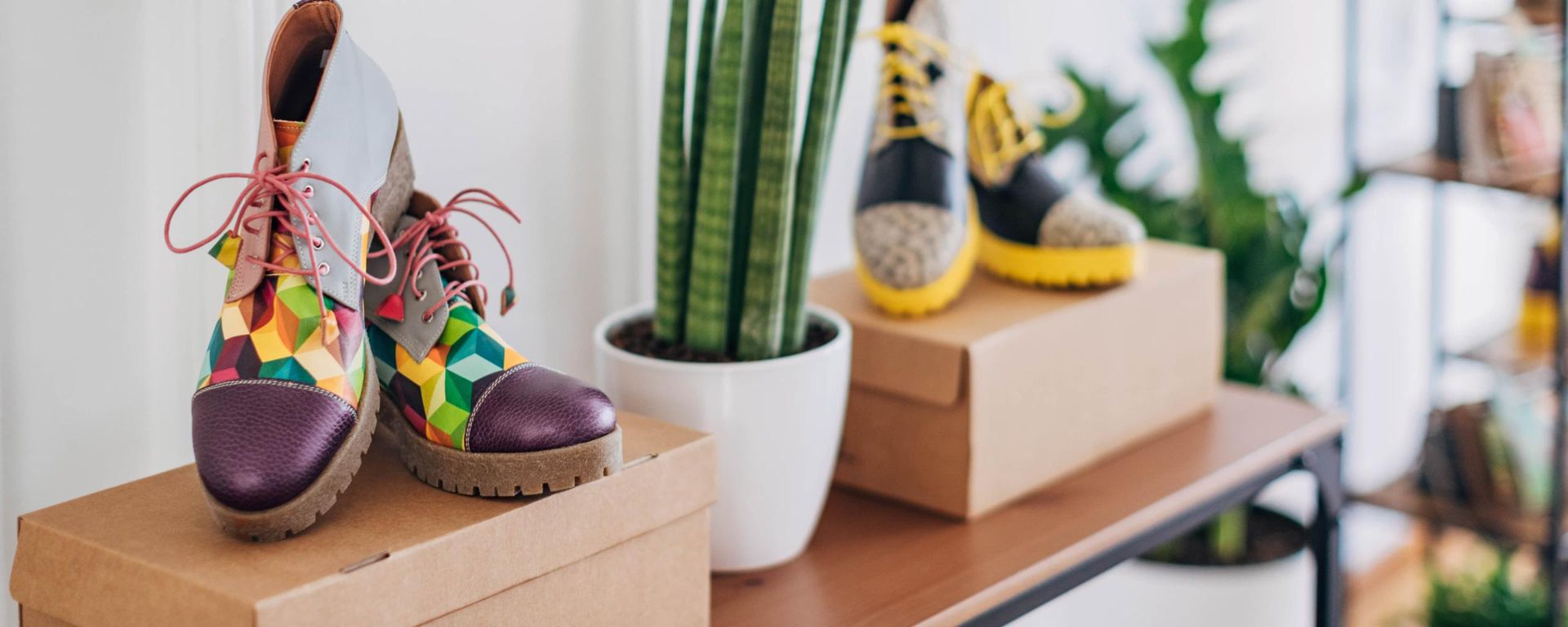
Predictive analytics in retail: use cases, examples & adoption guide
August 22, 2023
- Home
- Predictive analytics
- Predictive analytics in retail
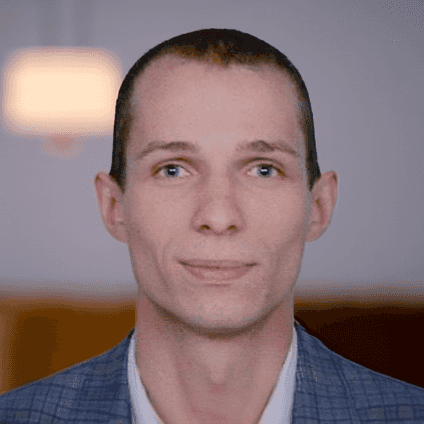
Head of AI/ML Center of Excellence
Predictive analytics has emerged as an invaluable tool for retailers. The ability to anticipate consumer behavior and timely respond to ever-changing market conditions is one of the main competitive advantages in retail. With data analytics becoming more accessible, retailers heavily rely on predictive analytics services to conquer the market.
Table of contents
The role of predictive analytics in retail
In 2022, predictive analytics-powered programmatic advertising and market forecasting accounted for a 33.1% share of the global AI in retail market revenue.
