomalies. Quality control solutions powered by deep learning and computer vision can go beyond simple anomaly detection and identify multiple types of defects. This almost completely eliminates the need for human intervention and significantly increases quality control efficiency.
Manufacturing equipment predictive maintenance
Traditionally, technicians perform equipment maintenance according to a predefined schedule to ensure that industrial machinery doesn't fail unexpectedly. Instead, IoT sensors can gather data from machinery parts and send this data to an ML-based system that detects anomalies, such as performance deviations, and alerts employees about potential failures. This way, automotive manufacturers can cut maintenance costs and minimize equipment downtime.
Production scheduling
AI-powered solutions can predict product demand based on economic conditions and industry trends, allowing manufacturers to adjust output in line with these forecasts and manage inventory. Combined with other Industry 4.0 technologies like IoT, AI systems can analyze additional real-time information on shipments to maximize supply chain visibility and help managers refine production process planning and product distribution.
AI use cases in sales & customer service
Artificial intelligence is radically changing the way vehicle manufacturers, dealers, and insurers approach sales and service management, enabling them to provide drivers with a better customer experience and competitive pricing while mitigating risks like vehicle breakdown or insurance fraud.
Customer engagement
AI has easily found its way into the toolkit of automakers’ marketing and sales departments. Organizations often rely on AI-powered corporate software like CRM systems to personalize advertisements based on lead and customer data, automate lead management, forecast product demand according to market trends, and optimize their marketing and sales strategies. Furthermore, sales teams can use these solutions to identify cross-selling and upselling opportunities according to customer behavior and interests.
Vehicle diagnostics
With the help of AI and predictive analytics, car manufacturers can gather data on vehicle operation and usage conditions and assess how their models perform in real-life for product development purposes. At the same time, diagnostics systems detect anomalous conditions through smart sensors and alert drivers about potential technical issues so that they can timely turn to car dealer servicing or independent workshops.
Customer service chatbots
Conversational AI is an increasingly popular tool for enhancing relationships between customers and brands and increasing brand loyalty. AI chatbots can take over employees’ mundane tasks like scheduling test drives, helping customers with car model selection, answering customers’ questions about car features, and gathering customer feedback.
Vehicle damage assessment
Some insurance companies currently leverage AI and computer vision to automate vehicle inspection and facilitate car accident case resolution. For instance, drivers can use their mobile phone cameras to take pictures of damaged cars and attach them when filing insurance claims. An AI-powered system analyzes such images and evaluates the extent of the damage through computer vision algorithms, making the assessment process much faster and more objective.
Vehicle insurance fraud detection
Every year, insurers pay out billions of dollars in fraudulent claims, whose cost is reflected in higher premiums for policyholders. ML systems for fraud detection can use natural language processing to spot inconsistencies in insurance claims and flag them for human review. With the help of predictive analytics, insurers can also estimate the risk of fraud based on the respective policyholder profiles.
Looking for an automotive software development partner?
Benefits of AI in the automotive industry
Improved fuel efficiency
AI-powered engineering helps optimize vehicle aerodynamics and engine efficiency to reduce fuel consumption and gas emissions.
Manufacturing cost savings
Automated assembly lines speed up vehicle production, while predictive maintenance enables manufacturers to minimize repair costs.
Enhanced road safety
AI solutions provide drivers with real-time information on potential hazards, automate driving maneuvers, and predict upcoming vehicle failures to prevent road accidents.
Personalized driver experience
AI-based infotainment systems enable in-vehicle configurations like seat and mirror settings tailored to driver preferences and needs.
Faster transportation
AI systems can monitor traffic flows in real-time to optimize routes and make journeys faster and more enjoyable for drivers.
Stronger customer relationships
AI-based software solutions enable manufacturers and dealers to provide clients with a personalized buying experience and effective car servicing, fostering retention and loyalty.
Reduced insurance risk
AI-powered software helps automotive insurance companies identify business risks like insurance fraud and calculate premiums based on risk levels more accurately.
Superior product quality
AI systems can identify surface vehicle defects and other issues with utmost precision to ensure compliance with quality standards.
AI in automotive helps achieve
Real-life AI applications in automotive
Hyundai’s generative vehicle design
Hyundai Motor Group applies AI-driven generative design to redefine product development and revolutionize how vehicles traverse. The company partnered with product innovation studio Sundberg-Ferarhas to build the Ultimate Mobility Vehicle “Elevate”, capable of traversing the most challenging terrain. Elevate can transform from a four-wheeled vehicle into a four-legged walking robot, and its inventors claim the vehicle will prove most useful for search and rescue.
