
Enterprise business intelligence: capabilities, key components, and best solutions
April 3, 2023
- Home
- Business intelligence
- Enterprise business intelligence
Enterprise business intelligence is BI practices and tech infrastructure that consolidate business data from the company’s departments and divisions in a secure central storage for analysis and present actionable insights to end users based on their roles. Itransition delivers full-scale enterprise BI solutions for companies across industries to support operational and strategic business decisions.
Enterprise BI services we offer
We help companies that want to implement or enhance their existing BI solution by developing business intelligence roadmaps, conceptualizing BI solutions, choosing optimal technology, as well as creating data governance policies and rules.
We design and integrate multi-component enterprise business intelligence solutions within a set time and budget by covering every project step from business needs analysis to BI solution development and launch.
About Itransition
15+ years of experience with business intelligence
25+ years in enterprise software development
40+ successful BI projects
Strategic partnerships with Microsoft, AWS, and Google Cloud
ISO 27001 and ISO 9001-certified to ensure services quality and customer data safety
Selected success stories
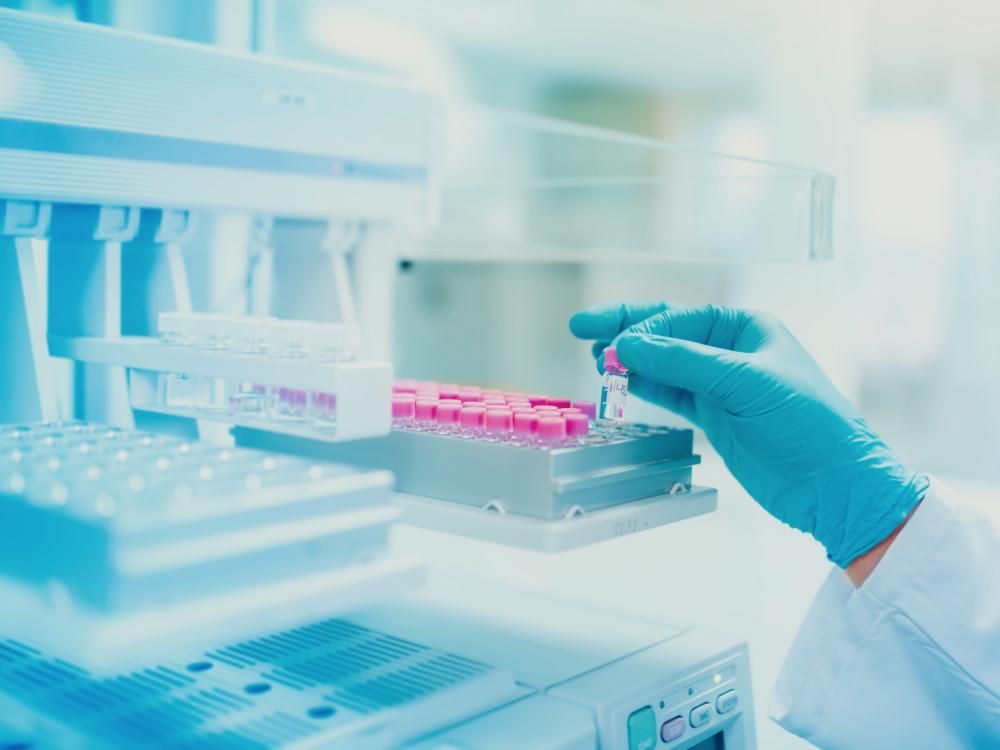
10x
faster data processing
Pharmaceutical BI for a US multinational
We redeveloped the customer’s business intelligence platform and migrated it to the cloud. Through this, we streamlined data processing, introduced market-forecasting algorithms, helped the customer get insights into employee performance, and reduced infrastructure costs.