Scheme title: Global AI in retail market revenue share, by application, 2022
Data source: gminsights.com — Artificial Intelligence in retail market
24%
projected CAGR of the global predictive analytics in retail market from 2023 to 2032
Global Market Insights
40%
more revenue is generated by companies that excel at personalization
McKinsey
Top 8 use cases of predictive analytics in retail
With the help of predictive analytics, retailers can solve various business challenges and gain considerable competitive advantage. Let’s explore the top 8 use cases of predictive analytics in retail:
sales increase when making data-driven decisions around stock and store optimization
McKinsey
Benefit
Personalizing customer experiences
of leading retailers consider customer loyalty and LTV as top priorities
Deloitte
Benefit
Developing targeted marketing campaigns
increase in product sales for retailers that use data-driven targeting
Deloitte
Benefit
Creating pricing strategies
immediate margin performance improvement due to data-driven pricing management
Deloitte
Benefit
Enabling smart upselling & cross-selling
sales increase for fashion companies that use data-powered product offering
McKinsey
Benefit
Automating customer service
acceptance rate of artificial intelligence chatbots in retail (higher than any other industry)
Invesp
Benefit
Optimizing merchandising strategies
In tandem with computer vision, predictive analytics can help optimize merchandising strategies by analyzing shoppers in-store movement and historical data on past purchases. Knowing the purchase history, merchandisers identify key metrics for improvement and develop hypotheses for improving UI accordingly to boost sales and profits.
of retailers in Deloitte's study scored themselves as mature or leading in product recommendations
Deloitte
Benefit
Reducing customer churn
Benefit
Itransition offers a suite of predictive analytics services for retailers, developing tailored solutions to help your business take full advantage of the power of retail predictive analytics.
- We provide expert consulting services that enable retailers to effectively identify areas where predictive analytics can bring the most value and develop optimal strategies for leveraging it.
- We help organizations define their goals and objectives, build effective data pipelines, and select the right technologies for their needs.
- We build predictive analytics models and algorithms that are tailored to each retailer’s unique needs.
- We use the latest machine learning techniques to develop advanced solutions that enable retailers to leverage real-time insights for better decision-making.
Ready to unleash the power of predictive analytics for your retail business?
Real-life predictive analytics examples in retail
Many retail industry leaders use predictive analytics to improve operational efficiency and customer experience. Some notable examples include:
Farfetch & Talkdesk
Farfetch & Talkdesk
Farfetch is one of the leading global platforms for the fashion industry, with a presence in over 190 countries worldwide. However, an internal audit revealed that Farfetch’s contact center faced many service and quality issues due to rapid international expansion. As the company opened offices and contact centers across the globe, new contact center agents were overwhelmed with information in the first 30 days on the job. To tackle this issue, the company needed an efficient solution that would cater to the needs of both veteran employees and newbies.
.png)
Image title: Agent Assist in action
Data source: talkdesk.com — Talkdesk Agent Assist, empowering agents to support customers
Farfetch turned to Talkdesk, a cloud contact center company, to find much-needed help in the form of AI and predictive analytics. As a result, one of Talkdesk’s signature services, Agent Assist, now provides customer support agents with real-time tips during conversations with customers. For example, with the help of NLP and predictive analytics, Agent Assist can transcribe calls in real time, automatically generate call summaries, suggest the next best actions, and reveal relevant articles from Farfetch’s existing knowledge base to provide customers with quick answers.
25%
increase in customer satisfaction
50%
decrease in resolution times
Belk & antuit.ai
Belk & antuit.ai
Belk is a California-based fashion retailer that operates nearly 300 retail stores across the US. Belk’s store managers were struggling to understand why some physical stores significantly outperformed others. Basing their sales forecasts on historical buying patterns rather than future trend analysis wasn’t effective. Realizing that a comprehensive data analytics solution is essential to solving such issues, Belk invested $130 million in a technological transformation. Predictive analytics-powered sales forecasting was Belk’s top priority, so the company partnered with antuit.ai to develop a custom end-to-end demand forecasting platform. Antuit’s bespoke platform incorporated AI-enabled analysis of seasonality, promotions, events, and many other factors to produce accurate forecasts. As a result, each store’s manager now has a much deeper insight into inventory management, which drastically increases their chances of accurately predicting product demand.