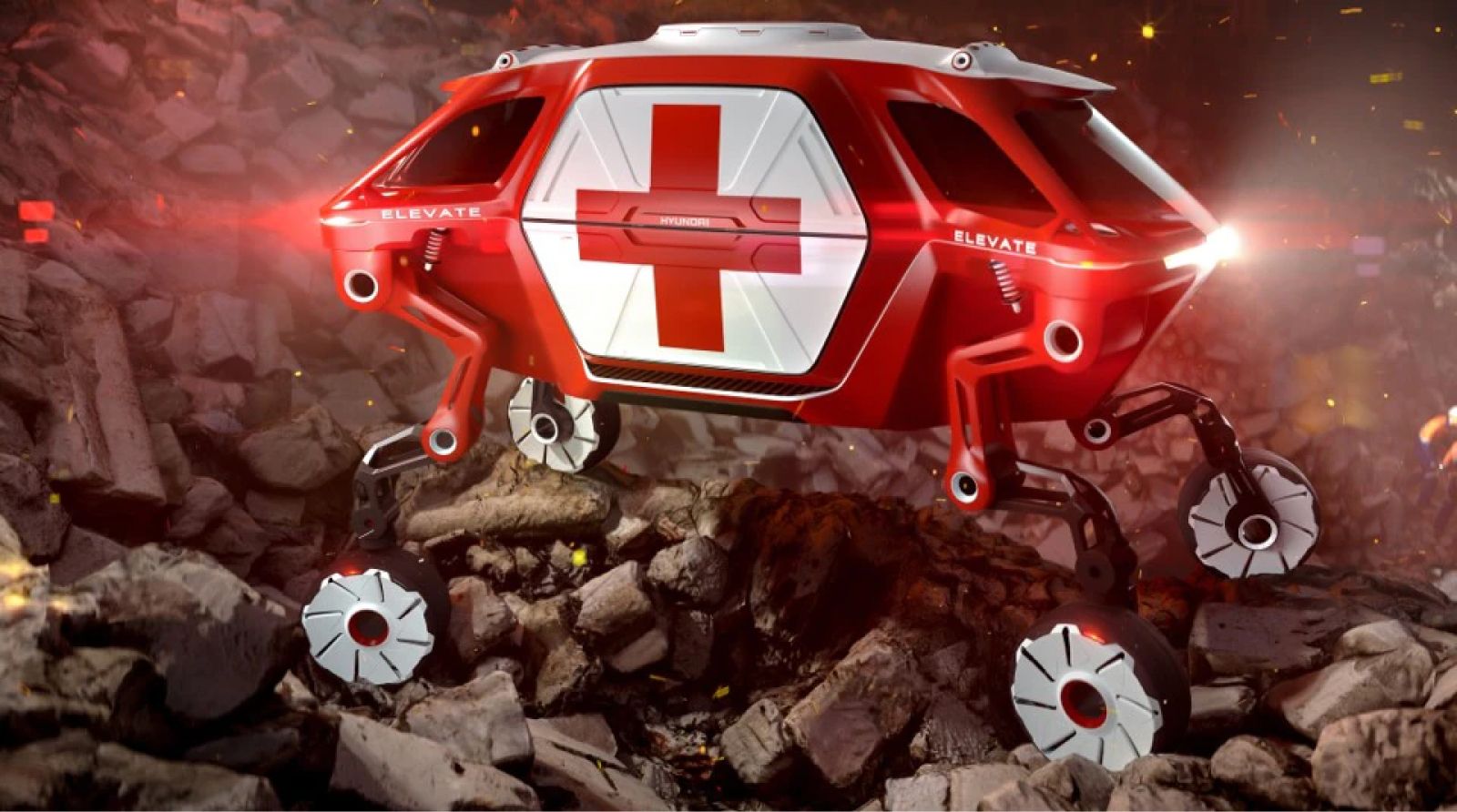
Heading: Image title: Hyundai’s walking vehicle
Image source: autodesk.com — Driving mobility innovation with generative design
Generative design really allows us to tackle complex problems that would take somebody a lot more time than they have to go through different analyses. It’s a mind multiplier, I like to call it, where a single designer or engineer can go through perhaps dozens or hundreds of different design iterations. It allows them to see things that they may not have otherwise considered.
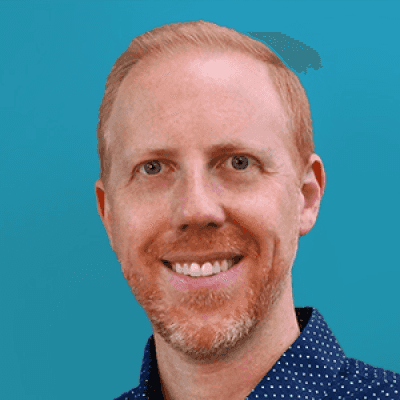
David Byron
industrial design manager at Sundberg-Ferar
BMW’s design simulation for prototyping
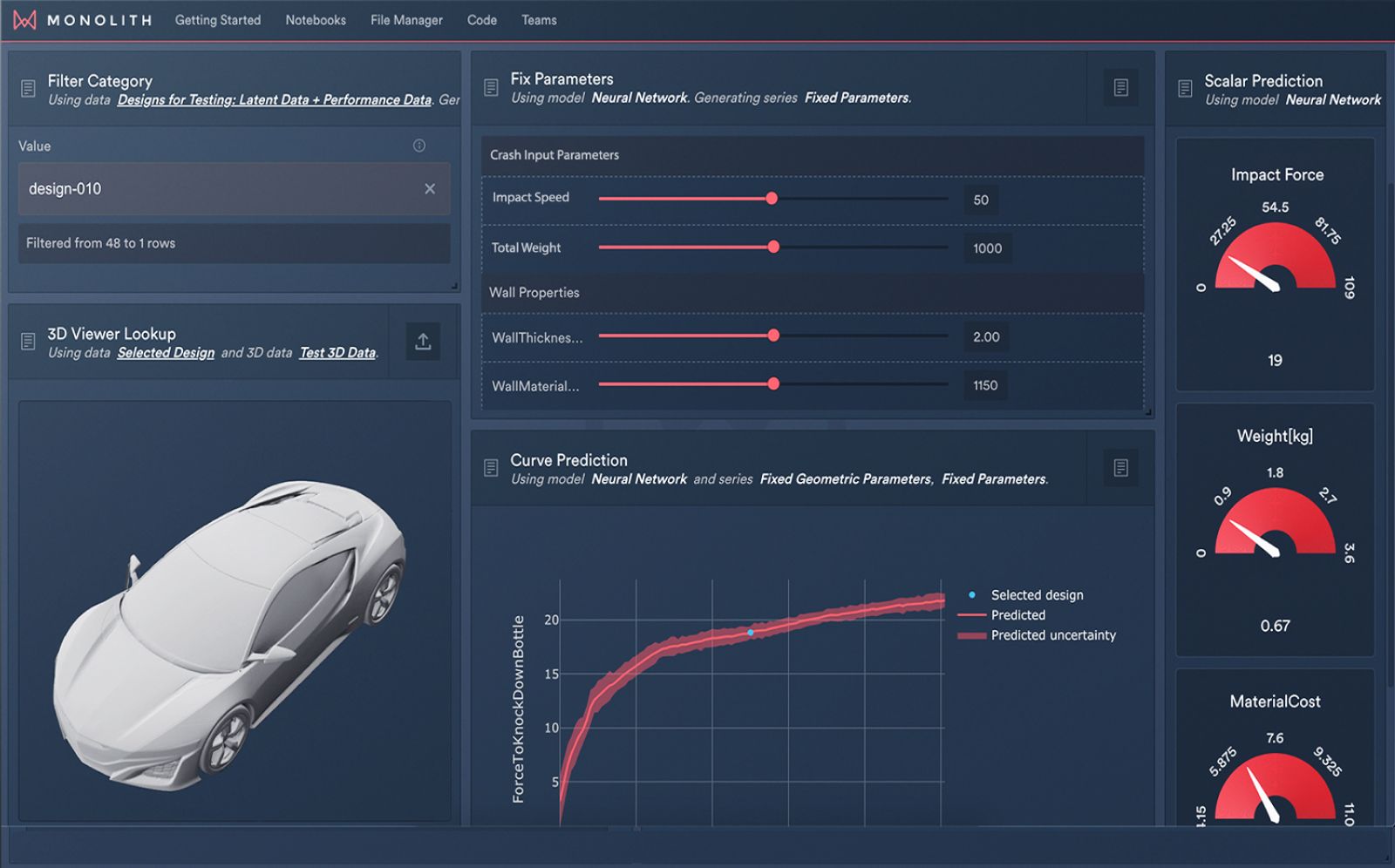
Image title: BMW’s AI-enabled engineering
Image source: monolithai.com — Monolith AI Software Accelerates Development of World-Class Vehicles
When the intractable physics of a complex vehicle system means it can’t be truly solved via simulation, AI and self-learning models can fill the gap to instantly understand and predict vehicle performance. This offers engineers a tremendous new tool to do less testing and more learning from their data by reducing the number of required simulations and physical tests while critically making existing data more valuable.
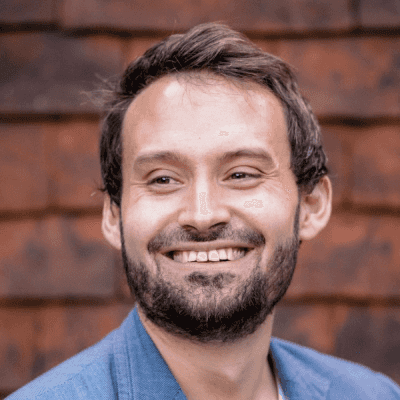
Dr Richard Ahlfeld
CEO and Founder at Monolith
Audi’s vehicle quality control
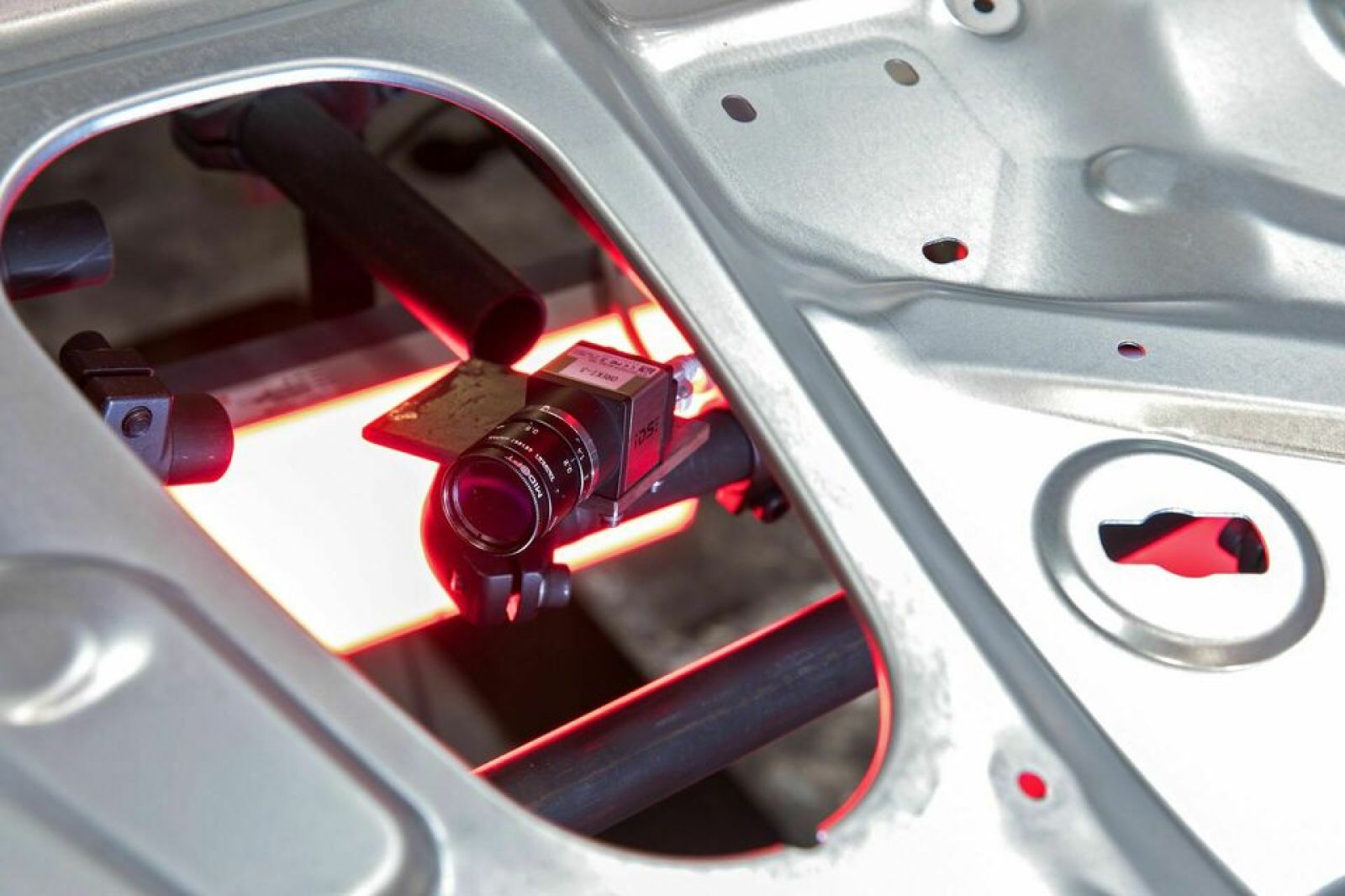
Image title: An AI-powered camera for quality control of Audi cars
Image source: audi-mediacenter.com — Audi optimizes quality inspections in the press shop with artificial intelligence
Mercedes-Benz’s autonomous vehicle system
Tesla’s Trip Planner navigation feature
AI in automotive adoption challenges
Despite the many benefits that AI can bring to drivers and automakers, companies should consider potential issues associated with implementing this technology.
