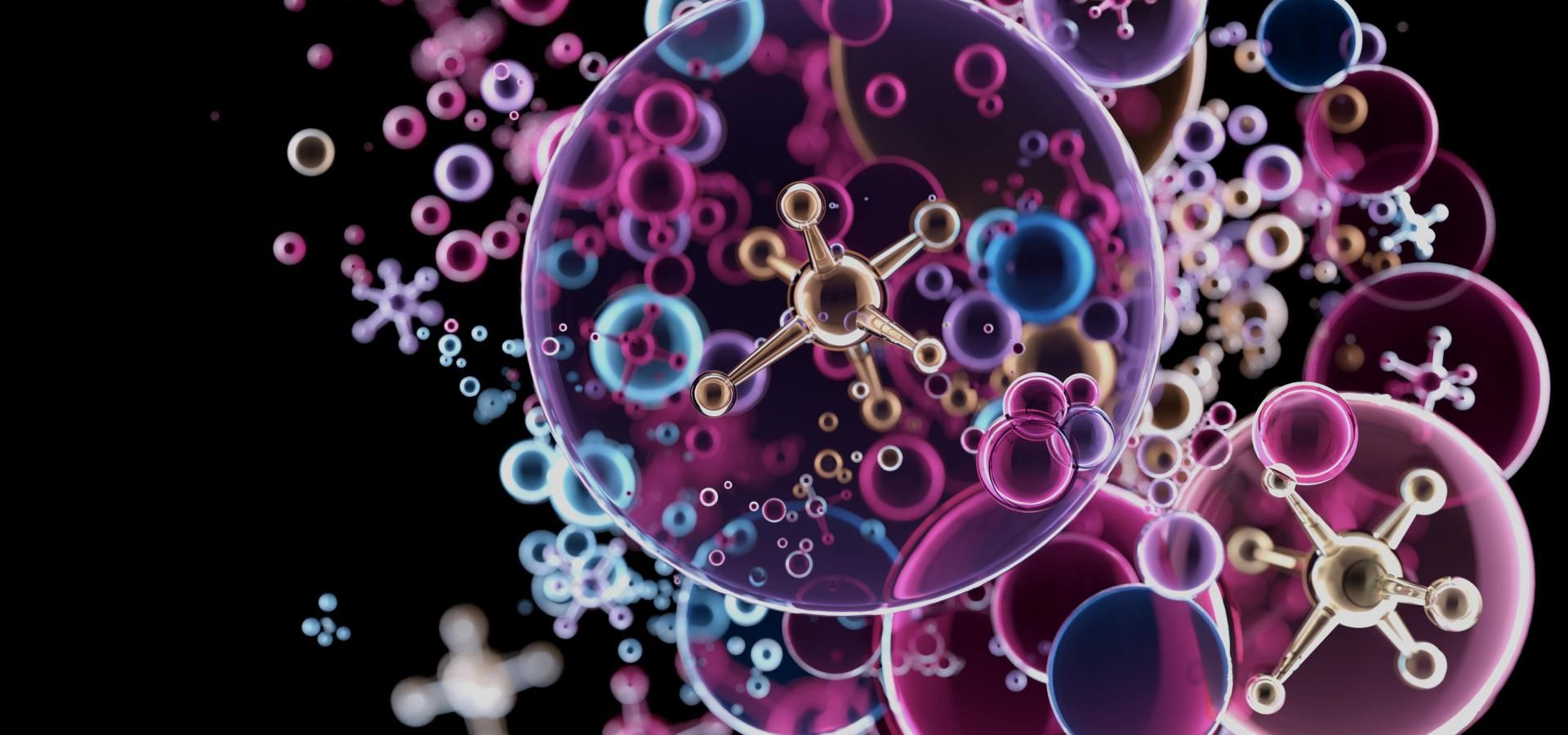
The role of machine learning in healthcare: use cases & real-life examples
February 13, 2025
- Home
- Machine learning
- Healthcare
Machine learning in healthcare helps draw insights from patient data and medical records and make informed decisions and accurate predictions based on them to automate healthcare professionals’ daily workflows, personalize medicine, accelerate medical research, and increase operational efficiency.
With 5+ years of machine learning expertise, Itransition can help you seamlessly integrate machine learning into your medical practice to streamline clinical data processing and enhance patient care.
Table of contents
Machine learning in the healthcare market overview
According to WHO, 10 million more health workers will be needed by 2030. However, the shortage of skilled medical professionals can be partially mitigated by artificial intelligence and machine learning technologies that can improve the efficiency of care delivery and free healthcare professionals' time, allowing for a more personalized approach to each patient. Here are some other relevant statistics about machine learning in healthcare:
share by application, 2022 (%)
Scheme title: Global AI/ML technology in the healthcare market
Data source: grandviewresearch.com — AI in healthcare market, 2024–2030
37.5%
CAGR of the global AI/ML in the healthcare market from 2023-2030
Grand View Research
$102.7 bn
expected AI in the healthcare market size by 2028
Research and Markets
17%
more time physicians can dedicate to patients due to AI implementation
Statista
Top nine use cases of machine learning for healthcare
There are multiple applications of machine learning in healthcare, from patient apps, e-triage tools, and online symptom checkers to virtual agents and bionic pancreas for patients with diabetes. Here are some popular ML use cases and real-world examples that demonstrate its potential value for healthcare practices.
Disease outbreak prediction
Nowadays, satellites can collect massive data volumes, including real-time and historical environmental data. Predictive analytics tools help aggregate this big data and forecast potential disease outbreaks. One example is predicting malaria outbreaks by analyzing monthly rainfall, temperature, and similar parameters. This can be particularly relevant in third-world countries that lack medical infrastructure to combat those diseases. Using predictive models to forecast such critical outbreaks upfront, governments can take precautions to minimize the negative impact and save lives.
Example: ProMED reporting disease outbreaks online
ProMED (the Program for Monitoring Emerging Diseases) offers an online real-time data analysis and reporting system showing outbreaks of infectious diseases worldwide and any exposure to toxins affecting human or animal health. ProMED aggregates data from various sources, including official, media, and subscriber reports. An expert team reviews these reports before they are accepted into the system. The data provided by ProMED is aggregated in a HealthMap to visualize disease outbreaks in every country.