DICK’S Sporting Goods & Adobe
DICK’S Sporting Goods & Adobe
DICK’S is one of the largest sports goods retail chains with over 150 million customers across 850 US stores. With such a massive number of customers, the ecommerce program manager at DICK’S realized that the only way to deliver personalization at scale is big data analytics. Therefore, DICK’S turned to Adobe to provide customers with tailored experiences across every channel. For example, the Adobe Customer Journey Analytics platform consolidates all the data in one place, allowing DICK’S to determine how exactly certain customer activities at different touchpoints impact their purchase decisions. With the help of Adobe Customer Journey Analytics and a stack of other Adobe tools, DICK’S can quickly decipher customer intent based on myriad factors, including customer interests, location-relevant events, and past purchases, to enable real-time personalization.
We know within milliseconds if someone is browsing a particular brand’s footwear on the website, that they are an athlete who would benefit from engaging more with that brand while they’re online.
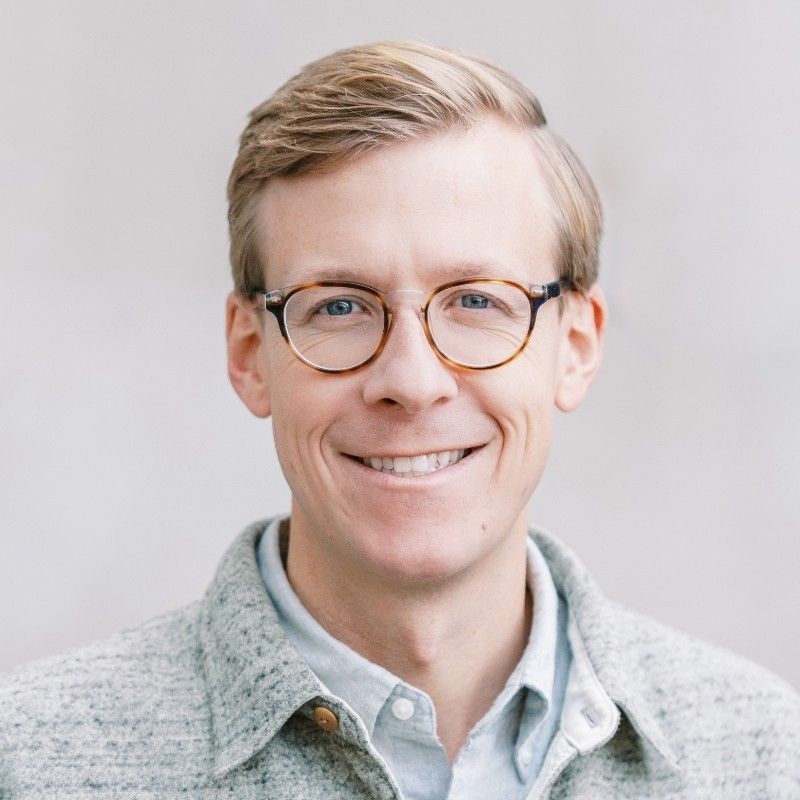
Steve Miller
Senior Vice President, Strategy, eCommerce & Analytics, DICK’S Sporting Goods
2X
more homepage visitors get a personalized experience
10%
more spent by visitors receiving a personalized experience than those who do not
Skullcandy & Sisense
Skullcandy & Sisense
Skullcandy is a US-based company that produces consumer-grade audio equipment, including headphones, earbuds, and speakers. The company’s product development team required a more detailed understanding of their customers’ needs and sentiments about existing products to come up with better-performing new products. By feeding Sisense’s bespoke predictive analytics engine with historical warranty costs, claims, product attributes, and attributes of potential new products, Skullcandy developed a reliable predictive data model to detect what impacts the warranty costs of a new product before it hits the market. On top of that, Sisense helped Skullcandy to leverage its BI platform, custom NLP engine, and Amazon Comprehend to granularly understand customer sentiment. Currently, Skullcandy can seamlessly correlate positive and negative sentiments to a particular product performance, which yields compelling insights for future product development.
Here at Skullcandy, we’re happy to report that “dropping in” to the predictive and sentiment analytics game was worth the initial uncertainty.