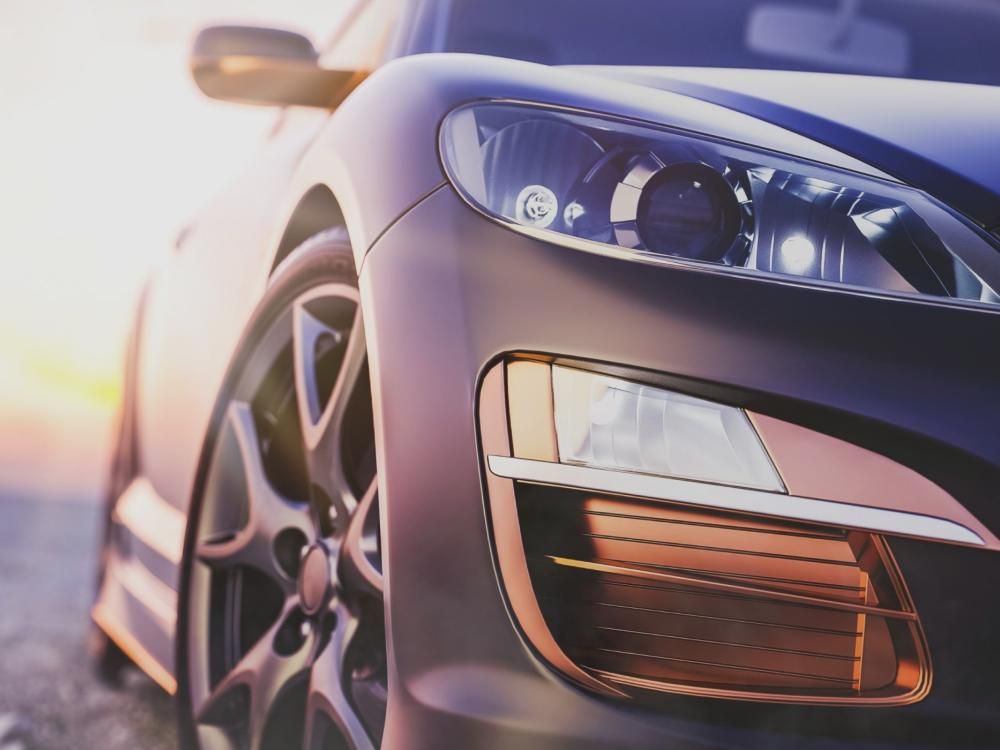
70%
faster time-to-market
Cloud BI for vehicle manufacturers
We transformed the customer’s legacy BI system to help them achieve a 15-20x higher system throughput, 100% predictability and transparency of working processes, and 50% higher productivity.
Looking for a trustworthy enterprise BI technology partner?
Key capabilities of enterprise BI
Data consolidation and storage
Consolidating diverse data sets in a unified data storage, making data accessible and ensuring its appropriate format and quality for analytics and reporting.
- Seamless connection to existing data sources located on-premises and in the cloudÂ
- Ingestion of structured, semi-structured and unstructured data
- Batch and streaming data processing
- Data transformation and quality management enabled by the ETL/ELT processesÂ
- Consolidation of company-wide data in an enterprise data warehouse and business line data in subordinate data marts
- Accompanying analytics data stores with an operational data store and a data lake for operational and high-volume business data
- Storing business data in a relational, columnar, and multi-dimensional format
Data analytics
Aggregating and analyzing business data with various approaches and techniques to generate business insights and support fact-based decision-making.
- Data mining
- Diagnostic analytics
- Exploratory data analysis
- Predictive analytics
- Statistical analysis
- Prescriptive analytics
- Descriptive analytics
- Online analytical processing
Business reporting
Delivering actionable analytics insights in an easy-to-read format for end-users to help them with daily tactical decisions as well as strategic decision-making and planning. Â
- Time-based and rule-based distribution of static reports and dashboardsÂ
- Custom reporting with a pre-determined layout and a predefined set of KPIs
- Managed interactive reporting with configurable chart types, filters, formatting, language, and locale settingsÂ
- BI content embedding into web pages and applications to minimize the learning curve
- Mobile reporting
Managed self-service
Empowering end-users, regardless of their tech expertise, to find the data they need, and answer their ad hoc queries.
- Ad hoc analytical querying
- Intelligent data drilling
- Augmented analytics capabilities
- Intuitive user interface
- Easy-to-use report design for ad hoc reporting and analysis
- Natural language user interface
- Advanced analytics features for power users and IT admins
Collaboration and sharing
Empowering users to collaborate with coworkers and outside organizations without sacrificing data security.