Concerns
The data-driven nature of AI implies that automotive solutions leveraging it should process large, high-quality datasets as well as streams of real-time information from relevant sources to perform analytical and operational tasks.
AI-powered solutions feature multi-layered architectures with interconnected components like IoT sensors and data analytics software, which can rely on different communication protocols to exchange multiple types of data. Poor interaction between such components results in information silos, inconsistent data, and unreliable analyses.
Recommendations
Configure ETL/ELT pipelines to integrate heterogeneous data, cleanse it, and consolidate it into a suitable data storage (data lake, TSDB, etc.). Cloud service providers offer cloud data integration solutions like Azure Data Factory and AWS Glue to facilitate this process.
Set up necessary application program interfaces (APIs) to enable seamless data exchange between components. Once again, you can rely on cloud-based services like Azure API Management and Amazon API Gateway.
Consider using middleware architectures like ESB to convert incompatible communication protocols, or data virtualization techniques to access data stored in other components without having to move or copy it.
Connectivity
Concerns
Several use cases of AI in the automotive industry involve data exchange between geographically distributed systems, including IoT sensors gathering vehicle information and data analytics solutions processing it. This requires very stable networks, especially when data processing needs to be done in real time, such as for route optimization.
Getting a reliable internet connection becomes even more difficult when experiencing load spikes or blind spots, such as tunnels, which can cause high latency and lead to inaccurate analytics.
