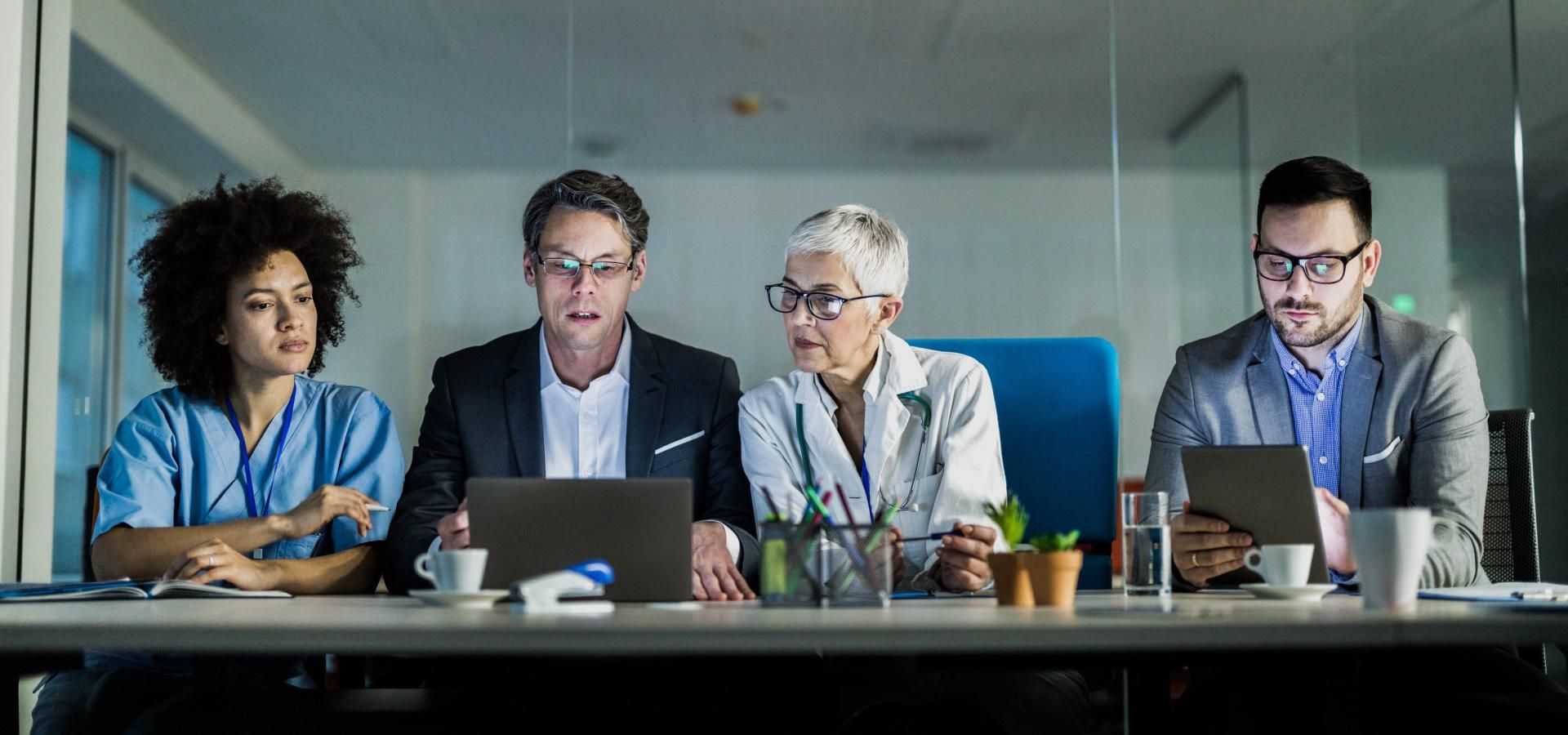
Predictive analytics in healthcare: top use cases & adoption tips
February 13, 2025
- Home
- Predictive analytics
- Predictive analytics in healthcare
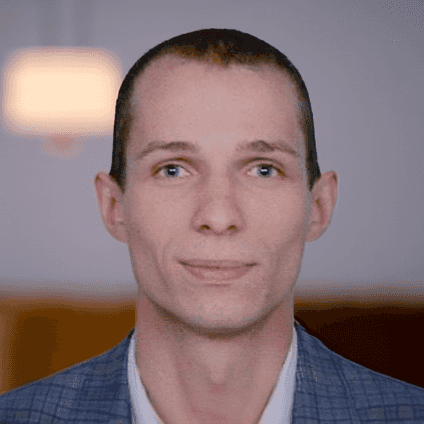
Head of AI/ML Center of Excellence
With solid experience in predictive analytics software development and implementation, Itransition’s experts help healthcare providers create custom solutions tailored to the latest industry trends and regulatory requirements.
