Only three years ago, caught off guard by the COVID-19 outbreak, businesses across the globe had to increase the funding for digital initiatives to stay afloat in an unknown and unstable business environment. Multiple surveys show that Covid-19 prompted the digitalization of customer experience, supply chains, products and services, and organizations themselves months or years earlier than expected.
Such rapid transformation has led to companies generating more data than they can process with existing capabilities. When turning to data management services, businesses face the paradox of choice, having to decide between approaches and technologies that are very similar at first glance, like an enterprise data warehouse, data lakes, data fabrics, and other popular data management solutions.
To help you make a sound decision for your company, in this article we shed light on two frequently opposed notions – a data lake and a data fabric.
Data lake, explained
What is a data lake?
Data lakes are repositories storing copies of information collected from various source systems (transactional databases, sensor devices, SaaS applications, file sharing systems, etc.) in its native format for processing by ML solutions, backup and archiving, big data analytics, etc.
How do data lakes work?
Firstly, information taken from a variety of sources enters the landing zone where it temporarily stays in an as-is state. When a company has established continuous ingestion, extraction, transformation, and loading (ETL), and change data capture (CDC) capabilities, then multi-type information can get into data lakes immediately after it is created.
As soon as data is inside the lake, each set is assigned a unique indicator, or an index, and a metadata tag to speed up queries and help users quickly look up the requested data. After that, data may undergo cleansing, deduplication, reformatting, enrichment, etc. and then moved to the trusted zone for permanent storage. When the information is ready to be consumed by downstream users, it may go directly to reports and dashboards or undergo another ETL round and be stored in the data warehouse for further processing. Â
Data lakes may also have separate environments called analytics sandboxes, where data scientists can explore the data. Â
To guarantee the quality, safety, availability, and timeliness of information, companies typically establish a data governance framework, because it helps control data pipelines at each stage.Â
Why choose a data lake?
These schema-agnostic repositories are gaining ground and are unlikely to lose their positions due to many reasons, including:
- Data lakes help consolidate near-infinite volumes of all sorts of information quickly since you don’t have to model and process the data a certain way before storing it. Data lakes are also more affordable solutions, compared to building data warehouses, which allow you to collect all possible data just in case, even without knowing where you will apply it.
- A data lake works well with a data warehouse, as it performs the cumbersome data transformation and saves data warehouse resources for analytics.
- Data lakes are easy to integrate with Hadoop and similar technologies, and that’s exactly what data scientists praise data lakes for. Due to this, they can deploy ML models in the lake and run advanced algorithms there.
- Data lakes may function as an always-on data archive and backup. With high availability and fault tolerance available by default, they are good for storing data that is old or unused for some reason.
Data lake limitations
Sometimes, data lake initiatives fail to succeed because of the following reasons:
- Being encouraged by the almost unlimited data consolidation capabilities of the data lake, companies end up just piling up all available data, hoping to do something meaningful with it down the road. Without a solid framework for creating, enriching and managing metadata, it’s likely your data lake will become a data graveyard, leaving you no chance to understand the data you have and how to make sense of it.
- Traditionally, data lakes are hard to secure and support to achieve the required regulatory compliance. You’ll need to put a lot of effort into securing and enforcing data governance to minimize the risk of information disclosure as well as fines and penalties for not abiding to data protection regulations.Â
Looking for a vendor to deliver a tailored data management solution?
Data fabric, explained
What is a data fabric?
Data fabric is a design approach that implies combining complex components of data ecosystems into a unified platform to provide complete and cohesive data management. Unlike a data lake, a data fabric doesn't require moving data into a centralized location but instead relies on robust data governance policies to achieve data management unification.
A data fabric is a more advanced solution, relied on by companies that want to improve their existing data processes. As a rule, they already have leveraged some kind of a data store, ETL solution, maybe a data catalog or a data protection software. Information is never static, so its types and volumes change. While you may want to move some of your information to the cloud, you may also feel it’s time to integrate your SaaS applications into analytics workflows and grant more freedom to business users in a secure way. But how do you manage all that data without compromising the quality and safety of information? This is where the data fabric concept comes in.
How does data fabric work?
To facilitate accessing information across disparate systems, managing its lifecycle, and exposing it to end-users, data fabric architecture supports:
Data integration
Any information regardless of its type, volume and location can be consolidated and accessed by users, as data fabric allows the leveraging of a data virtualization layer which consolidates data without moving it and creating numerous copies. Besides that, to ensure data integrity, data fabric may employ ETL, CDC, stream processing, etc.
Smart data catalogs
Data catalogs are detailed inventories of all data an enterprise has. As data fabrics unify huge volumes of information, data catalogs maintain the metadata to help data consumers, including analysts, database engineers, scientists, business users, etc., find and understand data, track its lineage, evaluate and govern it, and much more.
Dynamic metadata management
A data fabric typically employs AI capabilities which help automatically detect, analyze, collect and activate metadata.
Data governance
Data governance ensures that data consumers get access to only high-quality information they need with the help of respective policies (access policies, masking policies, data quality policies, etc.), which are automatically enforced due to the metadata activation capabilities.Â
Reasons to adopt a data fabric
As you can see, data fabric is not something you implement instead of a data lake, but rather an evolution that occurs  when you:
- Recognize the impossibility of physically consolidating your information in a single store without creating data silos.
- Want to unify data management, governance, analysis, etc. across your distributed data landscape to simplify information ingestion and quality management while democratizing data access.
- Seek ways to maximize the performance of the existing technology environment without structurally rebuilding it as well as future-proof it to ensure it’ll sustain increasing volumes of information, new analytics requirements, etc.
- Want to create a self-service data marketplace.
Maximize your data value with Itransition
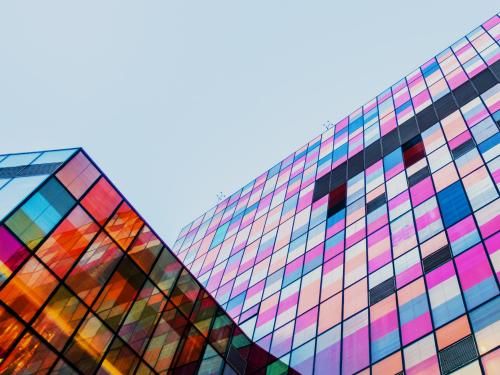
Data management services
We will help you analyze your operational and strategic goals to identify the optimal data management solution and implement it within the set time and budget.
Why you should be conscious of data fabric solutions
No mature technology solution
Although its global market share is forecasted to grow, data fabric is still an emerging design concept, with no mature technology solution so far.Â
While you may put together separate solutions to enable a comprehensive data fabric functionality, Gartner places data fabric to the Peak of Inflated Expectations stage, which means that its mainstream adoption is expected in no sooner than five years.Â
Insufficient cooperation of IT and business users
In terms of tech expertise, the data fabric project requires IT specialists who are well-versed in ETL tools, microservices architecture, cloud services, SQL and NoSQL, Hadoop and alike, Python, Java, etc. However, the data fabric project should not be a purely IT project, because otherwise you will waste your money. End-users must also be involved, especially at the data fabric requirements definition and solution rollout stages.
Afterword
As is evident, there can be no winner in the data fabric vs data lake debate, as both have their ups and downs and more importantly, serve different purposes, thus can be used as complementary solutions. If your current methods of managing data with a data lake and data warehouses fail to deliver the needed result, consider tapping into a data fabric. Although your current data repositories will remain important components in your data landscape, the incorporation of the data fabric approach would bring more agility into business operations and help you keep in step with current digital transformation trends.