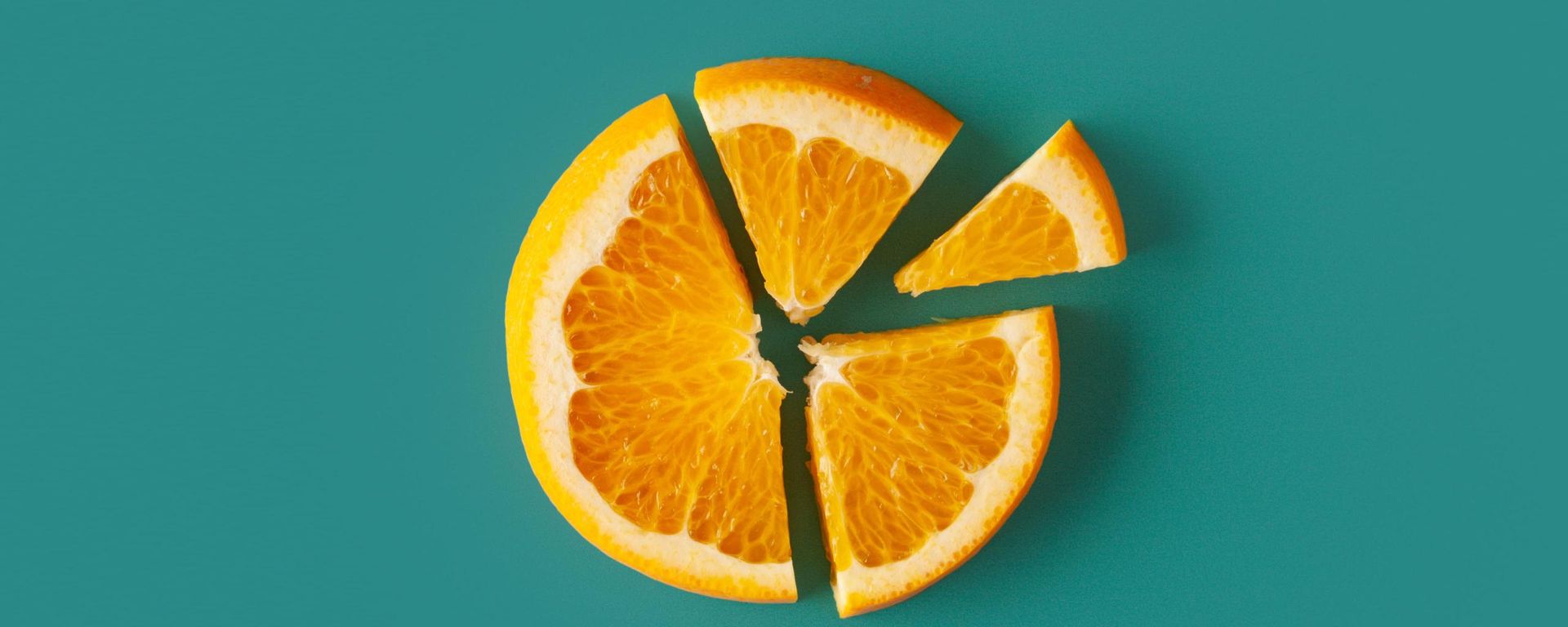
Business intelligence services and solutions
- Home
- Business intelligence services
Business intelligence (BI) services include designing, developing, and deploying processes and infrastructure for business information analysis and management. Itransition creates scalable BI solutions to help businesses transform raw data into immersive reports and interactive dashboards.
Table of contents
About Itransition
15+ years in business intelligence
25+ years in IT consulting and software engineering
Hands-on experience in creating custom, platform-based, and embedded BI solutions
Built-up expertise in delivering self-service BI solutions on top of Microsoft Power BI, Tableau, and Qlik
Strategic partnerships with Microsoft and AWS
ISO 27001 and ISO 9001-certified to ensure service quality and customer data safety
BI services we offer
Itransition offers a full scope of BI services to help companies across industries enhance decision-making and improve business process efficiency.
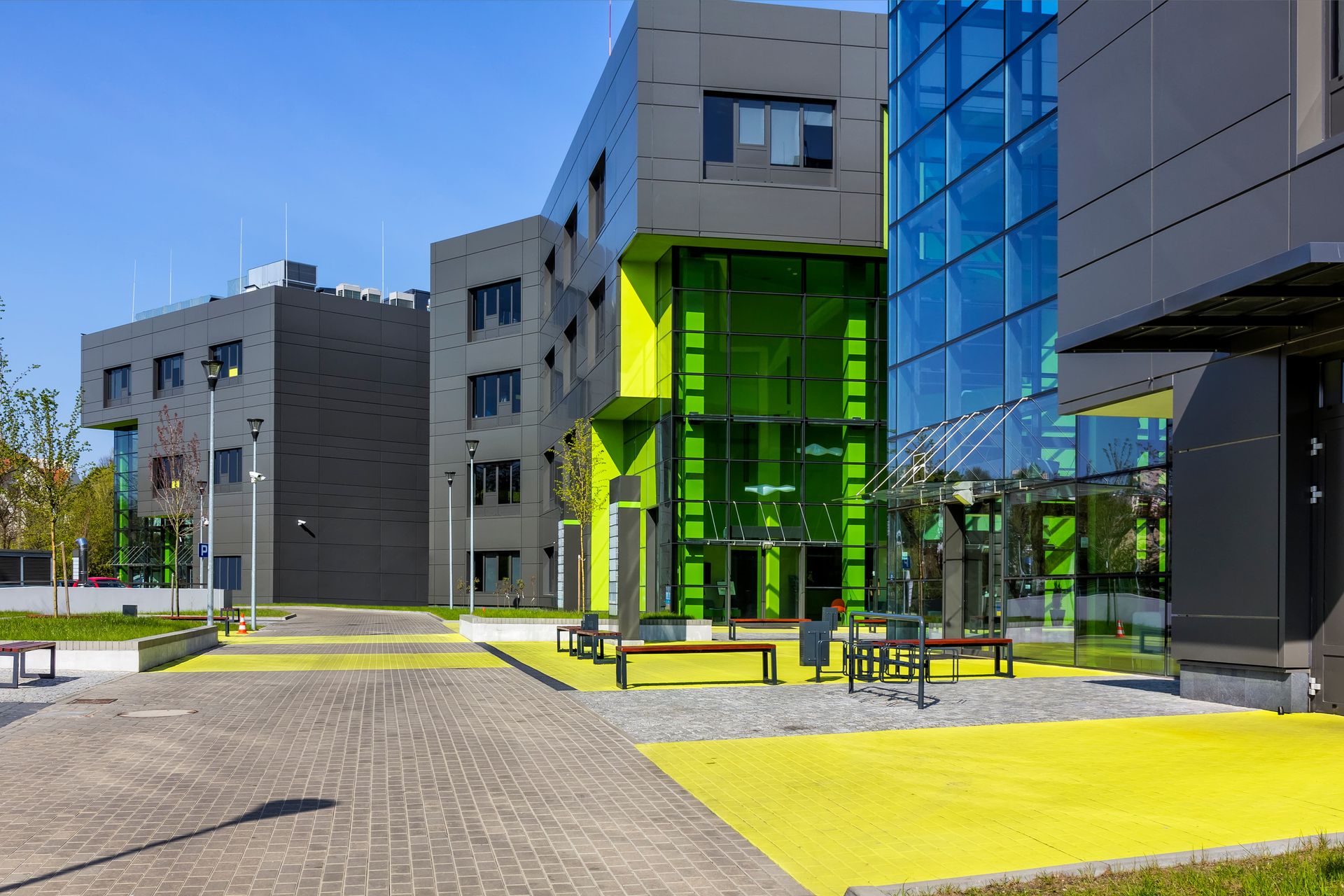
We guide our clients through the end–to-end BI implementation and provide on-demand advisory support, helping them choose a BI implementation approach, design BI solution architecture, select optimal technology, and set up comprehensive data management policies and practices.
We bring our hands-on BI expertise to deliver scalable and well-performing custom or platform-based BI solutions tailored to our clients’ business needs, operational challenges, and industry best practices.
Optimization
We audit and upgrade BI solutions with role-specific reports and dashboards, new data sources, complementary data storage, and advanced analytics capabilities to help our customers meet new BI needs and adapt to the evolving market.
Support
We offer post-development support services and basic and advanced support for BI solutions developed by other vendors to help our clients keep their BI solutions running smoothly and efficiently and avoid costly disruptions and downtimes.
Testing
We offer BI testing services as a part of our end-to-end BI implementation services or as a stand-alone service to validate the existing BI solution and ensure our customers make strategic and operational decisions based on timely, accurate, and high-quality data.
Looking for a trustworthy BI provider?
BI platforms we work with
Along with custom business intelligence solutions, we build business analytics solutions on top of leading BI platforms to help our clients accelerate time to value and reduce BI implementation costs.
Our BI implementation roadmap
1
Discovery & conceptualization
Elicitation of business needs and goals, expectations, risks, and concerns
Analytics and technology environment evaluation
Preliminary data source analysis
Analysis of data security and compliance practices
Definition of functional and non-functional requirements
2
Architecture design
Conceptual and logical data modeling
Creation of data integration and quality management policies and rules
ETL/ELT pipeline design
Design of a DWH, data marts, and OLAP cubes
Design of dashboards for different user roles with specific KPI sets
Creation of data security and governance standards and policies
3
Deployment environment & BI tools selection
Choosing the optimal deployment environment (cloud, on-premises, hybrid)
Defining optimal business intelligence tools for each component of the BI solution
4
Implementation project planning
BI solution development and testing scope planning
Project scheduling
Project resource allocation planning
Risk mitigation strategy development
BI implementation project estimation, including TCO and ROI
5
Components development & delivery to staging
Creation of custom data connectors
Implementation of ETL/ELT pipelines and data integration
Implementation of data quality management and data security rules
Development of a data warehouse and data marts
Reports and dashboards creation
6
QA & user training
Functional testing
Performance testing
Usability testing
Compatibility testing
Training sessions and support documentation
7
Deployment to business users
Running the BI solution in production
Monitoring BI solution performance and addressing arising issues
Adding new data sources
Configuring additional dashboards
BI infrastructure services we deliver
Data integration
We utilize various information consolidation methods, including ETL/ELT, change data capture, and data virtualization, to achieve a unified view of corporate data sets regardless of their type and source for efficient data analysis and reporting.
Data quality management
We set up and manage comprehensive data quality management practices and processes to make business decisions relying on accurate, timely, consistent, and complete data.
Data storage
We build and optimize DWH solutions across cloud and on-premises deployment environments and deliver other storage solutions, such as a data lake, a lakehouse, and an operational data store, to centralize business data and simplify the logic for its consumption.
We implement an analytics component that generates descriptive, diagnostic, predictive, and prescriptive insights to effectively solve business problems.
We equip the business analytics solution with big data analytics, data mining, data science, AI and ML capabilities to help companies identify patterns, detect anomalies and major outcome drivers, and make predictions and forecasts.
We create immersive, intuitive, and interactive reports and dashboards to make important metrics and actionable insights easy to access, interpret, and act on.
Data governance & security
We set up comprehensive data security practices and work out solid governance standards and policies to ensure BI workflows are well-governed and corporate data is processed, stored, analyzed, and accessed safely.
We let users access and analyze up-to-date and high-quality business information via mobile devices to increase operational efficiency, enhance customer experience, and improve collaboration between BI users.
We pack business intelligence solutions with self-service BI capabilities to enable non-technical end users to carry out data management and analytics activities independently. The features include intuitive user interfaces, NLP support, and ML-enabled data management and analysis.
