Know what your customers want most and what your company does best. Focus on where those two meet.
This quote epitomizes the essence of successful sales. Effective marketing has always been about recognizing and anticipating customer needs. With the increasing application of big data, artificial intelligence, and machine learning in marketing, identifying customer expectations has become easier than ever.
This technological expansion has provided marketers with powerful tools to make data-based assumptions about customer preferences and buying patterns. Today, predictive analytics experts help companies reduce risk and improve sales by answering mission-critical questions, such as:
“Who is my ideal target buyer?”
“What items do they typically look for?”
“How do they interact with my brand?”
“What might they need next?”
To understand how companies can gain that intelligence with technology, let’s take a step back to define predictive analytics solutions for marketing and understand how they work and how they are applied on the ground.
What is predictive analytics?
Predictive analytics in marketing means using data mining, predictive modeling, and machine learning to predict marketing trends, customer behavior, and campaign outcomes. By granularly analyzing huge amounts of both customer and market data, predictive analytics helps marketers to understand why something has happened in the past and what can be done to improve those outcomes in the future.
Stages of data analytics maturity
To get a better understanding of predictive analytics in marketing, let's turn to an iconic graph published by Gartner that illustrates the various stages of data analytics, including predictive analytics.
All of the presented stages (or types) allow companies to traverse the path from raw data to meaningful business insights, each offering unique outcomes and serving a different purpose:
Descriptive analytics Descriptive analytics is used to describe the current and past states of marketing campaigns, customer attrition, retention, or sales KPI. |
Diagnostic analytics Unlike descriptive analytics that allows companies to discover what happened, diagnostic analytics examines data to determine why it happened. |
Predictive analytics Predictive analytics builds on the findings of descriptive and diagnostic analytics to predict certain marketing outcomes. |
Prescriptive analytics Prescriptive analytics assigns what to do to prevent a future problem or capitalize on a potential opportunity. Prescriptive analytics processes only historical data in addition to external information and a variety of complex tools and technologies as sources for recommendations. |
Benefits of predictive analytics in marketing
Accurate trend prediction |
By analyzing large amounts of customer information, social media sentiment, and market data, marketers can predict industry trends earlier than their competitors. |
Intelligent customer segmentation |
Machine learning models can find hidden relationships between customers’ data points and make better clustering decisions. |
Efficient lead prioritization |
With the help of predictive analytics, marketers can quickly spot the most promising marketing prospects and be sure that they convert. |
Effective campaign modeling |
Predictive analytics allows companies to foresee the outcomes of a marketing campaign and make relevant decisions faster. |
Improved personalization of customer experience |
By analyzing historic customer data, marketers can better understand each customers’ needs and wants, create personalized messages and offers, and thus improve customer engagement. |
Streamlined churn prediction |
Marketers can use predictive analytics to detect reasons for customer churn, spot at-risk customers, and take proactive measures to reduce the current attrition rate. |
7 examples of predictive analytics in marketing
Predictive marketing is hardly a new phenomenon. Individual methods and applications have been around for years, used by savvy marketers to enhance products and improve the targeting of their marketing campaigns. However, in recent years, we have seen a remarkable evolution of data science and analysis, accompanied by the development of supporting technologies such as cloud computing, which have made predictive analytics accessible and affordable for almost every business.
Predictive marketing analytics can aid modern marketing teams in the following aspects:
1. Product development
What if a company could accurately predict what products will be in demand in the future ahead of its competitors? Before, such ideas were rightfully considered fantasies, but currently, predictive analytics is exactly what industry leaders use to win over their competitors.
Example: L’Oréal and Synthesio
For example, L’Oréal, the world’s leading cosmetic brand, uses an AI-enabled consumer intelligence platform developed by Synthesio to stay ahead of beauty trends and augment its product development with predictive analytics. To maintain leadership in such a competitive industry, L’Oréal would have to predict beauty trends at least 6 to 18 months before they emerge.