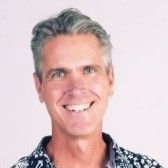
Mark Hopkins
Chief Information Officer, Skullcandy
Predictive analytics implementation roadmap
Here’s how you can start leveraging predictive analytics for your retail business:
1
Problem definition
2
Data analysis
3
Design
4
Implementation
5
Deployment
6
Support and maintenance
Top 5 predictive analytics platforms for retail
Pros
- Highly customizable
- Built-in data cleaning capabilities
Cons
- Expensive for small businesses
- Steep learning curve
Pricing
Pro version is
$13.70 user/month
Premium version
is $27.50 user/month, and $6,858.10 capacity/month
Pros
- Drag and drop interface
- Mobile-friendly
- Supports many data sources
Cons
- Inflexible pricing
- Poor versioning
- Requires SQL knowledge in many cases
Pricing
From $15 to $70 per month per user
Qlik Sense
Pros
- Allows for complex data analysis
- Supports self-service
Cons
- Computationally expensive
- Poor integration with other software
Pricing
Quote-based
TIBCO Data Science
Pros
- Highly secure
- Comprehensive analytics capabilities
- Seamless integration with other services
Cons
- Steep learning curve
- Lack of documentation
Pricing
Upon request
Alteryx
Pros
- Intuitive drag-and-drop interface
- Supports various data types
- Excellent data cleansing capabilities
Cons
- Limited visualization
- Limited data storage options
Pricing
From $2,300 to $5,195 per user per year
Start using predictive analytics to boost your sales and improve customer experience
Adoption challenges
Some of the most common challenges associated with the implementation of predictive analytics in retail include the following:
Challenge/Description
Challenge
Solution
Description
Solution
Outdated team structure
Outdated team structure
Lack of talent
Lack of talent
Inflexible legacy systems
Inflexible legacy systems
Modernize the existing architectures by leveraging microservices and APIs to reduce the complexity of integrating new analytics-driven solutions.
Siloed data
Siloed data
Join the retail leaders with predictive analytics
Predictive analytics has become essential for retail companies looking to strengthen their competitive edge, improve customer experience, and increase efficiency. By leveraging advanced data-driven technologies such as machine learning and artificial intelligence, retailers can gain actionable insights and unlock new market opportunities. With over two decades of expertise in cloud technologies and advanced data analytics solutions, Itransition provides full-cycle predictive analytics services tailored to the retail sector’s needs and specifics. Start your journey today and join the leaders in the industry.
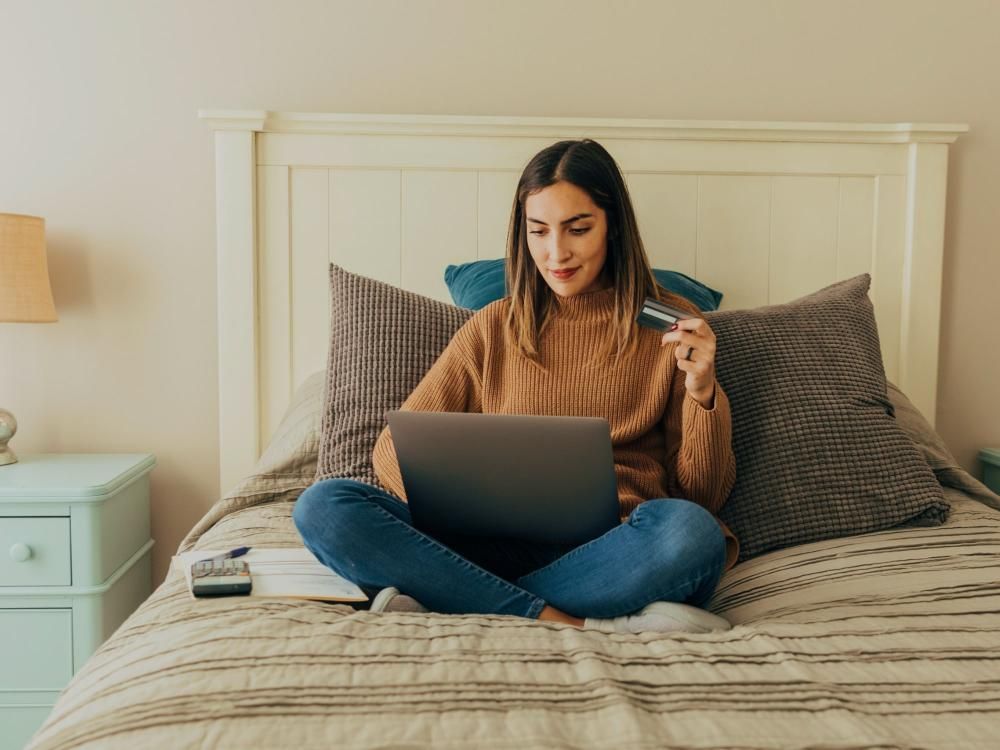
Insights
Ecommerce personalization: tactics, examples, and adoption guide
Personalization in ecommerce is indispensable today. Explore powerful tactics for creating unique customer experiences and get practical implementation tips.