Table of contents
Predictive analytics’ market stats
of healthcare leaders in the US have adopted
Statista
expected CAGR of the global healthcare predictive analytics market by 2030
Next Move Strategy Consulting
Top use cases for predictive analytics in healthcare
The integration of predictive analytics into the workflows of healthcare organizations opens up new possibilities for proactive and personalized care across the industry.
Predictive analytics helps healthcare professionals spot trends in patients’ information and medical records that are impossible to detect manually, helping them understand patient conditions better. Predictive modeling, in particular, allows forecasting the course of the disease, enabling medical professionals to avoid health risks like adverse reactions to medicine, genetically determined resistance to treatment, and failure to adhere to the regimen. This way, clinicians receive actionable insights that help them optimize individual patients’ treatment plans according to their physical and psychological needs.
Benefit
Scheme title: How to combine precision medicine and predictive analytics for personalized care
Data source: ncbi.nlm.nih.gov — Precision Medicine, AI, and the Future of Personalized Health Care
Avoiding readmissions
According to Medicare's Hospital Readmission Reduction program, healthcare organizations are subject to penalties in case of patient readmission within 30 days after receiving care. Therefore, reducing hospital readmission rates will improve patient satisfaction and save the funds of healthcare providers.
Predictive analytics helps identify patients with a high risk of readmission after a particular treatment. Predictive analytical tools usually use electronic health records (EHR) data to define which factors are most likely to increase patients' risk of readmission within 30 days. Care providers can use this information to make more informed clinical decisions to avoid readmission, like scheduling more frequent follow-up visits, optimizing discharge instructions, and prescribing additional medication.
Benefit
Developing new treatments
Medical researchers are turning to predictive modeling and analytics to augment traditional clinical study processes. For example, silico testing implies using extensive quantities of real-time and historical data from multiple sources as a basis for a predictive model assessing the therapy’s impact on patients. As a result, the derived insights are used to lower the risks for study participants and reduce the treatment research costs for researchers and manufacturers.
Benefit
Scheme title: Individualized predictive modeling for in silico clinical trials
Data source: arxiv.org — Artificial Intelligence for In Silico Clinical Trials: A Review
Enhancing care for patients with chronic conditions
Another common use of predictive analytics in healthcare is helping clinicians make better decisions when providing long-term, palliative, and other types of care for patients with chronic diseases. Predictive models help identify patients at risk of developing certain complications or adverse drug reactions and recommend adjusting their treatment plans to avoid adverse patient outcomes. Data analytics can also help spot patients likely to develop chronic conditions in the first place and suggest an early intervention. A scientific paper published in the Journal of Healthcare Engineering shows that using sophisticated data mining and machine learning algorithms such as convolutional neural networks (CNN) and K-nearest neighbor (KNN) can improve the accuracy of chronic disease prediction to 96%.
Benefit
Scheme title: Comparison of accuracies of Naïve Bayes, decision tree, logistic regression, and CNN & KNN algorithms
Data source: ncbi.nlm.nih.gov — Identification and Prediction of Chronic Diseases Using Machine Learning Approach
Streamlining inventory management
With predictive analytics tools, clinicians can discover specific trends in resource usage and foresee the facility’s upcoming needs. This can enable healthcare administrators to buy the required medical goods or redistribute them in a timely manner to prevent stockouts. Besides, hospitals can employ predictive analytics to determine what resources are prone to be out-of-stock faster than others, relying on trends among the population or seasonal needs. These insights help develop strategies to cut expenses, maintain affordable healthcare costs for patients, and reduce medical waste.
Benefit
Improving patient engagement
Predictive analytics offers healthcare providers deeper insights into patients and their needs. For example, Community Health Network from Indianapolis, Indiana, uses predictive analytics models to reduce no-shows. Their analytical tools help identify no-show risk factors based on patients’ demographic characteristics and develop special workflows to make sure patients confirm their appointments. Healthcare data analytics can also help pinpoint which line of conduct would resonate with certain patients or patient groups. Using this information, providers can design patient engagement strategies, offer personalized medicine, and improve patient outcomes.
Benefit
Accelerating insurance reimbursements
Healthcare organizations can employ predictive analytics tools to analyze claims and understand the likelihood of them being fulfilled or declined, find out which data is missing, and define how to improve the documentation before sending it to the insurer. This helps optimize claim submission and fulfillment processes, fight fraud, and yield higher insurance payments.