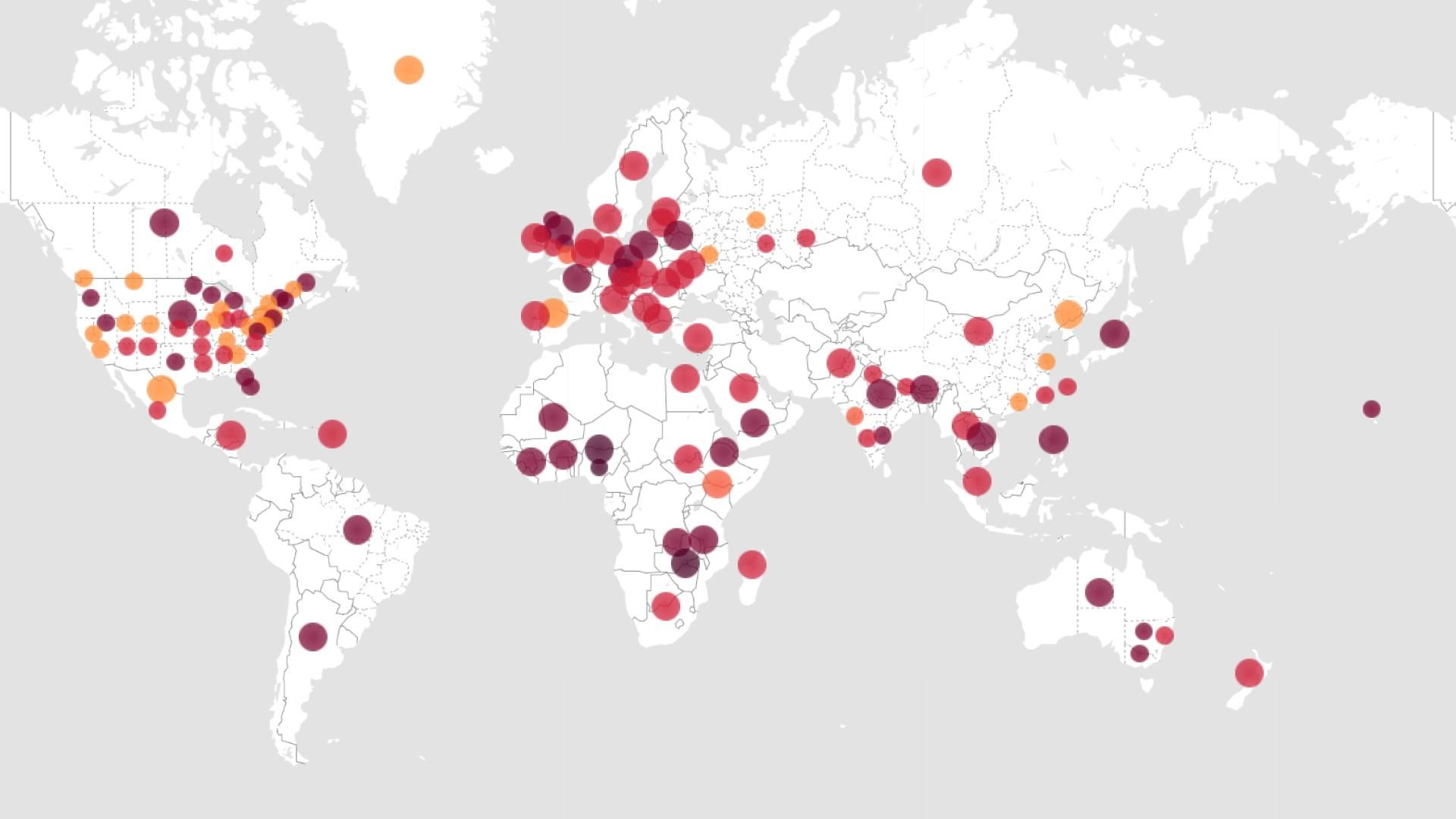
Image title: HealthMap by ProMED
Image source: healthmap.org
Patient behavior modification
Many prevalent diseases are manageable or even avoidable. For example, type 2 diabetes, obesity, and heart diseases in some cases can be detected at early stages or avoided by practicing a healthier lifestyle. However, adjusting the lifestyle requires a change in behavior with the help of constant reminders and follow-ups. For this purpose, machine learning algorithms can aggregate health data incoming from patients' connected medical devices and sensors to generate insights into their behavior and guide them during this transformational journey.
Example: Altering smoking habits with SmokeBeat
SmokeBeat is a smoking cessation app that passively gathers data on the user’s smoking behavior. The application uses an accelerometer on a smartwatch or a smart band to detect hand-to-mouth gestures. SmokeBeat processes this data and offers real-time cognitive behavior therapy incentives. User responses to those incentives are constantly measured and recorded to improve effectiveness. Additionally, SmokeBeat compares users' smoking data with their peers of choice, creating a supportive social network.
Virtual nursing
Healthcare facilities use virtual nurses to streamline multiple processes, including appointment scheduling and reminders, disease progression tracking, medication and chronic condition management, post-discharge care, health alerts, and emergency response. Virtual nurses are computer-generated avatars that are designed to be social, empathic, and informative and can interact with patients. They are available 24/7 and can quickly answer patient questions in-between doctor visits.
Example: Streamlining remote care with Molly
One example of a virtual nurse is Molly. This female avatar can remotely monitor medical conditions, receiving data such as blood pressure and weight from patients' monitoring devices connected via Bluetooth. These devices are positioned in patients' homes, which makes it convenient to take measurements as often as needed. Molly can recognize speech and verbally answer patients' queries. It also offers a chatbot for private discussions.
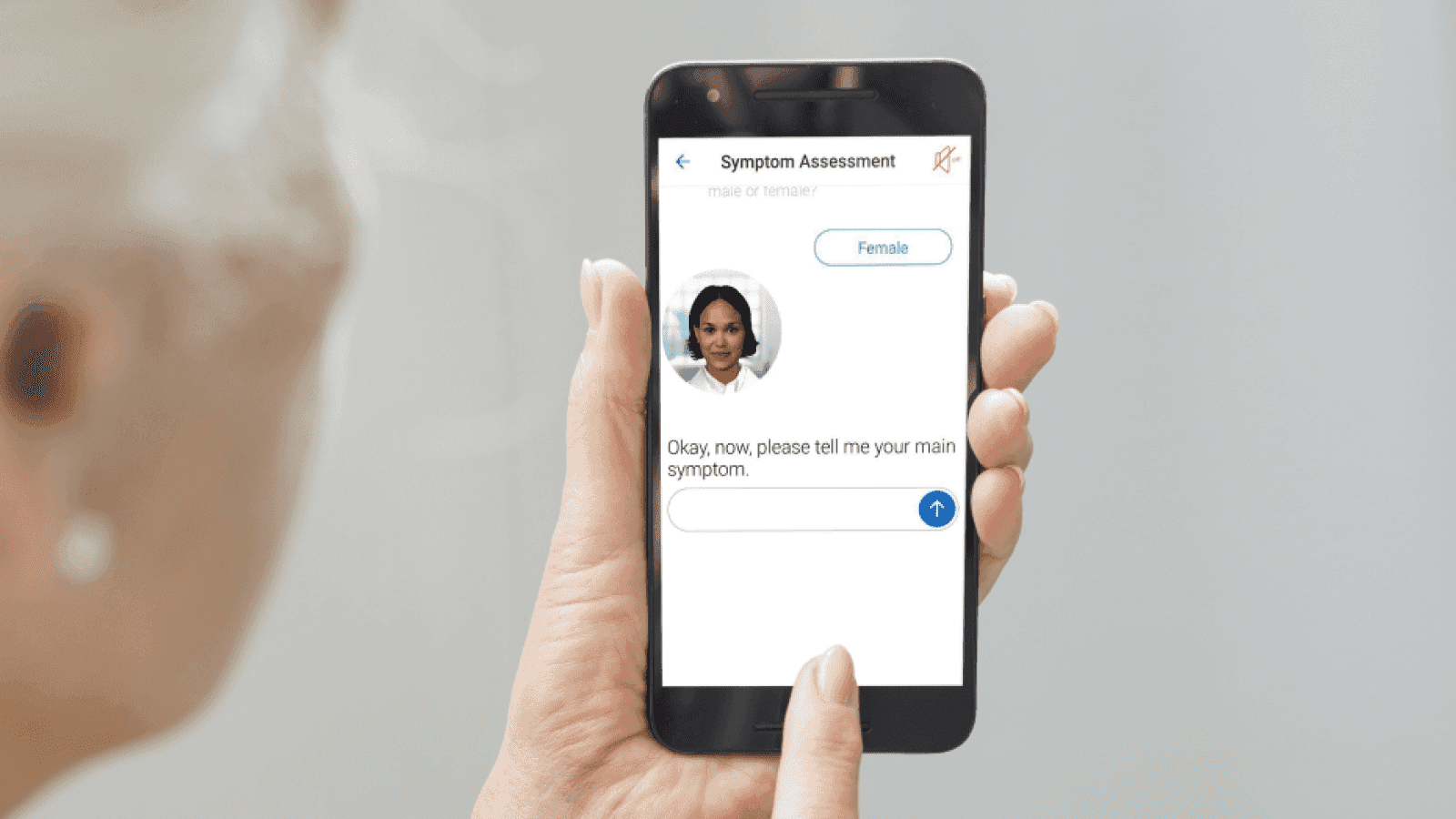
Image title: Virtual nursing
Medical imaging
Even with all the advancements in healthcare technologies and data science, radiology and medical image analysis is a tedious task prone to human error since it requires great attention to detail. Advanced medical image analysis software powered with machine learning and artificial neural networks, in turn, can identify even the subtlest changes in X-ray, CT, or MRI medical scans and help radiologists detect lesions, tumors, and other abnormalities for faster disease diagnostics.