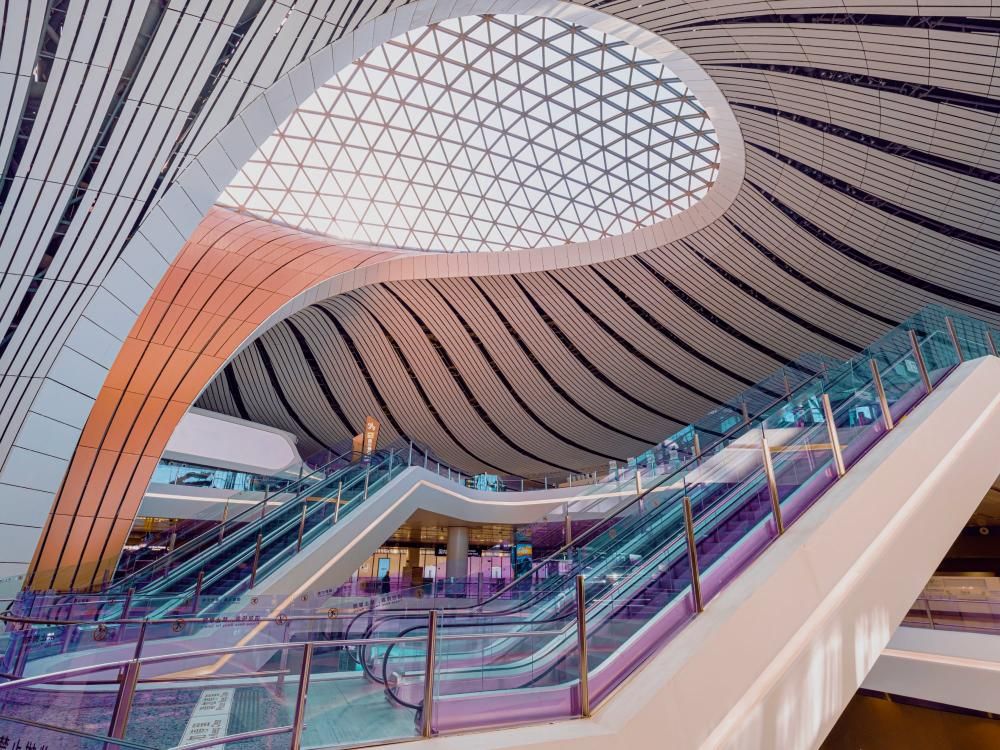
Insights
Business intelligence in the retail sector: key features, benefits, and platforms
Learn how business intelligence solutions transform the retail sector and explore their key features, integrations, best software, cost factors, and benefits.
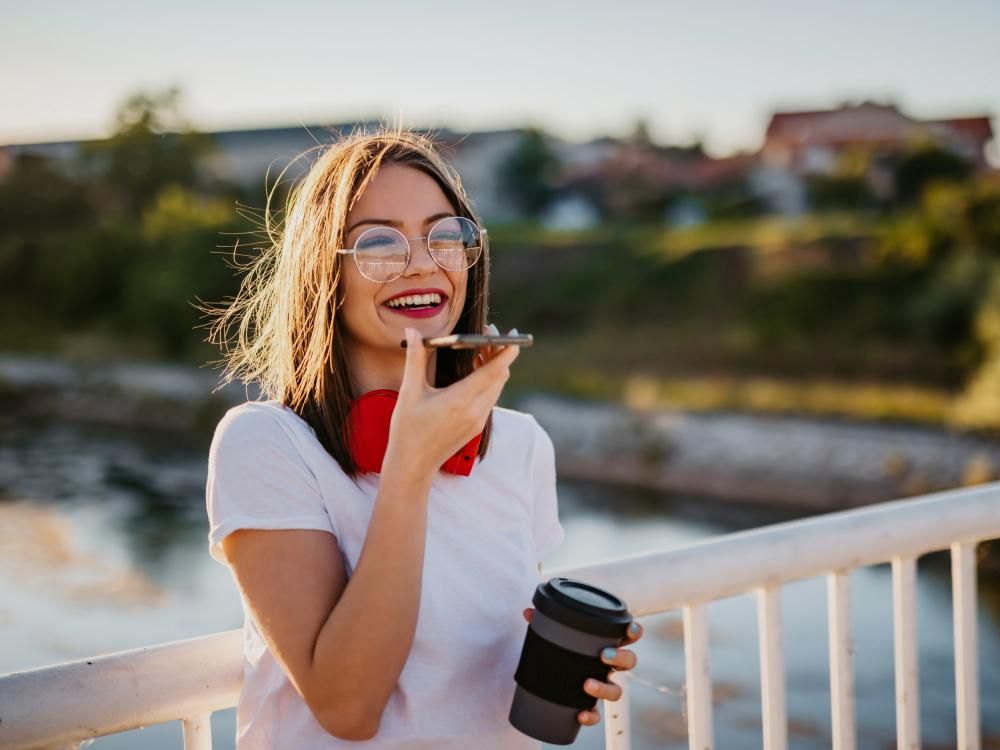
Insights
How voice commerce transforms online retail
This article explains the concept of voice commerce, covers its use cases and implementation challenges, and provides some adoption tips.