Benefit
Anticipating epidemics
A study published in Nature Communications demonstrated the feasibility of predictive analytics for accurate estimation and forecasting of respiratory diseases for locations without sentinel surveillance. Metapopulation models that had already been successfully used to prognose outbreaks of influenza, dengue, malaria, and COVID, were coupled with other analytical tools and employed to forecast when and where additional surveillance would be needed to prevent epidemics.
Benefit
Predictive analytics adoption roadmap
The implementation process varies from one company to another, heavily relying on project scope and specifics. Here, we share a typical roadmap a healthcare organization can adjust according to its specific workflows, goals, and needs.
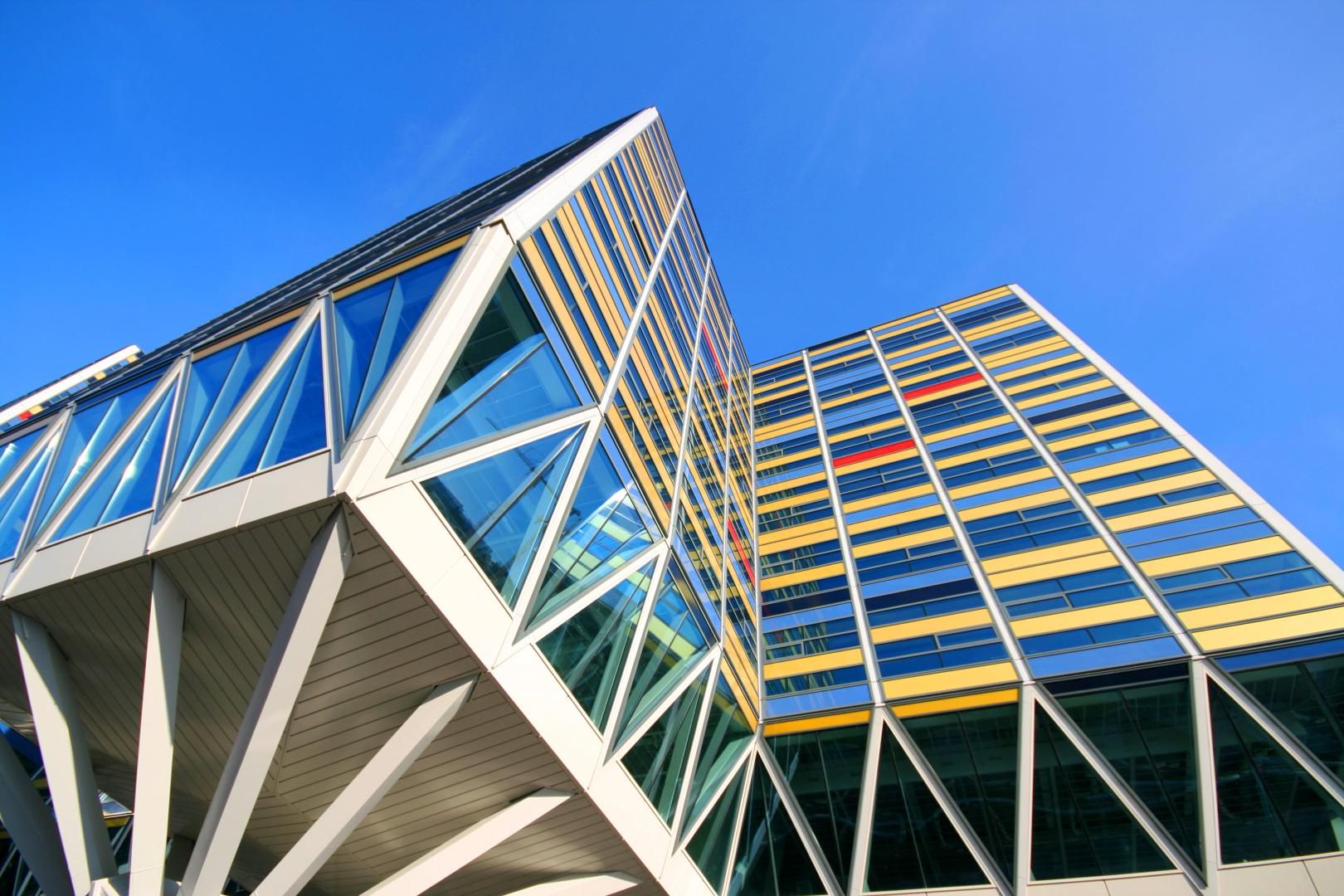
1 Â Requirements definition
The project team identifies healthcare providers’ business needs, objectives, user expectations, and concerns. Then, the specialists assess the technical environment and define functional and non-functional requirements for future analytical solutions.