- Reports and dashboards sharing and distribution
- Content commenting
- Alerting and notifications
- Personalized and chronological catalog of user activities and interactions
- Collaborative workplaces
- Secure BI content embedding
- Content search
- Automated report and dashboard updates
Visualization
Employing a wide range of visualization types, including heat maps, scatter plots, pie charts, and pivot tables, to turn complicated data into accessible reports and dashboards.Â
- Custom visualsÂ
- Pre-built visualsÂ
- Visual data querying
- Data storytelling
Data governance and security
Ensuring business data is used correctly and to the company’s benefit and that authorized users across all verticals access reliable, consistent, and timely information according to their roles.Â
- Role-based access control
- Configurable data securityÂ
- Dynamic data encryption and masking
- User activity log
- Data auditingÂ
- Enterprise data catalogs
- Compliance with internal and external regulations
Essential integrations for enterprise BI
CRM
- Customer behavior monitoring and modeling
- Customer satisfaction analysis
- Customer churn prediction
- Customer value analytics
- Analysis of marketing campaigns profitability
ERP
- Business process monitoring and evaluation
- Cause-effect analysis and bottleneck recognitionÂ
- Operational performance analysis, including driver analysis and gap analysis
- Operational performance forecasting
HRM
- Employee performance monitoring and evaluationÂ
- Employee satisfaction, engagement, and productivity analysis
- Employee turnover predictionÂ
- Employee retention analysis
- Workforce planning and scheduling
Supply chain management
- Procurement analytics
- Supplier performance analysis
- Demand forecasting
- Identification of optimal inventory levelsÂ
- Prediction of order fulfillment ratesÂ
- Supply chain risk analytics
Finance and accounting
- Financial performance monitoring and evaluation
- Profitability analysis
- Financial planning and budgeting
- Financial risk forecasting
Ecommerce platforms
- Sales performance analysis
- Customer behavior analytics
- Evaluation of marketing campaigns effectivenessÂ
- Customer churn analytics and forecasting
- Market trends analysis
Enterprise BI architecture and its components
Data sources
Data sources
These include internal and external data sources generating information for a BI system. Data sources range widely and include corporate solutions and applications supporting day-to-day business workflows, relational databases, IoT devices, internal documents and archives, a corporate website, surveys and statistics, in addition to information from business partners and competitors. Data sources for a BI system are defined by business needs, so not all digital information a company has access to should be analyzed by the BI solution.
Data integration and quality management
Data integration and quality management
Before analysis and reporting, data has to undergo some modifications to make it consistent, accurate, relevant, and complete. The choice of data integration methods (ETL, ELT, data replication, change data capture, streaming data integration, etc.) and the complexity of data quality management depend on the data type, format, volume and business analytics requirements.
Data repositories
Data repositories
When cleaned and consolidated, business information is further structured in an enterprise data warehouse according to the predefined data model. Traditionally, an EDW is complemented with data marts, built to cater to the needs of individual business departments and divisions. In addition to these analytics data repositories, an enterprise BI solution can include an operational data store (to store actual data for real-time reporting) and a data lake (to store various volumes of raw data for ML and big data workloads).
BI and analytics layer
BI and analytics layer
This component encompasses a set of solutions, such as query tools, OLAP software, data mining tools, and data visualization software, that enable both power and casual enterprise BI users to access and manipulate business data. The functional scope of this layer is based on the enterprise’s analytics maturity.
Data governance
Data governance
The aim of this component is to govern the BI workflow and ensure data security, availability, and quality, as well as proper and beneficial use.
Enterprise BI implementation roadmap
The typical procedure companies follow when implementing a BI solution includes the following steps:
1
Business needs analysis
2
Business and technology environment evaluation
3
Conceptualization
4
Architecture design
5
Deployment environment and tech stack selection
6
Project planning
7
Components development and delivery
8
QA and end users training
9
Launch
Our industry BI expertise
We create full-scale BI solutions for the retail sector to increase customer satisfaction, craft effective marketing and sales campaigns, and quickly identify new sales opportunities.
- Inventory levels optimization
- Inventory planning, including purchasing, replenishment, and allocation
- Product assortment assessment and planning
- Dynamic price modeling
- Customer satisfaction and loyalty analysis
- Customer lifetime value calculation
- Customer behavior modeling
- Customer attrition and retention analysis
- Supplier performance assessment and risk forecasting
- Analysis of marketing campaign effectiveness
- Marketing and sales KPIs tracking
- Sales performance analytics
- Sales forecasting and planning
Finance
Itransition delivers tailored enterprise BI solutions for financial institutions to mitigate financial risks, identify R&D opportunities, enhance customer experience, and increase customer lifetime value.
- Financial performance KPIs monitoring, including net profit, cash conversion cycles, and operating profit margins
- Tracking financial behavior and detecting fraudulent activities
- Spend analysis
- Analysis of investment strategies and benchmarking
- Revenue and profitability analysis
- Accounts receivable and accounts payable forecasting
- Financial risks monitoring and assessment
- Marketing campaigns profitability analysis
- Monitoring market trends and predicting customer needs/behavior
- Customer portfolio analysis
- Customer retention metrics monitoring, including attrition rate, average order value, repeat customer rate, and purchase frequency
- Customer lifetime value calculation
BI solutions for healthcare organizations to analyze patient and clinical data and improve patient care, diagnostics accuracy, care teams coordination, and treatment efficiency.