Business intelligence solutions we deliver
By industry
- Financial KPIs tracking
- Financial planning, budgeting, and forecasting
- Cost and profitability management
- Cash flow management
- Performance monitoring and analysis
- Customer intelligence
- Financial risk management and regulatory compliance
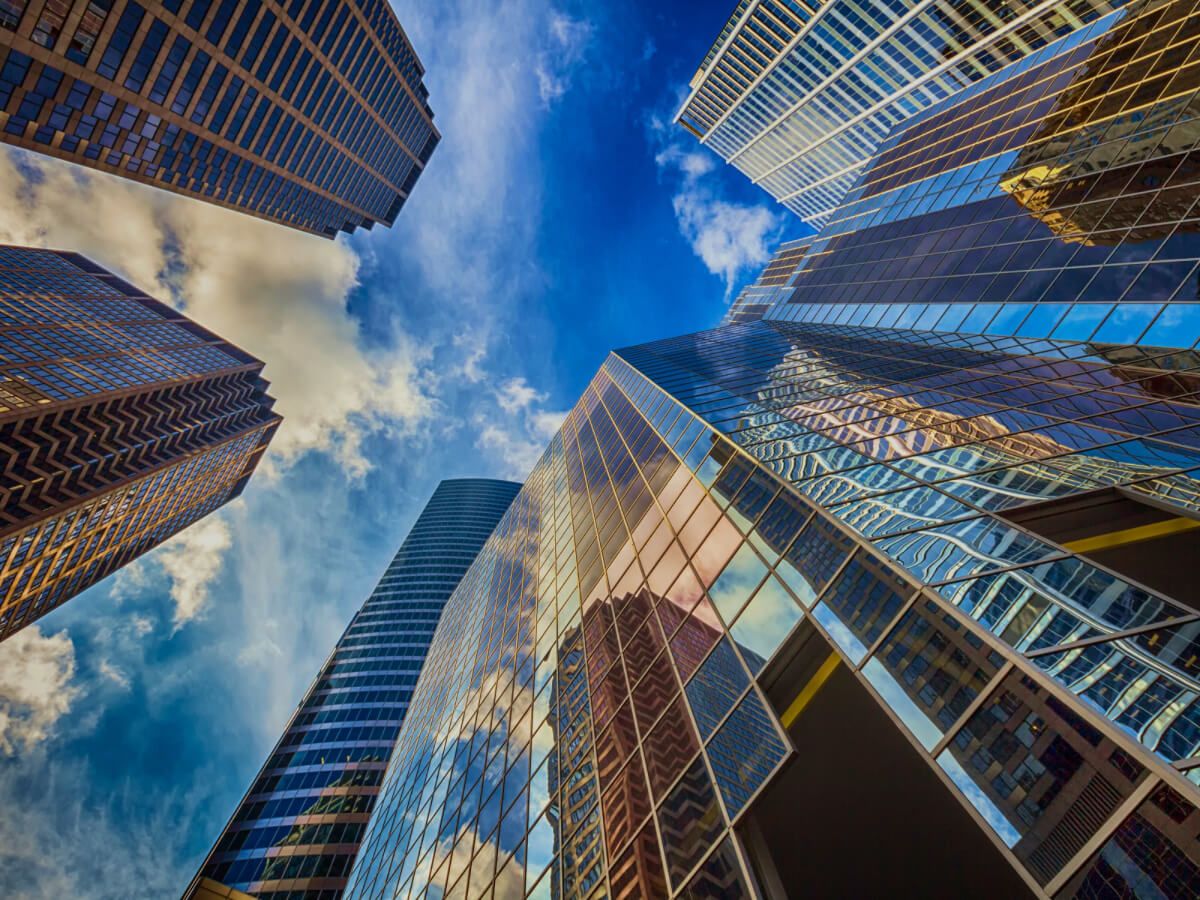
- Product assortment optimization
- Evaluation of promotional activities’ effectiveness
- Location intelligence
- Product price modeling and optimization
- Supply chain monitoring and balancing
- Sales tracking, analysis, and forecasting
- Sales channel performance analytics
- Personalized recommendations for up- and cross-selling
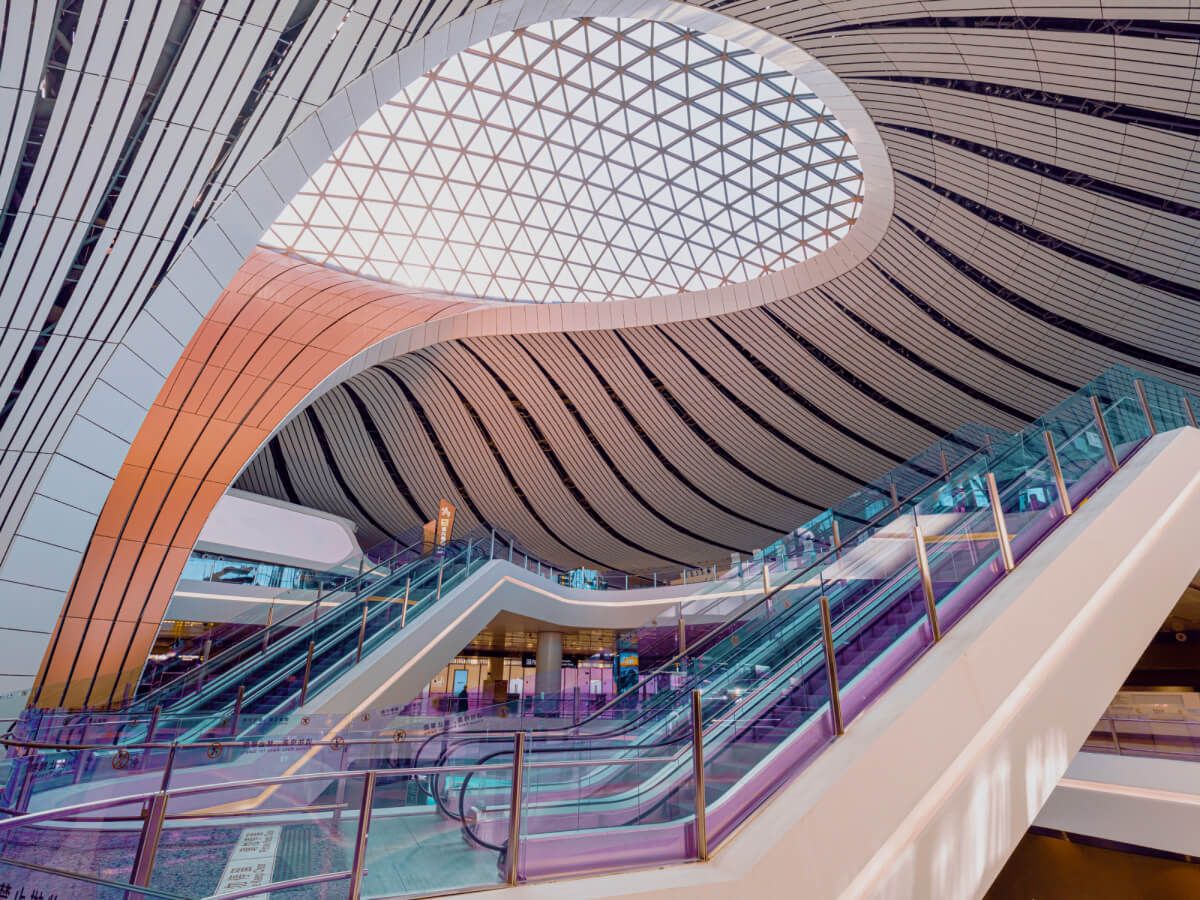
- Production analytics
- Bottlenecks and shift performance analysis
- Inventory control and procurement planning
- Supplier performance monitoring and evaluation
- Financial management and forecasting
- Maintenance intelligence
- Demand forecasting
- Price optimization
- Customer service and warranty management
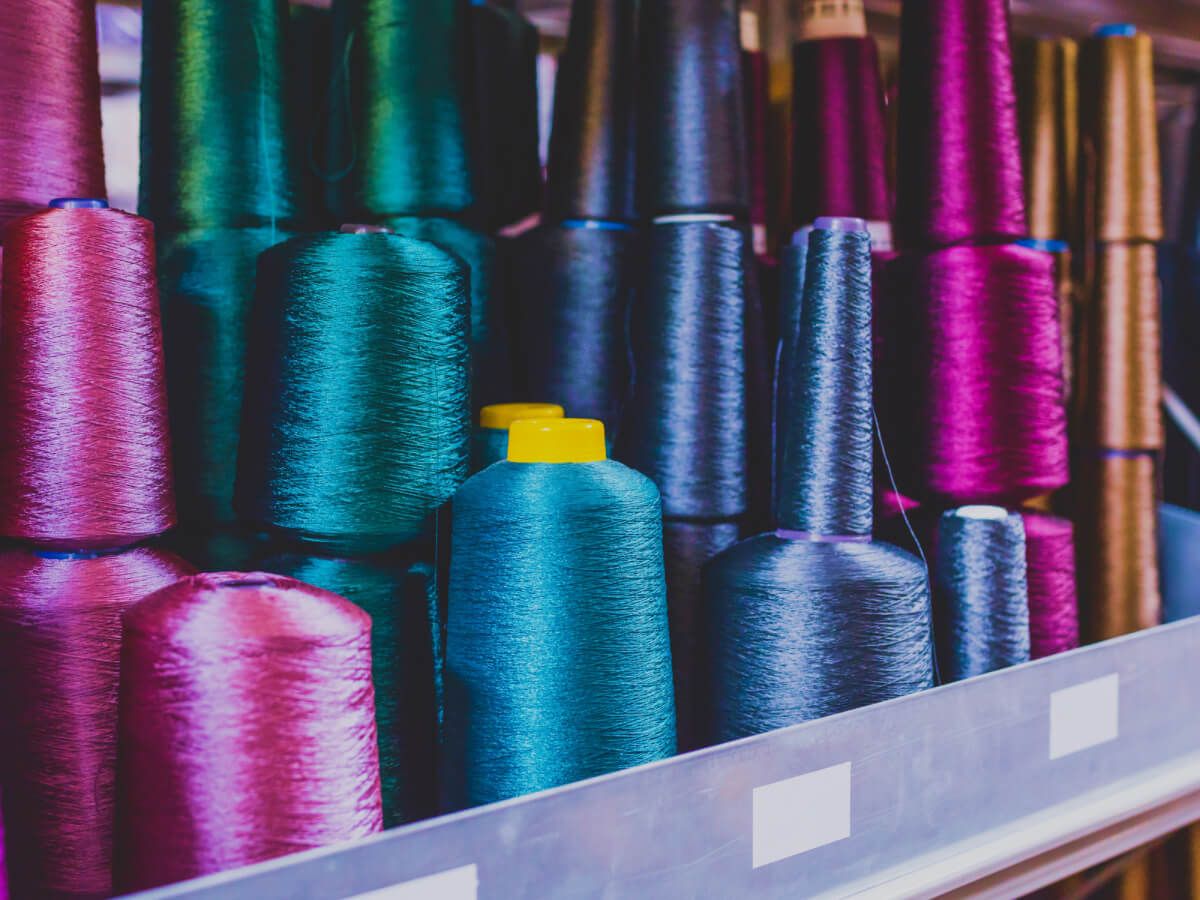
- Caregivers performance monitoring and evaluation
- Workload prediction and medical staff scheduling
- Patient satisfaction analysis
- Personalization and patient needs prediction
- Patient risks prediction
- Outbreaks prediction
- Healthcare insurance fraud detection
- Patient demographics analysis
- Patient care performance analysis
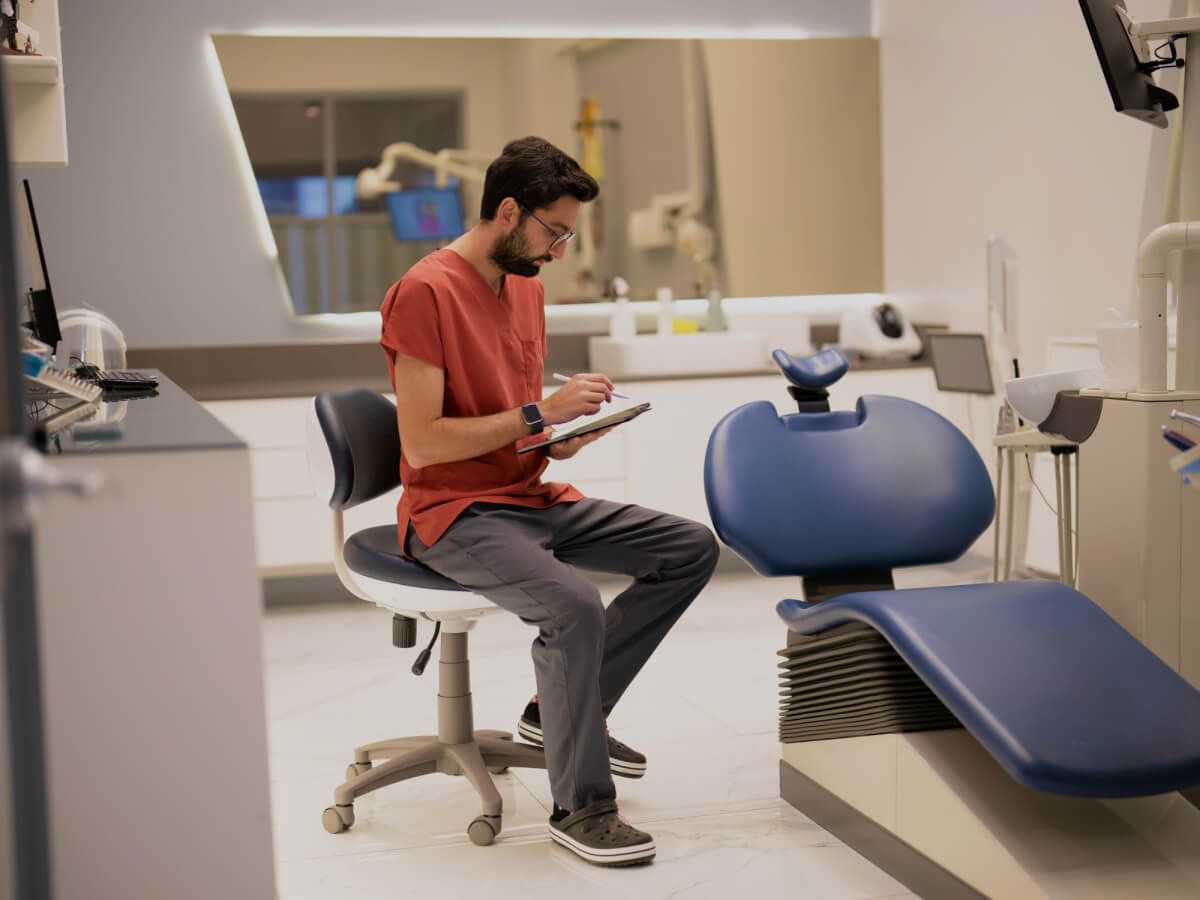
Education & eLearning
- Students’ performance analysis and educational planning
- Student intelligence, including course attendance, interests, GPA, teachers’ feedback, etc.
- Students’ retention analysis
- Teacher intelligence, including academic load, performance, and students’ feedback
- Expenses and revenue analytics
- Workforce planning and scheduling
- Room occupancy and inventory planning
- Marketing campaigns effectiveness evaluation
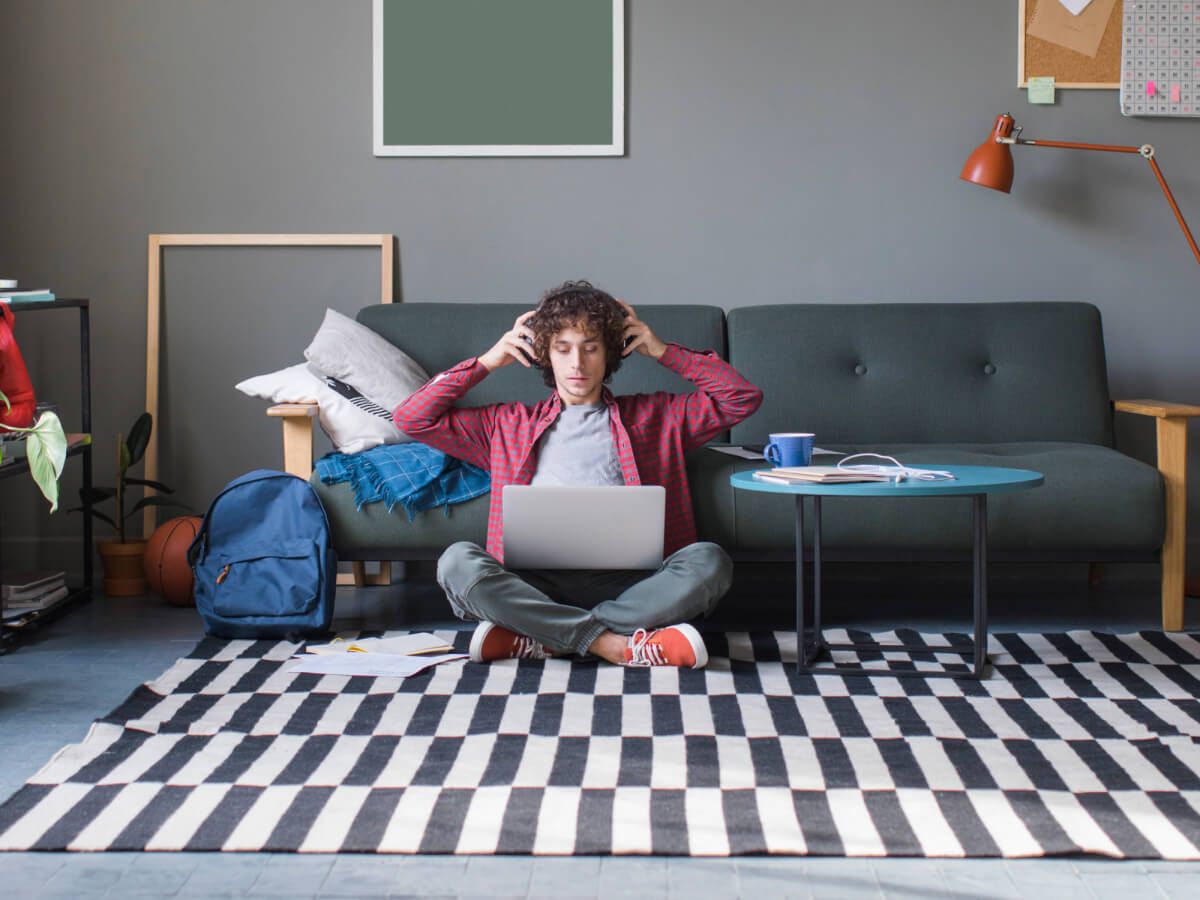
Telecommunications, media & entertainment
- Analysis of customer needs, preferences, and demographics
- Customer profitability analysis
- Calculation of customer lifetime value and average revenue per user
- Customer churn prediction
- Customer attrition analysis
- Personalized customer service and plans
- Customer usage patterns analysis