2 Â Data analysis
The project team then analyzes the current data management workflows and explores available data sources in the healthcare organization and public databases to ensure they meet project goals.
3 Â Design & planning
The data analytics team designs the solution’s architecture and defines the implementation strategy, predictive algorithms, and the suitable tech stack. At this stage, the team also sets the timeline and budget for the project.
4 Â Implementation
Data analysts and engineers process, cleanse, annotate, and transform datasets from various sources, define the evaluation criteria for the analytical solution, and then develop it in line with the implementation strategy.
5 Â Integration & deployment
The expert team integrates the solution with other health systems, deploys it to the production environment, and transfers the technical and business documentation to ensure the solution’s correct operation and maintenance.
6 Â Support & maintenance
The support team carries out model retraining and functional enhancements based on user feedback and new data from the production, as well as supports the system’s operability under heavy loads.
Ready to successfully set up an analytical solution?
Adoption challenges & how to solve them
When implementing analytical software, healthcare providers often face challenges related to technology, infrastructure, security, and employees’ resistance to change. As an experienced implementation partner, Itransition's team has developed a set of recommendations to help healthcare organizations address these and other challenges.
Challenge
Possible solution
Healthcare providers often deal with large volumes of inconsistently structured data. Incorrectly transferred and aggregated big data can lead to errors, duplicates, and, ultimately, faulty predictions.
Healthcare providers often deal with large volumes of inconsistently structured data. Incorrectly transferred and aggregated big data can lead to errors, duplicates, and, ultimately, faulty predictions.
To effectively consolidate all healthcare data in a centralized repository and ensure uninterrupted and accurate data collection, healthcare providers have to enable seamless integration between all their health systems, including EHR, CRM, LIS, wearable devices, medical imaging systems, and other sources that feed health data to the analytical software.
Data privacy remains a concern when it comes to collaboration between organizations or data exchange within one health system. Besides, population health management studies require data anonymization to ensure its safe use by any organization. This helps protect patient privacy while allowing valuable insights to be drawn from the data.
Data privacy remains a concern when it comes to collaboration between organizations or data exchange within one health system. Besides, population health management studies require data anonymization to ensure its safe use by any organization. This helps protect patient privacy while allowing valuable insights to be drawn from the data.
To ensure collected data is suitable for predictive modeling while complying with HIPAA regulations, healthcare organizations should:
- carefully consider the legal side of the agreement with a vendor regarding the non-disclosure procedure and penalties for its breach
- identify a person who will be responsible for the transmitted data and its obfuscation before transmission
- ensure that all staff members involved in data handling receive proper HIPAA training
- establish clear protocols for data encryption during transmission and storage
There are many other components to keeping data safe and secure, therefore it's best to discuss every unique case with professional data security consultants.
Some clinicians worry that doctors will rely too much on findings provided by analytical apps, and point to many biases the predictive algorithms have that can skew the results and potentially harm the patients.
Some clinicians worry that doctors will rely too much on findings provided by analytical apps, and point to many biases the predictive algorithms have that can skew the results and potentially harm the patients.
Healthcare organizations should develop a comprehensive analytics adoption strategy, determining its place in the decision-making and the data it will process and produce. Afterward, you need to conduct staff training, clarifying which tasks the system can handle and which are still the responsibility of medical professionals.
The future of predictive analytics
More and more healthcare providers use predictive analytics to enhance clinical decision-making, optimize resource usage, and manage population health and well-being. Analytical software powered with artificial intelligence and ML capabilities can help anticipate patient health risks at large scale, like pandemics, and take action faster and more efficiently than ever before. Its boundless potential enables data scientists, healthcare organizations, and software developers to augment analytical solutions further, opening new opportunities for its use. However, all healthcare industry players must strategically govern their data to keep it relevant, properly formatted, and error-free. Additionally, healthcare organizations must have a fully interoperable IT ecosystem with an uninterrupted data flow between their systems to use predictive analytics software to its full potential.