Recommendations
Deploy IoT sensors loosely coupled to other components of your AI solution according to the publish-subscribe pattern. Such devices can store data in an offline message queue in the event of poor or no connectivity and transmit it once the connection is restored.
Adopt edge computing to distribute part of the computing workload (typically handled by centralized data centers) across IoT devices. This will help reduce network dependency and mitigate latency risk.
Rely on bandwidth-efficient communication protocols, such as MQTT.
Data analysis
Concerns
Processing big data and data streams gathered from manufacturing plants or fleets of vehicles requires remarkable computing resources, enabled by a robust but potentially pricey technology infrastructure.
Machine learning algorithms require massive training data sets to build an AI model capable of deriving reliable insights.
AI model reliability can be hindered by overfitting (a model overtrained on a specific data set performs poorly when processing other data) or model drift (its performance degrades over time due to progressive changes in input variables).
Recommendations
Complement your computing resources with cloud-based AI and ML services. These typically provide built-in algorithms, pre-trained ML models, and scalable processing power. Amazon SageMaker and Azure Machine Learning are popular options in this regard.
Implement a masterless cluster architecture to scale up and down the processing nodes supporting your IoT network according to the actual workload.
Split your data for AI model building into training, validation, and test sets to prevent overfitting. Regularly monitor model drift based on metrics like the Population Stability Index and execute retraining iterations to update the model with fresh data.