- Prediction of peak hours and network issues
- Marketing campaign analysis
- Analysis of product performance, usage, and revenue
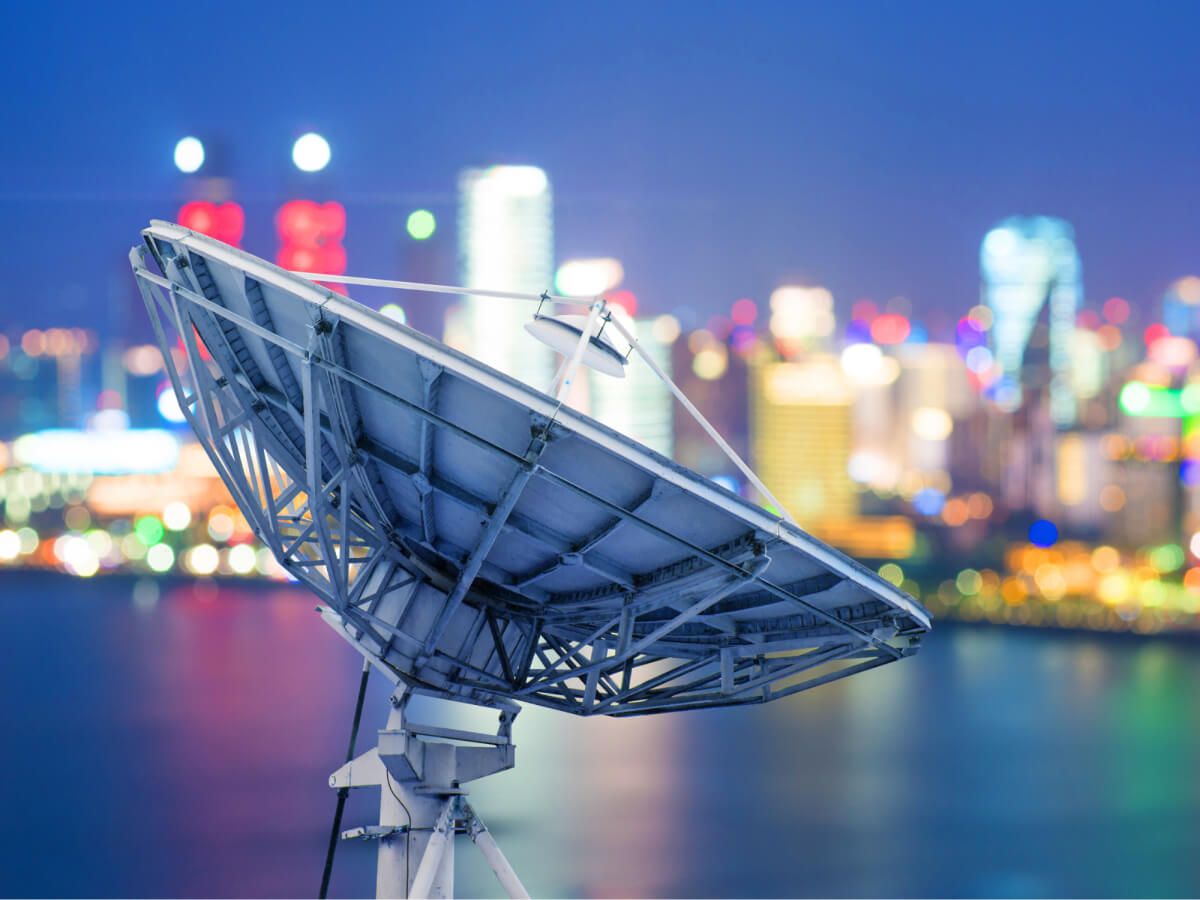
Real estate
- Property value analysis
- Analysis of variables affecting a property’s long-term profitabilityÂ
- Property sales analysis
- Marketing campaign monitoring and evaluation
- Property management analysis
- Market rent analysis
- Monitoring and analysis of brokerage agents’ activities and performanceÂ
- Risk forecasting
- Property price trend analysis
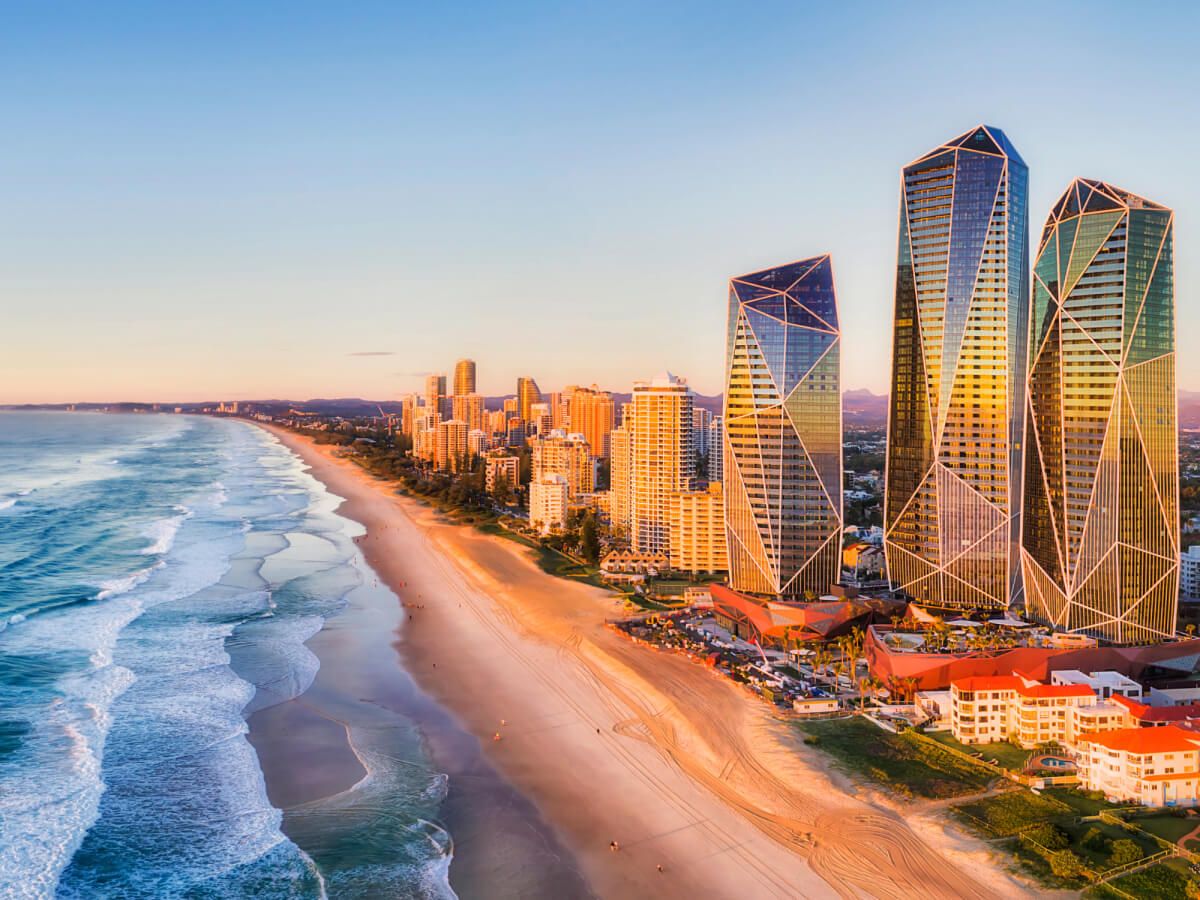
Logistics & transportation
- Fleet operations monitoring
- Distribution schedules and route planning
- Carrier and warehousing service providers’ performance analysis
- Vehicle condition analysis
- Transport volume analysis
- Predictive analytics for vehicle maintenance
- Driver behavior analysis
- Fuel consumption monitoring and analysis
- Delivery delays and failures analysis
- Defining optimal delivery time, speed, and transportation mode
- Storage capacity planning
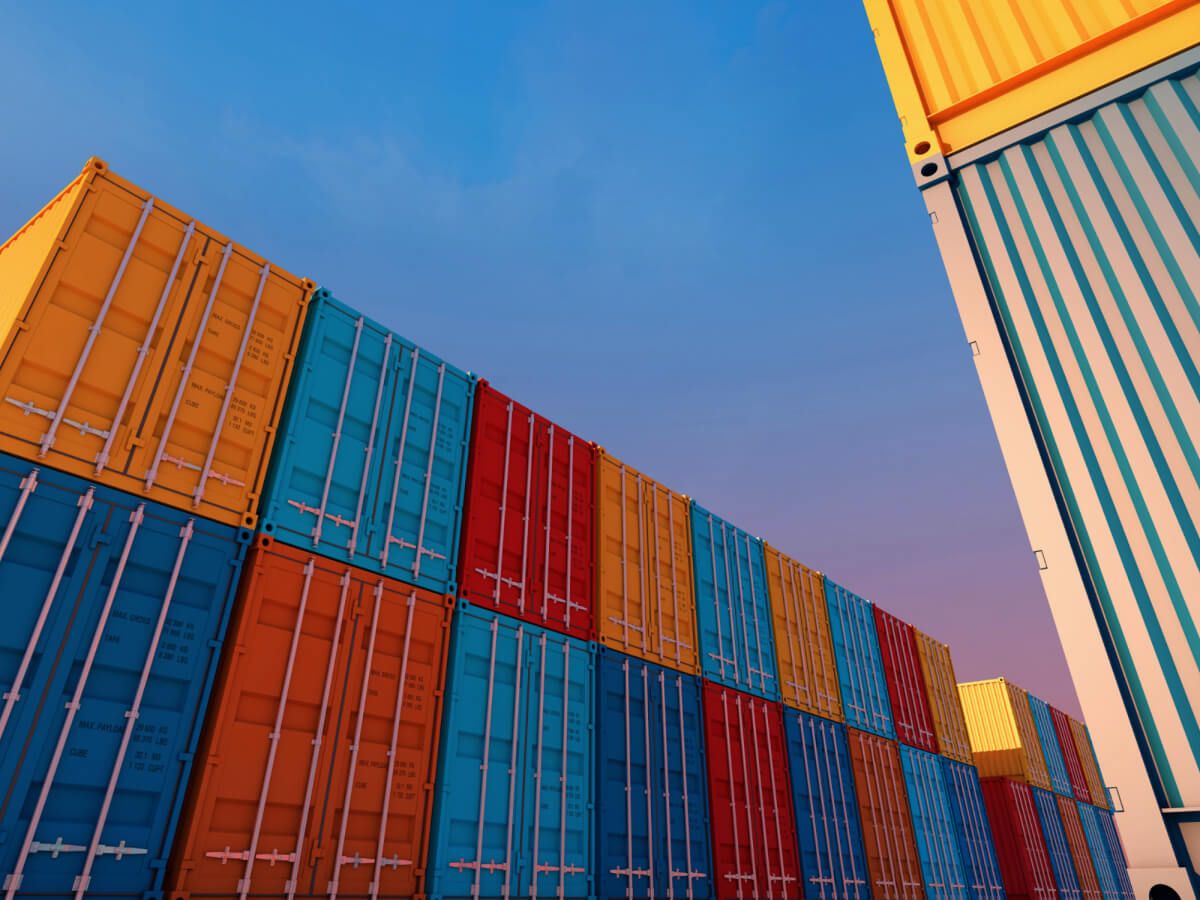
Agriculture
- Plant and livestock wellness monitoring and evaluation
- Soil and yield analysis
- Waste identification and analysis
- Recalls tracking and analysis
- Crop performance analysis
- Demand forecasting
- Production planning and forecasting
- Financial performance monitoring
- Farm machinery and equipment performance analysis
- Sustainability initiatives impact and performance analysis
- Recalled items tracking and analysis
- Feed and water consumption monitoring and analysis
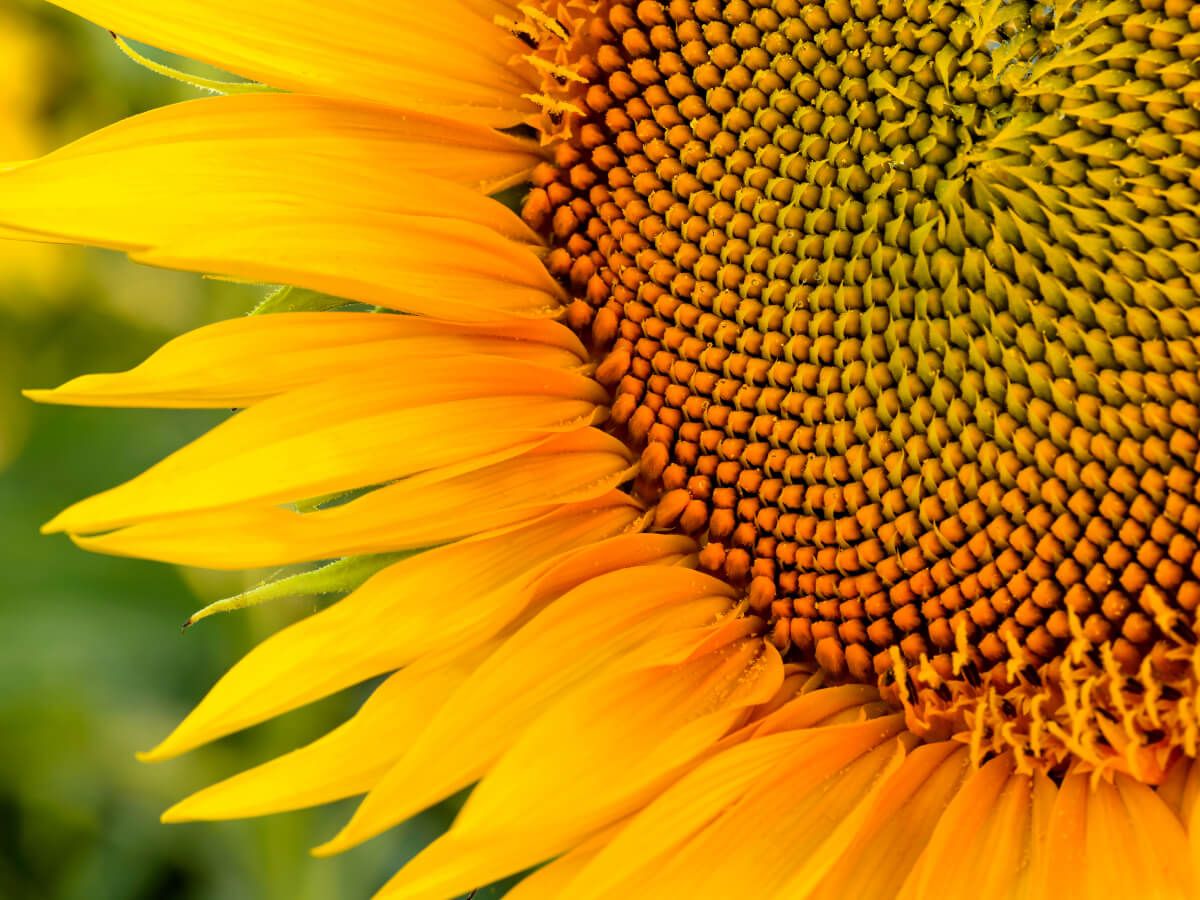
Insurance
- Insurance KPIs tracking (quota and quote rates, percentage pending, retention rate, average cost per claim, referrals, open claims, etc.)
- Customer intelligence
- Market trends monitoring and competitor benchmarking for recognizing growth opportunities
- Individual product and agency performance assessment
- Underwriting and sales processes bottleneck detection
- Identification of fraudulent claims and risk management
- Financial reporting and regulatory compliance
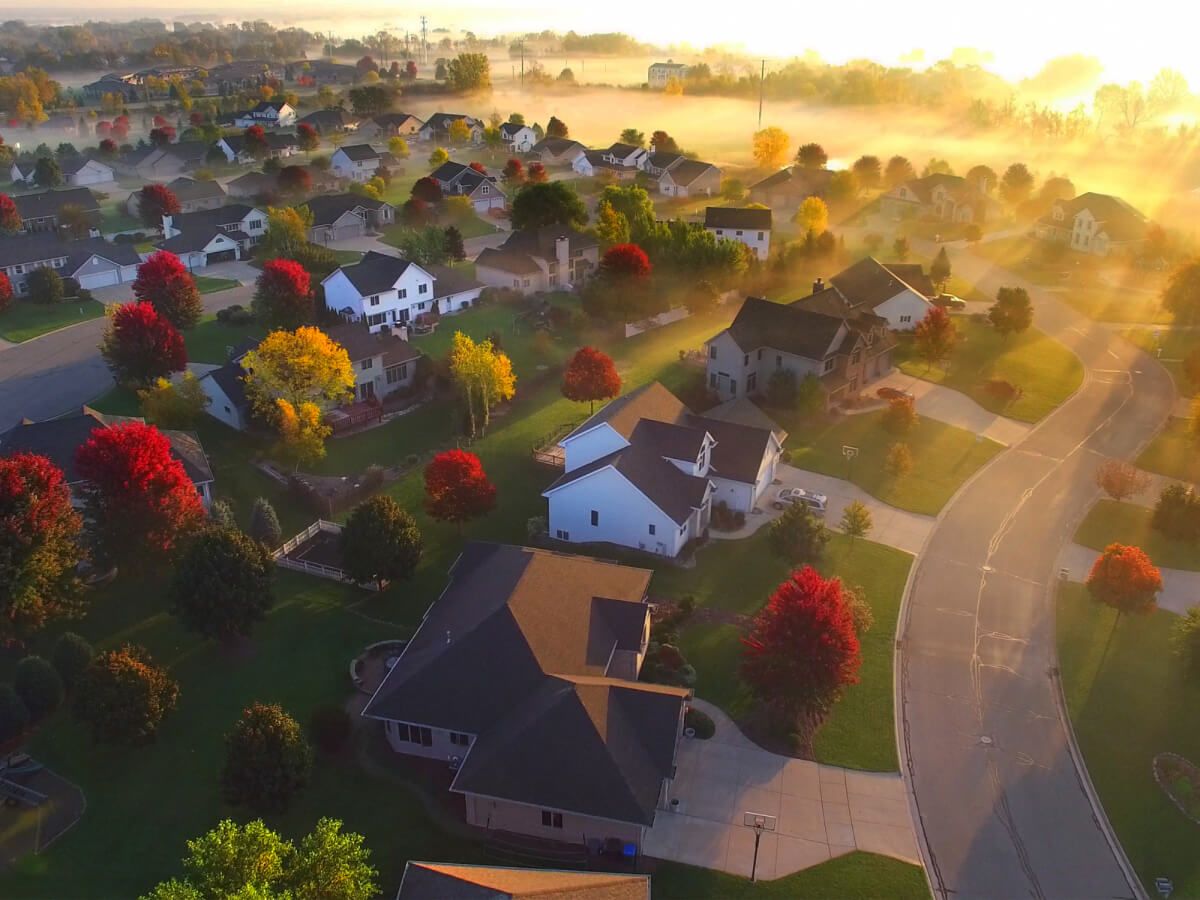
Professional services
- Corporate KPIs tracking and reporting (top customers by revenue, billable vs non-billable hours on projects, top projects budget-wise, etc.)