- Patient needs anticipation
- Customized treatment recommendations
- Patient risks prediction
- Patient readmission forecasting
- Patient satisfaction analysis
- Preventive appointment scheduling
- Caregivers performance monitoring and evaluation
- Outbreaks prediction
- Workload prediction and medical staff scheduling
- Healthcare insurance fraud detection
Manufacturing
We build enterprise BI solutions to aid manufacturing companies with production planning, informed decision-making, reducing production errors, and improving overall equipment efficiency.
- Tracking the performance of individual machines and departments
- Analyzing uptime and downtime per employee, machine, and department
- Scrap rates monitoring
- Production quality analysis
- Bottlenecks and shift performance analysis
- Suppliers performance monitoring and evaluation
- Demand forecasting
- Optimal inventory levels forecasting
- Procurement planning
Itransition creates BI solutions for marketing agencies to help target customers with the optimal products and services, maximize business performance with effective marketing strategies, and identify new target audiences.
- Measuring the effectiveness of marketing efforts by channels
- Analyzing marketing campaign performance
- Tracking trends in customer behavior
- Customer engagement analysis
- Customer satisfaction and loyalty analysis
- Customer lifetime value calculation
- Customer behavior modeling
- Marketing and sales resource planning
- Budget analysis and planning
- Customer attrition and retention analysis
Telecommunications
We deliver full-scale BI solutions to empower telecom providers to make meaningful upgrades to their services and support, create targeted campaigns, refine pricing strategies, launch new products or discontinue unprofitable ones based on customer needs.
- Analysis of customer needs, preferences, and demographics
- Customer profitability analysis
- Customer lifetime value calculation
- Customer churn prediction
- Customer attrition analysis
- Marketing campaign analysis
- Analysis of product performance, usage, and revenue
Real estate
Itransition builds BI solutions for the real estate sector that help companies spot new investment opportunities, enhance customer experience, maximize profits, and minimize risks.
- Property value analysis
- Analysis of variables affecting property’s long-term profitability
- Property sales analysis
- Marketing campaign monitoring and evaluation
- Property management analysis
- Market rent analysis
- Analysis of brokerage agents’ activities and performance
- Risk forecasting
Logistics
We create BI solutions for logistics organizations to work out cost-effective delivery routes, minimize delivery costs, improve their operational capacity planning, and maximize on-time deliveries.
- Fleet operations monitoring, including shipping time, loading time, and transportation costs
- Distribution schedules and route planning
- Carrier performance analysis
- Vehicle condition analysis
- Predictive analytics for vehicle maintenance
- Driver behavior analysis
- Fuel consumption monitoring and analysis
- Delivery delays and failures analysis
- Defining optimal delivery time, speed, and transportation mode
Education
We create tailored BI solutions for educational organizations to improve both students’ academic performance and the institution’s operational workflows, reduce management complexity, and foster data-driven decision-making.
- Students’ performance monitoring and evaluation
- Student intelligence, including course attendants, interests, GPA, teachers’ feedback, etc.
- Students’ retention analysis
- Teacher intelligence, including number of courses, course hours by grades, and students’ feedback
- Expenses and revenue analytics
- Workforce planning and scheduling
- Marketing campaigns effectiveness evaluation
Agriculture
Itransition designs and implements BI solutions for agricultural companies to optimize production, cut costs while ensuring high product quality, achieve their sales goals, and reduce production waste.
- Plant and livestock wellness monitoring and evaluation
- Soil and yield analysis
- Waste identification and analysis
- Recalls tracking and analysis
- Crop performance analysis
- Demand forecasting
- Production planning and forecasting
- Financial performance monitoring
- Farm machinery and equipment performance analysis
- Sustainability initiatives impact and performance analysis
- Food safety and security evaluation
- Weather tracking and forecasting
Looking for a vendor to implement enterprise business intelligence?
Best ready-made software for enterprise BI
Key features
- Ingestion of structured, semi-structured and unstructured data
- Streaming data ingestion
- Analytics querying of data from a data lake, an operational data store, and relational databases
- Support of big data workloads
- Native integration with the AWS ecosystem (AWS Lake Formation, Amazon EMR, Amazon QuickSight, and Amazon SageMaker)
- Built-in fault tolerance and disaster recovery
- Granular access controls
- Multi-factor user authentication
- Dynamic data masking
- End-to-end data encryption
- Compliant with SOC1, SOC2, SOC3, PCI DSS Level 1 and others
- Features for storage decoupling and resources computing (RA3 node type)
Category
- Data warehousing software
Platform pricing
On-demand pricing
from $0.25/hour
Reserved instance pricing
depends on the location type and region, term length, and payment option
Data storage (RA3 node type)
$0.024/GB/month
Free trial
Key features
- Ingesting data from 95+ data sources
- Native integration with Azure Data Factory, Azure Data Lake Storage, Azure Cosmos DB, Azure Machine Learning, Azure Cognitive Services, and Power BI
- Support for T-SQL, KQL, Python, Scala, Spark SQL, and .Net
- Separate billing and scaling of computing and storage resources
- Big data and streaming data ingestion with built-in Apache Spark and Azure Stream Analytics
- Built-in fault tolerance and disaster recovery
- Granular permissions on schemas, tables, views, and individual columns
- Multi-factor user authentication
- Data encryption
- Compliant with HIPAA, ISO 27001, PCI DSS, SOC1, SOC2, etc.