Data privacy & security
Concerns
As the volumes of data required by AI systems for training and analysis increase, so do security risks. Fraudsters and cybercriminals can target both private drivers and automakers, with threats ranging from car hacking to intellectual property leakage.
Most of the information processed by automotive AI solutions, such as video footage collected by dash cams or geolocation data, is classified as personal data. Current regulatory frameworks impose significant limitations on the collection of this information.
Recommendations
Automotive data should be processed in line with the GDPR and other applicable standards and legislation, so make sure your AI solution complies with such regulations.
Whenever possible, train your AI models with obfuscated data, namely data modified via data masking techniques like encryption to anonymize it.
Minimize cyber exposure of your AI system and related data by implementing effective security measures. These can include cryptographic key management systems exclusive to each vehicle, dynamic data masking for your databases, and device authentication options like X.509 certificates for your IoT sensors.
Our AI services
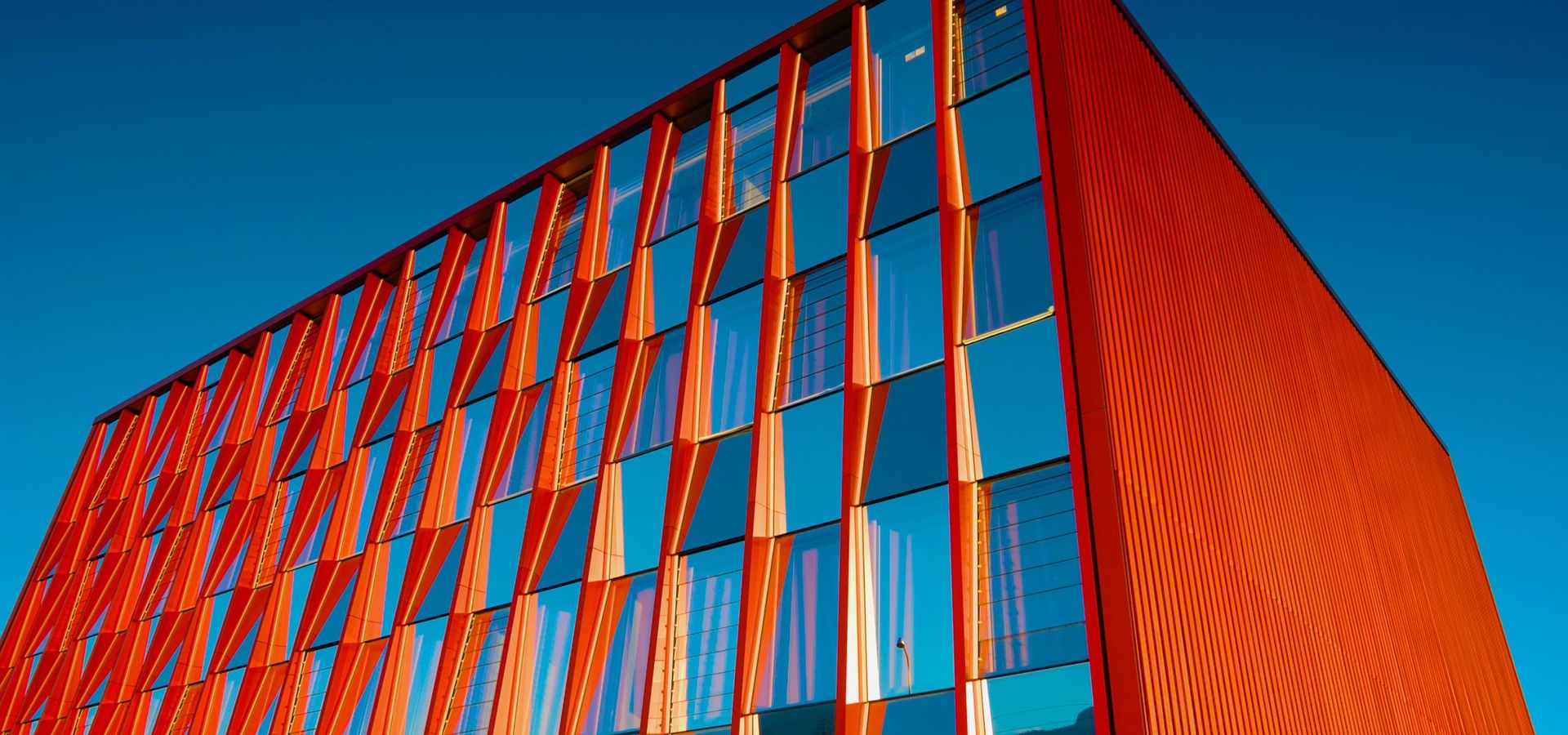
AI consulting
Our consultants provide expert guidance to plan and supervise your AI software development and implementation, helping maximize the adoption benefits of this technology and address technical roadblocks.
AI development
Itransition delivers AI-powered automotive software aligned with your business needs or modernizes your existing solution to meet evolving goals and embrace emerging market and tech trends
Transforming the automotive industry with AI
The automotive industry is set to transform radically, thanks to advances in AI technology. From manufacturing and design to sales, marketing, and servicing, AI can play a key role in making cars smarter, safer, and more efficient. Additionally, the inevitable shift from hardware to software in the automotive industry requires vehicle manufacturers to reimagine their workflows and pay close attention to the relevant regulatory frameworks. If you are planning to implement a robust AI solution to embrace the numerous tangible benefits the technology offers, consider collaborating with an expert partner like Itransition.