- Projects status, pipeline, and costs analysis
- Staff performance, utilization, and turnover analysis
- Staff churn monitoring and prediction
- Sales planning and forecasting
- Resource cost analysis
- Sales trends and demand forecasting
- Financial planning and project budgeting
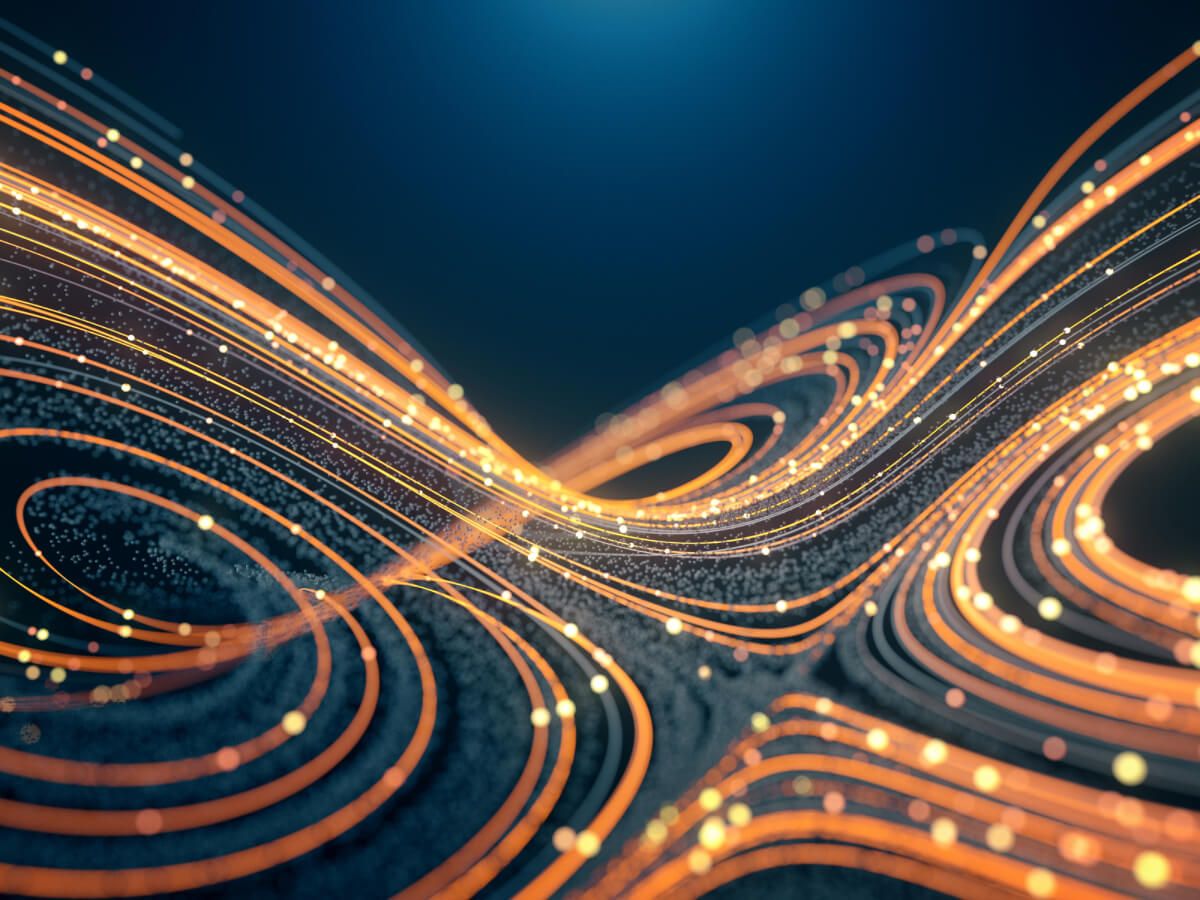
Construction
- Project data visibility (project type, client’s name, project status, start and end date, etc.)
- Project progress tracking (completion percentage, schedule performance index, cost performance index, cost breakdown)
- Cost and performance analysis
- Budget development optimization
- Contractor and subcontractor performance analysis
- Labor costs analysis
- Tracking and analyzing resource utilization (labor, materials, equipment, and subcontractors)
- Identification, analysis, and prediction of construction project risks (delays, cost overruns, supply chain disruptions)
- Project planning relying on industry trends (economic conditions, material costs, building patterns)
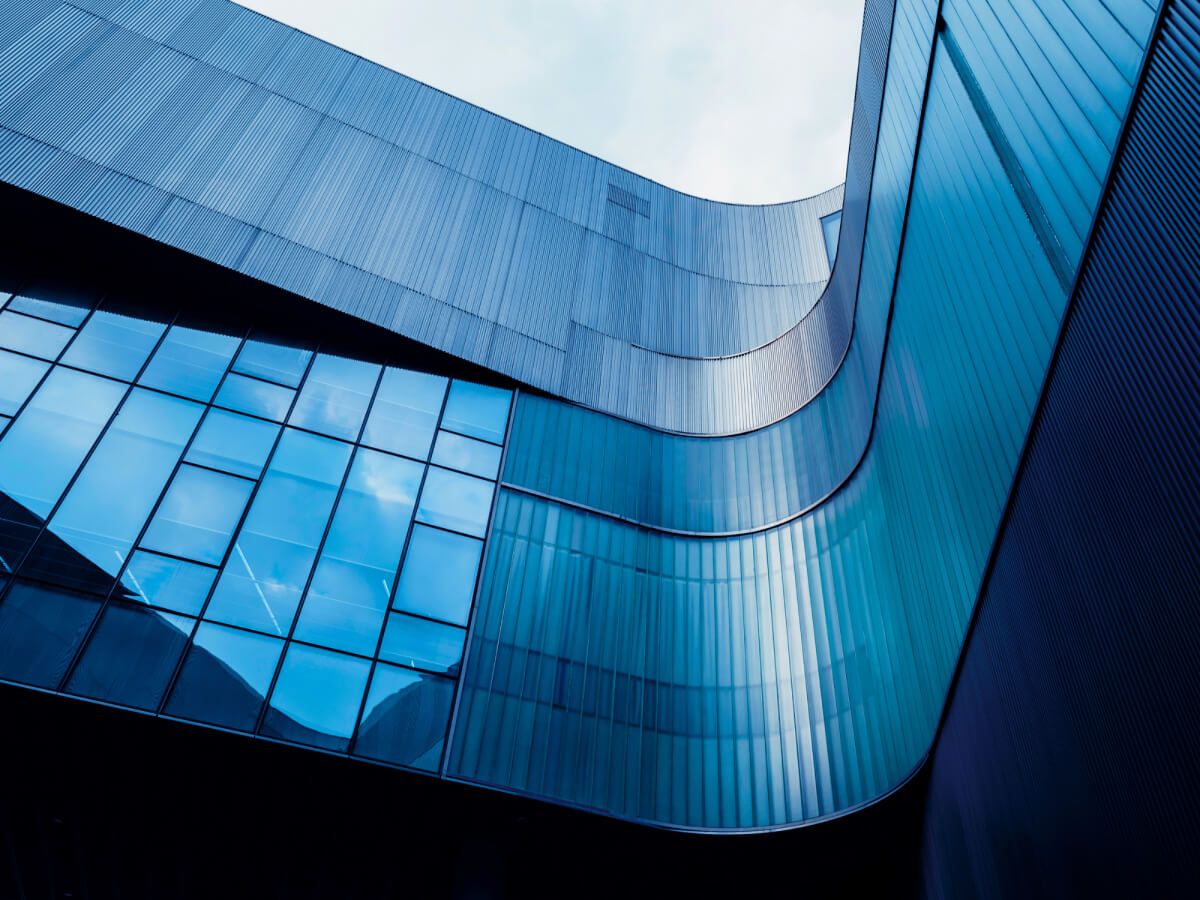
Automotive
- Production analytics
- Demand forecasting and production planning
- CTC cycle estimation and planning
- Supply chain analytics
- Quality control (analysis of product defects, warranty claims, and customer complaints)
- Devising targeted marketing campaigns and sales strategies
- Cost analytics (production costs, raw materials, labor, and overhead costs)
- Innovation and new product development
- Supplier performance assessment and analysis
- Employees performance analysis
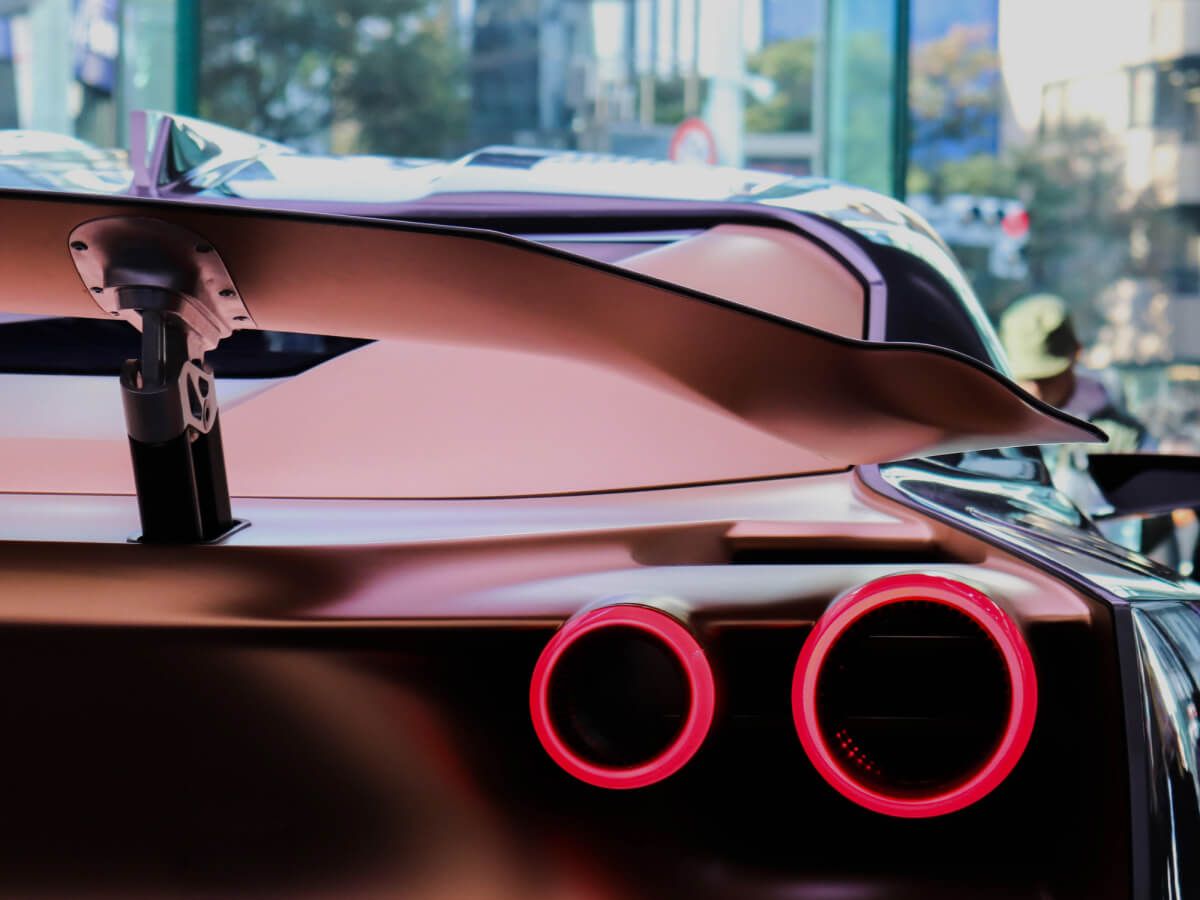
Energy
- KPIs tracking (average production rate, operating cash flow, production costs, energy production distribution, performance ratio, etc.)
- Equipment performance monitoring and analytics
- Production cost analysis
- Supply chain analytics
- Demand forecasting
- Sales analytics
- Supplier analytics
- Workforce analytics
- Health, safety, and environment analytics
- Risk analytics
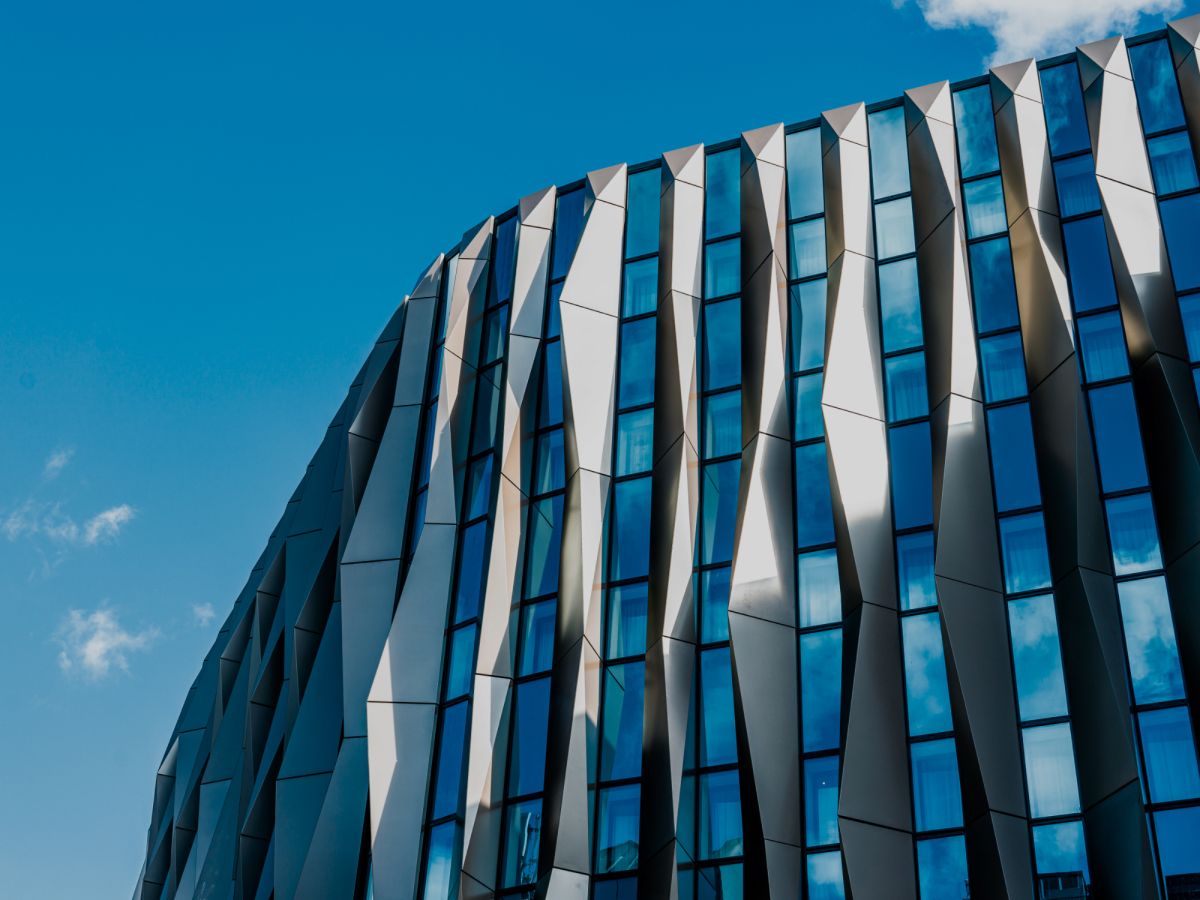
By functional area
Success stories
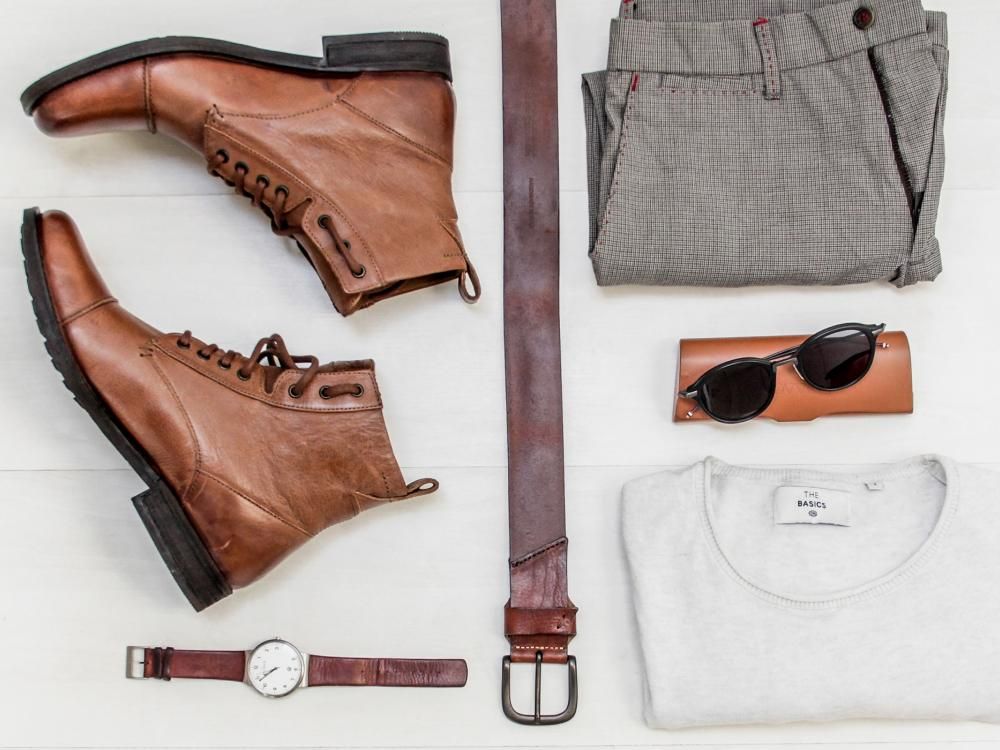
50% cut
infrastructure costs
BI platform for a fashion retailer
Itransition developed retail-specific BI software for data collection and analysis that helped the customer better understand online user behavior, apply AI-enabled personalization, and increase the visitors-to-buyers conversion rate by 8%.