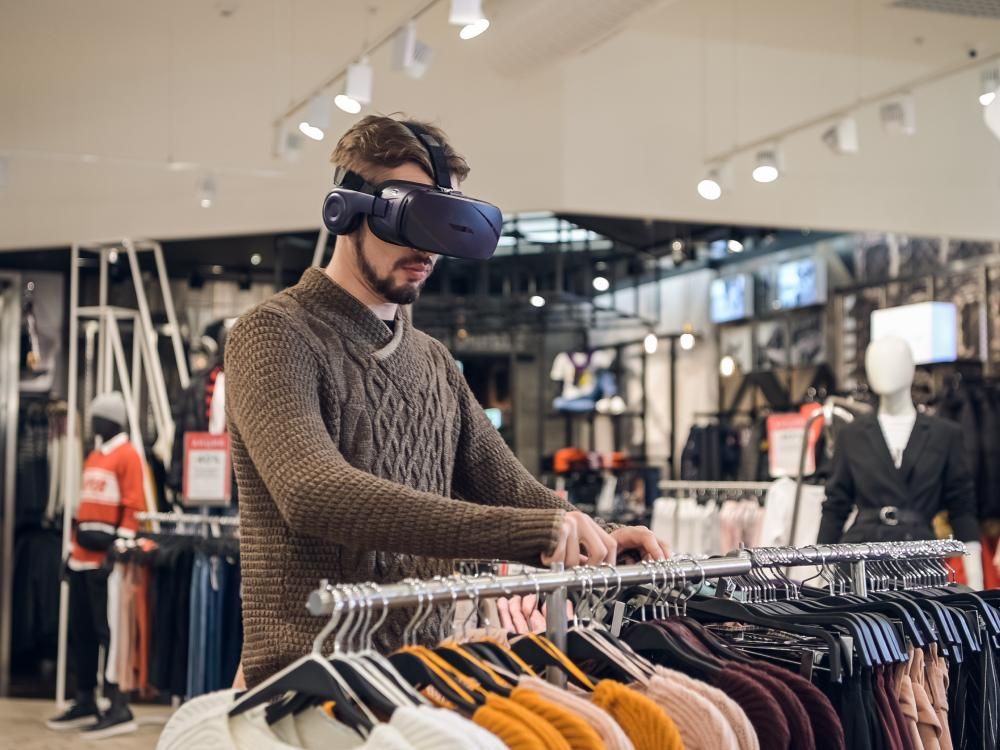
Insights
Virtual reality in retail: 11 use cases, benefits, and adoption practices
Explore the concept of virtual reality in retail, its benefits, use cases, and the best VR adoption practices
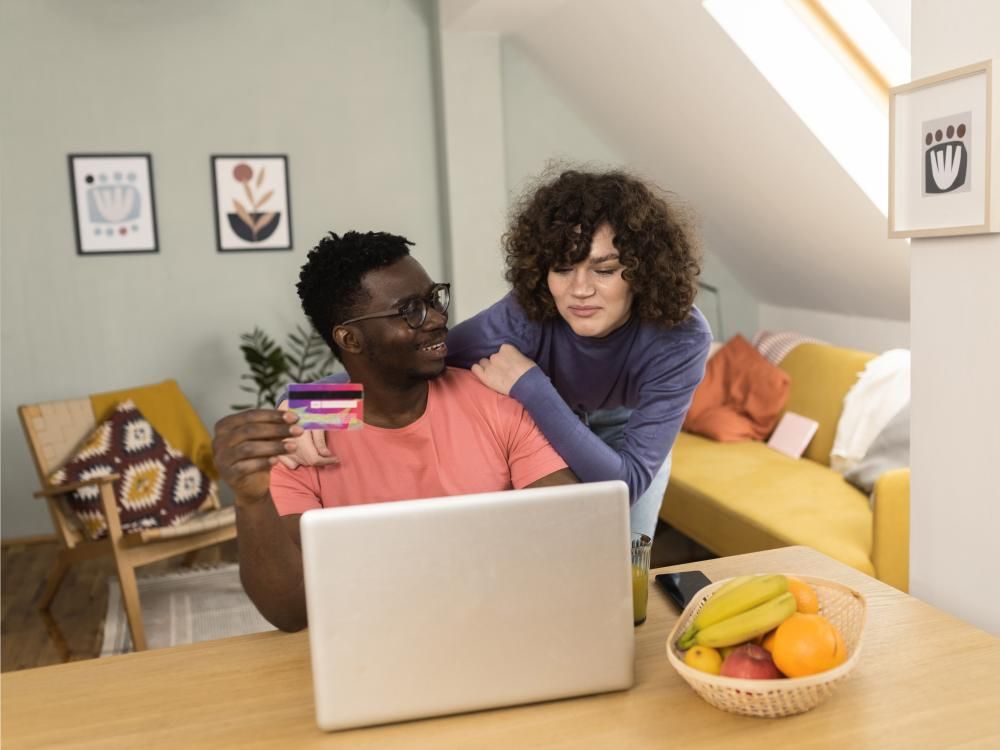
Insights
Ecommerce architecture: which one should you choose?