Example: Improving image quality with SubtleMR
For example, SubtleMR, developed by Subtle Medical, is a machine learning-based healthcare application that improves the quality of MRI protocols. With the help of denoising and resolution enhancement, SubtleMR improves image quality and the sharpness of any MRI scanner. For example, RadNet, a US leader in outpatient imaging with 335 centers nationwide, accelerated its protocols by 33-45% after adopting SubtleMR technology.
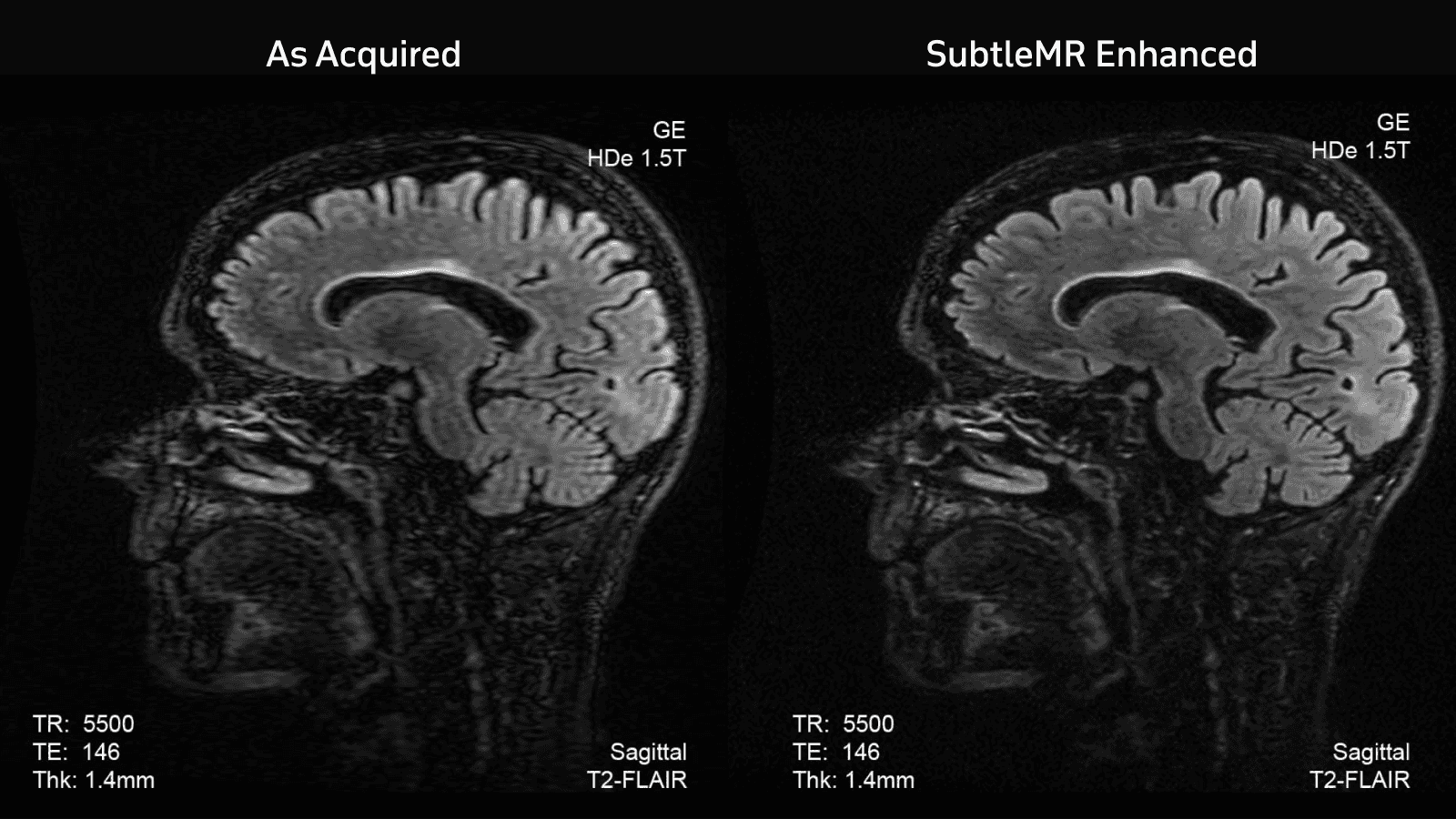
Image title: SubtleMR
Image source: subtlemedical.com
Accurate diagnostics & identifying high-risk patients
In healthcare, inaccurate or incomplete diagnosis can be detrimental to patient outcomes and, in the worst-case scenarios, lead to death. To address one of the most apparent healthcare challenges, many companies are implementing machine learning to make medical diagnostics more accurate. Moreover, by combining machine learning-powered abnormality and pattern recognition, clinicians can considerably reduce the time it takes to identify high-risk patients. ML systems can process PHI and stratify them into different risk groups based on the detected risk levels.
Example 1: Predicting syndromes with Face2Gene
The Face2Gene precision medicine app uses machine learning-enabled facial recognition technology that helps clinicians diagnose rare diseases more accurately. With the help of machine learning, Face2Gene can detect phenotypes, reveal relevant facial features, and evaluate the probability of a patient having a particular syndrome.
Example 2: Detecting high-risk patients with UiPath & Amitech
A mixture of optical character recognition and natural language processing helps structure and organize patient records. Then an RPA bot feeds this data to a machine learning system that scores patients for risks, provides them with a tailored healthcare plan, and alerts the appropriate clinicians and care managers about high-risk patients so the former can take immediate action. Besides significantly improving patient outcomes, the process now takes only minutes instead of days or weeks.