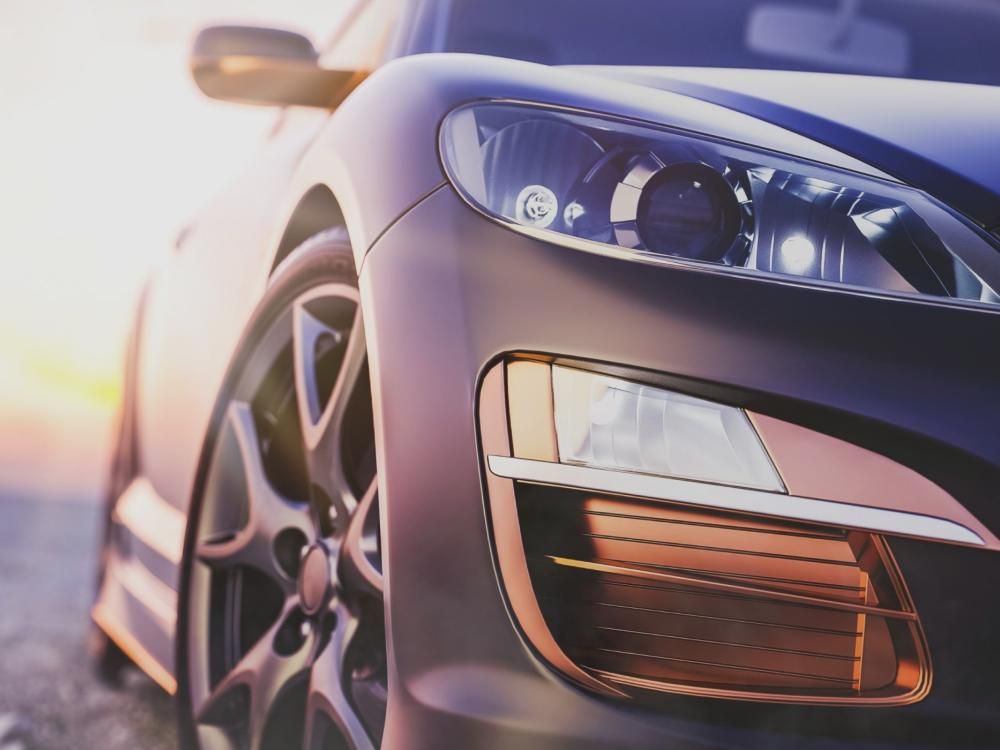
70% faster
time-to-market
Cloud BI for a vehicle manufacturer
Itransition transformed the customer’s legacy BI system to help them achieve a 15-20x higher system throughput, 100% predictability and transparency of work processes, and 50% higher productivity.
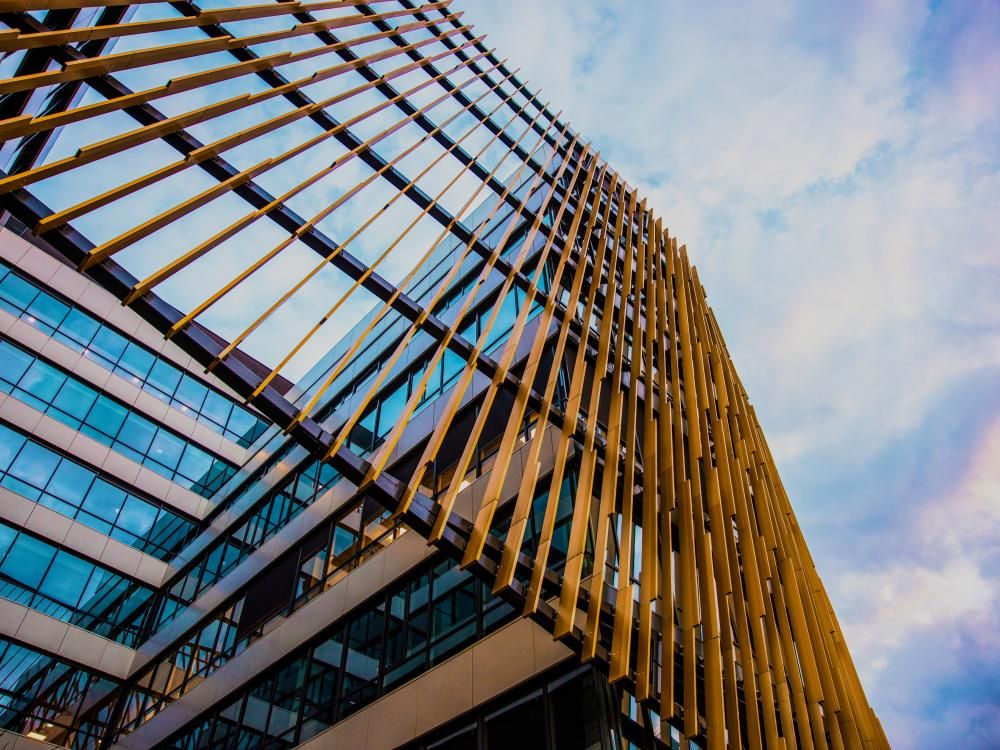
2x faster
market project delivery
Power BI implementation and training
Itransition developed a Power BI-based analytics solution and conducted employee training for an analytics and research consulting company to automate and improve their data processing and presentation.
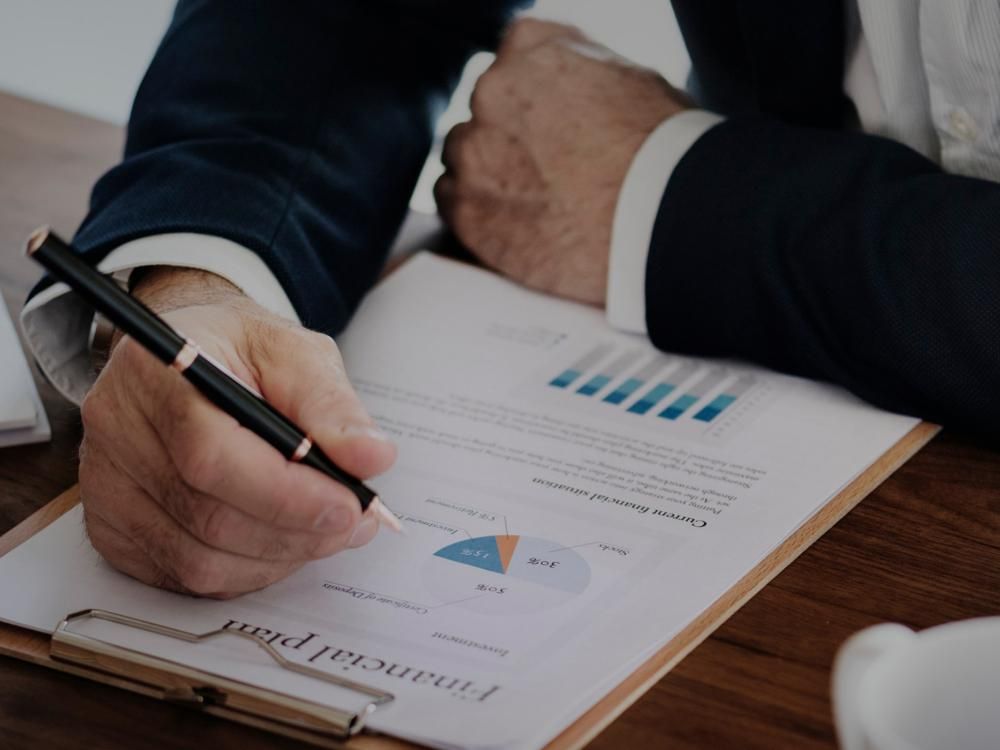
Detailed
BI implementation strategy and roadmap
BI consulting and engineering for a commercial bank
Itransition developed a detailed BI implementation strategy and prepared improvement recommendations for the customer’s data architecture as well as performed data modeling, redeveloped ETL operations, and conducted a detailed comparison of BI platforms.
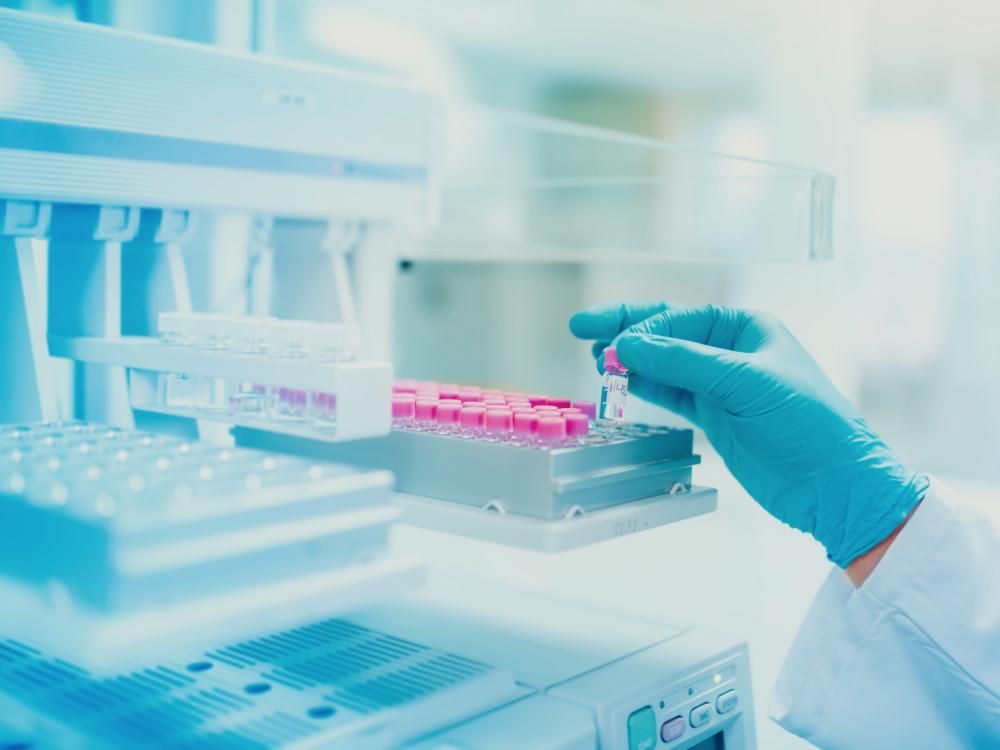
10x faster
data processing
Pharmaceutical BI for a US multinational
Itransition redeveloped the customer’s BI platform and migrated it to the cloud to help the customer streamline data processing, introduce market-forecasting algorithms, and get valuable insights into employee performance.
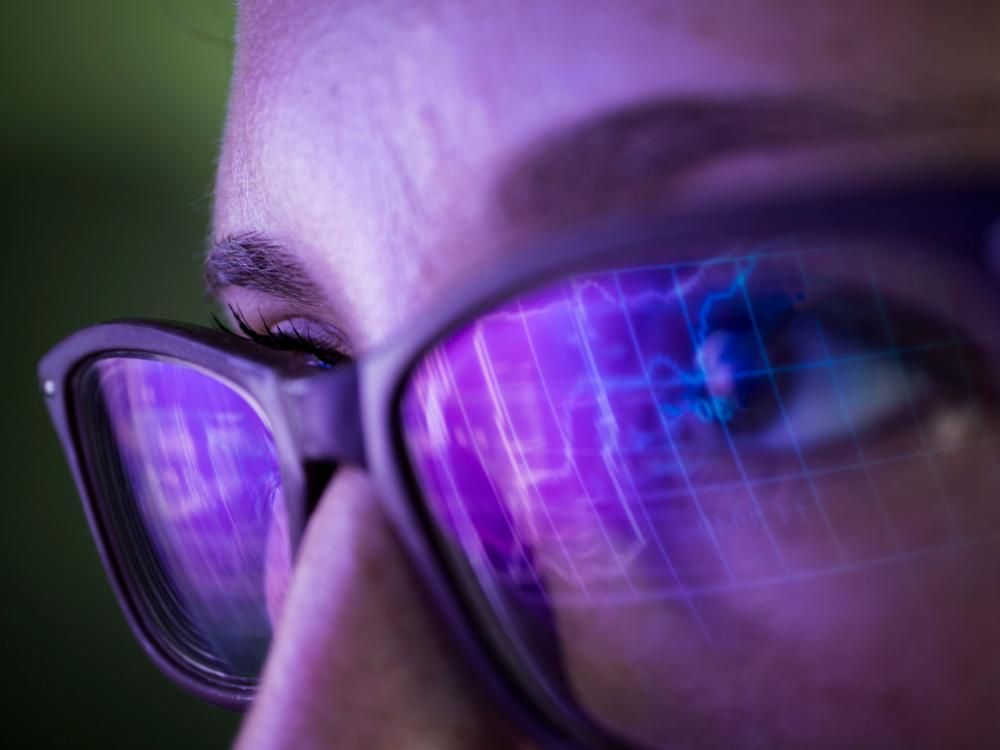
30% reduced
report opening time
BI solution enhancement for InsightSoftware.com
Itransition improved the solution’s stability and report-building performance, implemented history tracking features, and performed integration with SAP to help the customer decrease report memory usage and reduce report opening time by 30%.