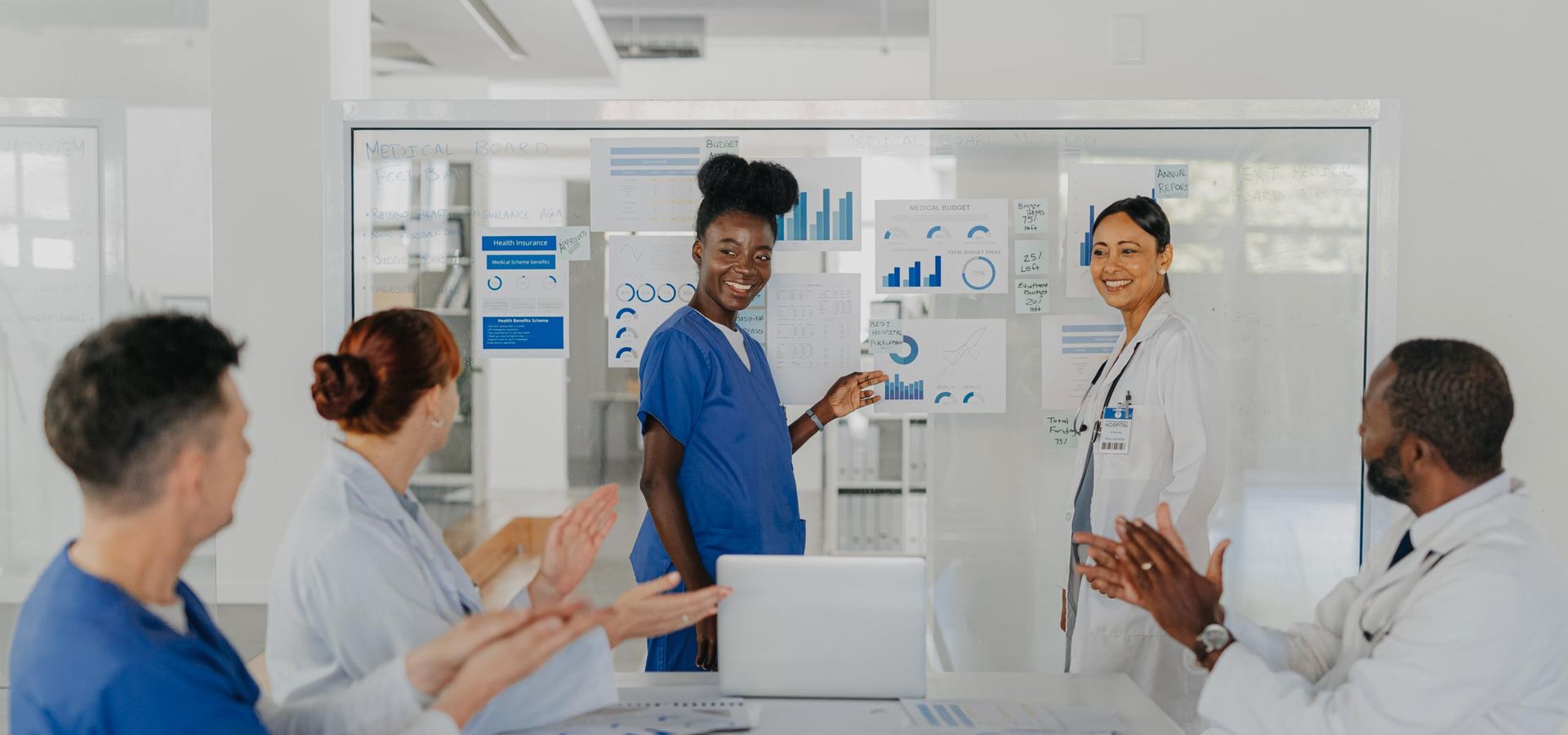
Service
Healthcare data analytics services: key types, features, and applications
Discover how our healthcare data analytics services can enhance patient loyalty while growing revenue and learn which solutions fit your business goals.