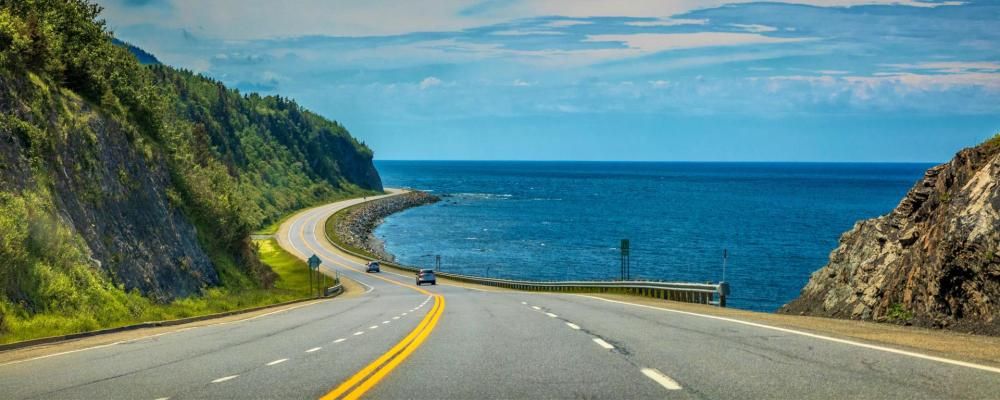
Insights
Automotive IoT: applications, real-life examples & technologies
We explore IoT applications in the automotive industry, describe the most prominent real-life examples, and explain the architecture of connected cars.
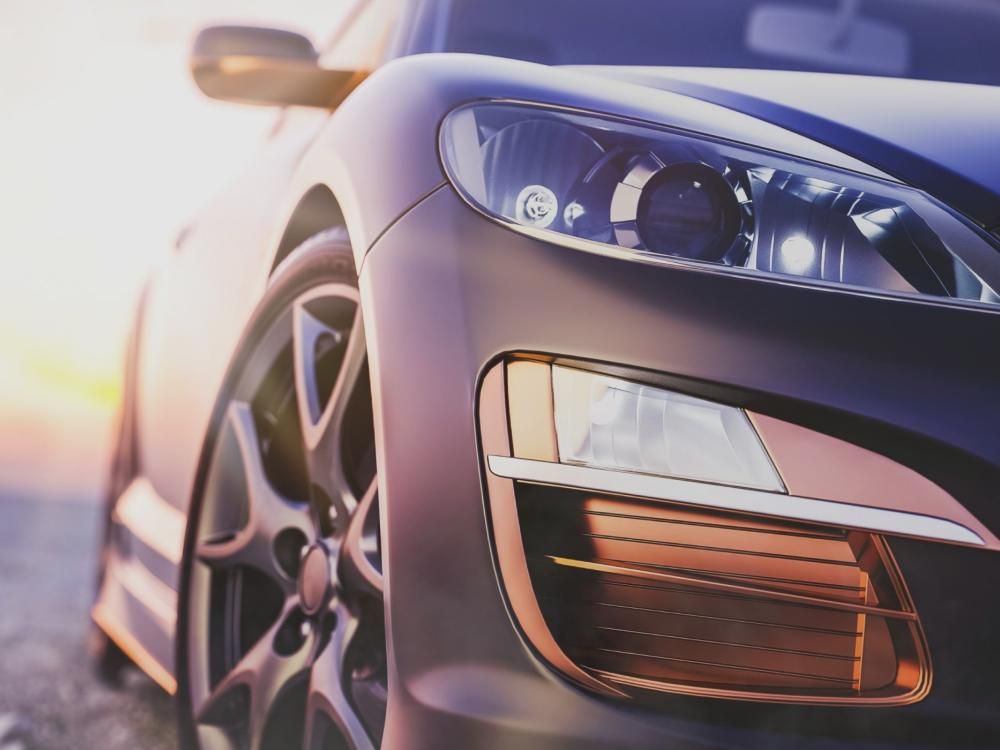
Case study
Cloud business intelligence system for vehicle manufacturers
Find out how Itransition migrated a BI suite to the cloud and delivered brand-new cloud business intelligence tools for the automotive industry.