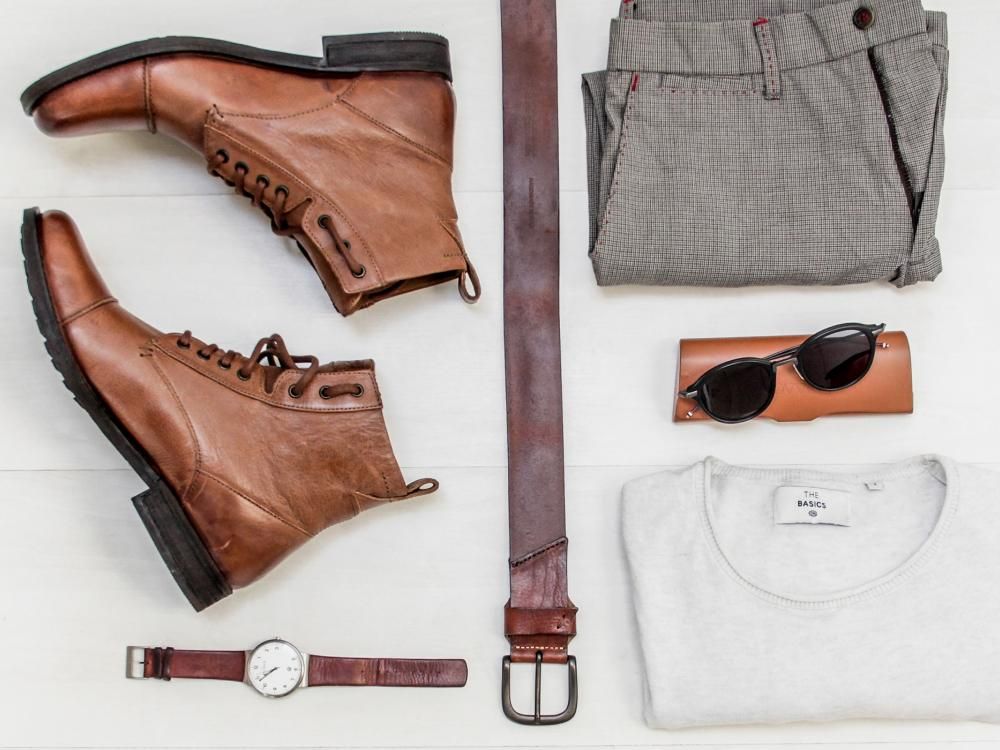
50% cut
infrastructure costs
BI platform for a fashion retailer
Itransition developed retail-specific BI software for data collection and analysis that helped the customer better understand online user behavior, apply AI-enabled personalization, and increase the visitors-to-buyers conversion rate by 8%.
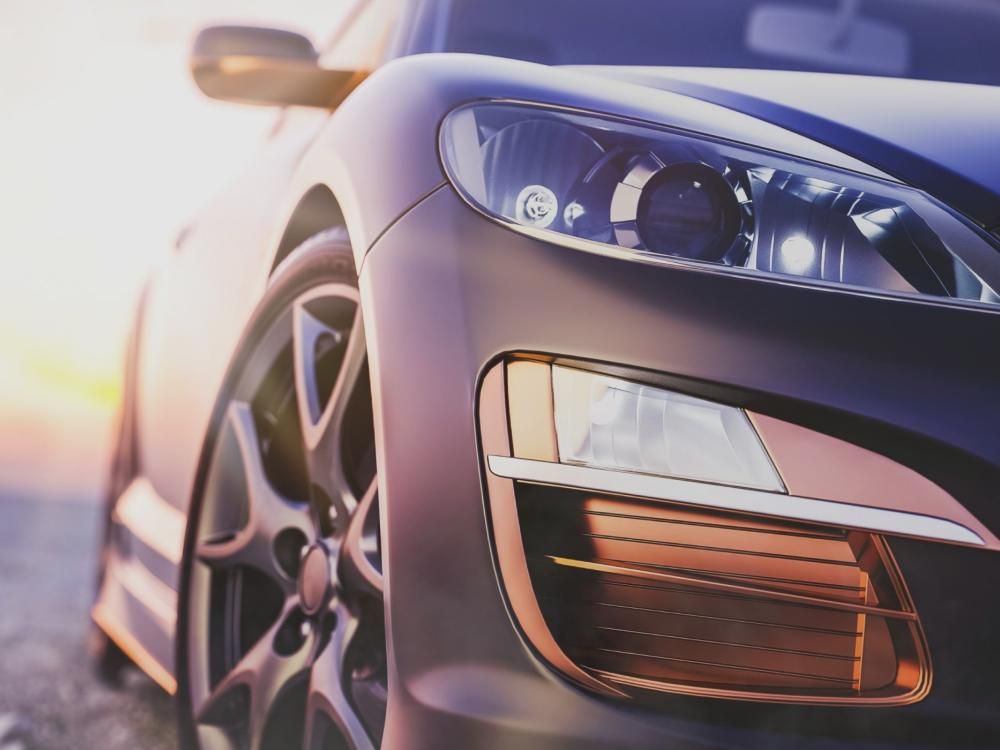
70% faster
time-to-market
Cloud BI for a vehicle manufacturer
Itransition transformed the customer’s legacy BI system to help them achieve a 15-20x higher system throughput, 100% predictability and transparency of work processes, and 50% higher productivity.
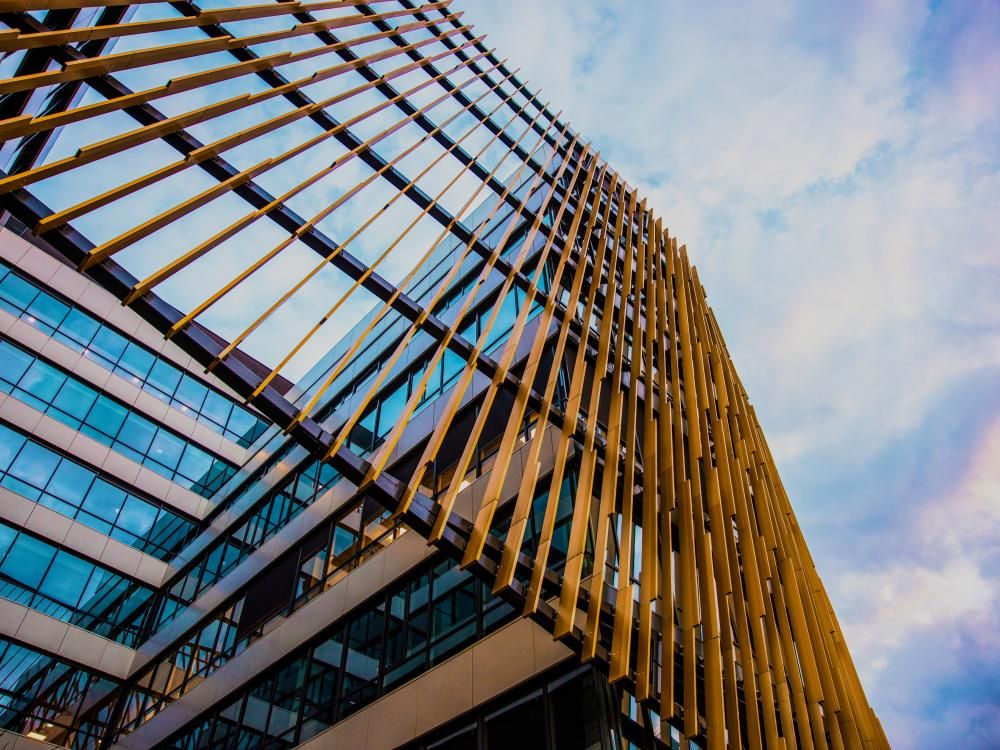
2x faster
market project delivery
Power BI implementation and training
Itransition developed a Power BI-based analytics solution and conducted employee training for an analytics and research consulting company to automate and improve their data processing and presentation.
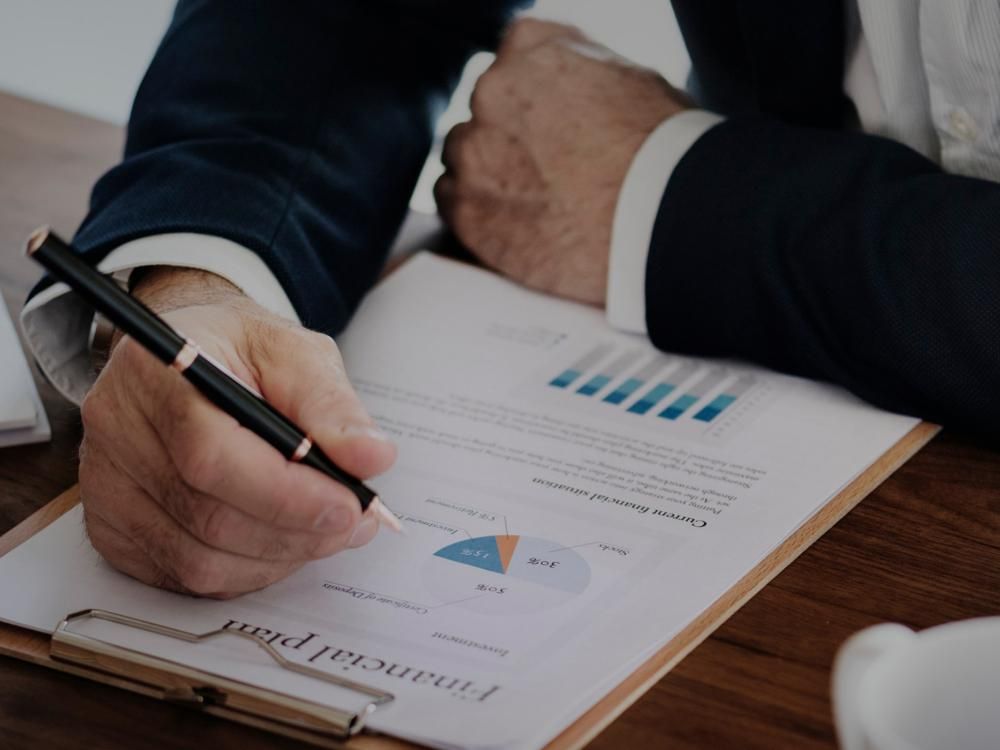
Detailed
BI implementation strategy and roadmap
BI consulting and engineering for a commercial bank
Itransition developed a detailed BI implementation strategy and prepared improvement recommendations for the customer’s data architecture as well as performed data modeling, redeveloped ETL operations, and conducted a detailed comparison of BI platforms.
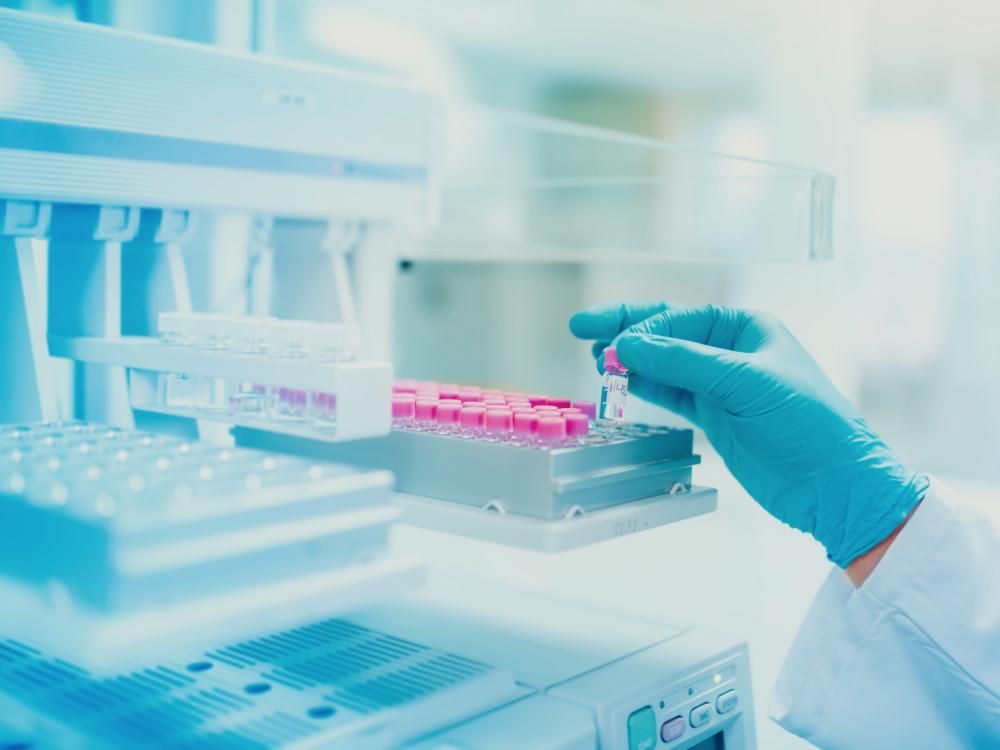
10x faster
data processing
Pharmaceutical BI for a US multinational
Itransition redeveloped the customer’s BI platform and migrated it to the cloud to help the customer streamline data processing, introduce market-forecasting algorithms, and get valuable insights into employee performance.
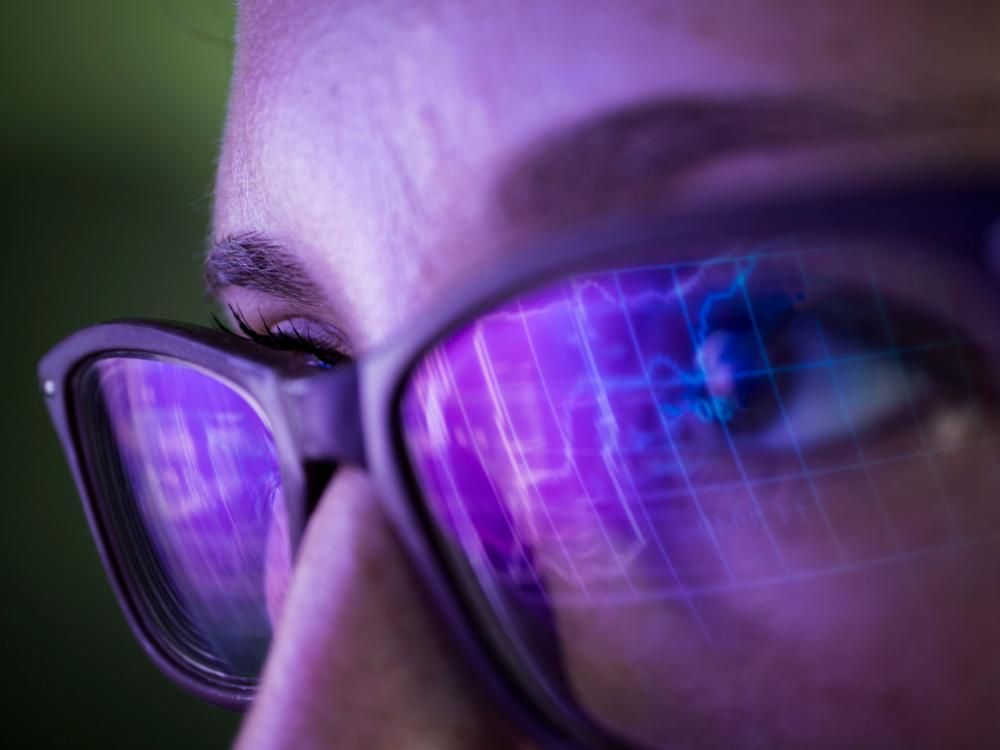
30% reduced
report opening time
BI solution enhancement for InsightSoftware.com
Itransition improved the solution’s stability and report-building performance, implemented history tracking features, and performed integration with SAP to help the customer decrease report memory usage and reduce report opening time by 30%.