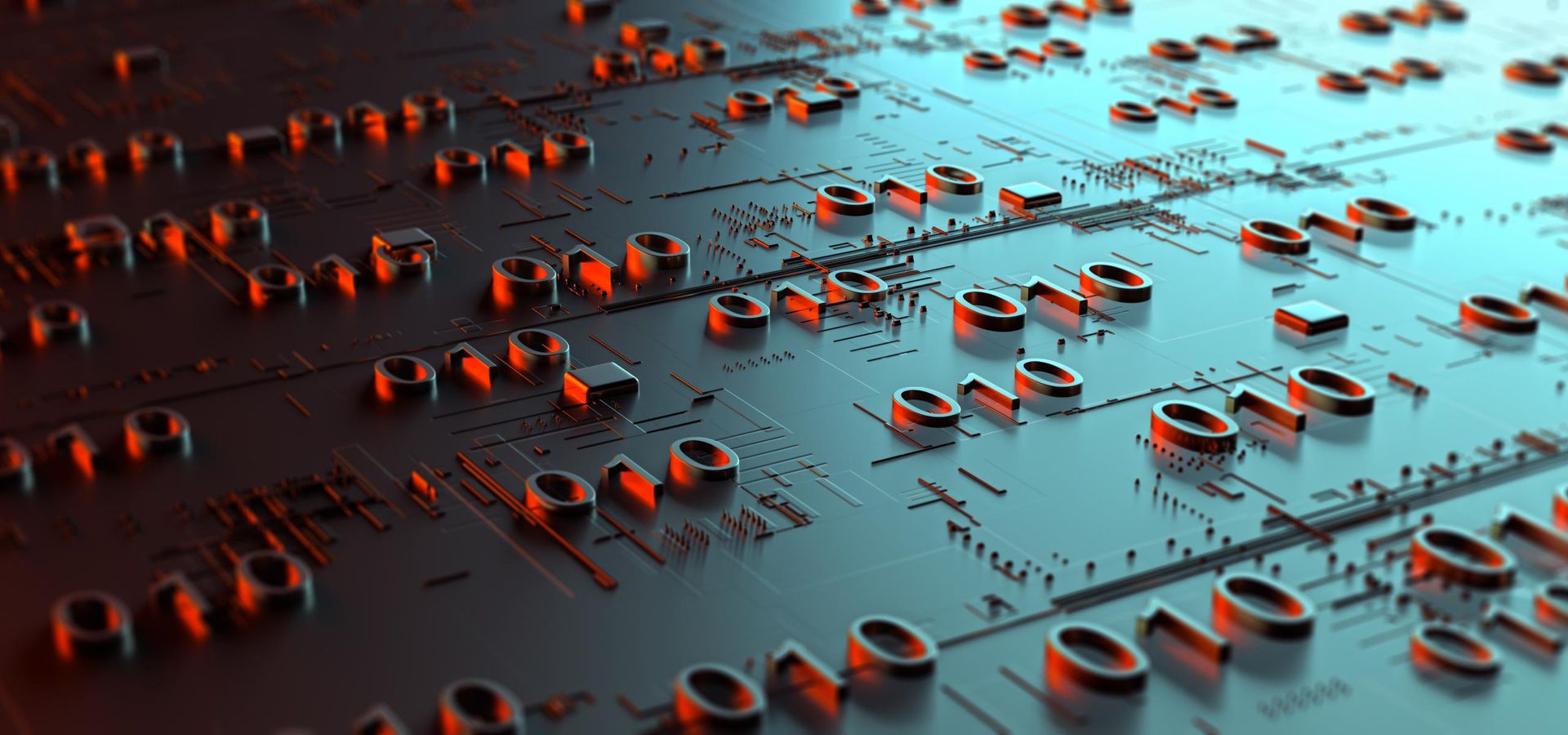
Service
Data analytics services
Rely on Itransition's data analytics services to turn your data assets into business insights and streamline your decision-making.
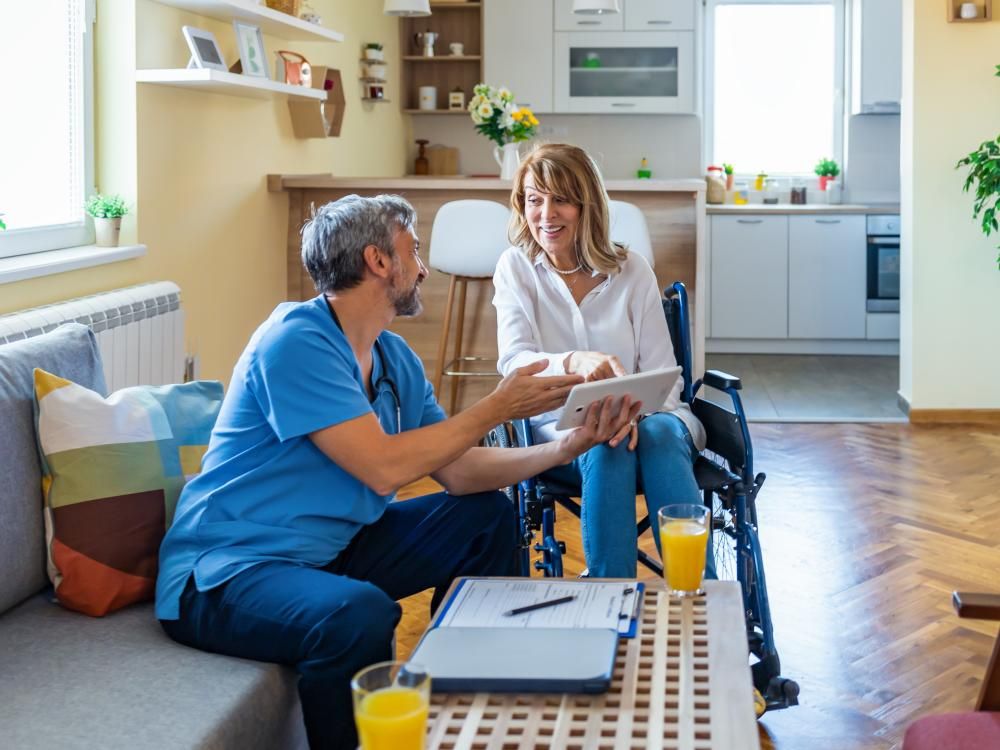
Case study
Oncology treatment platform
Learn how we developed an oncology treatment platform that streamlines therapy order creation and facilitates evidence-based patient treatment decisions.