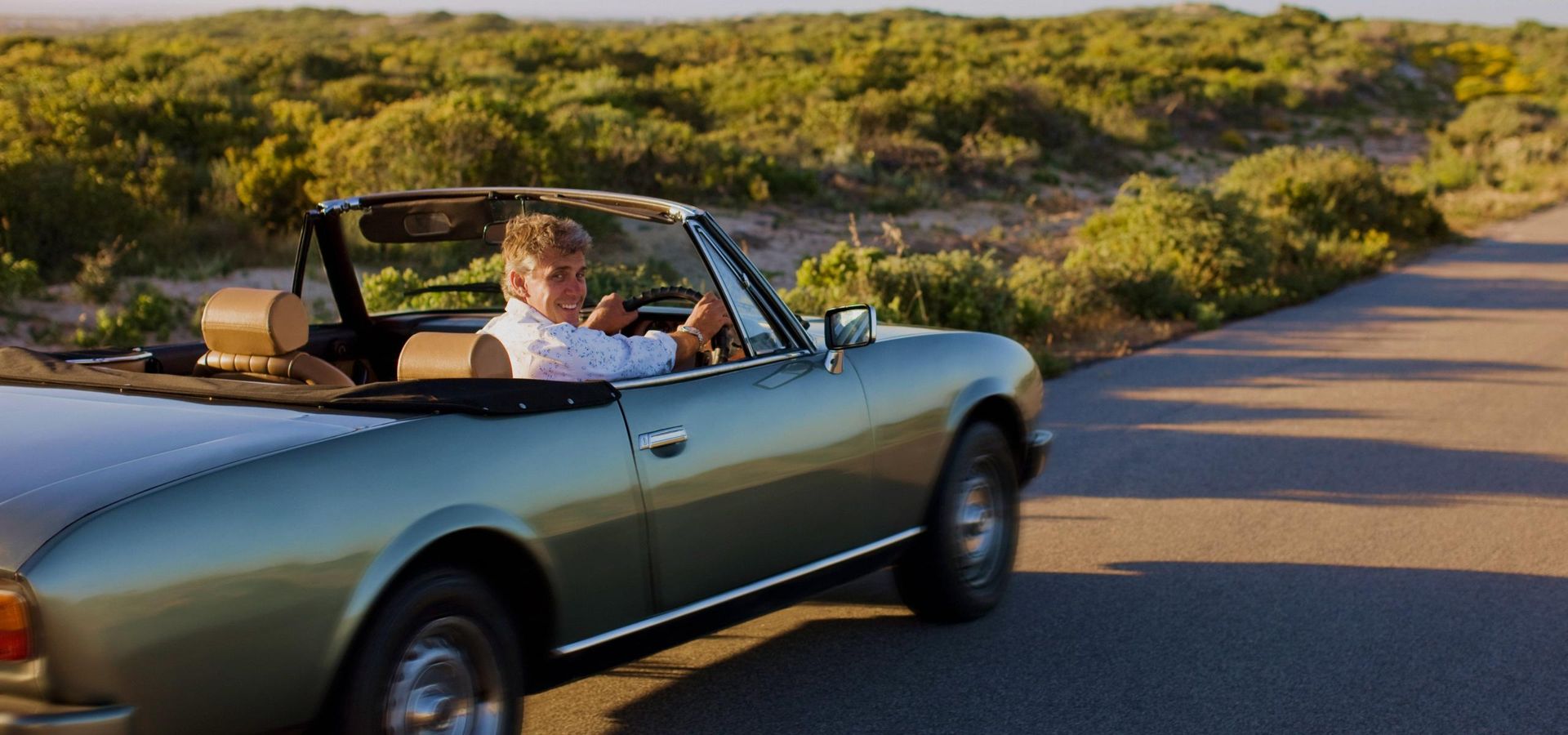
Service
Automotive ERP: key functionality, integrations & software options
Itransition presents an overview of ERP solutions for the automotive industry and their benefits, features, key integrations, best software & selection factors.
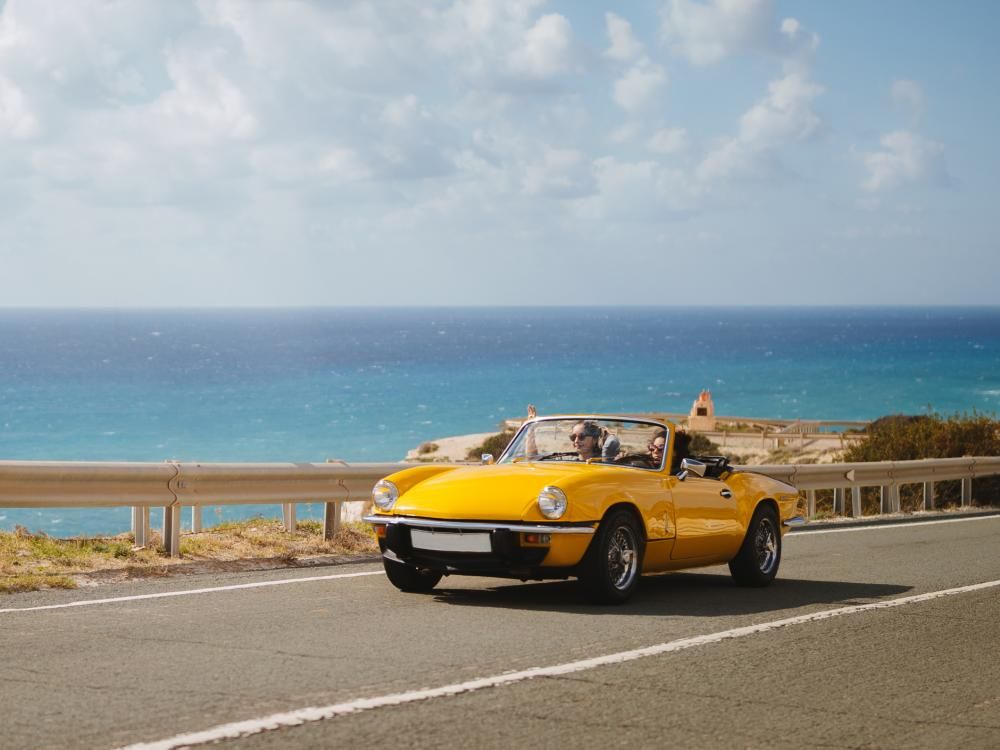
Case study
Vehicle data platform for an automotive startup
Learn how Itransition helped an automotive startup launch a SaaS platform that transforms how vehicle owners and companies work together for mutual benefit.