Robot-assisted surgery
Using robots in healthcare systems is not a new trend. ML-powered robotic surgery assistants increase precision, can access different areas of the human body with minimal intervention, and reduce the length of some operations, alleviating the pressure from human surgeons. Robots can assist in performing complex surgeries, minimizing patient trauma and recovery time, and are indispensable in microsurgery.
Example: Improving surgical outcomes with Senhance Surgical System
Senhance is a console-based, multi-armed surgical system that allows surgeons to control it remotely. The system heavily relies on machine learning and deep learning models to bring the most challenging healthcare ideas to reality. For example, during the preoperative stage, a machine learning-driven database allows surgeons to go through simulation training. Then, during surgeries, based on data from the eye-tracking camera, the system's Intelligent Surgical Unit can automatically adjust the camera view and predict when a surgeon needs to zoom in or enhance images in real-time.
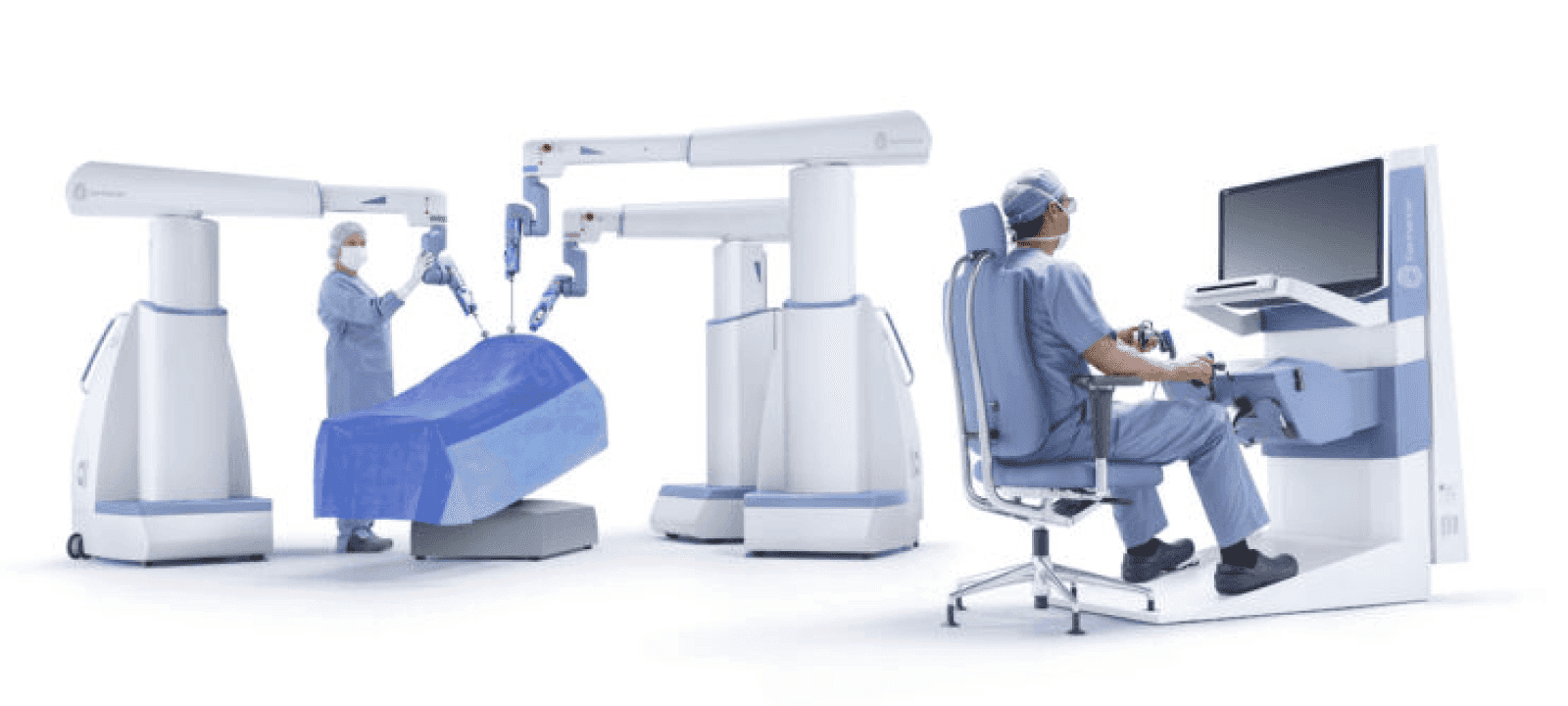
Image title: Senhance Surgical System
Image source: surgicalroboticstechnology.com—Russia Introduces First Asensus Surgical Senhance Robotic System
Drug discovery
Drug discovery is an expensive and lengthy process. Researchers test thousands of elements and their combinations until one of them becomes a viable drug. Machine learning algorithms are used in the drug discovery process for the following purposes:
- Minimizing clinical trial duration by predicting how potential drugs will perform
- Identifying combinations of existing drugs that can form a new treatment option
- Discovering new drugs based on compound testing
- Finding new uses for previously tested substances
Example 1: Accelerating oncology research with IBM Watson
The pharmaceutical company Pfizer is using IBM Watson for its immune-oncology research. While a human researcher can read around 300 articles annually, Watson processes one million journal articles and data on four million patents. Using machine learning-generated insights, Pfizer employees can identify non-obvious connections and help create treatment plans out of drug combinations.
Example 2: Finding cures to diseases with Google’s Deep Mind
Another example is AlphaFold, a machine learning-powered system built by Google’s DeepMind that can automatically predict protein structures. Accurately predicting how different proteins interact with each other is a vast biological breakthrough, as it can significantly accelerate drug screening and development. In 2021, Google also launched Isomorphic Labs, a company that will use AlphaFold’s technology to find cures for prevalent diseases.
Hospital management optimization
With the ever-growing demand for healthcare services, hospitals’ enterprise management systems are becoming increasingly chaotic. However, carefully tuned machine learning-based systems can master administrative data processing and facilitate most hospitals’ executive functions, including staff scheduling, supply chain and inventory management, resource allocation, medical records management, and compliance monitoring.
Example: Optimizing staffing with Globus.ai
A Norway-based company Globus.ai created a system to help healthcare institutions streamline staffing. With the help of natural language processing and machine learning, the system can match healthcare employees to specific tasks based on their skill sets, making task scheduling far more efficient. Importantly, Globus.ai’s system considers legal requirements when making scheduling decisions. For example, in some cases, the law limits the number of working hours or requires a professional with particular expertise to be present during a certain procedure.
Health insurance
Health insurance is an essential component of the healthcare industry that is key in making healthcare accessible. However, there is plenty of room for improvement in mostly manual-based processes of health insurance. For example, machine learning-enabled pattern recognition algorithms can assist in the early detection of fraudulent activities. Rule-based fraud detection systems that the majority of health insurers currently use can flag too many claims as potentially fraudulent. Machine learning systems, on the other hand, learn and gradually decrease the probability of false positives. Machine learning can also help automate different health insurance processes, including credit underwriting, risk assessment, claims to process, and customer support.
Example: Optimizing health insurance with Maya Intelligence
For example, Temple University Health System (TUHS), a nationally recognized academic health system in Philadelphia, partnered with Accolade, which provides the Maya Intelligence platform to help patients choose the most appropriate healthcare coverage option. The system utilizes machine learning to analyze medical claims, lab results, and other relevant patient information to offer tailored healthcare plans to patients. As a result of the implementation, TUHS has saved more than $2 million in healthcare claim costs and achieved a 50% increase in employee engagement.