Synthesio’s AI platform collects data from over 3,500 online sources including all popular social media platforms, YouTube, fashion blogs, and beauty forums. By analyzing millions of beauty-related data points, L’Oréal can detect product ingredients and packaging, as well as lifestyles that will be trendy in the near future.
2. Customer segmentation
With predictive analytics-enabled customer segmentation in place, an ML model can automatically cluster customers based on a myriad of data points instead of marketers that traditionally spend hours manually doing so.
Example: Aydinli and Acquia
For example, Aydinli, a large brand distributor operating in Asia, the Middle East, and Europe turned to Acquia, a digital experience company, to quickly and accurately determine audiences for their targeted campaigns.
Acquia’s state-of-the-art machine learning models identified behavioral- and product-based clusters, allowing Aydinli to segment customers into high-returners, digital-only buyers, and other categories. As a result, Aydinli earned an additional $50,000 per campaign and achieved a ROI of more than 3,500%.
3. Uplift modeling
Today, accurately predicting the outcome of marketing campaigns is an essential marketing skill that heavily relies on data crunching. Assisted by machine learning models that are actually much more efficient when processing huge amounts of data, marketers can substantially decrease the time it takes to model campaign uplift.
Example: IDT and Optimove
For example, IDT, a telecommunication and financial services business, turned to Optimove, a company that helps brands optimize their marketing campaigns with the help of predictive analytics and AI, to personalize individual customer messages based on their history, language, and previous campaign responses. Optimove’s predictive analytic-enabled solution allowed IDT to determine campaign uplift within just 2-3 days instead of weeks.
With the help of Optimove’s predictive customer model, lifecycle segmentation, and churn forecasting models, IDT marketers were able to increase the number of customers purchasing new services by 50% and achieve a 17% increase in the lifetime value of active customers.
4. Recommendation systems
A recommendation engine is one of the most widespread use cases for predictive analytics in marketing. By accurately predicting what customers want to watch, listen to, or buy next, some of the world's biggest companies like Amazon and Spotify have conquered their respective industries.
Example: Itransition