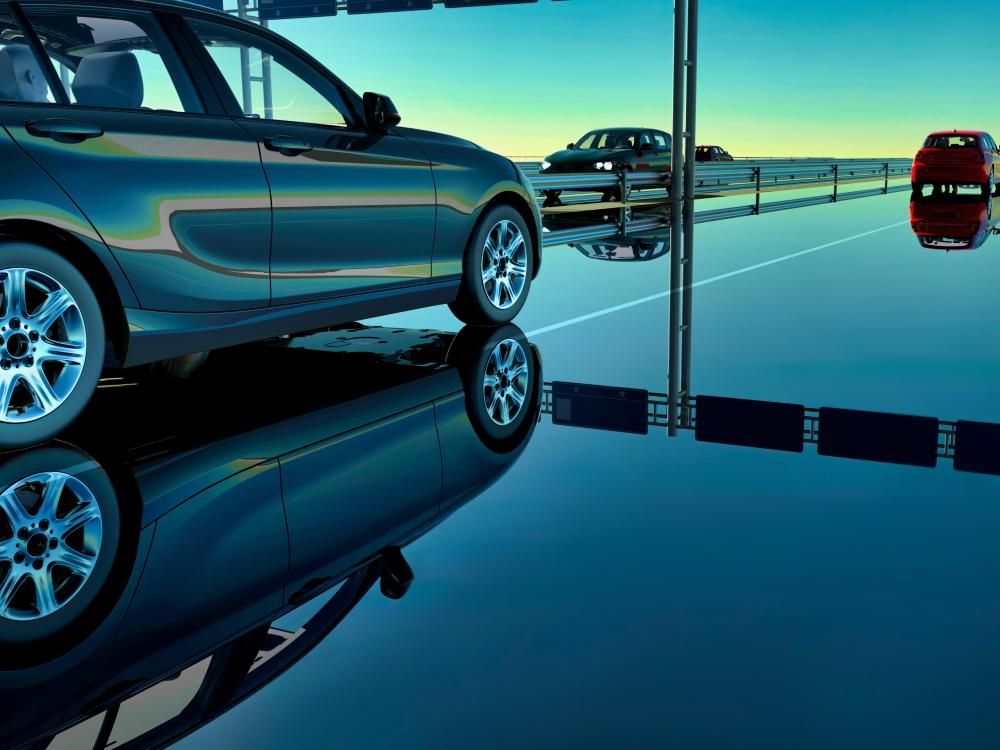
Insights
CRM for the automotive industry: key features, benefits & best platforms
Discover automotive CRM’s recommended features, adoption payoffs, and best platforms on the market, along with Itransition’s range of CRM services.
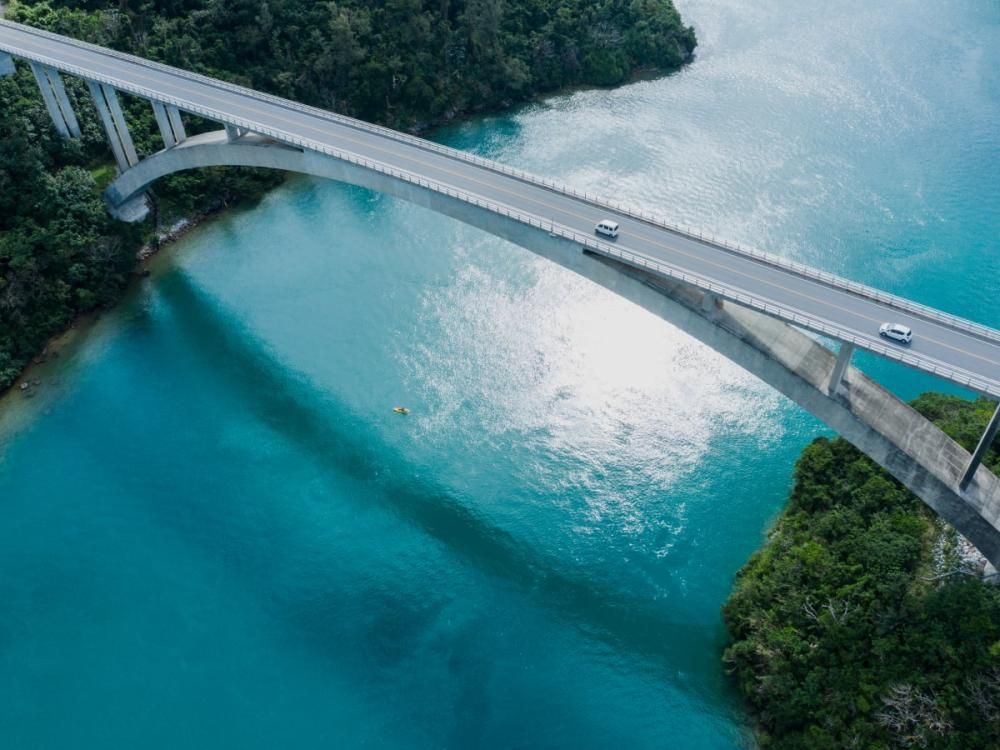
Insights
RPA in the automotive industry: key use cases, examples & best practices
Learn how to improve the efficiency of your automotive business processes and reduce operational costs with robotic process automation implementation.