Enhance your healthcare services with machine learning
Five real-life examples of machine learning in healthcare
Numerous companies worldwide use machine learning to increase the quality, accessibility, and interoperability of healthcare systems with the ultimate goal of improving patient health.
The Viz.ai tool uses machine learning algorithms to optimize care coordination and improve communication between healthcare professionals. Viz.ai streamlines care by quickly connecting frontline healthcare providers to specialists, resulting in faster case resolution and improved patient outcomes.
The Deep Genomics’ artificial intelligence-powered platform accelerates research by helping healthcare professionals quickly find candidates for the development of drugs for specific disorders. The company uses machine learning to identify and trace potential causes of genetic diseases. The system can figure out how a person’s mutation changes their DNA and triggers disease. Even better, the ML-enabled solution can analyze millions of potential medicines within hours and tell researchers which ones are most likely to be effective.
Intuitive Surgical is the developer of the most widely used machine learning-powered surgical system called Da Vinci. The Da Vinci Surgical System allows surgeons to perform robotic-assisted, minimally invasive surgeries that significantly improve surgery outcomes.
Oncora Medical is a Philadelphia-based startup that streamlines cancer research and treatment. By collecting large amounts of data from medical records, EHRs, cancer registries, and other software systems, Oncora’s platform can assess care quality and suggest better treatment methods. Oncora treats the development of machine learning models in the same way a biopharmaceutical company approaches medicine development. Each ML model undergoes a thorough assessment consisting of several phases.
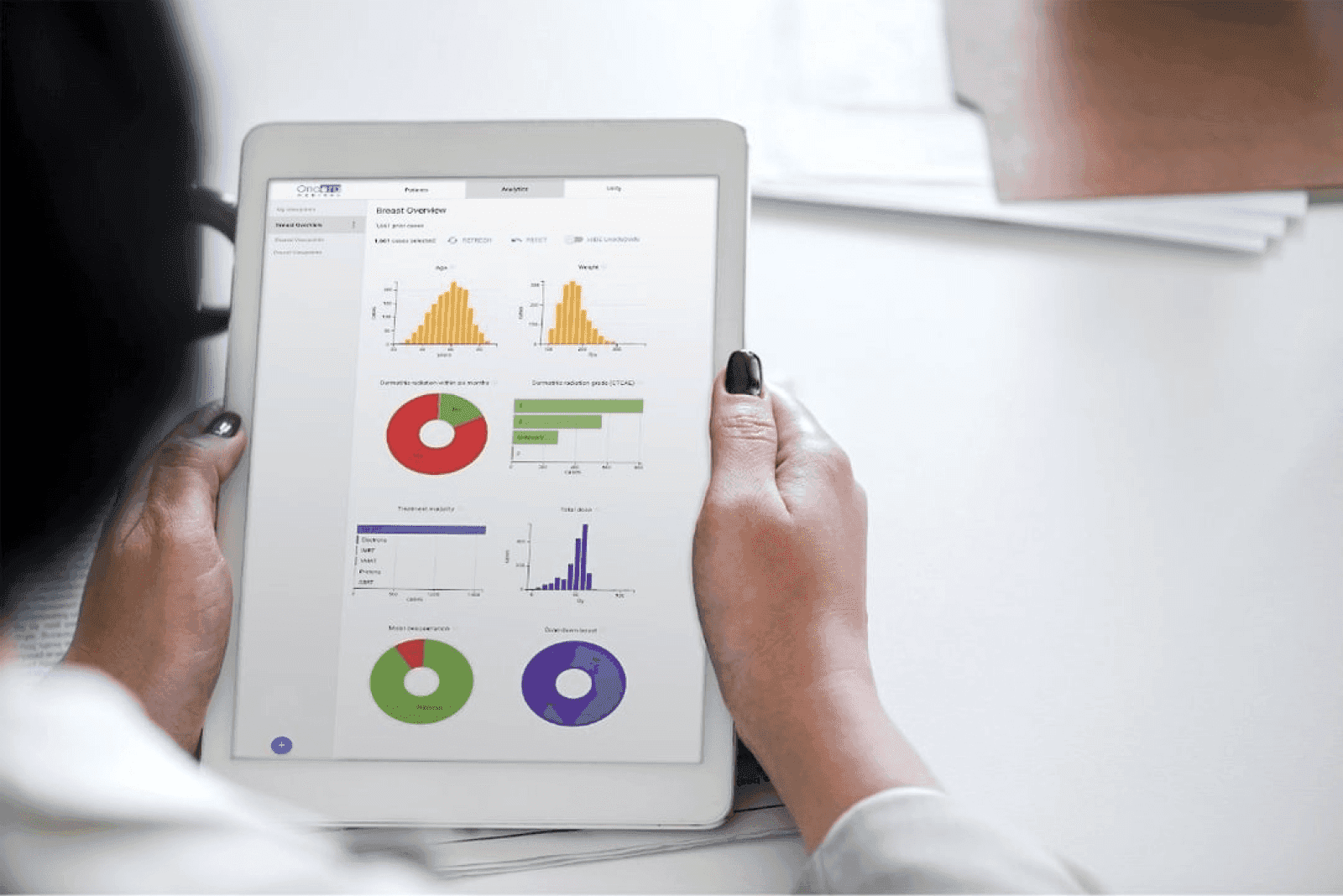
Image title: Oncora ML-powered analytics solution for cancer treatment
Image source: oncora.ai
PathAI uses machine learning to help pathologists make more informed diagnostic decisions. The company delivers algorithms for clinical trials and develops ML models that can optimize the analysis of patient tissue samples. PathAI works with renowned drug developers and healthcare organizations to extend the reach of artificial intelligence and machine learning in healthcare.
Seven benefits of machine learning in healthcare
Here are some of the advantages healthcare organizations gain by implementing machine learning into their clinical practices:
Automated routine tasks
From streamlining electronic health records management to virtual nursing, machine learning can help healthcare professionals automate many routine and repeatable tasks, reducing operational costs.
Improved decision-making
By detecting patterns in enormous healthcare data sets, machine learning helps clinicians streamline diagnostics and make data-driven decisions, therefore enhancing patient outcomes.
Enhanced patient experience
With the help of chatbots and virtual assistants, healthcare organizations can improve patient experience by streamlining access to basic healthcare services.
Accelerated innovation
By optimizing drug discovery and development, machine learning helps pharmaceutical companies reduce time-to-market and research costs.
Extended access to healthcare
With efficiencies unlocked by machine learning, organizations can help more patients without compromising care quality.
Reduced risks
ML adoption allows for early detection of serious illnesses, reduced risks during robot-assisted surgeries, and quick identification of high-risk patients.
Refined data governance
Machine learning algorithms can help healthcare organizations identify inconsistencies within disparate healthcare datasets, cleanse data, and validate its integrity.
Want to adopt machine learning in your healthcare practice?
Machine learning challenges in healthcare
While machine learning has immense potential to transform healthcare, it’s critical to consider the challenges and risks associated with its implementation.