Itransition helped a big multinational ecommerce company to adopt an AI- and computer vision-powered recommendation engine that provides customers with personalized experiences and improves customer engagement.
Using a collaborative filtering algorithm, our engine can sift through large sets of customer data to figure out what next product a given customer is the most interested in. As a result of the system implementation, our customer saw an 8% increase in visitors-to-buyers conversion rate.
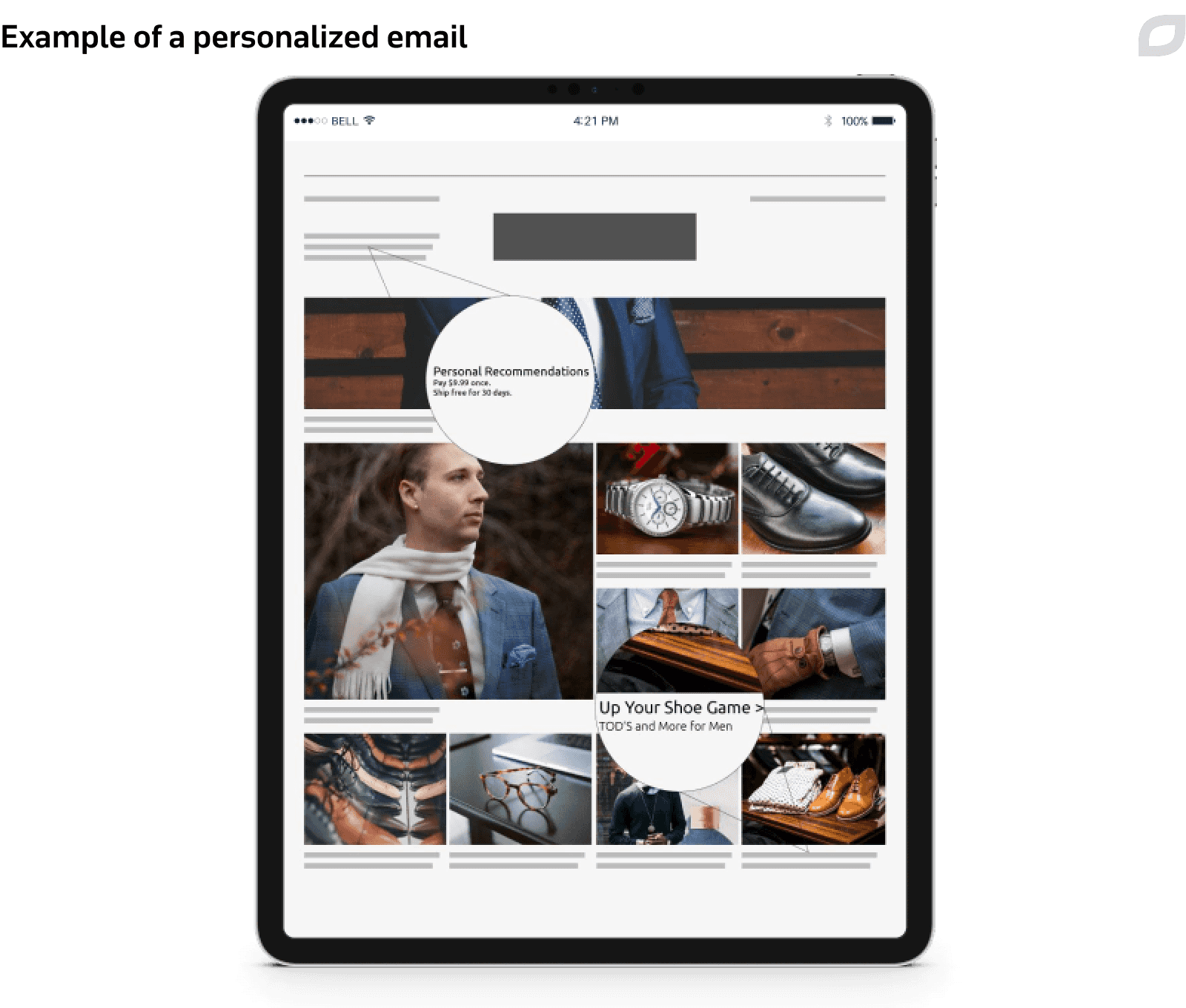
5. Lead prioritization
Traditionally, an in-house marketing team needs to manually analyze user data to prioritize leads. This laborious process takes a substantial amount of time, which leads to delayed decisions and, consequently, missed opportunities to strengthen relationships with potential customers. Predictive analytics can accelerate this process significantly, resulting in a more reactive decision-making process and increased conversions.
Example: WNS
WNS, a company that uses data analytics to improve companies’ business outcomes, helped a leading digitally-native company to improve the conversion of potential leads with the help of a predictive analytics platform. The machine learning-based model categorized leads as hot, warm, and cold, with 'hot' having the highest chance of conversion. This allowed the marketing team to immediately detect high-quality leads, nurture them, and significantly improve customer engagement. As a result, the company increased lead conversion by 38%.
6. Churn prediction
In the marketing realm, churn rate is an essential measure of customer satisfaction. The correlation is pretty straightforward: the higher the churn rate, the lower the customer satisfaction. Applying predictive models, marketers can accurately estimate the probability of churn for a given customer in real time and take proactive measures to prevent it.
Example: Lityx
For example, Lityx, a company that offers AI-based analytics solutions to organizations in a wide range of industries, assisted a retail firm to predict customer behavior. The retail company noticed that many customers wouldn't return to the website after a single visit. They wanted to identify these at-risk customers early and take proactive measures to decrease churn.
Based on the retail firm's data on customer demographics, line-item transactions, marketing activity, and other metrics, Lityx created multiple predictive models that help marketers estimate the probability of winning back customers that didn’t return to the site. As a result, the customer saw a 330% increase in the accuracy of spotting at-risk customers and a 265% boost in predicting re-purchase behavior following first visits.