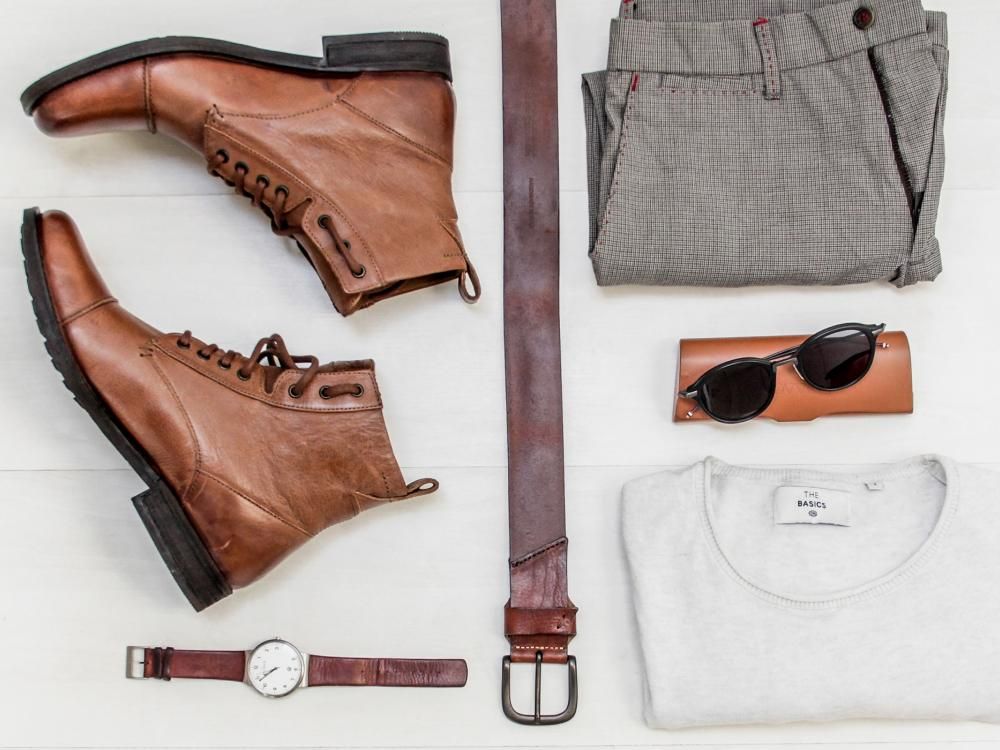
50% cut
infrastructure costs
BI platform for a fashion retailer
Itransition developed retail-specific BI software for data collection and analysis that helped the customer better understand online user behavior, apply AI-enabled personalization, and increase the visitors-to-buyers conversion rate by 8%.
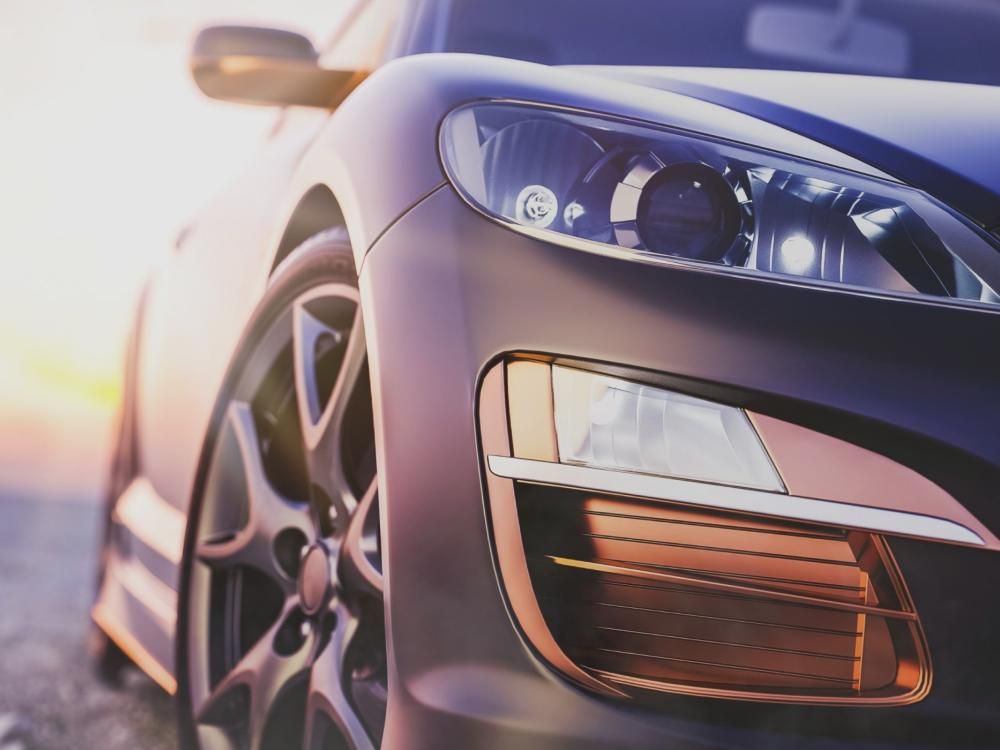
70% faster
time-to-market
Cloud BI for a vehicle manufacturer
Itransition transformed the customer’s legacy BI system to help them achieve a 15-20x higher system throughput, 100% predictability and transparency of work processes, and 50% higher productivity.
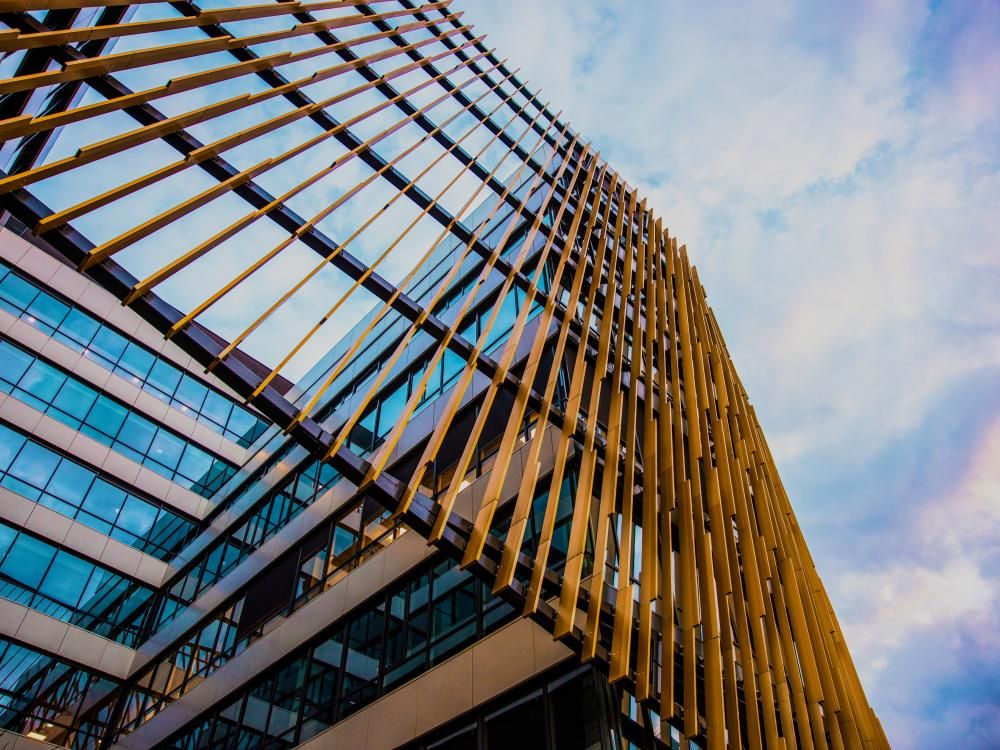
2x faster
market project delivery
Power BI implementation and training
Itransition developed a Power BI-based analytics solution and conducted employee training for an analytics and research consulting company to automate and improve their data processing and presentation.
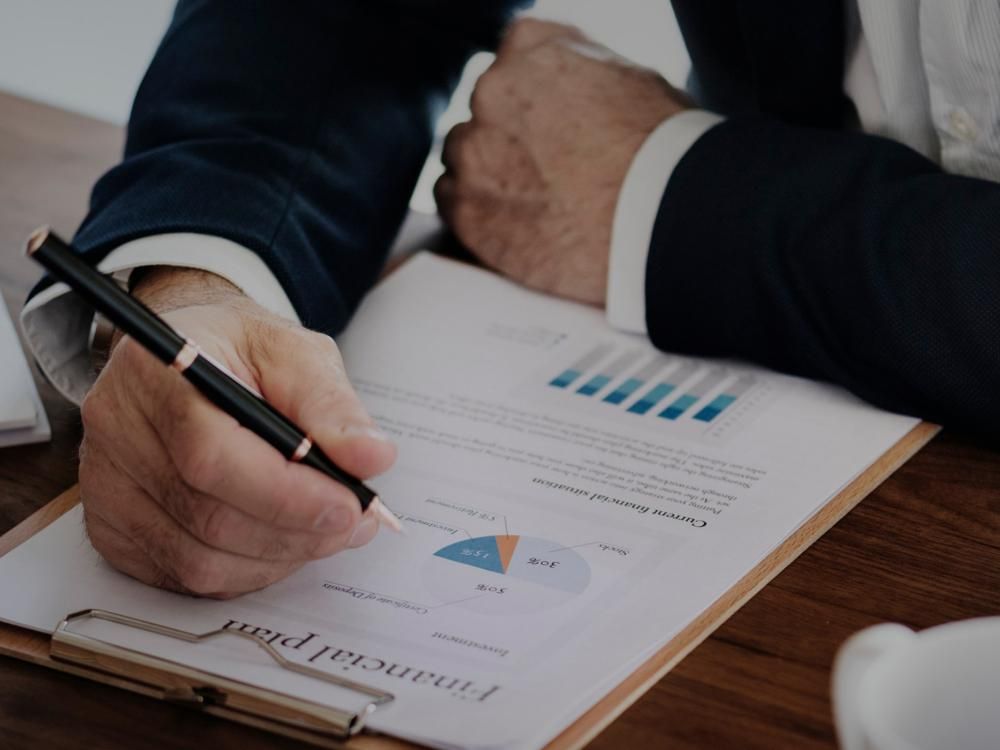
Detailed
BI implementation strategy and roadmap
BI consulting and engineering for a commercial bank
Itransition developed a detailed BI implementation strategy and prepared improvement recommendations for the customer’s data architecture as well as performed data modeling, redeveloped ETL operations, and conducted a detailed comparison of BI platforms.
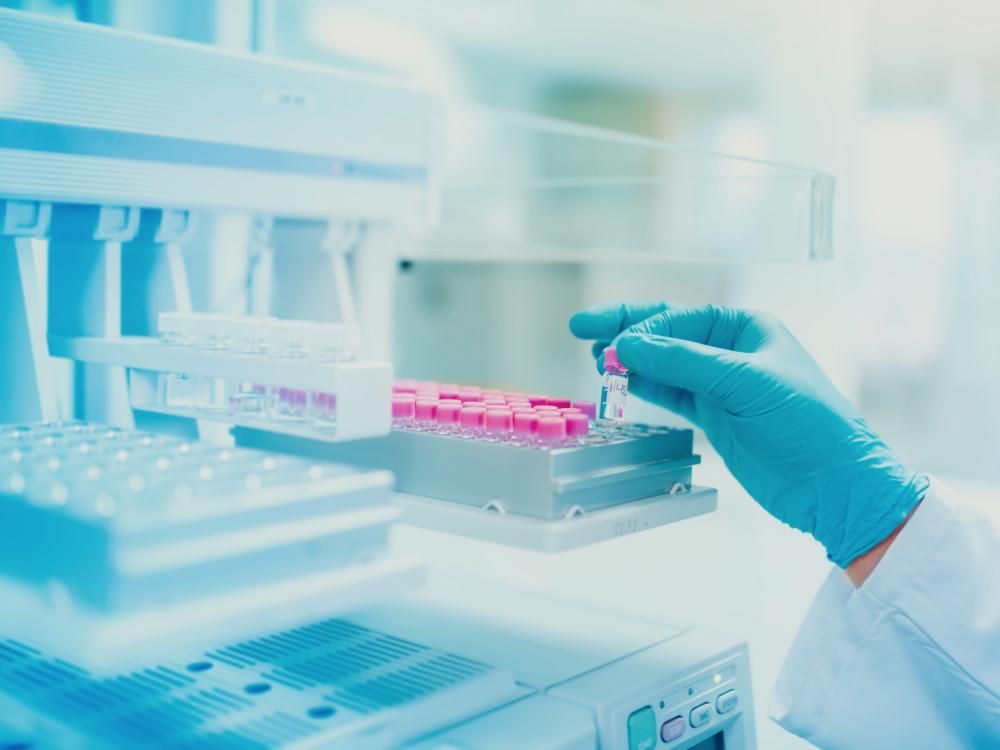
10x faster
data processing
Pharmaceutical BI for a US multinational
Itransition redeveloped the customer’s BI platform and migrated it to the cloud to help the customer streamline data processing, introduce market-forecasting algorithms, and get valuable insights into employee performance.
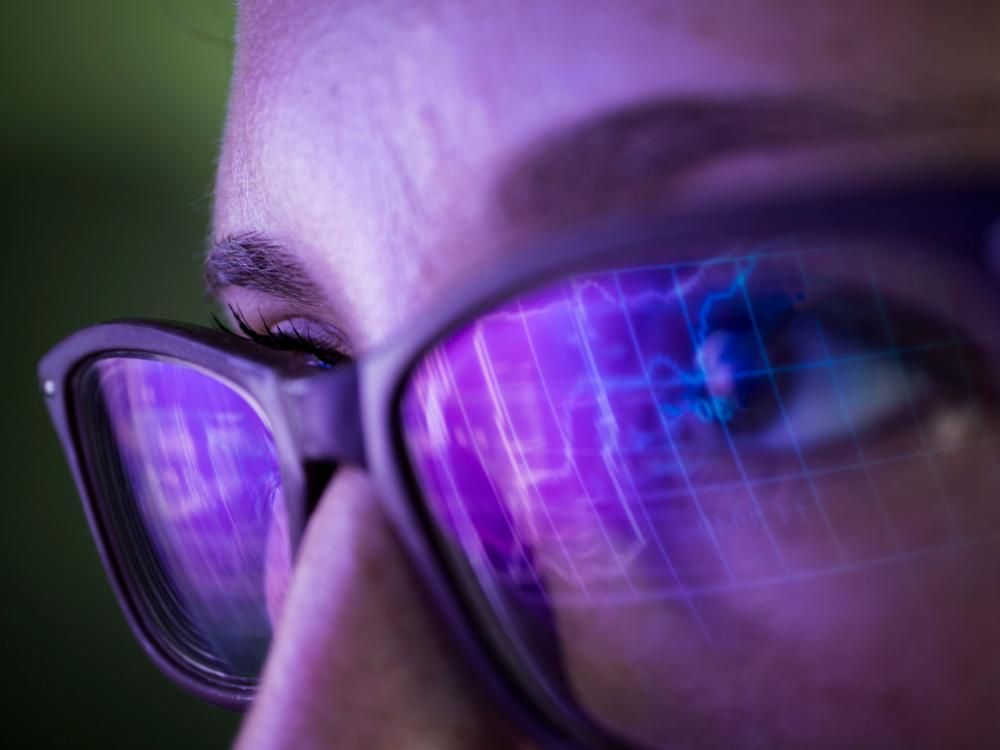
30% reduced
report opening time
BI solution enhancement for InsightSoftware.com
Itransition improved the solution’s stability and report-building performance, implemented history tracking features, and performed integration with SAP to help the customer decrease report memory usage and reduce report opening time by 30%.
Our customers say
We recommend Itransition as a competent and reliable technology consultant and partner, capable of unlocking the full potential of your data management solution, while aligning it with your specific needs. They are able to dive deep into your business-critical project, no matter how challenging and time sensitive, and quickly uncover and resolve issues of any complexity, demonstrating a highly professional approach and true dedication to project success.
Vamsi Behara
Data Platform Director, 5CA
We are extremely happy with the quality of Itransition’s work, as evidenced by the reduction in time to render the report from 60 to 1-2 seconds! It took the Itransition team only 2 weeks to optimize the embedded Power BI and enhance its maintainability. Their impact didn’t stop there. They also enhanced the Power BI report design to make the navigation responsive and logical, provided support and enhancement of our .NETbased web app, and applied Azure DevOps best practices. We were consistently impressed with the skills and dedication their team employed to fulfill our business needs.
John Joseph
Head of Technology, Solomon Coyle
Itransition provided a flexible team of highly skilled Microsoft-certified experts that was always in touch with us, reacting to project changes in real time. During the move to Power BI, we requested an acceleration of the process, and Itransition instantly onboarded additional Power BI developers to make the deadline of 6 months for migrating 150+ reports. Itransition professionals consulted us on the latest BI practices, providing ideas for the most optimized configuration of services and products and delivering a stable and flexible solution to production. We have already recommended Itransition to our partners. We will not hesitate to suggest collaborating with their BI and data warehousing experts on projects that require a professional, scalable, and highly skilled team.