Challenge
Solution
1Lack of data
The lack of clean, structured data is an overarching problem for organizations across every industry. Still, training and deploying value-adding machine learning models requires companies to reimagine their approaches to data governance. Given that datasets from one organization rarely suffice for model training, engineers obtain patient data from other healthcare organizations. The problem is that the majority of these datasets are incompatible with each other.
The lack of clean, structured data is an overarching problem for organizations across every industry. Still, training and deploying value-adding machine learning models requires companies to reimagine their approaches to data governance. Given that datasets from one organization rarely suffice for model training, engineers obtain patient data from other healthcare organizations. The problem is that the majority of these datasets are incompatible with each other.
Enforcement of industry-wide data governance frameworks is paramount at this point. Standardization of medical data requires equal effort from governmental bodies and industry players.
2Bias
While all machine learning applications can suffer from bias, its implications in healthcare are the most concerning. Since humans are responsible for training machine learning algorithms, our inherent biases inevitably influence the process. Moreover, ML models do not only sustain these prejudices but often amplify them.
While all machine learning applications can suffer from bias, its implications in healthcare are the most concerning. Since humans are responsible for training machine learning algorithms, our inherent biases inevitably influence the process. Moreover, ML models do not only sustain these prejudices but often amplify them.
To address the challenge of bias in machine learning for healthcare, companies have to ensure that training data is diverse and representative of all patient demographics, including different races and genders. Integrating human oversight plays a crucial role in preventing ML models from perpetuating biases: having healthcare professionals review and challenge the predictions made by algorithms guarantees that final decisions account for human context and empathy.
3Lack of strategy
In most cases, machine learning brings tangible long-term benefits when all parts of the organization support its adoption. However, since machine learning has a much more drastic impact on conventional healthcare workflows than the majority of other technologies, it can require some changes in usual processes.
In most cases, machine learning brings tangible long-term benefits when all parts of the organization support its adoption. However, since machine learning has a much more drastic impact on conventional healthcare workflows than the majority of other technologies, it can require some changes in usual processes.
Many organizations are reluctant to undergo significant changes for the sake of technology adoption, fearing the disruptions that such transformations can bring. However, a recent Deloitte survey shows that redefining team roles, investing in change management, and launching workforce reskilling programs are essential for increased productivity, improved innovation, and better employee engagement.
4Limited in-house expertise
Integrating such a complex technology as machine learning into intricate healthcare workflows requires both excellent technical skills and a deep understanding of medical science. On the one hand, many ambitious AI startups fail to incorporate clinical expertise during the early phases of development, while, on the other hand, many credible and experienced clinicians have insufficient understanding of machine learning to provide tangible input.
Integrating such a complex technology as machine learning into intricate healthcare workflows requires both excellent technical skills and a deep understanding of medical science. On the one hand, many ambitious AI startups fail to incorporate clinical expertise during the early phases of development, while, on the other hand, many credible and experienced clinicians have insufficient understanding of machine learning to provide tangible input.
It’s not only important to hire exceptional talent in multiple fields but also to ensure that data scientists, machine learning engineers, medical professionals, legal advisers, and other experts can collaborate with each other.
Unleash the power of machine learning
While the benefits of machine learning in healthcare are apparent and indisputable, its implementation requires significant resources and operational changes within medical organizations, which can't happen overnight. Still, while the demand for healthcare services is growing, the use of machine learning remains the best solution. For machine learning and artificial intelligence to solve healthcare legacy challenges, medical institutions must move beyond tests and pilot projects to implementing fully functional machine learning solutions. With solid experience in machine learning consulting and development and a deep understanding of the healthcare domain, Itransiton is ready to support this transition.
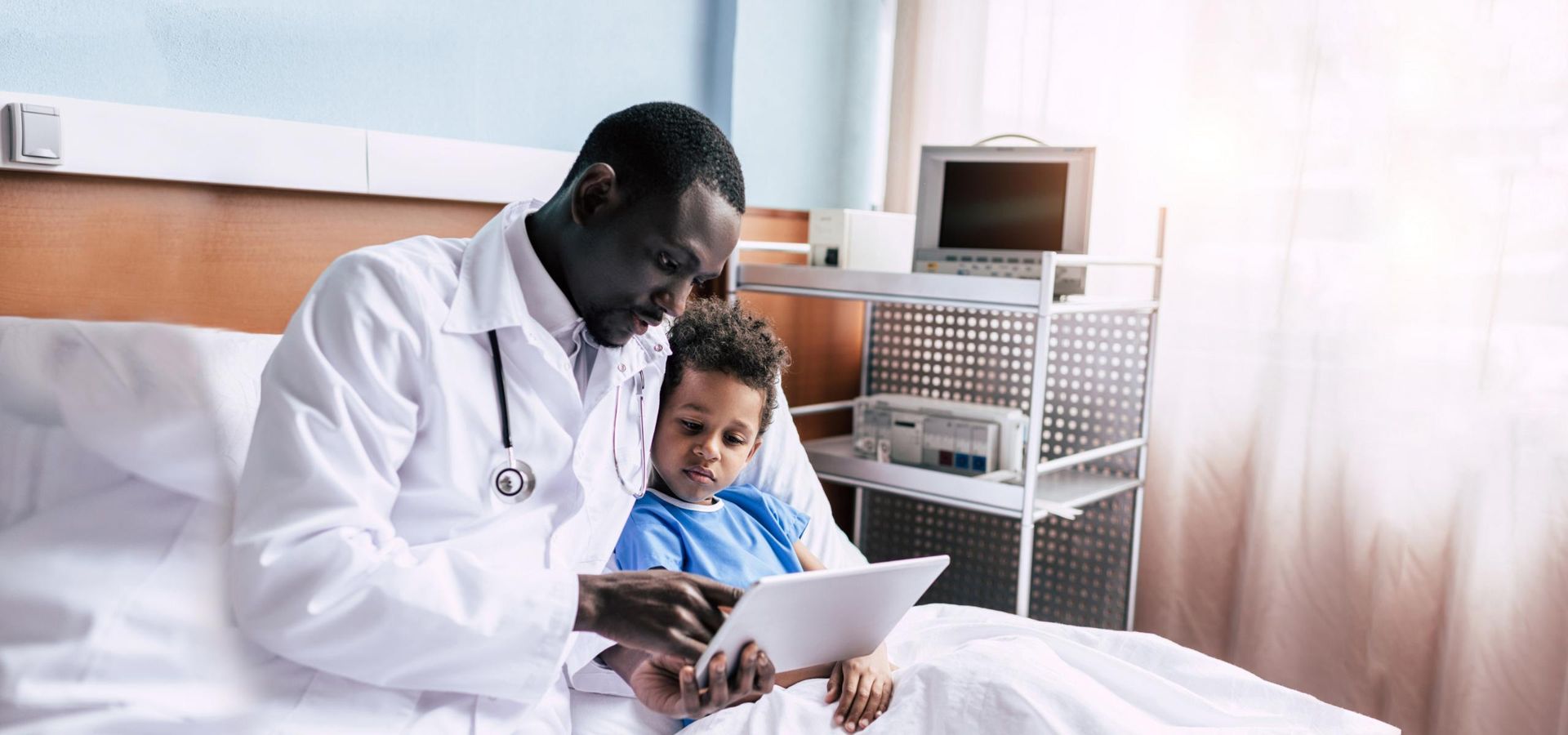
Service
Healthcare software development solutions & services
Entrust your medical software development to a company with 25+ years of experience in healthcare IT. Compliance and security guaranteed.