7. Ad personalization
In the marketing realm, the level of personalization is directly correlated with click-through rates (CTR), and, consequentially, ad performance. Crafting high-performing creatives for each audience and each campaign is a highly resource-intensive process, to say the least. With predictive analytics, it’s possible to use real-time consumer data to deliver personalized ad campaigns at scale.
Example: IBM and Mastercard
For example, Mastercard used IBM Watson Advertising Accelerator to boost awareness about their partnership with ‘Stand Up to Cancer’ and their campaign to donate $4 million to fund cancer research. Mastercard’s marketing team used IBM’s AI-based platform to detect the most engaging creative elements for each target audience based on location, device type, and time of day.
Apart from an impressive 144% increase in the click-through rate, Mastercard’s marketing team learned valuable insights about creative generation overall. For example, unique and provoking CTAs like ‘Start Something Priceless’ resonated with customers the most, while generic CTAs like ‘Learn More’ were much less impactful.
The stages of predictive marketing analytics implementation
Every data analytics project is unique, not only due to the high level of customization in each case, but also because of the distinctive data sets that each company uses as input. However, most implementations of predictive analytics for marketing follow a workflow similar to the one presented below.
1. Project definition |
This stage is about determining all the essential ingredients of the business intelligence project plan. It is vital to establish the expected outcomes and deliverables, available data input and resources, and specialists required to execute the implementation. As the outcome of this stage, a company should have a clear understanding of what it hopes to achieve when the project is completed. |
2. Data collection |
At this stage, the adopting company gathers all the data they consider useful as input for its predictive analytics project. Since data processing is the essence of predictive analytics, choosing the right types, scopes, and sources of data is critical for a positive outcome. For instance, if a predictive analytics project aims to refine the segmentation for lead nurturing campaigns, it needs to operate on demographic and behavioral data collected from a variety of sources. These sources include traditional tools for collecting customer information, such as CRM systems, face-to-face interactions, or over-the-phone engagements, as well as social media conversations, and data from wearables and other customer IoT devices. It is important to know that most of the time data is collected into a data lake and stored there in a raw, unprocessed state, which brings us to the next phase. |
3. Data processing |
Before any insights are derived from it, the supplied data needs to be scrubbed. It is quite a tedious and resource-heavy process, which however can have the greatest impact on the project results. Data gathered at the previous stage is typically rather heterogeneous. It can be structured (e.g. aligned in a neat table, with sections and labels in place) but most of the time it’s unstructured, with some attributes missing and in various formats (audio, video, text). Such unstructured data is difficult for machines to process, so it needs to be processed before algorithms can digest it. Compliance is another aspect to be considered. Especially in the context of a marketing project, it is of crucial importance to ensure strict observance of data protection laws when processing personal or sensitive information. |
4. Modeling |
Modeling is a core part of a data analytics project - it’s where the magic happens. This process refers to the creation and extension of data models that define how an organization should collect, update, and store data. Models are used to understand the logic within data and formulate predictions based on the drawn conclusions. You may picture them as abstract graphs that organize data sets and determine the relationships between them. They range from linear models that work with two correlated variables to complex machine learning neural networks that are capable of independently processing large data volumes to identify subtle correlations and make conclusions using them. |
5. Interpretation |
Once various statistical algorithms and models have processed data, the output can be interpreted and presented to answer the business questions asked at the beginning of the project. Visualization is applied to ensure a better understanding of the predictions. Finally, the obtained intelligence should be translated into actionable steps and incorporated into strategic marketing planning. |
Unlock marketing value with data-based predictions
A few years ago, advanced data analytics powered by AI was only accessible to large enterprises. The implementation cost was inhibitive, and there was a lack of scalable, affordable solutions for companies to use for the development of predictive analytics tools that could cater to their needs.
Fast forward to today, and predictive analytics has become a crucial tool that can transform every aspect of marketing with data-based predictions, from lead generation to churn prediction. Thanks to smart data analysis, marketers can discard useless data and use only valuable insights to power their sales and marketing strategies. By tapping into these benefits, they strengthen the bond with customers and secure sustained growth.