Category
- Data warehousing software
Platform pricing
On-demand
from $1.20/hour
Reserved instance pricing
depends on the location type and region, term length and payment option
Data storage
$23/TB/month
Free trial
Key features
- Pre-built connectors for 150+ data sources, including Salesforce, Excel, Google Analytics, Dynamics 365, text and CSV files
- Native integration with Azure Data Lake Storage Gen2, Azure Synapse Analytics, Azure SQL database, Azure Machine Learning Studio
- Support for DAX, Power Query, SQL, R, and Python
- Self-service data preparation, analysis, reporting, and visualization
- Pre-built and custom visuals
- Embedded BI
- Data storytelling capabilities
- NLP capabilities
- Team commenting and content subscriptions
- Row-level security with role-specific data protection and row-level security measures
- Mobile-ready
Category
- BI software
Platform pricing
Power BI Desktop
free
Power BI Pro
$9.99 per user/month
Power BI Premium
$20 per user/month or $4,995 per capacity/month with an annual subscription
Power BI Embedded
from $1.0081/hour
2-month free trial
for each new user
Key features
- Native integrations to 80 data sources, including local files, spreadsheets, relational and non-relational databases, data warehouses, big data, and on-cloud data sources
- Self-service data preparation, analysis, and reporting
- Team collaboration and sharing
- Drag-and-drop user interface
- Embedded analytics
- NLP capabilities
- Custom dashboard creation
- Row-level security
- Mobile-ready
Category
- BI software
Platform pricing
Tableau Creator
$70/user/month
Tableau Explorer
$35/user/month (fully hosted by Tableau)
Tableau Explorer
$42/user/month (on-premises or public cloud)
Tableau Viewer
$12/user/month (fully hosted by Tableau)
Tableau Viewer
$15/user/month (on-premises or public cloud)
Free trial
Key features
- Broad data connectivity, including file-based, on-premises, cloud-based and web sources
- Self-service data preparation, analytics, and reporting
- Automated visual recommendations
- Data storytelling
- Group sharing and collaboration
- Multiple user types support
- NLP capabilities
- Row- and column-level security
- Mobile-ready
- Embedded analytics
Category
- BI software
Platform pricing
Qlik Sense Business
$30/user/month
Qlik Sense Enterprise SaaS
custom pricing available upon request
Free trial
Key features
- Extract, load and transform architecture
- Prebuilt big data connectors for Hadoop, Spark Streaming, Hive, Kafka, HBase, Sqoop, Pig, Cassandra, NoSQL databases
- Extensive database support, including Oracle Autonomous Database, ERP and CRM systems, B2B systems, flat files, XML, JSON, LDAP, JDBC, ODBC
- Integration with Oracle GoldenGate, Oracle Enterprise Metadata Management, Oracle Enterprise Manager
Category
- Data integration and quality management software
Platform pricing
Data Integrator Enterprise Edition
$900 per named user plus $198 for a software update license and support
Enterprise BI: adoption best practices
Balance agility and data governance
On the one hand, enterprise business intelligence drives data democratization at all corporate levels and data-driven culture expansion, especially when paired with self-service capabilities. On the other hand, if not managed properly by a solid data governance framework, the solution’s validity can be compromised by sensitive data exposure, lack of trust in data, and data inconsistency.
Open architecture
When implementing a BI solution for an enterprise, make sure it scales easily without performance bottlenecks and a sharp increase in spending. Enterprise BI architecture should allow for a seamless integration with new data sources, the addition of new data repositories (a data lake and/or an operational data store, and data marts), and an increase in the number of users and analytics complexity.
Focus on end users
The success of enterprise BI implementation depends on user adoption rates. To increase user adoption, introduce self-service capabilities, conduct tailored user trainings and workshops on a regular basis, track user activity to spot problems early on, and ensure data quality from the very beginning to foster trust in the new software.