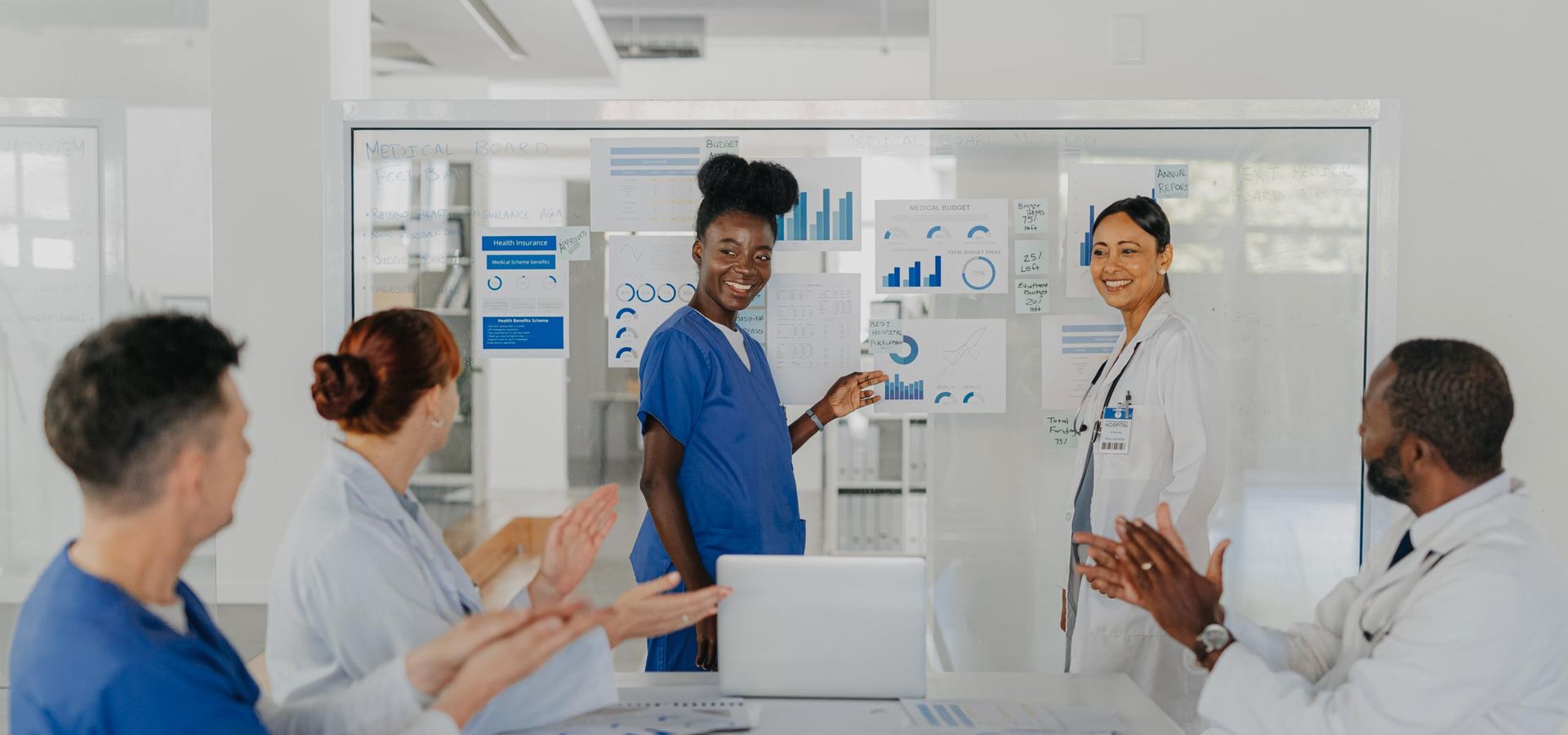
Service
Healthcare data analytics services: key types, features, and applications
Discover how our healthcare data analytics services can enhance patient loyalty while growing revenue and learn which solutions fit your business goals.
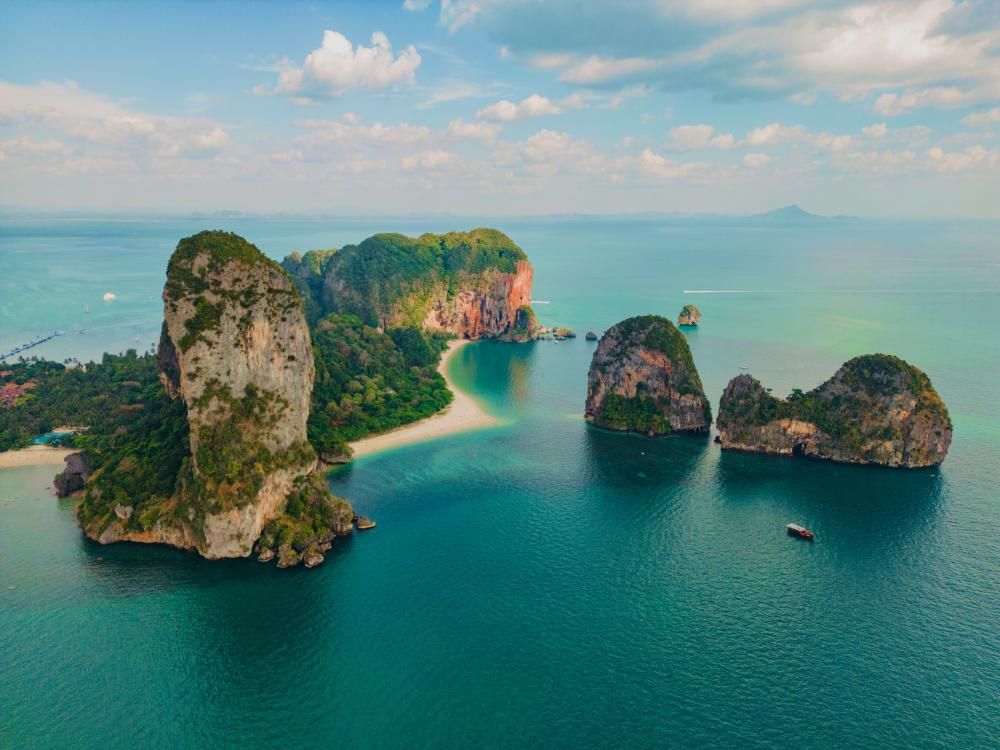
Insights
Healthcare data governance: components, benefits & strategy building
Discover the benefits of a data governance strategy for healthcare providers, tips on its successful implementation, and solutions to possible challenges.