Alon Kovner
Managing Director, Indigina Technologies Limited
The delivered solution for clinical trials analytics is sure to become an indispensable tool in our everyday interactions with end clients, helping visualize complex data sets, identify recurring trends, and enable streamlined decision-making. We found collaboration with Itransition straightforward and effortless as the team showcased a high degree of personal accountability and creativity, from the start positioning themselves as Power BI consultants and going beyond our expectations. The team's professionalism, expertise, and attention to detail were impressive and resulted in an excellent product, and we would recommend Itransition to anyone looking for true Power BI experts.
Dr. Syed Shah
Executive Vice President, Clinical & Strategic Operations Transformative Pharmaceutical Solutions
We recommend Itransition as a competent and reliable technology consultant and partner, capable of unlocking the full potential of your data management solution, while aligning it with your specific needs. They are able to dive deep into your business-critical project, no matter how challenging and time sensitive, and quickly uncover and resolve issues of any complexity, demonstrating a highly professional approach and true dedication to project success.
Vamsi Behara
Data Platform Director, 5CA
We are extremely happy with the quality of Itransition’s work, as evidenced by the reduction in time to render the report from 60 to 1-2 seconds! It took the Itransition team only 2 weeks to optimize the embedded Power BI and enhance its maintainability. Their impact didn’t stop there. They also enhanced the Power BI report design to make the navigation responsive and logical, provided support and enhancement of our .NETbased web app, and applied Azure DevOps best practices. We were consistently impressed with the skills and dedication their team employed to fulfill our business needs.
John Joseph
Head of Technology, Solomon Coyle
Itransition provided a flexible team of highly skilled Microsoft-certified experts that was always in touch with us, reacting to project changes in real time. During the move to Power BI, we requested an acceleration of the process, and Itransition instantly onboarded additional Power BI developers to make the deadline of 6 months for migrating 150+ reports. Itransition professionals consulted us on the latest BI practices, providing ideas for the most optimized configuration of services and products and delivering a stable and flexible solution to production. We have already recommended Itransition to our partners. We will not hesitate to suggest collaborating with their BI and data warehousing experts on projects that require a professional, scalable, and highly skilled team.
Alon Kovner
Managing Director, Indigina Technologies Limited
The delivered solution for clinical trials analytics is sure to become an indispensable tool in our everyday interactions with end clients, helping visualize complex data sets, identify recurring trends, and enable streamlined decision-making. We found collaboration with Itransition straightforward and effortless as the team showcased a high degree of personal accountability and creativity, from the start positioning themselves as Power BI consultants and going beyond our expectations. The team's professionalism, expertise, and attention to detail were impressive and resulted in an excellent product, and we would recommend Itransition to anyone looking for true Power BI experts.
Dr. Syed Shah
Executive Vice President, Clinical & Strategic Operations Transformative Pharmaceutical Solutions
Ensure the success of your BI project with Itransition
Our approach to BI services provision
Flexible pricing models
Partnerships should be flexible and adaptable. Thus, to match the size and scale of your BI project, we offer time and material and a fixed-price model.
Data security
We create data-centric BI solutions with robust security capabilities to reduce the risk of data breaches and other security incidents, as well as ensure compliance with internal governance policies and external regulations.
Agile delivery
We follow an iterative approach to reduce BI solution’s payback time and allow our clients to be actively involved in the project.
Technologies we work with
Data integration & quality management
- Azure Data Factory
- AWS Glue
- Talend
- Syncsort
- Azure Stream Analytics
- AWS Data Exchange
- Informatica PowerCenter
- Striim
- Azure Functions
- Amazon MWAA
- MuleSoft Anypoint Platform
- Pentaho
- Azure Event Grid
- Oracle Data Integrator
- IBM InfoSphere Information Server
- Fivetran
- Azure Event Hubs
- Databricks
- SnapLogic
- Matillion
- Microsoft SQL Server Integration Services
- Apache NiFi
Data storage
- Azure Blob Storage
- Azure Cosmos DB
- Amazon ElastiCache
- MySQL
- Azure Data Lake Storage
- Amazon S3
- Google BigQuery
- Oracle
- Azure SQL Database
- Amazon Redshift
- Google Cloud Storage
- IBM Cloud Object Storage
- Azure Synapse Analytics
- Amazon Aurora
- Microsoft SQL Server
- SAP HANA Cloud
- Azure SQL Database elastic pool
- Amazon RDS
- PostgreSQL
- Teradata
- Azure SQL Managed Instance
- Amazon DynamoDB
Data analytics
- Azure Synapse Analytics
- Microsoft SQL Server Analysis Services
- Amazon Athena
- Google BigQuery
- Azure Analysis Services
- Amazon Redshift
- Amazon Kinesis Data Analytics
Data visualization
- Microsoft Power BI
- Amazon Quicksight
- Apache Superset
- Chartist.js
- Altair
- Fusion Charts
- Tableau
- Looker
- Metabase
- seaborn
- ggplot
- D3.js
- Qlik Sense
- Looker Studio
- Redash
- bokeh
- Infogram
- Excel
- QlikView
- Google Cloud Charts
- Datawrapper
- Plotly
Data security management
- Azure Active Directory
- Azure Key Vault
- AWS Cognito
- AWS KMS
- AWS Secrets Manager
- Azure Firewall
- Azure VPN Gateway
Artificial intelligence & machine learning
- Azure Machine Learning
- Amazon Rekognition
- Google Cloud AutoML
- NumPy
- Azure Cognitive Services
- Amazon Lex
- Google Cloud Dialogflow
- NLTK
- Azure Applied AI Services
- Amazon Polly
- OpenAI language models
- XGBoost
- Azure Bot Service
- Azure Language Understanding
- Scikit-learn
- CatBoost
- Azure LUIS
- Google Cloud Vision API
- SciPy
- PyTorch
- AWS Deep Learning AMIs
- Google Cloud Natural Language AI
- Matplotlib
- TensorFlow
- Amazon SageMaker
- Google Cloud Speech-to-Text
- Seaborn
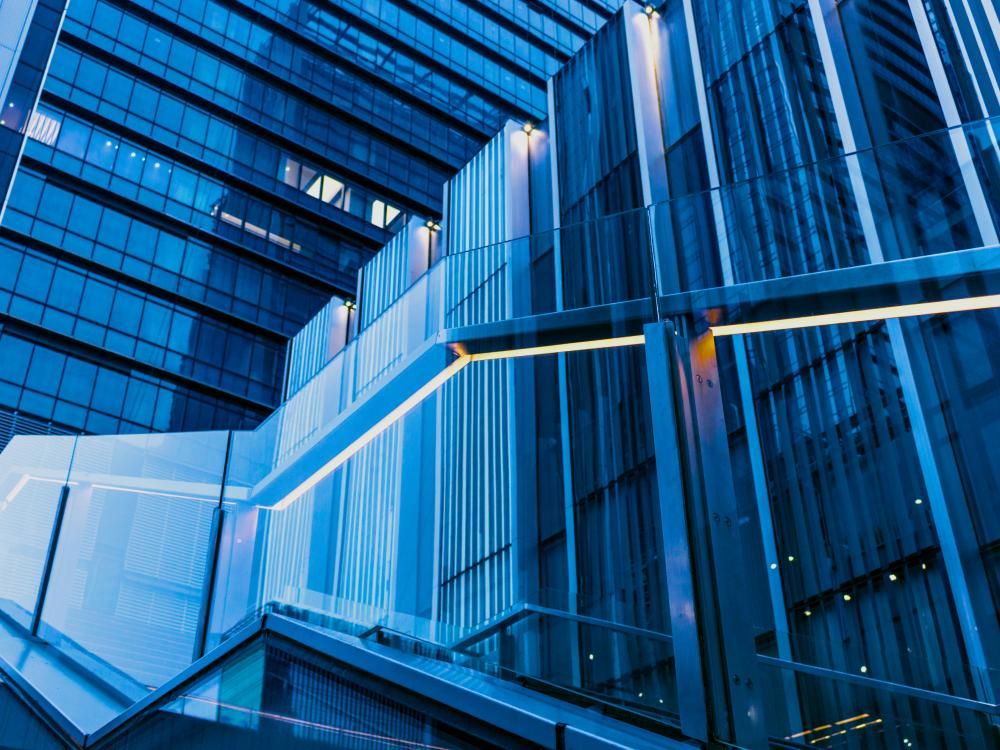
Case study
BI solution for case study coverage analysis
Read about Itransiiton’s case study and expertise scoring systems that help us easily analyze expertise coverage and pinpoint areas for improvement.
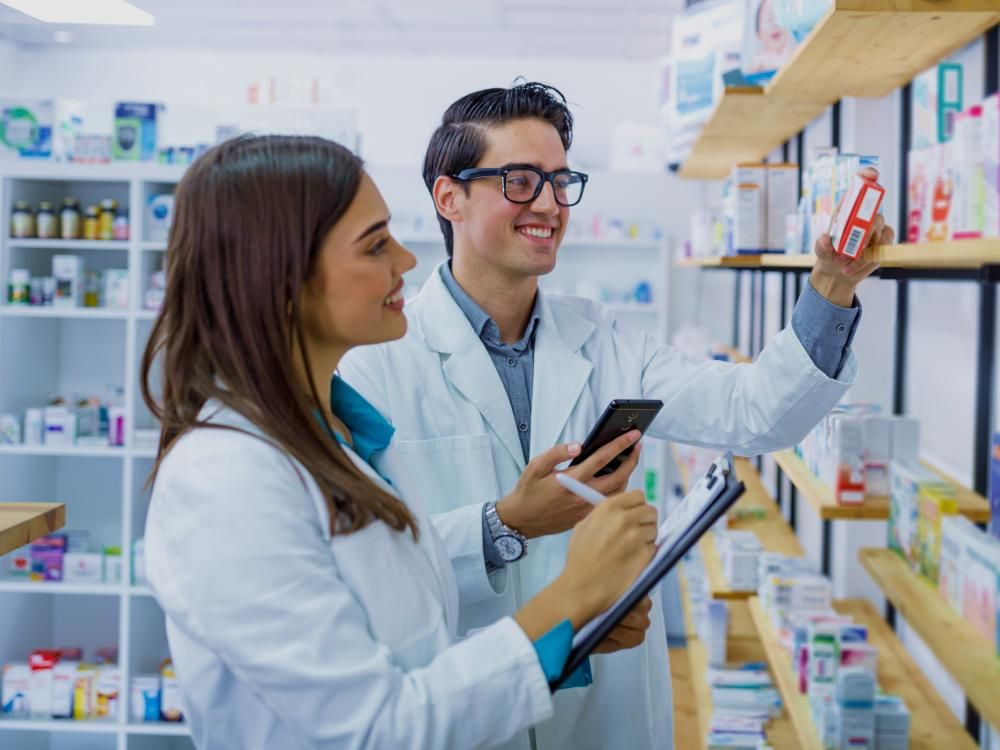
Case study
A dedicated client delivery team for healthcare BI
Learn how we set up a client delivery team to handle change requests, code reviews, and product support, accelerating new feature launches 8 times.
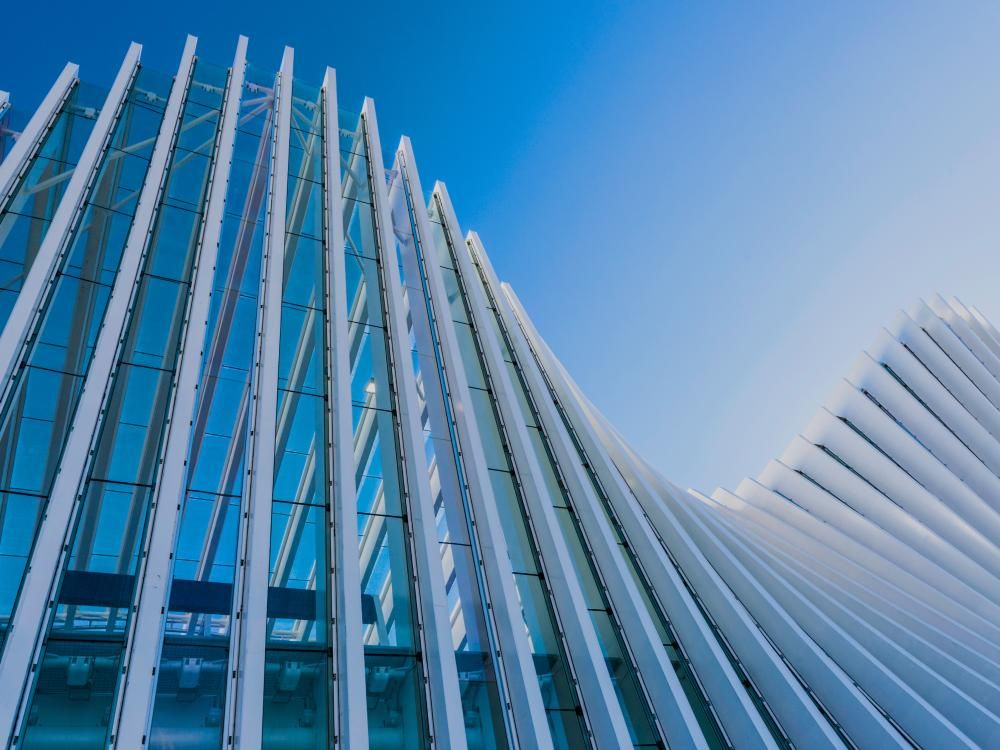
Insights
Business intelligence architecture: key components, benefits & tools
Learn about the components of BI architecture and their contents. Explore top software to implement BI architecture and how to overcome its adoption challenges.
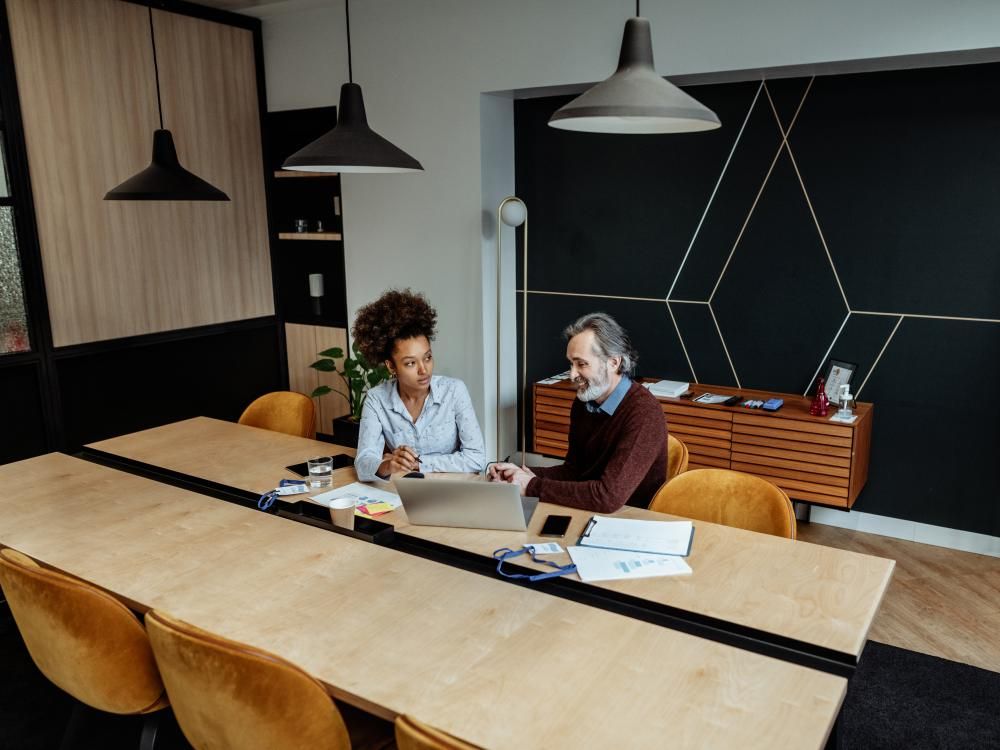
Insights
Operational business intelligence: essentials, pros, and applications
Let’s discuss operational business intelligence’s tech stack, use cases, benefits, challenges, and differences with traditional BI.
More about BI services
Services
Insights