Selecting a suitable ecommerce architecture isn’t easy, so in this article we cover main ecommerce architecture types and provide tips on choosing between them.
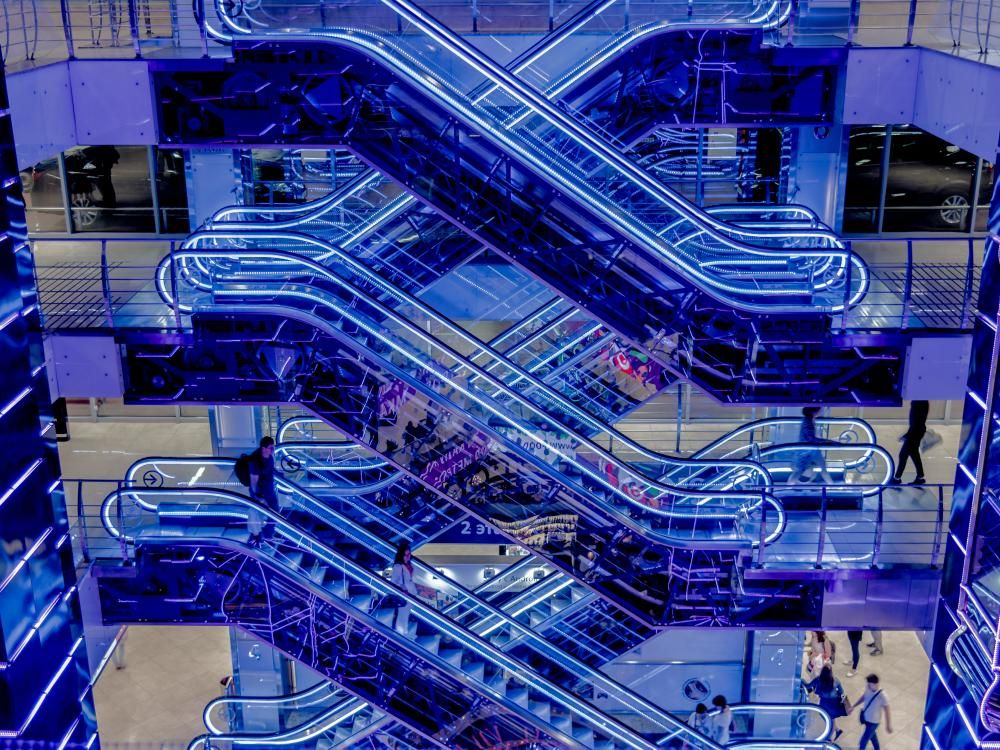
Insights
Machine learning in retail: 10 ways to upgrade your store
Explore the top use cases of machine learning in retail and find out what benefits this technology can bring to your business.