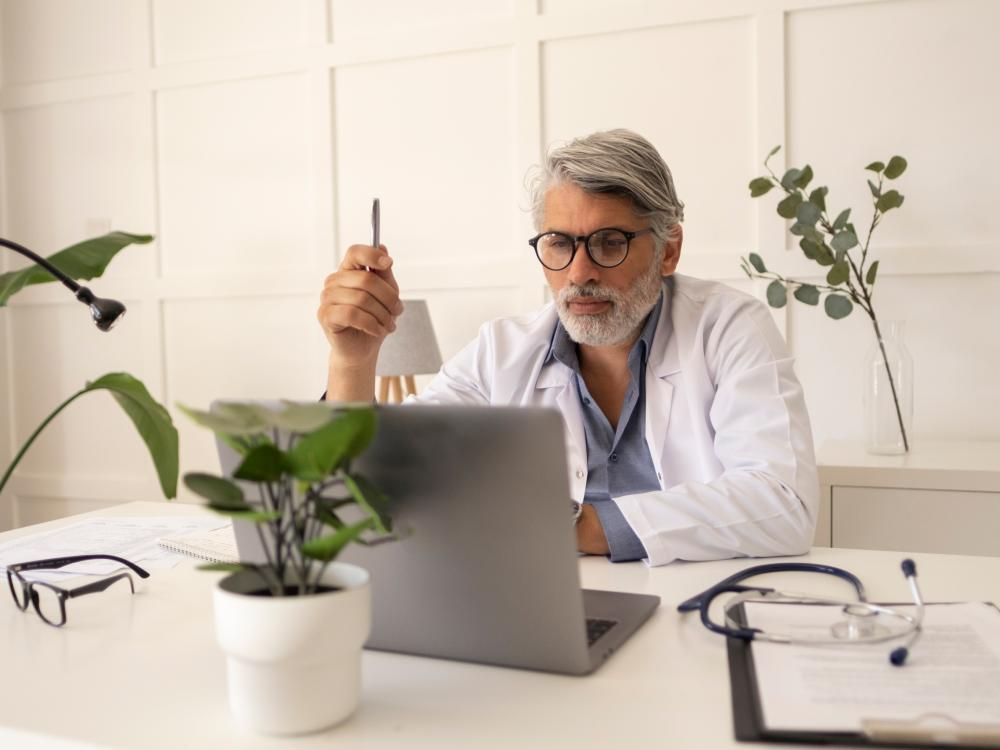
Insights
Virtual hospital: key features, examples & benefits of remote care
Learn about virtual hospitals, their architecture, integrations, and types. Explore their benefits for doctors and patients and implementation challenges.
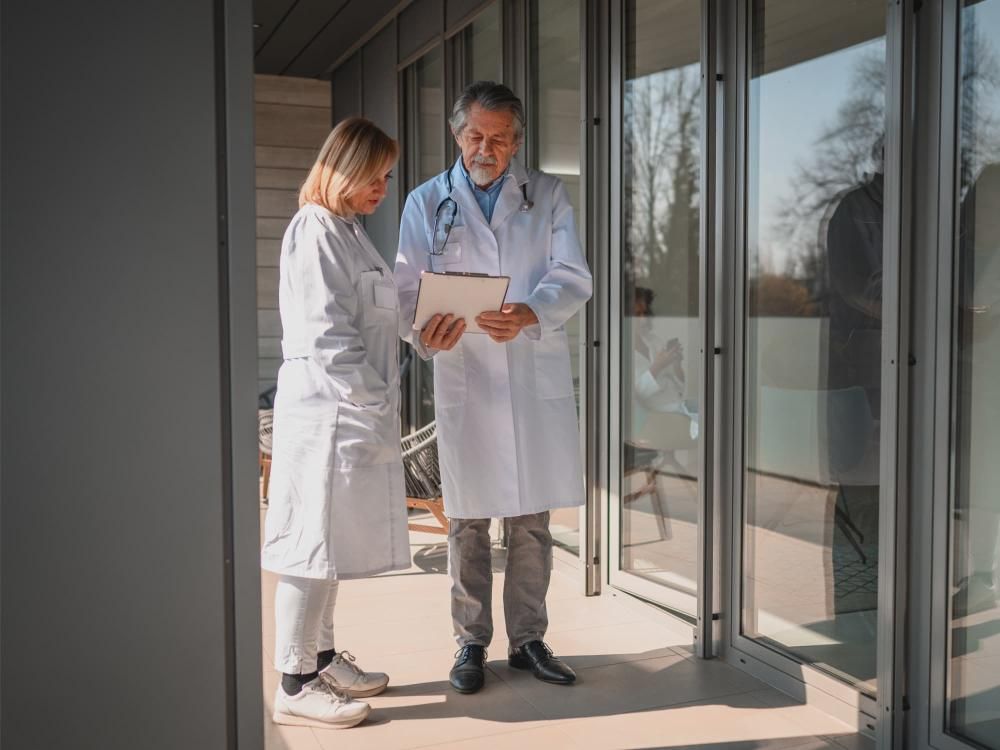
Insights
Hospital inventory management software: architecture, features, and platforms
Discover key features of the hospital inventory management software, explore the top solutions on the market, and learn their benefits for your organization.
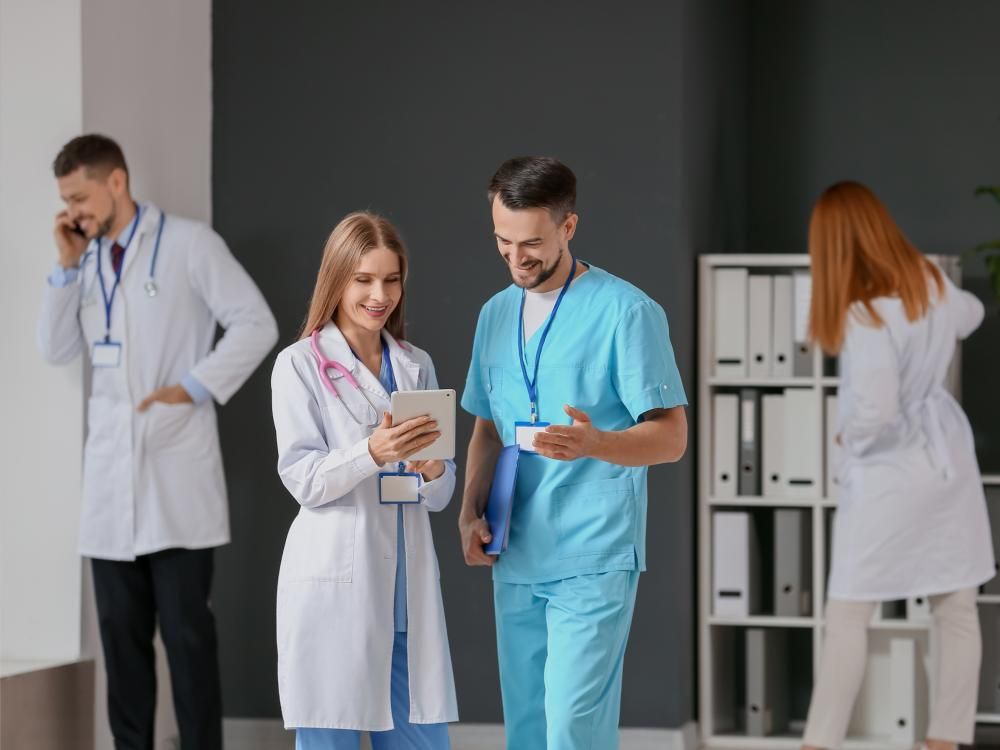
Insights
RPA in healthcare: use cases, benefits & challenges
Learn how robotic process automation streamlines processes in the healthcare industry and improves care delivery. Explore top RPA vendors, use cases, and costs.
More about machine learning services
Services
Industries