Enterprise BI cost factors

- Data sources - their number, attributes and integration flexibility
- Data complexity – data structure (structured, unstructured, semi-structured), data variety, and data volume
- Data cleansing complexity - is defined by the amount of corrupt, duplicate or inconsistent dataÂ
- Data storage requirements – if analytics data stored are to be complemented with a data lake or an ODS
- Data analytics complexity – if real-time, self-service, or augmented analytics is requiredÂ
- Data reporting complexity – number of reports and dashboards, their complexity and frequency, custom data visualization, and self-service capabilities
- Data security complexity - compliance requirements, complexity of access management and controls, and data backups
Need help with choosing the optimal tech stack for your enterprise BI solution?
Benefits of implementing enterprise BI
Data consolidation
Integration of different types of enterprise data from siloed systems with varying data models into a single unified storage.
Enhanced data quality and value
Automation of data management and integration of a centralized data governance approach. This helps ensure data safety, consistency, accuracy, timeliness, completeness, and validity across different departments and divisions.
Data-driven decision-making
Quick access to high-quality relevant business data, elimination of communication bottlenecks, and convenient self-service capabilities to meet varying analytics and reporting needs of different-level users.
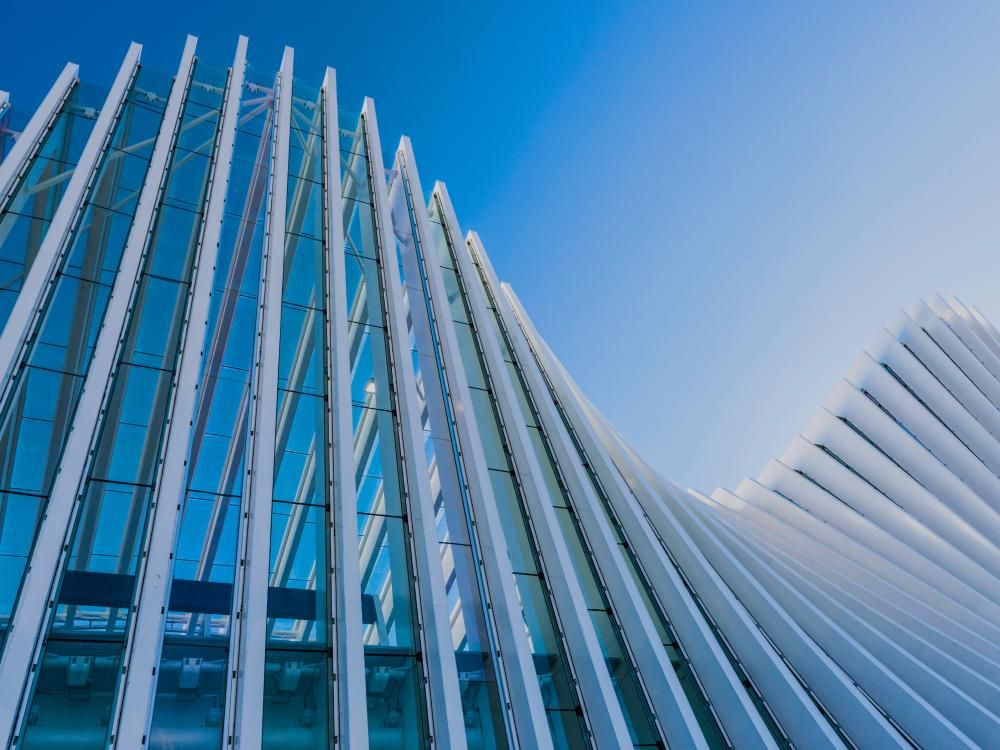
Insights
Business intelligence architecture: key components, benefits, and BI team
Discover what business intelligence architecture (BI architecture) is and what components and skills are needed to build a high-performing BI solution.