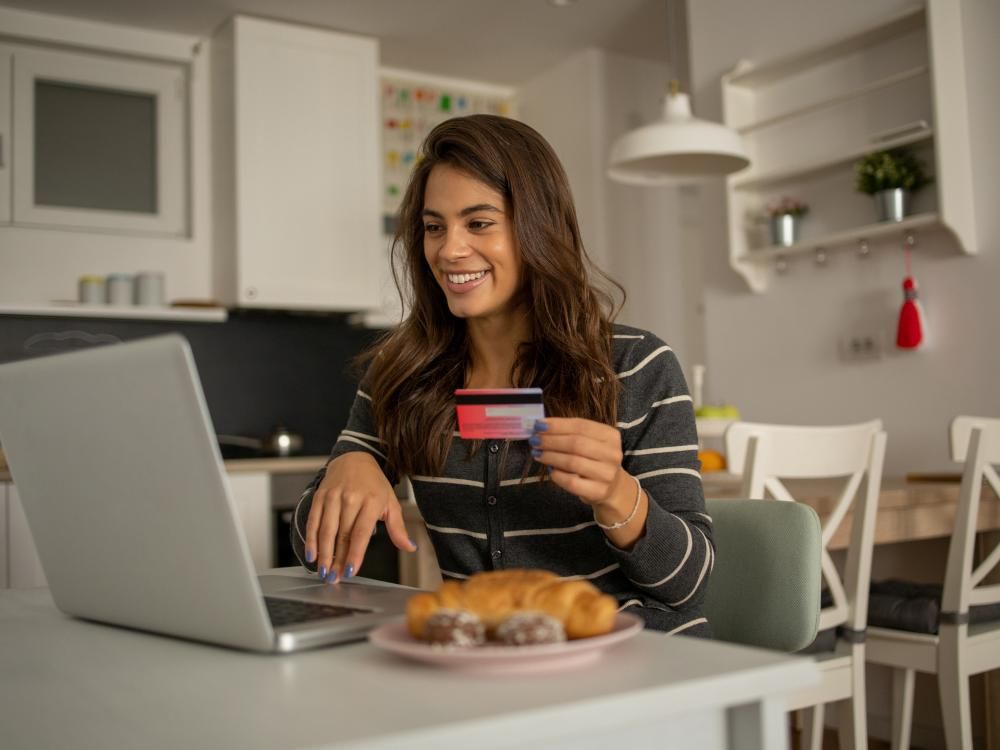
Insights
MACH architecture: components, benefits, and implementation tips
Explore the concept of MACH architecture, its advantages, pitfalls, examples of successful adoption, and implementation best practices.
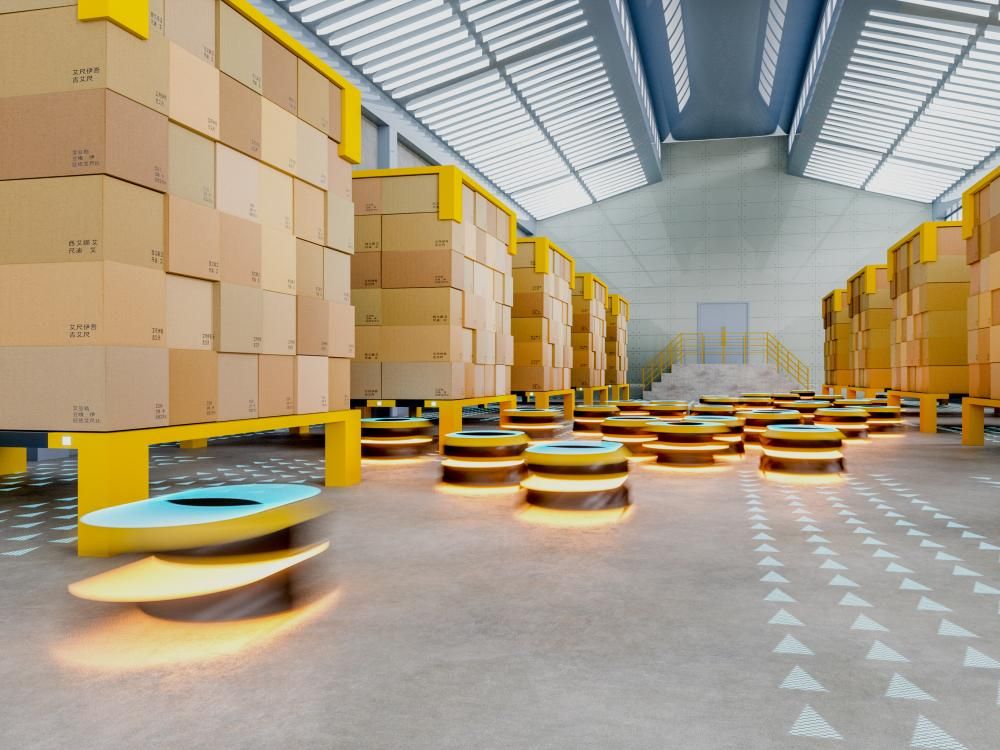
Insights
A guide to making your supply chain smart
Learn what a smart supply chain is and what its key components are and discover how it can transform modern businesses.