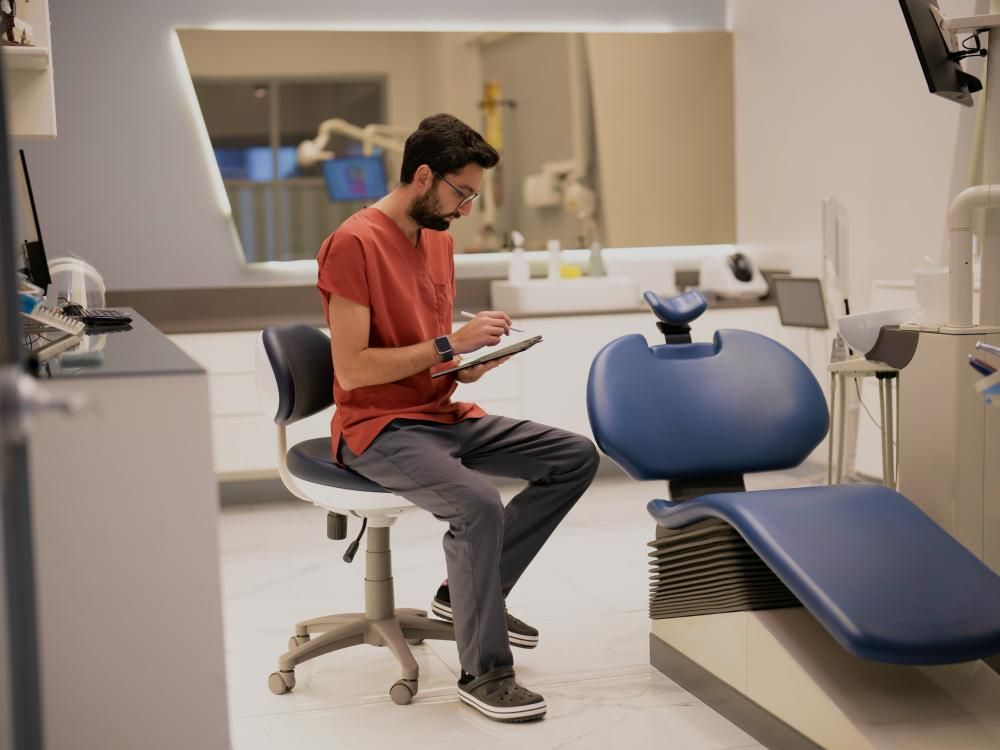
Insights
Business intelligence for healthcare: a full implementation guide
Discover the types and benefits of BI tools for healthcare organizations and learn how to implement a secure solution in your company to avoid common pitfalls.