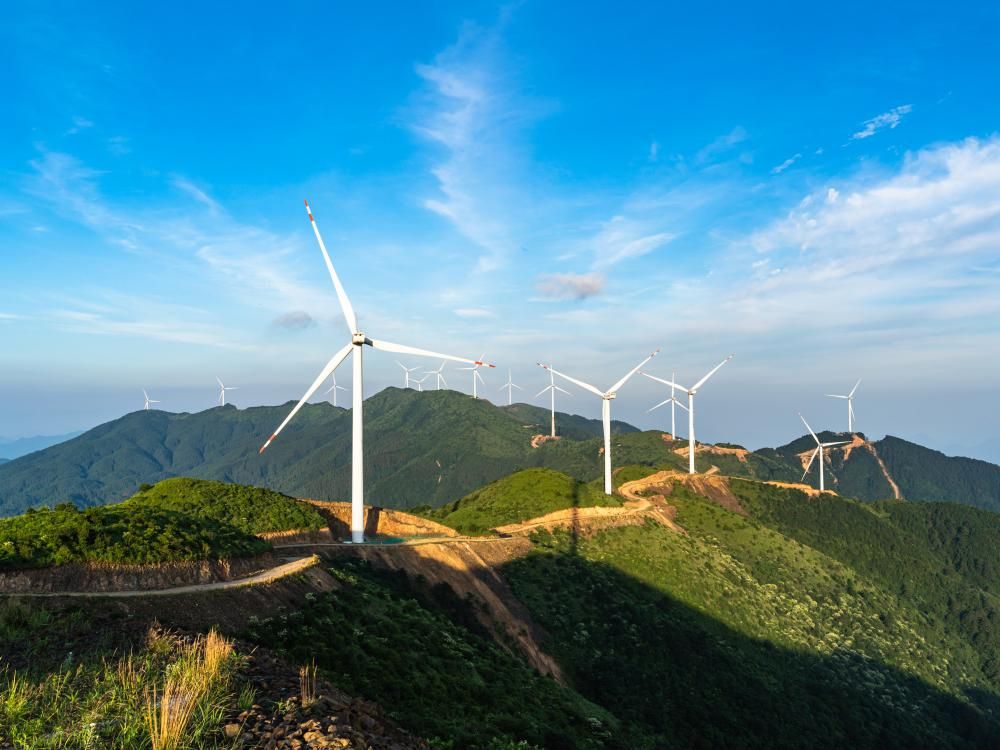
Insights
Self-service BI: use cases, features, and platforms to consider
Explore the concept of self-service BI and how it differs from traditional BI systems, as well as the value it brings and the challenges it may pose.
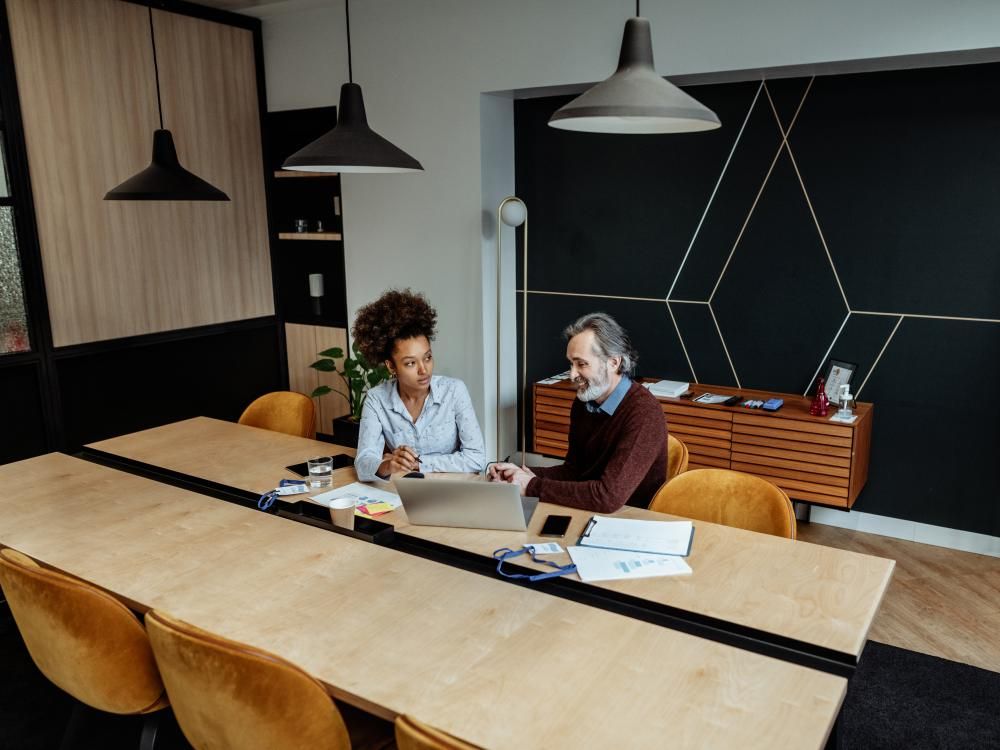
Insights
Operational business intelligence: essentials, pros, and applications
Let’s discuss operational business intelligence’s tech stack, use cases, benefits, challenges, and differences with traditional BI.