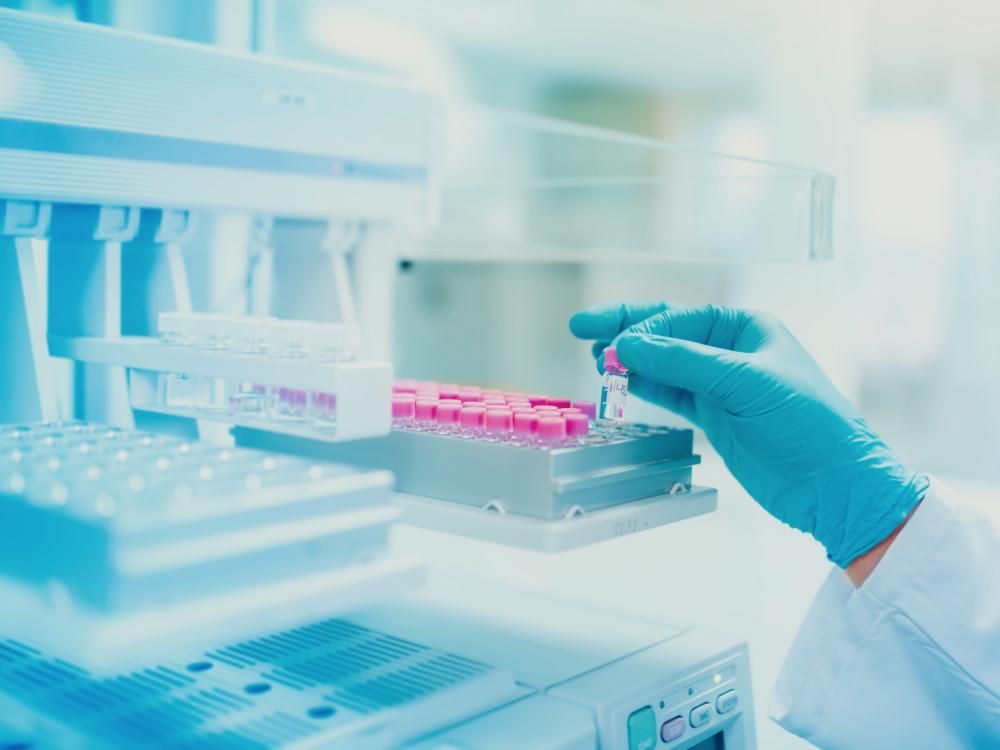
Case study
Pharmaceutical data analytics suite for a US multinational
Read about Itransition’s 10+ years long cooperation with a US-based multinational to create their flagship pharmaceutical data analytics products.
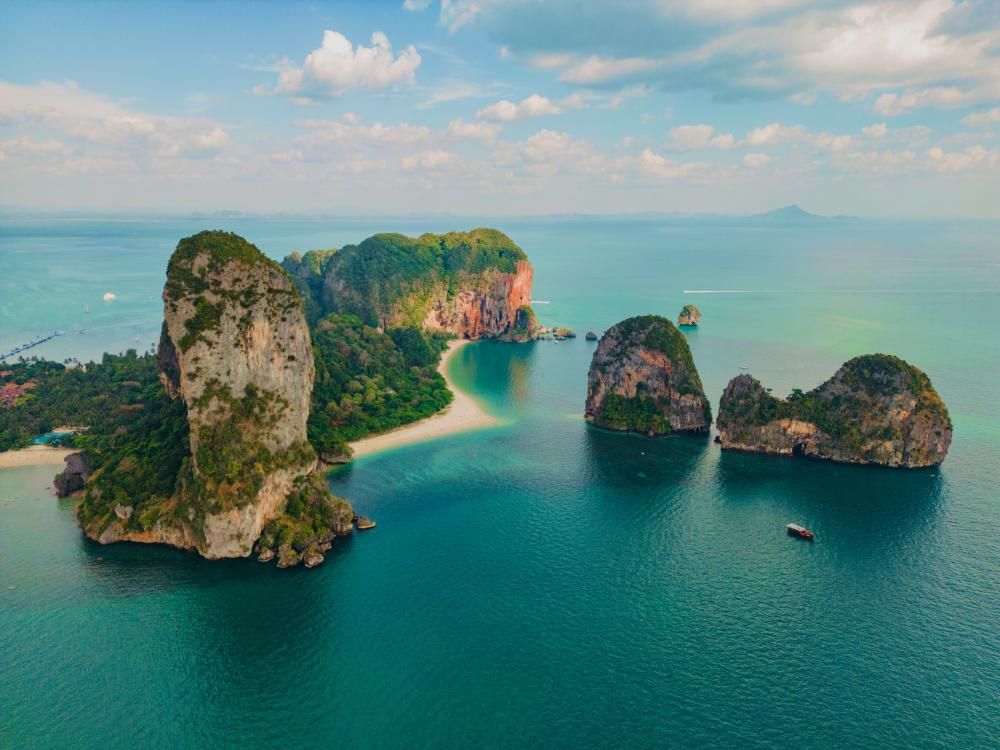
Insights
Healthcare data governance: components, benefits & strategy building
Discover the benefits of a data governance strategy for healthcare providers, tips on its successful implementation, and solutions to possible challenges.