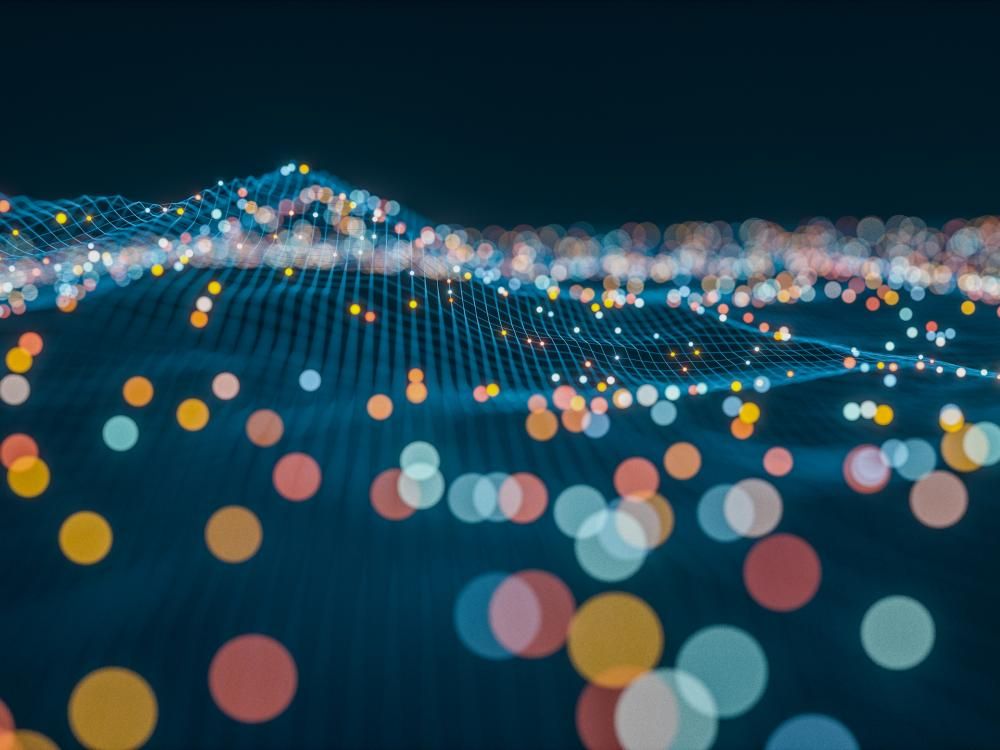
Insights
Building a data warehouse: a step-by-step guide
We overview the process of building a data warehouse (DWH), including architectural approaches, key steps, talents required, software and best practices.
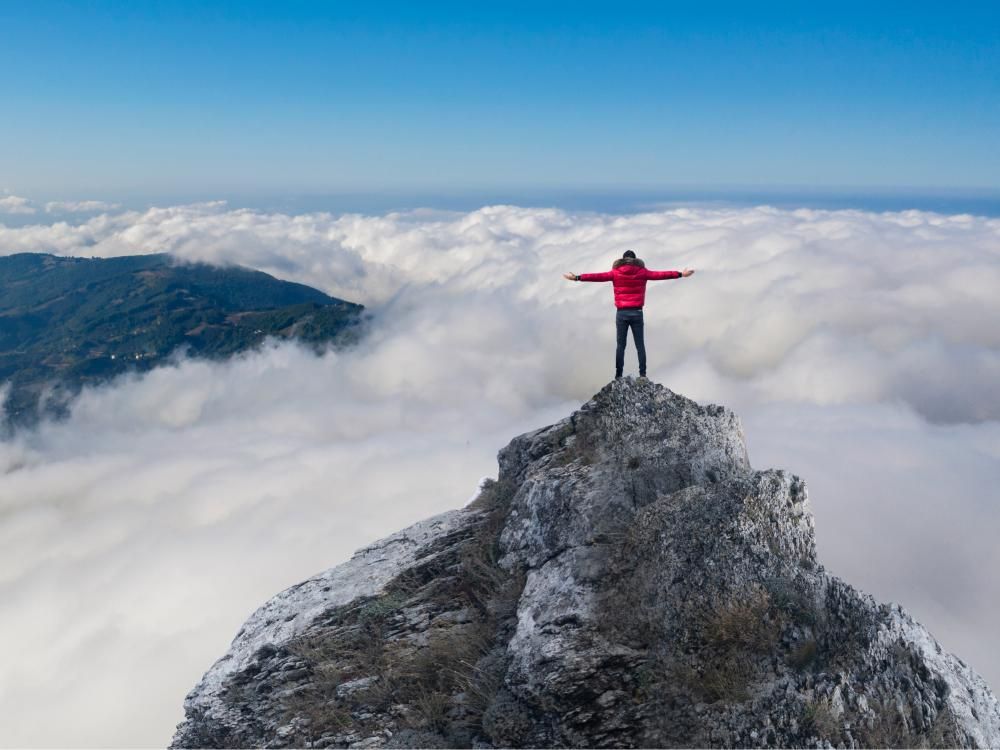
Insights
Cloud business intelligence: the whys and hows
Learn why cloud business intelligence has become an imperative for enterprise success and how businesses can choose the right cloud BI tool for their needs.